Biophysical technologies for understanding circulating tumor cell biology and metastasis
Introduction
Despite the dramatic advances in targeted therapies, the major difficulty in the treatment of a metastatic malignancy is the eventual exhaustion of therapeutic options available to physicians and patients. Invariably, the development of new mutations, changes in cancer cell populations, and other resistance mechanisms lead to therapeutic resistance. Consequently, repeated tumor biopsies have been consistently shown to guide subsequent therapies in various malignancies (1,2). However, repeated biopsies are not only unfavorable to the patient due to inconvenience and discomfort, but they also carry significant procedural risks, add cost and labor, and may contribute to delay in treatment due to extended processing time. Thus, there exists the appeal of a so-called “liquid biopsy” that can less invasively act as a reliable surrogate for tumor biopsies, yet provide as much or even more information pertinent to cancer diagnosis, characteristics, and treatment options. The need for an alternative to physical biopsies is no more apparent than in lung cancer given the high risks associated with lung biopsies, as well as the discovery of actionable driver mutations like the epidermal growth factor receptor (EGFR) and anaplastic lymphoma kinase (ALK) for which targeted therapies now exist (3-5) together with identified resistance mutations to tyrosine kinase inhibitor therapy. Recent developments in liquid biopsy have increasingly focused on the expanding role of the circulating tumor cell (CTC). Herein, we review the history and the current status of CTC detection, discuss the prognostic, predictive, and diagnostic capabilities of these technologies, and describe application of CTCs as models for the study of tumor biology.
History
The first report of a CTC came in 1869 from Thomas Ashworth, who described the presence of tumor-derived epithelial cells in the blood compartment of a patient with end stage metastatic cancer, and postulated that this circulation of cells may account for the presence of multiple tumor metastases throughout the body of the patient (6). Subsequently, in 1948, Mandel and Métais found cell-free nucleic acid circulating in the human blood (7). Since that time, a variety of technologies have become available to characterize tumor elements in the peripheral blood. An early approach was to identify the presence of free nucleic acids released from cancer cells into the blood by cancer cell apoptosis and necrosis, as well as by active secretion (8,9). Based on the presence of cytokeratins on tumor cells, it was shown that the detection of non-tumor specific epithelial cell mRNA transcripts, such as cytokeratin, by real-time reverse transcriptase polymerase chain reaction (RT-PCR) was sensitive and could be used to prognosticate early stage breast cancer (10,11). However, the risk of illegitimate transcription (12) of epithelial protein transcripts by non-epithelial cells rendered this approach problematic, as even low levels of illegitimate transcription in the setting of the high ratio of hematopoietic cells to tumor cells would raise enough background noise to interfere with the accuracy of the assay (13). More recently, nucleic acid based detection of tumor DNA has focused on tumor mutations that are distinct from germ cell DNA and not subject to the problem of illegitimate transcription. This technique, known as circulating tumor DNA (ctDNA), has been shown to inform on treatment response and mutations associated with drug resistance (14,15).
Whole cell based CTC assays are another approach that can serve as a liquid biopsy. As the number of cancer cells in circulating blood ranges only at around 1–1,000 cells per 10 mL, and these cells are approximately 100–1,000 fold more rare than basophils and eosinophils (13), conventional methods of cellular detection are hence inadequate. Flow cytometry has been used to enumerate CTCs (16), but the number necessary for characterization is on the order of 103–104, much higher than exist CTCs in peripheral blood (13). The development of a fluorescence-based automated digital microscopy (ADM) system has shown promise with regards to reliable recognition of immunocytochemically labeled occult tumor cells, but at a cost of slow scanning speeds (17). The ADM system was further refined by the development of a fiber-optic array scanning technology (FAST) which allowed for scan rates 500 times faster than the conventional ADM (18). More recently, ADM techniques, with the capacity to scan and analyze cells from a single detector have been successfully employed and have become the basis for the high definition CTC (HD-CTC) described in more detail below (19).
Overview of current technologies for the detection of CTCs
CellSearch
The CellSearch CTC test was initially developed by Immunicon in the 1980s and subsequently acquired by Veridex in 2008, which in turn was acquired by Janssen in 2012. It remains the first and only FDA-cleared blood test used for the enumeration of CTCs. CellSearch relies on ferrofluid based immunomagnetic separation of cells expressing the epithelial cellular adhesion molecule (EpCAM). EpCAM has been shown to be expressed in a variety of human carcinomas of the gastrointestinal tract, upper aerodigestive and respiratory tract, and neuroendocrine tumors (20). The premise of the CellSearch method (Figure 1) hence capitalizes on this EpCAM expression: epithelial cells from samples of peripheral whole blood are immunomagnetically enriched by incubation with EpCAM specific immunomagnetic ferrofluid, then stained with CD45, DAPI, and cytokeratins 8, 18, and 19. The bound cells are then magnetically separated out from unbound cells and fixed (21,22). To characterize a cell as a CTC, the following criteria are then applied: round to oval morphology, DAPI positive-staining to identify visible nuclei, positive staining for cytokeratin, and negative staining for CD45 to rule out leukocytes (21). Using the CellSearch method, it was found that CTCs are rarely detected in the blood of normal patients, with ≥2 CTCs found in 0.3% of healthy and nonmalignant patients, whereas ≥2 CTCs were found in 36% of patients with metastatic carcinoma (21). The CellSearch method was subsequently shown to be feasible in isolating and characterizing CTCs from metastatic colorectal cancer (CRC) (22), and validated for clinical use in metastatic breast cancer (23). In 2011, Krebs et al. showed the ability to detect CTCs in non-small cell lung cancer (NSCLC), with a change in CTC number correlating with therapeutic interventions and survival outcome (24).
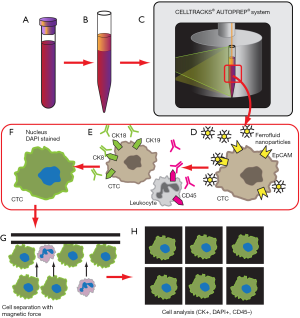
However, there are numerous limitations of CellSearch in the detection of CTCs. As EpCAM expression is the primary mechanism for positive selection of CTCs in CellSearch, CTCs not expressing EpCAM will hence be missed by the assay (23). This characteristic of the assay limits its application to analysis of differentiated tumor cells. EpCAM expression is downregulated when carcinoma cells enter the circulation (25). It has been shown that certain cancers had low levels of CTCs detected at baseline (26), and that in other tumor types, patients with poor prognostic characteristics demonstrated undetectable CTC status as measured by CellSearch (27). This underestimation may be explained by carcinoma cells that lose epithelial antigens while undergoing epithelial-to-mesenchymal transition (EMT) being missed (28). De-differentiated and EMT cell populations are relevant to understanding cancer behavior and are important to detect, as the presence of mesenchymal markers on CTCs predicts worse prognosis than the expression of cytokeratins alone (28). Because the number of white blood cells (WBCs) dwarfs the CTC population, reliance on single-parameter enrichment techniques may reduce the overall sensitivity of the assay in the background of noise from the WBC compared with multiparameter enrichment (13).
Microfluidic devices
The CTC-chip is a microfluidic silicon chip composed of an array of 78,000 microposts within a 970 mm2 surface arranged in an efficient geometric pattern (Figure 2, panel I) (29,30). The microposts are coated with antibodies to the EpCAM, designed to specifically capture CTCs from unfractionated blood given the frequent overexpression of EpCAM on various carcinomas and absence on cells of hematologic origin (20,29,31). On validation with 166 samples from patients with cancer and 20 healthy patients, the sensitivity of the CTC-chip was 99.1%, and the specificity was 100% (29). The average number of cells captured per 1 mL of blood was 155 for NSCLC, 86 for prostate, 79 for breast, and 121 for colorectal cancers (29). A further advance of the CTC-chip was the ability to maintain a mean viability of the captured cells of 98% (29), which makes the chip an attractive option for subsequent molecular diagnostics (30). Based on a small cohort of patients with metastatic cancer receiving active treatment, the changes in CTC number per mL correlated well with tumor volume as measured radiographically (29).
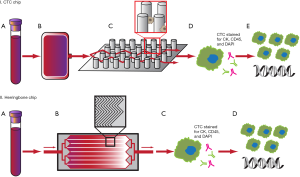
The first generation CTC-chip has since been followed with multiple iterations and enhancements. A limitation of the CTC-chip was that the intricate micropost design is difficult to produce on a large-scale basis for high-throughput production (32). The second generation herringbone-chip (HB-chip) improves on this design by utilizing herringbone-patterned ridges etched into the EpCAM antibody-coated wall of the device to create microvortices to disrupt streamlines and maximize interaction with target cells, and this simpler design more easily allows for production on a larger scale (Figure 2, panel II) (32). The HB-chip was able to detect CTCs in 93% of patients with metastatic prostate cancer with a mean CTC count of 386 per 1 mL blood, and had a 26.3% increased capture efficiency compared to the CTC-chip. An unexpected improvement of the HB-chip over the CTC-chip was its able to detect CTC microclusters, thought to be important in the role of cancer metastasis (32).
Numerous newer generations of the CTC-chip have been developed using updated technologies or other enhancements, such as wavy herringbone (33), size-based filtration (34), high throughput Vortex (35), nanotube-antibody microarrays (36), nanoroughened glass substrate (37), fluid cell-microarray chip (FCMC) (38), and nanovelcro chip (39), all with the intent to increase yield, accelerate analysis time, and improve capture of CTCs exhibiting EMT and various characteristics.
HD-CTC
Identification of CTCs in high definition (HD-CTC) is based on ADM techniques (Figure 3). Rather than using single protein enrichment strategies, all nucleated cells are retained and analyzed. The methods for HD-CTC detection were extensively described by Marrinucci et al. in 2012 (19). Whole blood specimens are subjected to red cell lysis and centrifugation. Nucleated cells are then re-suspended in phosphate-buffered saline (PBS) and attached as a monolayer to custom-made glass slides, each slide able to accommodate approximately 3 million nucleated cells, or about 0.5 mL of blood. These slides are frozen and thawed just before further analysis, and then stained for CD45, cytokeratin, and DAPI. Images are then scanned using a modified fluorescent scanning microscope and analyzed by digital pathology software. Cells need to meet specific criteria to be classified as HD-CTC, namely to have the following features: an intact DAPI nucleus without identifiable apoptotic changes or a disrupted appearance, express cytokeratin, lack expression of CD45, and be morphologically distinct from surrounding nonmalignant leukocytes (19,40). The advance of this platform is that images not meeting HD-CTC inclusion criteria were not discarded, but rather, were retained and digitally catalogued for subsequent re-analysis of HD-CTCs (40). This retention of all cells is touted by Epic Sciences, the primary licensee of this technology, as having the ability to maintain a “no cell left behind” approach.
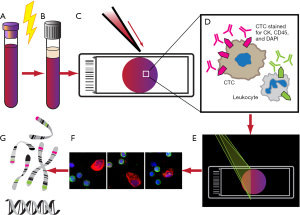
This assay has been used to characterize the morphology of CTCs. HD-CTCs were shown to have nuclei up to five times the average size of surrounding leukocyte nuclei, irregular nuclear contours, large cytoplasmic domain with an eccentric distribution of cytoplasm relative to nucleus, polygonal or elongated cytoplasmic shape, and clusters of two or more HD-CTCs (19). In comparison to CellSearch, the HD-CTC assay found significantly higher numbers of CTCs in significantly more patients with metastatic breast, prostate, and lung cancer (19,41). Furthermore, tumor staging did not seem to make a difference in the detection of CTCs in NSCLC (40). The major disadvantage with the HD-CTC assay, however, is the inability to analyze live cells due to the fixing of cells during processing (13).
Aggregates of CTCs may also be informative. The HD-CTC assay was shown to be feasible in identifying and characterizing CTC aggregates, and found to occur often in patients with metastatic breast, NSCLC, pancreatic, and prostate cancer (42). Subsequent mouse models have shown that CTC clusters arise from oligoclonal tumor cell groups rather than aggregation intravascularly, and these cluster are important mediators of cancer metastases (43).
Prognostic, predictive, and diagnostic values of CTC detection technology
While the aforementioned CTC detection methods have their respective strengths and weaknesses, the most important characteristic remains their utility in the biology of specific malignancies. The best barometer for the clinical utility of these tests is their prognostic, predictive, and diagnostic capabilities. These terms are often used erroneously and lead to confusion in interpreting data measuring these aspects. A concise review of this topic was provided by Dr. Karla Ballman in her 2015 Journal of Clinical Oncology article (44). In brief, a biomarker prognosticates for a disease if it is able to describe an outcome such as survival, disease recurrence, disease progression, or risk of metastasis, without attribution to treatment rendered. A predictive biomarker, on the other hand, denotes a difference in outcome as it relates to treatment based on whether the biomarker was positive or negative. For example, the presence of an EGFR mutation predicts response to targeted therapy such as gefitinib and erlotinib (3). The term “diagnostic” is more straight-forward and indicate a biomarker’s ability to detect a disease if it already exists. The prognostic, predictive, and diagnostic abilities of each method of CTC detection will be described here.
Prognostic
In lung cancer, the prognostic ability of CellSearch in enumerating CTCs has been demonstrated in both small cell and non-small cell patients. In small cell lung cancer (SCLC), a higher number of CTCs >300 per 7.5 mL blood was associated with a shorter median survival of 134 days compared to 443 days in patients with CTCs <2 per 7.5 mL blood (45). In NSCLC, Krebs et al. in 2011 showed that 32% of patients with metastatic disease had ≥2 CTCs per 7.5 mL blood prior to treatment with chemotherapy. When comparing baseline CTCs with post-treatment after one cycle of chemotherapy, patients with ≥5 CTCs per 7.5 mL blood compared to <5 at both time points had a hazard ratio (HR) of progression of 12.06, and a HR of death of 15.65 (24).
The most advanced work with CellSearch has been done in a study of metastatic breast cancer where ≥5 CTCs per 7.5 mL blood was shown to have a HR of 1.76 for progression and 4.26 for death compared to <5 CTCs per 7.5 mL blood (46). The large SWOG S0500 study confirmed the prognostic ability of using CellSearch for CTC enumeration, but also underscored the lack of its predictive capacity. A total of 595 patients with metastatic breast cancer were stratified into three groups based on the lack or presence of increased CTCs at baseline as well as after 21 days of therapy. Increased CTCs was defined as having five or more CTCs per 7.5 mL of blood. Patients who had persistently elevated CTCs after 21 days of first line chemotherapy were then randomized to either continue present therapy, or switch to an alternative therapy. There was no overall survival benefit when patients had early switch to alternate therapy based on persistence of elevated CTCs. However, the study confirmed that the number of CTCs was strongly prognostic, with patients having low CTCs at baseline demonstrating a more favorable median OS of 35 months, followed by patients with high CTCs converting to low CTCs after treatment doing slightly worse with a median OS of 23 months, followed by patients with persistently high CTCs despite treatment having the worst median OS of 13 months (47).
The CTC-chip has been used to assess for outcome in metastatic NSCLC (48). In a cohort of patients with advanced NSCLC, CTCs were found in all patients with a median of 74 cells/mL. Radiographic characterization of tumor burden did not appear to correlate with the number of CTCs from blood drawn at corresponding time points, and was thought to be related to influences on CTC number by other tumor characteristics. However, serial measurements did show a correlation between decrease in CTC number with treatment, and increase in CTC number with progression (48). A different fluidic-based chip platform termed the FCMC was developed in 2016 by Sawada et al. (38). The FCMC, which employs thousands of microchambers on a cell microarray chip, was used to examine the prognostic value compared to CellSearch in patients with advanced breast cancer. Using a threshold of 3 CTCs/1.6mL blood, the FCMC found a shorter PFS in CTC positive versus CTC negative patients with a HR of 11.31, which was much higher than the HR of 4.229 in CTC+ vs. CTC− patients using CellSearch. The increase in HR of FCMC over CellSearch is believed to be due to the improved detection of EMT-CTC in the latter (38). Similar findings have not yet been demonstrating in lung cancers.
In a single institution longitudinal analysis CTCs in metastatic NSCLC using the HD-CTC platform, higher numbers of CTCs were also shown to be associated with a poor prognosis. Of a total of 66 blood samples from 28 patients with NSCLC were evaluated, HD-CTCs were detected in 68% of the samples. A significant increase in the risk of death was found in patients with 5 or more CTCs per mL blood, compared to patients who had fewer than 5/mL, with a HR of 4.0 (41). Similarly, PD-L1 expression seems to correlate with worse prognosis in patients with NSCLC. Using the EPIC HD-CTC platform, peripheral blood samples from patients with NSCLC demonstrating PD-L1 expression in >1 cell/mL corresponded to worse overall survivals independent to staging in pre-biopsy and follow up samples (49). While not commercially available, this finding has the potential to allow the use of PD-L1 expression on CTCs as a predictive biomarker for immune checkpoint inhibition therapy (49).
Predictive
Unfortunately, as the CellSearch platform is designed purely for CTC enumeration, it offers no predictive capabilities alone unless combined with other modalities. In the phase II TRIGGER study, blood samples from patients being treated with erlotinib for EGFR mutated advanced NSCLC were taken for analysis. CTCs were captured using the CellSearch System and subsequently analyzed by Next Generation Sequencing (50). Of the 37 patients examined, CTCs that met all of Veridex’s criteria were found in only 41% of patients. However, in addition to the captured CTCs, when cells with potential neoplastic elements were also sequenced for EGFR mutations, a sensitivity of 84% and specificity of 100% was found (50). Essentially, this methodology acknowledges that not all neoplastic cells have the morphologic phenotype of a classical CTC. Such an approach of combining cell-based and genomic assessment may represent the future of CTC application as the combined techniques are more accurate.
In contrast, perhaps the most promising aspect of the CTC-chip is its predictive qualities. Indeed, the CTC-chip has been shown to effectively detect EGFR mutations in circulating lung cancer cells (48). In comparison of blood samples against tumor biopsies from 23 patients with NSCLC, CTCs were found in all patients with a median of 74 cells/mL, with a 92% sensitivity in detection of EGFR mutations. Of particular interest are observations that the number of CTCs did not correlate well with tumor burden radiographically at single time points, but did increase and decrease with radiographic progression and response, respectively, and that additional EGFR mutations including T790M emerged in CTCs throughout the course of treatment. The detection of mutational evolution in response to therapy in CTCs may suggest a clinical strategy of real-time tumor genotyping with the CTC chip, as well as obviate the need for repeated multiple tumor biopsies (30,48).
In a proof of concept study, the HB-chip showed promise in predicting for treatment responses in castrate resistant prostate cancer. Miyamoto et al. demonstrated that the HB-chip was able to detect changes in the androgen receptor (AR) and prostate-specific membrane antigen (PSMA) expression patterns on metastatic prostate cancer CTCs during the course of treatment with androgen deprivation therapy. Furthermore, an increase in AR reactivation in CTCs despite treatment with abiraterone acetate was associated with poor prognosis manifested by worse survival. While not validated, these findings suggest a potential for using pretreatment and posttreatment AR signaling in CTCs as a noninvasive method to individualize and guide decisions for second-line therapies (51).
The HB-chip has subsequently been studied in the detection of the T790M mutation in NSCLC. A series of patients with EGFR mutated NSCLC underwent tumor biopsies and blood draws for evaluation of CTCs and ctDNA. Of 37 patients whose blood was available for evaluation, 76% of CTC isolates had sufficient genetic material for genotyping. The EGFR T790M mutation was 74% concordant between CTCs and tumor biopsies, whereas the ctDNA was concordant with tumor biopsies in 61% of cases. When CTC and ctDNA genotyping were combined, the T790M mutation was detected in 100% of cases, with 35% detection in in patients that had a negative or indeterminate concurrent biopsy (52). While this study showed that using CTC for detection of the T790M mutation was feasible, the yield may be increased by the combination of multiple genotyping modalities. More recently, the detection of ALK-rearrangement in NSCLC by break-apart FISH has been described in other microfluidic chip platforms like the nanovelcro chip (39) and the label-free CTChip® FR1 by Clearbridge BioMedics (53).
HD-CTC has also been used to assess for predictability to treatment in metastatic castrate resistant prostate cancer (mCRPC). Pretreatment blood from 161 patients with mCRPC were assessed for expression of the androgen-receptor splice variant 7 (AR-V7) by immunofluorescence on CTCs captured using Epic Sciences’ non-EpCAM based HD-CTC platform. All patients with AR-V7 expressing CTCs demonstrated resistance to AR signaling inhibitors, and had significantly worse PFS and OS when compared with patients with CTCs negative for AR-V7. These findings support the use of AR-V7 as a predictive biomarker for response failure to treatment with AR signaling inhibitors (54). HD-CTC has been lesser studied in the predictive setting for NSCLC, but in a small series of patients with NSCLC, detection of ALK rearrangement by FISH on peripheral blood CTCs using the Epic platform demonstrated modest sensitivity and specificity (55).
One can also learn tumor biology from CTCs without the use of any device at all aside from the use of animals. Direct injection of CTCs from blood of SCLC patients has been successful in developing tumor xenografts in mice. Hodgkinson et al. described the first successful CTC-derived xenograft (CDX) model in which CTCs from SCLC were transplanted into mice to form tumors, showing that SCLC CTCs were tumorigenic, that CDXs represented clinical SCLC, and that CDXs accurately reflected patient response to therapy (56). This powerful new advancement provides a unique opportunity to minimally invasively create accurate in vivo models of lung cancer, and is currently being used to study tumor behavior, elucidate mechanisms of drug resistance, and develop novel targeted therapies for SCLC (Figure 4) (57-59).
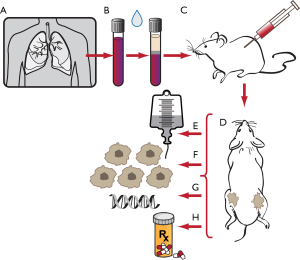
Diagnostic
The microfluidic nanovelcro chip showed a 75% sensitivity and 96.4% specificity for the diagnosis of primary pancreatic ductal adenocarcinoma, with 60.7%, 78.6%, and 96.3% detection in stages II, III, and IV, respectively; however, there was 0% detection in stage I disease (60). A size-based filtration microfluidic chip used to detect CTCs in lung cancer patients demonstrated a 73.3% sensitivity and 100% specificity, with a threshold for definition of lung cancer by CTC level set at 5.15 cells per mL (34).
Similarly, in 78 chemotherapy naïve patients with stage I–IV NSCLC, blood samples were obtained and analyzed for HD-CTC. There was a high HD-CTC detection rate of 73% across the whole population, with a median of 4.4 HD-CTCs per 1 mL blood and a mean of 44.1 HD-CTCs per 1 mL blood (40). Notably, HD-CTCs were detected in 85% of patients with stage I and II disease and 79% in stage III (40). When examined against FDG-PET imaging in NSCLC, HD-CTC number did not correlate with tumor diameter, and increasing HD-CTC counts only weakly correlated with increasing SUV values. The heterogeneity of HD-CTC numbers seen in both early and late stage diseases suggest a potential use of HD-CTC to gain insight into individual tumor biology, and use as a noninvasive diagnostic tool (61). Indeed, when circulating tumor microemboli (CTM) were examined in concordance with imaging in patients with NSCLC, it was shown that CTMs in combination with clinical and imaging data was able to significantly discriminate for diagnostic accuracy in all stages as well as stage I disease (62).
Future technologies for tumor characterization of CTCs
An understanding of cancer evolution over time must take into account the genomics of a tumor. This is now possible on a single cell basis at the level of whole genome copy number analysis (63-67). Combined with morphologic analysis of CTCs, the addition of genomic analysis provides a capacity to study cancer populations as a collection of individual cells, often revealing cancers to be oligoclonal proliferations of cancer cells, rather than truly clonal populations possessing a singular genome (68). The unique nature of CTC analysis, permitting serial analysis has been successfully performed on patients, creating a method for the identification of new tumor lineages to be identified as they evolve in real time (69).
Genomic analysis of tumors is not ideal for understanding phenotypic changes in tumor over time. Proteome analysis at the single cell level has often been performed by using immune detection of cancer cells. However, because most technologies for CTC collection already expend much of the available color spectrum for collection and identification of tumor cells, often there are only 1 or 2 color channels left to perform analysis of protein. Mass spectrometry provides an alternative platform for studying proteomics. Historically, the matrix assisted laser desorption ionization imaging mass spectrometry (MALDI IMS) set the stage for the mass spectrometric analysis of biological specimens (70). For tissue samples, specimens are spray-coated or microspotted with MALDI matrix in a grid pattern, and a laser beam fired at the tissue section “paints” a two-dimensional map of the signal intensity across the intact tissue section (71,72). The result is the ability to analyze hundreds of molecules quickly and simultaneously (71). The advantage of MALDI IMS over other in situ molecular analysis techniques is the lack of target-specific reagents required, as is needed in immunohistochemistry (73). This technology has already been used to characterize diagnostic and prognostic markers in tumors such as breast (74), brain (75), colon (76), gastric (77), lung (78-80), and prostate cancer (81). In a large retrospective study by Taguchi et al., blood collected from patients with NSCLC before treatment with an EGFR TKI was analyzed by MALDI IMS and compared against patient outcome, and an algorithm was developed to stratify patients into “good” or “poor” risk groups based on possible clinical benefit from EGFR TKI treatment (82). Subsequently, the large phase 3 PROSE trial was able to prospectively demonstrate that patients with NSCLC carrying a good proteomic test classification predicted for benefit with erlotinib therapy over chemotherapy, whereas those with a poor proteomic classification predicted for benefit with chemotherapy over erlotinib (83).
Fluidigm has developed a mass cytometry technology that takes advantage of inductively coupled plasma mass time-of-flight spectrometry (ICP-TOF-MS). Cells are tagged with antibodies bound to rare earth elements rather than fluorescent labels, nebulized, and sent to a fast elemental reader, which is able to detect proteins and other molecules in individual cells (84). This technology has been used to assay antigens from patients’ samples of leukemia and breast cancer (84-86). This technology has been successfully applied to CTC analysis in combination with genomic analysis (87). Thus the future of cancer research will benefit from technology that allows for repeat sampling of tumors, single cell genomic characterization, and subsequently single cell proteomic analysis. This combined platform, known as High Definition Single Cell Analysis (HD-SCA), is being expanded outside of the laboratory that developed it and will open additional operations at new laboratories in the United Kingdom and in Maryland, USA, in addition to its current location in Los Angeles. As the technology for rapid and inexpensive combined proteogenomic analysis of single cells becomes available to an increasing number of investigators, new knowledge can be gained as the scale of research performed is increased.
Acknowledgements
The authors wish to thank Yekaterina Kadyshevskaya for her assistance with illustrations.
Funding: This work was supported in part by award number P30CA014089 from the National Cancer Institute.
Footnote
Conflicts of Interest: The authors have no conflicts of interest to declare.
References
- Pusztai L, Viale G, Kelly CM, et al. Estrogen and HER-2 receptor discordance between primary breast cancer and metastasis. Oncologist 2010;15:1164-8. [Crossref] [PubMed]
- Shaw AT, Friboulet L, Leshchiner I, et al. Resensitization to Crizotinib by the Lorlatinib ALK Resistance Mutation L1198F. N Engl J Med 2016;374:54-61. [Crossref] [PubMed]
- Pao W, Miller V, Zakowski M, et al. EGF receptor gene mutations are common in lung cancers from “never smokers” and are associated with sensitivity of tumors to gefitinib and erlotinib. Proc Natl Acad Sci U S A 2004;101:13306-11. [Crossref] [PubMed]
- Mok TS, Wu YL, Thongprasert S, et al. Gefitinib or Carboplatin–Paclitaxel in Pulmonary Adenocarcinoma. N Engl J Med 2009;361:947-57. [Crossref] [PubMed]
- Solomon BJ, Mok T, Kim D-W, et al. First-Line Crizotinib versus Chemotherapy in ALK-Positive Lung Cancer. N Engl J Med 2014;371:2167-77. [Crossref] [PubMed]
- Ashworth TR. A case of cancer in which cells similar to those in the tumours were seen in the blood after death. Aust Med J 1869;14:146-9.
- Mandel P, Metais P. Les acides nucléiques du plasma sanguin chez l'homme. C R Seances Soc Biol Fil 1948;142:241-3. [PubMed]
- Schwarzenbach H, Hoon DS, Pantel K. Cell-free nucleic acids as biomarkers in cancer patients. Nat Rev Cancer 2011;11:426-37. [Crossref] [PubMed]
- Stroun M, Maurice P, Vasioukhin V, et al. The origin and mechanism of circulating DNA. Ann N Y Acad Sci 2000;906:161-8. [Crossref] [PubMed]
- Ignatiadis M, Xenidis N, Perraki M, et al. Different prognostic value of cytokeratin-19 mRNA positive circulating tumor cells according to estrogen receptor and HER2 status in early-stage breast cancer. J Clin Oncol 2007;25:5194-202. [Crossref] [PubMed]
- Xenidis N, Perraki M, Kafousi M, et al. Predictive and prognostic value of peripheral blood cytokeratin-19 mRNA-positive cells detected by real-time polymerase chain reaction in node-negative breast cancer patients. J Clin Oncol 2006;24:3756-62. [Crossref] [PubMed]
- Chelly J, Concordet JP, Kaplan JC, et al. Illegitimate transcription: transcription of any gene in any cell type. Proc Natl Acad Sci U S A 1989;86:2617-21. [Crossref] [PubMed]
- Nieva JJ, Kuhn P. Fluid biopsy for solid tumors: a patient's companion for lifelong characterization of their disease. Future Oncol 2012;8:989-98. [Crossref] [PubMed]
- Murtaza M, Dawson SJ, Tsui DW, et al. Non-invasive analysis of acquired resistance to cancer therapy by sequencing of plasma DNA. Nature 2013;497:108-12. [Crossref] [PubMed]
- Dawson SJ, Tsui DWY, Murtaza M, et al. Analysis of Circulating Tumor DNA to Monitor Metastatic Breast Cancer. N Engl J Med 2013;368:1199-209. [Crossref] [PubMed]
- Racila E, Euhus D, Weiss AJ, et al. Detection and characterization of carcinoma cells in the blood. Proc Natl Acad Sci U S A 1998;95:4589-94. [Crossref] [PubMed]
- Kraeft SK, Ladanyi A, Galiger K, et al. Reliable and sensitive identification of occult tumor cells using the improved rare event imaging system. Clin Cancer Res 2004;10:3020-8. [Crossref] [PubMed]
- Hsieh HB, Marrinucci D, Bethel K, et al. High speed detection of circulating tumor cells. Biosens Bioelectron 2006;21:1893-9. [Crossref] [PubMed]
- Marrinucci D, Bethel K, Kolatkar A, et al. Fluid biopsy in patients with metastatic prostate, pancreatic and breast cancers. Phys Biol 2012;9:016003. [Crossref] [PubMed]
- Went PTH, Lugli A, Meier S, et al. Frequent EpCam protein expression in human carcinomas. Human Pathology 2004;35:122-8. [Crossref] [PubMed]
- Allard WJ, Matera J, Miller MC, et al. Tumor Cells Circulate in the Peripheral Blood of All Major Carcinomas but not in Healthy Subjects or Patients With Nonmalignant Diseases. Clin Cancer Res 2004;10:6897. [Crossref] [PubMed]
- Cohen SJ, Alpaugh RK, Gross S, et al. Isolation and characterization of circulating tumor cells in patients with metastatic colorectal cancer. Clin Colorectal Cancer 2006;6:125-32. [Crossref] [PubMed]
- Riethdorf S, Fritsche H, Muller V, et al. Detection of circulating tumor cells in peripheral blood of patients with metastatic breast cancer: a validation study of the CellSearch system. Clin Cancer Res 2007;13:920-8. [Crossref] [PubMed]
- Krebs MG, Sloane R, Priest L, et al. Evaluation and prognostic significance of circulating tumor cells in patients with non-small-cell lung cancer. J Clin Oncol 2011;29:1556-63. [Crossref] [PubMed]
- Rao CG, Chianese D, Doyle GV, et al. Expression of epithelial cell adhesion molecule in carcinoma cells present in blood and primary and metastatic tumors. Int J Oncol 2005;27:49-57. [PubMed]
- Gradilone A, Iacovelli R, Cortesi E, et al. Circulating Tumor Cells and “Suspicious Objects” Evaluated Through CellSearch® in Metastatic Renal Cell Carcinoma. Anticancer Research 2011;31:4219-21. [PubMed]
- Mego M, De Giorgi U, Dawood S, et al. Characterization of metastatic breast cancer patients with nondetectable circulating tumor cells. Int J Cancer 2011;129:417-23. [Crossref] [PubMed]
- Gradilone A, Raimondi C, Nicolazzo C, et al. Circulating tumour cells lacking cytokeratin in breast cancer: the importance of being mesenchymal. J Cell Mol Med 2011;15:1066-70. [Crossref] [PubMed]
- Nagrath S, Sequist LV, Maheswaran S, et al. Isolation of rare circulating tumour cells in cancer patients by microchip technology. Nature 2007;450:1235-9. [Crossref] [PubMed]
- Sequist LV, Nagrath S, Toner M, et al. The CTC-chip: an exciting new tool to detect circulating tumor cells in lung cancer patients. J Thorac Oncol 2009;4:281-3. [Crossref] [PubMed]
- Balzar M, Winter MJ, de Boer CJ, et al. The biology of the 17–1A antigen (Ep-CAM). Journal of Molecular Medicine 1999;77:699-712. [Crossref] [PubMed]
- Stott SL, Hsu CH, Tsukrov DI, et al. Isolation of circulating tumor cells using a microvortex-generating herringbone-chip. Proc Natl Acad Sci U S A 2010;107:18392-7. [Crossref] [PubMed]
- Wang S, Thomas A, Lee E, et al. Highly efficient and selective isolation of rare tumor cells using a microfluidic chip with wavy-herringbone micro-patterned surfaces. Analyst 2016;141:2228-37. [Crossref] [PubMed]
- Huang T, Jia CP, Jun Y, et al. Highly sensitive enumeration of circulating tumor cells in lung cancer patients using a size-based filtration microfluidic chip. Biosens Bioelectron 2014;51:213-8. [Crossref] [PubMed]
- Che J, Yu V, Dhar M, et al. Classification of large circulating tumor cells isolated with ultra-high throughput microfluidic Vortex technology. Oncotarget 2016;7:12748-60. [Crossref] [PubMed]
- Khosravi F, Trainor PJ, Lambert C, et al. Static micro-array isolation, dynamic time series classification, capture and enumeration of spiked breast cancer cells in blood: the nanotube-CTC chip. Nanotechnology 2016;27:44LT03. [Crossref] [PubMed]
- Chen W, Allen SG, Reka AK, et al. Nanoroughened adhesion-based capture of circulating tumor cells with heterogeneous expression and metastatic characteristics. BMC Cancer 2016;16:614. [Crossref] [PubMed]
- Sawada T, Araki J, Yamashita T, et al. Prognostic Impact of Circulating Tumor Cell Detected Using a Novel Fluidic Cell Microarray Chip System in Patients with Breast Cancer. EBioMedicine 2016;11:173-82. [Crossref] [PubMed]
- He W, Xu D, Wang Z, et al. Detecting ALK-rearrangement of CTC enriched by nanovelcro chip in advanced NSCLC patients. Oncotarget 2016. [Epub ahead of print].
- Wendel M, Bazhenova L, Boshuizen R, et al. Fluid biopsy for circulating tumor cell identification in patients with early-and late-stage non-small cell lung cancer: a glimpse into lung cancer biology. Phys Biol 2012;9:016005. [Crossref] [PubMed]
- Nieva J, Wendel M, Luttgen MS, et al. High-definition imaging of circulating tumor cells and associated cellular events in non-small cell lung cancer patients: a longitudinal analysis. Phys Biol 2012;9:016004. [Crossref] [PubMed]
- Cho EH, Wendel M, Luttgen M, et al. Characterization of circulating tumor cell aggregates identified in patients with epithelial tumors. Phys Biol 2012;9:016001. [Crossref] [PubMed]
- Aceto N, Bardia A, Miyamoto David T, et al. Circulating Tumor Cell Clusters Are Oligoclonal Precursors of Breast Cancer Metastasis. Cell 2014;158:1110-22. [Crossref] [PubMed]
- Ballman KV. Biomarker: Predictive or Prognostic? J Clin Oncol 2015;33:3968-71. [Crossref] [PubMed]
- Hou JM, Greystoke A, Lancashire L, et al. Evaluation of circulating tumor cells and serological cell death biomarkers in small cell lung cancer patients undergoing chemotherapy. Am J Pathol 2009;175:808-16. [Crossref] [PubMed]
- Cristofanilli M, Budd GT, Ellis MJ, et al. Circulating Tumor Cells, Disease Progression, and Survival in Metastatic Breast Cancer. N Engl J Med 2004;351:781-91. [Crossref] [PubMed]
- Smerage JB, Barlow WE, Hortobagyi GN, et al. Circulating tumor cells and response to chemotherapy in metastatic breast cancer: SWOG S0500. J Clin Oncol 2014;32:3483-9. [Crossref] [PubMed]
- Maheswaran S, Sequist LV, Nagrath S, et al. Detection of mutations in EGFR in circulating lung-cancer cells. N Engl J Med 2008;359:366-77. [Crossref] [PubMed]
- Boffa DJ, Nieva JJ, Bazhenova L, et al. Programmed cell death-1 ligand (PD-L1) expression on circulating CD45(-) cells is an independent prognostic factor for overall survival in patients (Pts) with lung cancer in a prospective, multicenter cohort. J Clin Oncol 2016;34:abstr 8524.
- Marchetti A, Del Grammastro M, Felicioni L, et al. Assessment of EGFR mutations in circulating tumor cell preparations from NSCLC patients by next generation sequencing: toward a real-time liquid biopsy for treatment. PLoS One 2014;9:e103883. [Crossref] [PubMed]
- Miyamoto DT, Lee RJ, Stott SL, et al. Androgen Receptor Signaling in Circulating Tumor Cells as a Marker of Hormonally Responsive Prostate Cancer. Cancer Discov 2012;2:995-1003. [Crossref] [PubMed]
- Sundaresan TK, Sequist LV, Heymach JV, et al. Detection of T790M, the Acquired Resistance EGFR Mutation, by Tumor Biopsy versus Noninvasive Blood-Based Analyses. Clin Cancer Res 2016;22:1103-10. [Crossref] [PubMed]
- Tan CL, Lim TH, Lim T, et al. Concordance of anaplastic lymphoma kinase (ALK) gene rearrangements between circulating tumor cells and tumor in non-small cell lung cancer. Oncotarget 2016;7:23251-62. [Crossref] [PubMed]
- Scher HI, Lu D, Schreiber NA, et al. Association of AR-V7 on Circulating Tumor Cells as a Treatment-Specific Biomarker With Outcomes and Survival in Castration-Resistant Prostate Cancer. JAMA Oncol 2016;2:1441-9. [Crossref] [PubMed]
- Lu D, Krupa R, Bales N, et al. Abstract 2841: Characterization of ALK fusions in circulating tumor cells (CTCs) of NSCLC. Cancer Res 2014;74:2841. [Crossref]
- Hodgkinson CL, Morrow CJ, Li Y, et al. Tumorigenicity and genetic profiling of circulating tumor cells in small-cell lung cancer. Nat Med 2014;20:897-903. [Crossref] [PubMed]
- Potter DS, Galvin M, Brown S, et al. Inhibition of PI3K/BMX Cell Survival Pathway Sensitizes to BH3 Mimetics in SCLC. Mol Cancer Ther 2016;15:1248-60. [Crossref] [PubMed]
- Owonikoko TK, Zhang G, Kim HS, et al. Patient-derived xenografts faithfully replicated clinical outcome in a phase II co-clinical trial of arsenic trioxide in relapsed small cell lung cancer. J Transl Med 2016;14:111. [Crossref] [PubMed]
- Jahchan NS, Lim JS, Bola B, et al. Identification and Targeting of Long-Term Tumor-Propagating Cells in Small Cell Lung Cancer. Cell Rep 2016;16:644-56. [Crossref] [PubMed]
- Ankeny JS, Court CM, Hou S, et al. Circulating tumour cells as a biomarker for diagnosis and staging in pancreatic cancer. Br J Cancer 2016;114:1367-75. [Crossref] [PubMed]
- Nair VS, Keu KV, Luttgen MS, et al. An observational study of circulating tumor cells and (18)F-FDG PET uptake in patients with treatment-naive non-small cell lung cancer. PLoS One 2013;8:e67733. [Crossref] [PubMed]
- Carlsson A, Nair VS, Luttgen MS, et al. Circulating Tumor Microemboli Diagnostics for Patients with Non–Small-Cell Lung Cancer. J Thorac Oncol 2014;9:1111-9. [Crossref] [PubMed]
- Pratt ED, Stepansky A, Hicks J, et al. Single-cell copy number analysis of prostate cancer cells captured with geometrically enhanced differential immunocapture microdevices. Anal Chem 2014;86:11013-7. [Crossref] [PubMed]
- Navin N, Kendall J, Troge J, et al. Tumour evolution inferred by single-cell sequencing. Nature 2011;472:90-4. [Crossref] [PubMed]
- Baslan T, Kendall J, Ward B, et al. Optimizing sparse sequencing of single cells for highly multiplex copy number profiling. Genome Res 2015;25:714-24. [Crossref] [PubMed]
- Baslan T, Kendall J, Rodgers L, et al. Genome-wide copy number analysis of single cells. Nat Protoc 2012;7:1024-41. [Crossref] [PubMed]
- Baslan T, Hicks J. Single cell sequencing approaches for complex biological systems. Curr Opin Genet Dev 2014;26:59-65. [Crossref] [PubMed]
- Ruiz C, Li J, Luttgen MS, et al. Limited genomic heterogeneity of circulating melanoma cells in advanced stage patients. Phys Biol 2015;12:016008. [Crossref] [PubMed]
- Dago AE, Stepansky A, Carlsson A, et al. Rapid phenotypic and genomic change in response to therapeutic pressure in prostate cancer inferred by high content analysis of single circulating tumor cells. PLoS One 2014;9:e101777. [Crossref] [PubMed]
- Caprioli RM, Farmer TB, Gile J. Molecular Imaging of Biological Samples: Localization of Peptides and Proteins Using MALDI-TOF MS. Anal Chem 1997;69:4751-60. [Crossref] [PubMed]
- Cornett DS, Reyzer ML, Chaurand P, et al. MALDI imaging mass spectrometry: molecular snapshots of biochemical systems. Nat Methods 2007;4:828-33. [Crossref] [PubMed]
- Schwamborn K, Caprioli RM. MALDI Imaging Mass Spectrometry—Painting Molecular Pictures. Mol Oncol 2010;4:529-38. [Crossref] [PubMed]
- Schwamborn K, Kriegsmann M, Weichert W. MALDI imaging mass spectrometry—From bench to bedside. Biochim Biophys Acta 2017;1865:776-83. [PubMed]
- Cornett DS, Mobley JA, Dias EC, et al. A novel histology-directed strategy for MALDI-MS tissue profiling that improves throughput and cellular specificity in human breast cancer. Mol Cell Proteomics 2006;5:1975-83. [Crossref] [PubMed]
- Schwartz SA, Weil RJ, Thompson RC, et al. Proteomic-based prognosis of brain tumor patients using direct-tissue matrix-assisted laser desorption ionization mass spectrometry. Cancer Res 2005;65:7674-81. [Crossref] [PubMed]
- Meding S, Balluff B, Elsner M, et al. Tissue-based proteomics reveals FXYD3, S100A11 and GSTM3 as novel markers for regional lymph node metastasis in colon cancer. J Pathol 2012;228:459-70. [Crossref] [PubMed]
- Balluff B, Rauser S, Meding S, et al. MALDI Imaging Identifies Prognostic Seven-Protein Signature of Novel Tissue Markers in Intestinal-Type Gastric Cancer. Am J Pathol 2011;179:2720-9. [Crossref] [PubMed]
- Groseclose MR, Massion PP, Chaurand P, et al. High-throughput proteomic analysis of formalin-fixed paraffin-embedded tissue microarrays using MALDI imaging mass spectrometry. Proteomics 2008;8:3715-24. [Crossref] [PubMed]
- Yanagisawa K, Shyr Y, Xu BJ, et al. Proteomic patterns of tumour subsets in non-small-cell lung cancer. Lancet 2003;362:433-9. [Crossref] [PubMed]
- Rahman SM, Gonzalez AL, Li M, et al. Lung Cancer Diagnosis from Proteomic Analysis of Preinvasive Lesions. Cancer Res 2011;71:3009. [Crossref] [PubMed]
- Cazares LH, Troyer D, Mendrinos S, et al. Imaging mass spectrometry of a specific fragment of mitogen-activated protein kinase/extracellular signal-regulated kinase kinase kinase 2 discriminates cancer from uninvolved prostate tissue. Clin Cancer Res 2009;15:5541-51. [Crossref] [PubMed]
- Taguchi F, Solomon B, Gregorc V, et al. Mass Spectrometry to Classify Non–Small-Cell Lung Cancer Patients for Clinical Outcome After Treatment With Epidermal Growth Factor Receptor Tyrosine Kinase Inhibitors: A Multicohort Cross-Institutional Study. J Natl Cancer Inst 2007;99:838-46. [Crossref] [PubMed]
- Gregorc V, Novello S, Lazzari C, et al. Predictive value of a proteomic signature in patients with non-small-cell lung cancer treated with second-line erlotinib or chemotherapy (PROSE): a biomarker-stratified, randomised phase 3 trial. Lancet Oncol 2014;15:713-21. [Crossref] [PubMed]
- Bandura DR, Baranov VI, Ornatsky OI, et al. Mass Cytometry: Technique for Real Time Single Cell Multitarget Immunoassay Based on Inductively Coupled Plasma Time-of-Flight Mass Spectrometry. Anal Chem 2009;81:6813-22. [Crossref] [PubMed]
- Giesen C, Wang HAO, Schapiro D, et al. Highly multiplexed imaging of tumor tissues with subcellular resolution by mass cytometry. Nat Methods 2014;11:417-22. [Crossref] [PubMed]
- Schüffler PJ, Schapiro D, Giesen C, et al. Automatic single cell segmentation on highly multiplexed tissue images. Cytometry A 2015;87:936-42. [Crossref] [PubMed]
- Carlsson A, Kuhn P, Luttgen MS, et al. Paired high-content analysis of prostate cancer cells in bone marrow and blood characterizes increased androgen receptor expression in tumor cell clusters. Clin Cancer Res 2017;23:1722-32. [Crossref]