Radiomics of pulmonary nodules and lung cancer
Introduction
The addition of the term “omics” to fields of science originates in basic science. It is now a widely used suffix in clinical medicine research to denote the concept of examining large volumes of complex data to identify precise characteristics or outcomes. In the setting of lung nodules and lung cancer, radiomics is aimed at deriving automated quantitative imaging features that can predict nodule and tumour behaviour non-invasively (1,2).
Pulmonary nodules are a frequently encountered incidental finding on CT, and the challenge for radiologist and clinicians is differentiating benign from malignant nodules. In current practice pulmonary nodules identified on CT are managed according to widely accepted guidelines (3-5). These guidelines recommend that nodules be ignored, kept under surveillance or undergo further testing. The prime driver behind nodule management is nodule size on the basis that the risk of lung cancer increases exponentially with nodule size (6-11). Additionally, a host of subjective visual signs that may predict malignancy have also been described. However, existing guidelines are subject to limitations: ultimately most small nodules require surveillance and it is challenging or even impossible to predict with certainty the likelihood of malignancy from a single CT. Furthermore, nodule evaluation based on visual morphological signs (for example smoothness, irregularity and speculation), are subject to considerable interpretation and variability (12,13) and the number of visually identifiable discriminators in lung nodules is limited. By contrast, in radiomics the number of extractable nodule features is substantially greater and can be identified with far greater reproducibility. For example, in one study of lung cancer patients a total of 440 radiomic features were extracted (14) that were capable of predicting tumor histological subtype from a single time point CT.
The rationale for using radiomics in lung nodules
There are a number of reasons why the application of radiomic feature analysis may be particularly suited to the assessment and management of pulmonary nodules.
Accurately delineating a nodule from adjacent lung parenchyma and other structures is crucial if image features are to be assessed in a reproducible and robust fashion. In the case of pulmonary nodules, the high contrast resolution between pulmonary nodules and lung parenchyma makes them ideal candidates for volumetric segmentation. More work needs to be done however to develop powerful segmentation methods ensuring precise and reproducible nodule segmentation (15).
Another important feature is that within the volume of a pulmonary nodule there is genomic heterogeneity which manifests as subtle differences within the nodules substance that cannot be readily appreciated by the naked eye. Nevertheless, this heterogeneity can be represented with radiomics, allowing for the statistical assessment of image datasets to produce diagnostic, predicative and prognostic models.
Finally, very substantial numbers of lung cancer datasets can be accessed via large inter-institutional databases such as the National Cancer Institute (NCI) Quantitative Imaging Network (QIN) as well as large trails like the National Lung Cancer Screening Trail (NLST). Sufficiently high powered statistical analysis of information extracted from these large cohorts is necessary for the extraction of useful quantitative imaging features.
Radiomic features in lung nodules and terminology
Pulmonary nodule radiomic features can vary considerably in complexity. At the most basic level automated radiomic features aim to replicate features traditionally captured by visual assessment by radiologists such as nodule size, shape and outline. Nodule histogram and texture analysis are more intricate forms of radiomics. A typical histogram analysis of a pulmonary nodule denotes the distribution of densities within the nodule. Entropy for example is a term that describes the randomness of discrete neighboring intensities within a greyscale image while skewness is a marker of the symmetry, or more precisely asymmetry, of a data-set around the sample mean.
More complex forms of texture analysis use mathematical equations to describe the relationship between neighbouring pixels and their distribution through the nodule. For example a widely used form of radiomics called wavelet analysis describes the concept of breaking imaging data down into different frequency components called wavelets and using this data to extract features relating to image texture and intensity.
While subjective correlates of histogram analyses are usually not possible to make with the naked eye, the concept of Hounsfield unit distribution within a region of interest is one that can be readily understood by most radiologists. Even some of the more complex forms of textural analysis which are less easy to understand, nevertheless do have visual descriptive correlates such as ‘coarseness’.
Once features have been extracted, the next process in radiomics is establishing relationships between patterns of features and clinical variables, also known as data mining. This can vary from straightforward statistical analyses based on a priori hypotheses, to machine learning methods. Machine learning is a field of computer science which combines the study of pattern recognition and computational learning theory to construct algorithms that can learn from data and make predictions on outcomes as well as uncover hidden insights. Random forests is an example of a machine learning algorithm which works by constructing a collection of decision trees.
Figure 1 illustrates the types of steps involved in the radiomic analysis of lung nodules
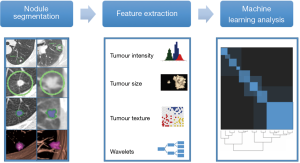
Clinical application of pulmonary nodule radiomics
Differentiating lung cancer from benign pulmonary nodules
Nodule size evaluation
In CT based lung cancer screening and incidentally detected indeterminate pulmonary nodules, a number of studies have shown that radiomics can improve the diagnostic accuracy to discriminate cancer from benign pulmonary nodules.
Nodule size can be more accurately and quantitatively assessed with the use of volumetry software than with manual caliper measurements. Nodule stability and growth rate can also be more confidently assessed by calculating a nodule’s volume doubling time (VDT). Data from the NELSON lung cancer screening trial indicates that the likelihood of malignancy can be estimated according to VDT. For example, nodules with a VDT of <400 days have a chance of malignancy of 9.7% compared to 4.1% for VDTs between 400–600 days. Indeed, this basic form of radiomic nodule assessment has been integrated into the British Thoracic Society (BTS) guidelines for the initial assessment and surveillance of solitary pulmonary nodules (16).
Texture based analyses
A study by Ma et al. illustrated the potentially vast number of radiomic textural features that can be extracted from pulmonary nodules. The authors assessed 127 indeterminate pulmonary nodules and identified 583 features of nodule intensity, shape and heterogeneity. By analyzing these features they achieved 82.7% accuracy in classification of malignant primary lung nodules and benign nodules (17).
In another study, Hawkins et al. assembled two cohorts of 104 and 92 patients with screen-detected lung cancer matched with two cohorts of 208 and 196 screening subjects with benign pulmonary nodules. Image features were extracted from each nodule and used to predict the subsequent emergence of cancer. Twenty three stable features in a random forests classifier could predict nodules that would become cancerous 1 and 2 years hence with accuracies of 80% (area under the curve 0.83) and 79% (area under the curve 0.75), respectively (18).
Radiomic analysis has also been shown to have a role in evaluating subsolid nodules. Studies have identified textural features that are able to differentiate transient inflammatory nodules from malignant part-solid nodules, and also differentiate indolent adenocarcinoma in situ from invasive adenocarcinoma.
Lee et al. assessed textural features of 86 part-solid nodules. They found that low mean attenuation, lower 5-percentile CT number and higher positive skewness of attenuation were significant discriminators of transient from persistent part solid nodules. When texture analysis was used in combination with clinical and CT features differentiating power increased significantly when compared to clinical and CT features alone; the AUC rising from 79% to 92.9% (19).
In another study of 86 part-solid ground glass nodules Chae et al. successfully differentiated preinvasive lesions from invasive lung adenocarcinoma using a combination of texture based features (area under the curve, 0.981) with higher kurtosis and smaller mass being particularly good differentiators (20).
Histological sub-typing
There are a number of studies which have successfully demonstrated an association between radiomic features and NSCLC tumour histology (21,22)
Wu et al. extracted 440 radiomic features from segmented lung tumours on the pretreatment CTs of 350 patients. They observed that 53 radiomic features were significantly associated with tumor histology. By using a combination of wavelet based feature analysis tumour histological subtype could be reliably predicted (AUC 72%) (14).
One application (known as CANARY) has been specifically developed for the pathology evaluation of lung nodules. This radiomics tool has been shown to non-invasively risk stratify lung adenocarcinomas into aggressive (invasive adenocarcinoma) and more indolent forms (adenocarcinoma in situ and minimally invasive adenocarcinoma). Through the use of cluster analysis, CANARY aims to reduce the wide and complex pattern of voxel densities seen within pulmonary nodules down to nine representative so-called exemplars. These exemplars correspond to the predominant histopathology across the lung adenocarcinoma spectrum (23).
These results highlight the impressive long-term potential of non-invasive and cost effective radiomics tools for informing treatment choices and personalized therapy for lung cancer patients.
Gene expression-radiogenomics
There is emerging evidence that radiomics can be useful in the underlying gene expression profiling of NSCLCs and has been used to predict EGFR and KRAS mutation status in NSCLC. Currently the genetic subtyping of lung cancers often requires biopsy and re-biopsy of lung nodules often with multiple samples taken. The ability in the future to accurately predict genetic labels from CT offers the potential for enormous clinical benefit.
Liu et al. retrospectively evaluated the capability of CT-based radiomic features to predict epidermal growth factor receptor (EGFR) mutation status in 299 surgically-resected peripheral lung adenocarcinomas. Statistically significant differences were found in 11 radiomic features between EGFR mutant and wild type groups. They found that mutant EGFR status could be predicted by a set of 5 radiomic features (AUC 0.647). When radiomic features were added to a clinical model the predictive power increased significantly with the AUC increasing from 0.667 to 0.709 (P>0.0001) (24).
Another study has assessed the potential of textural analysis to differentiate K-ras mutant from pan-wildtype tumors, as well as it’s prognostic potential by applying radiomics to 48 pre-treatment non-contrast CT studies with NSCLC. It was found that positive skewness with fine-texture and lower kurtosis with coarse-texture were significantly associated with K-ras mutations. They also demonstrated that quantitative CT textural analysis could accurately differentiate K-ras mutant from pan-wildtype tumors in 89.6% of cases and that Kurtosis was a significant predictor of overall survival and disease free survival, with a lower kurtosis value linked with poorer survival (25).
Prognostic indicators and disease response
In NSCLC, prognostic models have been developed for patients treated with surgery, radiotherapy or with targeted therapies. In each of these cases classifiers have been used to predict response and/or survival (26,27).
Coroller et al. examined whether pre-treatment radiomics data could predict pathological response after neoadjuvant therapy in patients with locally advanced NSCLC. In a cohort of 127 NSCLC patients they found 7 radiomic features were predictive for pathologic gross residual disease (AUC>0.6), and one for pathologic complete response (AUC=0.63). By contrast, no conventional imaging features were predictive. Tumors that did not respond well to neoadjuvant therapy were more likely to present a rounder shape and heterogeneous texture (28).
Other studies have shown that radiomic features extracted from CT images of lung cancers can be useful to distinguish radiation-induced fibrosis from tumor recurrence as well as identify patients with locally advanced lung adenocarcinoma at risk of developing distant metastasis (29).
A study performed by Mattonen et al. evaluated the accuracy of radiomics, for the predication of eventual local recurrence based on CT images acquired within 6 months of stereotactic ablative radiotherapy (SABR) in 45 patients with early stage NSCLC. A combination of five radiomic features demonstrated a AUC of 0.85 at 2–5 months post- SABR. At the same time point, three physicians assessed the majority of images as benign injury with overall errors of 34–37%. These results suggest that radiomics can detect early changes associated with local recurrence which are not typically considered by clinicians. This could lead to a decision support system which could potentially allow for early salvage therapy of patients with local recurrence following SABR (30).
Conclusions
The ever increasing number of indeterminate pulmonary nodules detected incidentally or during CT-based lung cancer screening provides considerable diagnostic and management challenges. It also however provides excellent opportunity for early stage characterization and tailored intervention and has enabled the development of a new field of research, namely radiomics. Radiomics also has the potential to revolutionize the diagnosis, surveillance and treatment planning of lung cancer allowing for personalized management in a way that is non-invasive and cost-effective.
Despite the initial evidence being promising more work needs to be done to improve the validity of these results before they can be applied in clinical practice. Foremost among the current challenges faced by radiomics in lung nodule assessment is the development of a universal language for CT descriptors, and harmonization of image acquisition parameters.
Acknowledgements
None.
Footnote
Conflicts of Interest: The authors have no conflicts of interest to declare.
References
- Gillies RJ, Kinahan PE, Hricak H, et al. Radiomics: Images Are More than Pictures, They Are Data. Radiology 2016;278. [Crossref] [PubMed]
- Lambin P, Rios-Velazquez E, Leijenaar R, et al. Radiomics: extracting more information from medical images using advanced feature analysis. Eur J Cancer 2012;48:441-6. [Crossref] [PubMed]
- MacMahon H, Austin JH, Gamsu G, et al. Guidelines for management of small pulmonary nodules detected on CT scans: a statement from the Fleischner Society. Radiology 2005;237:395-400. [Crossref] [PubMed]
- Naidich DP, Bankier AA, MacMahon H, et al. Recommendations for the management of subsolid pulmonary nodules detected at CT: a statement from the Fleischner Society. Radiology 2013;266:304-17. [Crossref] [PubMed]
- Callister ME, Baldwin DR, Akram AR, et al. British Thoracic Society guidelines for the investigation and management of pulmonary nodules. Thorax 2015;70:ii1-ii54. [Crossref] [PubMed]
- Ko JP, Berman EJ, Kaur M, et al. Pulmonary Nodules: growth rate assessment in patients by using serial CT and three-dimensional volumetry. Radiology 2012;262:662-71. [Crossref] [PubMed]
- Henschke CI, Yankelevitz DF, Yip R, et al. Lung cancers diagnosed at annual CT screening: volume doubling times. Radiology 2012;263:578-83. [Crossref] [PubMed]
- Hasegawa M, Sone S, Takashima S, et al. Growth rate of small lung cancers detected on mass CT screening. Br J Radiol 2000;73:1252-9. [Crossref] [PubMed]
- Wilson DO, Ryan A, Fuhrman C, et al. Doubling times and CT screen–detected lung cancers in the Pittsburgh Lung Screening Study. Am J Respir Crit Care Med 2012;185:85-9. [Crossref] [PubMed]
- Jennings SG, Winer-Muram HT, Tann M, et al. Distribution of stage I lung cancer growth rates determined with serial volumetric CT measurements. Radiology 2006;241:554-63. [Crossref] [PubMed]
- Winer-Muram HT, Jennings SG, Tarver RD, et al. Volumetric growth rate of stage I lung cancer prior to treatment: serial CT scanning. Radiology 2002;223:798-805. [Crossref] [PubMed]
- Revel MP, Bissery A, Bienvenu M, et al. Are two-dimensional CT measurements of small noncalcified pulmonary nodules reliable? Radiology 2004;231:453-8. [Crossref] [PubMed]
- Revel MP, Merlin A, Peyrard S, et al. Software volumetric evaluation of doubling times for differentiating benign versus malignant pulmonary nodules. AJR Am J Roentgenol 2006;187:135-42. [Crossref] [PubMed]
- Wu W, Parmar C, Grossmann P, et al. Exploratory Study to Identify Radiomics Classifiers for Lung Cancer Histology. Front Oncol 2016;6:71. [Crossref] [PubMed]
- Balagurunathan Y, Gu Y, Wang H, et al. Reproducibility and Prognosis of Quantitative Features Extracted from CT Images. Transl Oncol 2014;7:72-87. [Crossref] [PubMed]
- Horeweg N, van der Aalst CM, Vliegenthart R, et al. Volumetric computed tomography screening for lung cancer: three rounds of the NELSON trial. Eur Respir J 2013;42:1659-67. [Crossref] [PubMed]
- Ma J, Wang Q, Ren Y, et al. Automatic lung nodule classification with radiomics approach. Proc. Proc SPIE 2016;9879. doi:1117/12.2220768.
- Hawkins S, Wang H, Liu Y, et al. Predicting Malignant Nodules from Screening CT Scans. J Thorac Oncol 2016;11:2120-8. [Crossref] [PubMed]
- Lee SH, Lee SM, Goo JM, et al. Usefulness of texture analysis in differentiating transient from persistent part-solid nodules(PSNs): a retrospective study. PLoS One 2014;9:e85167. [Crossref] [PubMed]
- Chae HD, Park CM, Park SJ, et al. Computerized texture analysis of persistent part-solid ground-glass nodules: differentiation of preinvasive lesions from invasive pulmonary adenocarcinomas. Radiology 2014;273:285-93. [Crossref] [PubMed]
- Colen R, Foster I, Gatenby R, et al. NCI Workshop Report: Clinical and Computational Requirements for Correlating Imaging Phenotypes with Genomics Signatures. Transl Oncol 2014;7:556-69. [Crossref] [PubMed]
- Parmar C, Leijenaar RT, Grossmann P, et al. Radiomic feature clusters and prognostic signatures specific for Lung and Head & Neck cancer. Sci Rep 2015;5:11044. [Crossref] [PubMed]
- Maldonado F, Boland JM, Raghunath S, et al. Noninvasive characterization of the histopathologic features of pulmonary nodules of the lung adenocarcinoma spectrum using computer-aided nodule assessment and risk yield (CANARY)--a pilot study. J Thorac Oncol 2013;8:452-60. [Crossref] [PubMed]
- Liu Y, Kim J, Balagurunathan Y, et al. Radiomic Features Are Associated With EGFR Mutation Status in Lung Adenocarcinomas. Clin Lung Cancer 2016;17:441-8.e6. [Crossref] [PubMed]
- Weiss GJ, Ganeshan B, Miles KA, et al. Noninvasive image texture analysis differentiates K-ras mutation from pan-wildtype NSCLC and is prognostic. PLoS One 2014;9:e100244. [Crossref] [PubMed]
- Ganeshan B, Panayiotou E, Burnand K, et al. Tumour heterogeneity in non-small cell lung carcinoma assessed by CT texture analysis: a potential marker of survival. Eur Radiol 2012;22:796-802. [Crossref] [PubMed]
- Gevaert O, Xu J, Hoang CD, et al. Non-small cell lung cancer: identifying prognostic imaging biomarkers by leveraging public gene expression microarray data--methods and preliminary results. Radiology 2012;264:387-96. [Crossref] [PubMed]
- Coroller TP, Agrawal V, Narayan V, et al. Radiomic phenotype features predict pathological response in non-small cell lung cancer. Radiother Oncol 2016;119:480-6. [Crossref] [PubMed]
- Coroller TP, Grossmann P, Hou Y, et al. CT-based radiomic signature predicts distant metastasis in lung adenocarcinoma. Radiother Oncol 2015;114:345-50. [Crossref] [PubMed]
- Mattonen SA, Palma DA, Johnson C, et al. Detection of Local Cancer Recurrence After Stereotactic Ablative Radiation Therapy for Lung Cancer: Physician Performance Versus Radiomic Assessment. Int J Radiat Oncol Biol Phys 2016;94:1121-8. [Crossref] [PubMed]