Blood-based biomarkers for precision medicine in lung cancer: precision radiation therapy
Background
Precision medicine aims to take into account individual variability in genes, environment, and lifestyle for each person in order to achieve the most accurate treatment and prevention. This is in contrast to the traditional “one-size-fits-all” or “standard” approach, in which treatments are given based on the overall response or tolerance in the whole patient population, with little consideration for individual differences. Typically, only a proportion of patients will be cured by the standard therapy (1,2). The concept of “precision medicine” may not be completely new. As an example, in radiotherapy for lung cancer, the anatomy of the patient and its relation with the tumor are delineated on an individual basis and the prescribed dose to the target volumes are often limited by constraints of the organs at risk (OARs) (3). However, it is clear that these physical parameters are only the beginning of the move to a more holistic view of a patient and disease.
It is compelling that biomarkers in the blood can reflect the status of a tumor and of the OARs to allow precision radiation dose prescription and adaptation during therapy. However, these blood biomarkers should not be viewed alone, but in the context of the anatomical deposition of the radiation dose in the tissue. Indeed, the extent of radiation damage is not only determined by the radiosensitivity of an OAR, but also by dose-volume parameters and the regional characteristics of the OAR (4). Both deterministic and stochastic factors play a role in the outcome of radiotherapy. Moreover, irradiation of functionally irrelevant parts of the lung without the capacity to function, such as emphysematous bullae, will not have an effect on toxicity, even in individuals who may be sensitive to radiation injury (5,6).
High doses of radiotherapy to the lung and heart have been reported to negatively correlate with survival (7,8). Although severe organ toxicities are life-threatening and there is a high possibility of unreported or unidentified grade 5 toxicities, most cases of death in non-small cell lung cancer (NSCLC) patients treated with radiotherapy may not be caused by direct organ toxicity, because the number of reported grade 5 toxicities is much less than the number of deaths without evidence of disease progression. Recently, accumulating evidence has supported the idea that the immune system may play an important role in tumor control and survival (9-12). The immune system is also susceptible to radiation damage, which may impact tumor control and survival. Therefore, survival may also serve as an endpoint of normal tissue toxicities of the immune system that has not been clearly defined previously. Considering that a tumor originates from normal tissue, it may share some similar radiosensitivity traits with the normal tissue. Under such circumstances, biomarkers of the radiosensitivity of normal tissue could also partially reflect the radiosensitivity of the tumor. Using a predictive model that incorporates dosimetry to both tumor and normal tissue, radiosensitivity biomarkers can be applied to guide precision radiotherapy. Such a model can also be used to better identify radiosensitivity biomarkers, because otherwise, the biomarkers would be buried under the noise of dosimetric variations.
Therefore, an integral approach that combines biomarker detection, dosimetry, normal tissue preservation, and tumor control can be employed to identify and utilize biomarkers for precision radiotherapy. In this review, we will discuss blood-based biomarkers with an emphasis on the methodology for how these biomarkers can be identified and utilized in the integrated approach.
Biomarkers for normal tissue complications
Radiation damage of the normal tissue is a major cause of radiation complications. Several studies support the hypothesis that normal tissue radiosensitivity is influenced by genetic features of the patient (13). Normal tissue radiation complications may also be influenced by immunological or inflammation responses to the injury (14,15). Therefore, in terms of functionality, both radiosensitivity and non-radiosensitivity biomarkers will be potentially associated with the normal tissue complication, while in terms of originality of the biomarkers, both genetic biomarkers [such as single nucleotide polymorphisms (SNPs)] and serum or plasma biomarkers (such as inflammation cytokines) will be potentially associated with radiation-induced lung toxicity (RILT), including radiation pneumonitis (RP) and pulmonary fibrosis. In addition, other factors, such as gender, body weight, life style habits, medication use, etc., also influence the risk and severity of side effects. In this section, we will discuss blood biomarkers for normal tissue complications in terms of: (I) genomic biomarkers; (II) proteomics biomarkers; (III) cytokines; and (IV) an integrated approach to identifying predictive markers.
Genomic biomarkers
The majority of studies on genomic blood markers investigated the association SNPs and radiation toxicity (13,16,17). SNPs associated with the side effects of radiation have been identified by high-throughput genotyping, through genome-wide association studies (GWAS), and by candidate gene studies (13). The candidate genes are usually those involved in DNA repair such as excision repair cross-complementing (ERCC), X-ray repair cross complementing (XRCC), and so on (18,19), in inflammation such as transforming growth factor beta 1 (TGF-β1) and tumor necrosis factor alpha (TNFα), in oxidative stress, and in apoptotic pathways such as p53. Many studies have reported potential SNPs in these genes that are associated with radiation toxicities (20,21).
An SNP of the ataxia telangiectasia mutated (ATM) gene, a central mediator of the radioprotective machinery in response to radiation therapy that participates in cellular stress responses, control of cell cycle checkpoints, repair of double-strand breaks (DSBs), and initiation of apoptosis, has also been reported to be a risk factor for RP. Xiong et al. reported that ATM variants [e.g., rs189037 (−111 G) and rs228590T] independently and jointly have a substantial impact on the risk of severe RP in NSCLC patients treated with radiotherapy and also reported a consistent effect of the rs189037G/rs228590T/rs1801516G (G-T-G) haplotype (17).
SNPs of TGF-β1 or proteins in its pathway have been reported to have a significant influence on radiation toxicity. The HSPB1 rs2868371 SNP was associated with grade 3 or greater RP in both training (P=0.031) and validation sets (P=0.025) (22). Interestingly, this SNP was also associated with the risk of grade 3 or greater radiation-induced esophagitis in both training (P=0.045) and validation cohorts (P=0.031) (23). The hazard ratio (HR) was approximately 0.25. In addition, the TGF-β1 rs1800469 SNP was associated with a higher risk of radiation esophagitis in both training (P=0.045) and validation (0.023) cohorts with a HR of about 2.50 (23). Yuan et al. from the University of Michigan reported that genetic variation in the TGFβ1 pathway genes may be associated with combined thoracic toxicity of lung, heart, and esophagus (16). TGF-β1−509 T allele carriers had significantly less severe radiation esophagitis (P=0.019) and a lower mean grade of radiation-induced thoracic toxicity (RITT) (P=0.009) than TGF-β1 CC patients. Patients with sensitive genotypes in this pathway had a significantly higher grade of toxicity in lung, esophagus, and heart than those without sensitive genotypes (P<0.01).
Yuan et al. discovered that patients with different genotypes of rs1982073:T869C in TGF-β1 are at lower risk of RP after radiation therapy for NSCLC (16) independent of dosimetric factors such as total lung volume receiving more than 20 Gy (V20) and mean lung dose (MLD). Patients with the CT or CC genotype in TGF-β1 rs1982073:T869C had a significantly lower incidence of severe RP than those with the TT genotype, especially among patients who had received an MLD <20 Gy or a V20 <30%. Indeed, this SNP in TGF-β1 could be used to separate patients into different risk groups even after correction for MLD. External validation, however, is not yet available for this finding.
Another SNP is the TNF-α 0629:308 G > A AA genotype, which was shown to be associated with severe RP, although the number of patients in that study was small (24). Among four patients with the AA genotype, three (75%) experienced severe RP versus 21 of 92 patients (23%) with the AG/GG genotype. This difference could not be accounted for by differences in MLD.
DNA repair is important in radiation injury. Functional polymorphisms of the base-excision repair genes XRCC1 and APEX1 and genetic variants of the nonhomologous end-joining gene LIG4 can predict the risk of RP, not only in Caucasians but also in Han Chinese patients (18,19,25). Furthermore, polymorphisms in vascular endothelial growth factor (VEGF) may modulate the risk of RP, and the CC genotype of HSPB1 rs2868371 is associated with a higher risk of severe RP (22,26). Subsequent analyses of patients treated with chemoradiation showed that XRCC1 Q399R = WW (versus PP or PW), VEGF4032 CT/TT, and TNF0629 = AA all confer a higher risk of severe RP. Patients carrying the ATM rs189037 variant AA genotype are at high risk of developing severe RP, particularly those who receive an MLD of ≥19.0 Gy (17).
Incorporating SNPs into normal tissue complication probability predictive models such the Lyman dosimetric model has been shown to improve the model’s ability to predict the likelihood of RP (24). Tucker et al. studied 16 potentially functional SNPs in genes related to DNA repair, cell cycling, TGF-β1, TNFα, folic acid metabolism, and angiogenesis from 143 patients. Five SNPs were selected for inclusion in a multivariate normal tissue complication probability model based on MLD alone. SNPs associated with an increased risk of severe RP were found in genes of TGF-β1, VEGF, TNFα, XRCC1, and APEX1. When smoking status was included in the multivariate model, SNPs associated with an increased risk of RP were found in TGF-β1, VEGF, and XRCC3. The SNP that most significantly improved the “t” of the Lyman model based on lung dosimetry alone was XRCC. These findings provide evidence that SNPs may significantly improve the predictive ability of the Lyman model. Even with a small number of SNPs, it is possible to distinguish cohorts with >50% risk versus <10% risk of RP upon exposure to a high MLD.
However, independent validation of these findings has been challenging due to differences in endpoints, patient populations, and toxicity scoring criteria (27-30).
Large datasets with objective and consistent measurements of endpoints and tightly controlled variables are needed to validate these findings and to prospectively test the models developed in clinical settings. A significant drawback to identifying SNPs that are related to radiation injury is that large numbers of patients are needed, whereas the incidence of severe toxicity is fortunately low. Moreover, the major driver for radiation injury is the radiation dose and volume represented by the dose volume histogram (DVH), and this factor should always be taken into account when assessing markers of radiosensitivity.
Cytokine and proteomic markers
TGF-β1 has been extensively studied as a biomarker predicting RILT (16,31-40). A persistently elevated plasma TGF-β1 above baseline level at the end of RT, the high ratio of TGF-β1 level over baseline during RT such as a significant elevation of TGF-β1 level at 4 weeks after RT are significantly associated with symptomatic RILT (35,36). A return of the plasma TGF-β1 to a normal level could identify patients who will not develop RP (31). However, TGF-β1 is also produced by lung cancer and also a known immune inhibitor, both of these may have confounded the toxicity results. TGF-β1 is also a sticky molecule, richly stored in platelet. Inconsistent TGF-β1 results were also noted, largely attributed to platelet contamination/degradation during blood sample handling procedures.
A complex network of cytokines may be involved in the process of RILT. Early variations in circulating interleukin (IL)-6 and IL-10 levels during RT were reported to be significantly associated with the risk of RP in a multivariate analysis (P=0.011) (41,42). IL-6 levels before, during, and after thoracic radiation therapy also were reported to be significantly higher in those who developed pneumonitis (41). A low baseline level of IL-8 expression was consistently reported to be highly associated with RILT in two independent studies (42,43). However, most of these results have not been repeated by other investigators (44,45). Recently, it was proposed that the early response of cytokines within 1–24 hours after RT may be a better predictor of RILT (46).
Proteomic approaches may identify new plasma protein markers for toxicity prediction. Cai et al. identified C4b-binding protein alpha chain, complement C3, and vitronectin as having significantly higher expression levels in patients with symptomatic RILT compared with patients without, based on both the datasets from the RT start to 3 months post-RT (P<0.01) and from RT start to the end-RT (P<0.01) (47). Using comparative, high-resolution liquid chromatography (LC)-MS, Oh et al. identified α-2-macroglobulin (α2M) as the top candidate protein, validated independently using enzyme-linked immunosorbent assay (ELISA) on samples from an independent cohort of 20 patients (P=0.002) (48). Unfortunately, effort and knowledge are limited on proteomics to identify and validate new biomarkers for planning of precision radiation, partially due to expensive cost associated with the testing.
Blood biomarkers for toxicity can therefore help to personalize adaptive dose prescription (49).
Blood-based biomarkers for tumor control and survival
Many factors contribute to tumor control and survival for a given patient. In addition to patient and tumor factors, treatment-related factors like radiation dose also play important roles. Further complicating this scenario, the factors related to normal tissue complications like immune suppression may also contribute to tumor control and survival because the immune system is also critical for tumor control.
Radiosensitivity biomarkers
Tumor cells circulate in the blood such that these circulating tumor cells (CTCs) and their mutation types can be detected with advanced genomic technology in blood samples. CTCs may reflect the most active types of tumor cells in the primary tumor so that they may be a better representation of the tumor heterogeneity. While the mutation types have been used as radiosensitivity biomarkers for the tumor tissue samples, no systematic studies have been performed to correlate mutation types of CTCs with radiosensitivity because CTCs are a recently immerging field of study. Similarly, cell-free circulating tumor DNA (ctDNA) can be released from the tumor and quantified in blood. ctDNA analysis has become an established alternative to determine EGFR status (50). There is great promise that serial ctDNA quantification during and after radiation therapy can be used to monitor tumor response to therapy (51-54). The quantification of early ctDNA release after radiation therapy, which reflects early cell death, is likely related to tumor radiosensitivity. Moreover, serial quantification of ctDNA might allow identification of certain mutations representing tumor subclones that do not respond favorably to radiation therapy, thus possibly reflecting the presence of radioresistant clones. Ongoing studies will clarify the potential of ctDNA quantification for radiosensitivity analysis.
It is challenging to study biomarkers for radiosensitivity, as heterogeneous responses from sensitive and resistant patients may cancel out the dose and survival relationship, and often there is not enough treatment variation to generate a dose-response relationship. In a study of 92 stage III NSCLC patients who received doses varying from 60–91 Gy, Jin et al. used a stratified dosimetric modeling approach to identify genotypic markers associated with DNA repair pathways for radiosensitivity assessment (55). These investigators reported that one SNP signature (combining ERCC2:rs238406 and ERCC1:rs11615) was significant for the radiotherapy dose-response. As shown in Figure 1, the RT doses required to generate a 50% OS rate at 2 years were 64 and 76 Gy, for sensitive and resistant groups, respectively. A future study with independent validation is needed to confirm this finding.
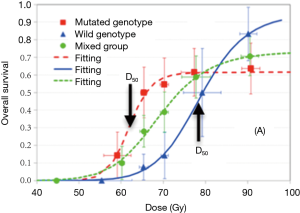
Non-radiosensitivity biomarkers
Many studies have reported that serum cytokine levels are correlated with survival (56). These include C-reactive protein, IL-6, IL-8, IL-10, and TNF-α. TGF-β1 expression at baseline and after treatment also was reported to correlate with disease status and treatment outcome in NSCLC patients (57). IL-6 and IL-8 were reported to significantly correlate with surgical treatment outcome in stage 1 NSCLC patients, and the combination of IL-6 and IL-8 increased the prediction value. Notably, the prediction value was better for stage IA patients than Stage IB patients.
While cytokines can be considered as immune markers, other proteins that directly reflect immune check points, such as programmed death 1 (PD-1), programmed death ligand 1 (PD-L1), or the number of tumor-infiltrating CD8+ T lymphocytes have been reported to be prognostic biomarkers for NSCLC. Most of the studies measured PD-L1 expression or CD8+ T cells in tumor, while other studies measured them in the peripheral blood (58). A high expression level of PD-L1 was usually correlated with positive responses in PD-L1/PD-1-based immune checkpoint blockade treatments (59). However, the high PD-L1 expression level was reported to be a poor prognostic factor for patients treated with surgery (60,61). High indoleamine 2,3-dioxygenase (IDO) expression level also was reported to be associated with poor prognosis (62-64).
In NSCLC, associations between ctDNA, tumor size, stage, and the reduction after surgical resection or radiation therapy have been suggested (52,53,65). It remains to be determined under which circumstances are ctDNAs of prognostic value for NSCLC patients (51,54).
Radiosensitivity biomarkers in combination of dosimetric factors to guide precision RT
Radiosensitivity biomarkers for both tumor and normal tissues are the key factors to determining the optimal radiation dose via a holistic approach. As the radiation dose to a tumor increases, the killing of tumor cells increases, and the tumor control/survival will increase. However, increasing the tumor dose also proportionally increases the doses delivered to the normal tissue, and damage to the immune system and other normal structures increases. This can reduce tumor control/survival. Therefore, an appropriate balance of these two competing factors will result in superior survival at an optimal prescription dose of treatment.
Figure 2 illustrates an example of how 2-year survival varies with the dose of radiation delivery to the tumor for patients with differing radiosensitivity. A patient with a radiation-sensitive genotype had an optimal prescription dose of 66 Gy, whereas a patient with radio-resistant genotypic features had an optimal dose of 85 Gy. Using this biomarker-based model, a personalized dose prescription may be generated to improve 2-year survival from 50% to 85% and 3% to 73% for hypothetically sensitive and resistant patients, respectively.
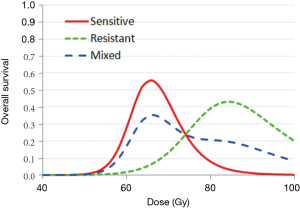
Summary and future direction
Given the individualized complexity of tumors and patients, models that determine survival and toxicity of radiotherapy or any other treatment must take into account all spectrum variability as well as its dynamic state. However, until recently, there were no means to identify or quantify these complex biological processes. This has changed dramatically and with an ever-increasing pace. The genetic features of the tumor and the host, and increasingly also the epi-genetic and proteomic characteristics, are being unraveled. However, radiation effects on the tumor and normal tissues are also affected by the dose and volume of irradiated tissues. These dosimetric parameters are often reduced to a single figure such as the maximal or the mean dose or some volumetric-dose parameter Vx such as the volume of the lungs receiving 20 Gy or more.
A major challenge is the combination of all these biological, clinical, imaging, and dosimetric parameters in a validated prognostic and predictive model. Major improvements have been made, as summarized in the present review. It is important to stress that comprehensive dosimetric parameters must be taken into account in order to make the models complete, as dose and volume are still the major drivers of radiation effects in all tissues.
A combined biological-mathematical approach opens new doors beyond prognostication of patients; It allows truly precise oncological treatment. Indeed, the core for individualized and precision medicine is not only appropriate selection of patients, but even more the optimization of the therapeutic window on an individual basis. The holistic model will enable the determination of an individual dose-response relationship for each OAR for each tumor in each individual patient for the complete oncological treatment package. This includes, but is not limited to, radiotherapy alone. With an individual’s dose-response curve in hand, it will be possible to test different doses of radiation and the combination of radiotherapy with any drug to plan treatments to achieve the optimal balance between toxicity and the chance for cure. Insights in the interactions between a multitude of parameters will lead to the discovery of new pathways and networks and fuel new biological research on target discovery for true precision medicine.
Very large datasets will sometimes, but not always, be required to construct these models, for the precision of the model is determined by the magnitude of the effects, which may or may not be large. Increasingly precise dosimetric models will reduce the number of patients needed to identify genetic determinants of toxicity, supporting the idea that these models can be established with a reasonable number of patients.
Therefore, we are moving away from intuitive changes in planning, such as lowering the dose for very radiosensitive patients without knowing the therapeutic window, to a thorough, objective and quantifiable holistic model.
Acknowledgements
None.
Footnote
Conflicts of Interest: FM Kong—Speaker’s Honorarium and Travel Support from Vairan Medical Inc.
References
- Eberhardt WE, De Ruysscher D, Weder W, et al. 2nd ESMO Consensus Conference in Lung Cancer: locally advanced stage III non-small-cell lung cancer. Ann Oncol 2015;26:1573-88. [Crossref] [PubMed]
- Bradley JD, Paulus R, Komaki R, et al. Standard-dose versus high-dose conformal radiotherapy with concurrent and consolidation carboplatin plus paclitaxel with or without cetuximab for patients with stage IIIA or IIIB non-small-cell lung cancer (RTOG 0617): a randomised, two-by-two factorial phase 3 study. Lancet Oncol 2015;16:187-99. [Crossref] [PubMed]
- van Baardwijk A, Wanders S, Boersma L, et al. Mature results of an individualized radiation dose prescription study based on normal tissue constraints in stages I to III non–small-cell lung cancer. J Clin Oncol 2010;28:1380-6. [Crossref] [PubMed]
- Kerns SL, L, West CM, Andreassen CN, et al. Radiogenomics: the search for genetic predictors of radiotherapy response. Future Oncol 2014;10:2391-406. [Crossref] [PubMed]
- Defraene G, van Elmpt W, Crijns W, et al. CT characteristics allow identification of patient-specific susceptibility for radiation-induced lung damage. Radiother Oncol 2015;117:29-35. [Crossref] [PubMed]
- Defraene G, van Elmpt W, Crijns W, et al. Regional variability in radiation-induced lung damage can be predicted by baseline CT numbers. Radiother Oncol 2017;122:300-6. [Crossref] [PubMed]
- Speirs CK, DeWees TA, Rehman S, et al. Heart Dose Is an Independent Dosimetric Predictor of Overall Survival in Locally Advanced Non–Small Cell Lung Cancer. J Thorac Oncol 2017;12:293-301. [Crossref] [PubMed]
- Tucker SL, Liu A, Gomez D, et al. Impact of heart and lung dose on early survival in patients with non-small cell lung cancer treated with chemoradiation. Radiother Oncol 2016;119:495-500. [Crossref] [PubMed]
- Formenti SC. Silvia Formenti on the Promise of Combining Radiotherapy and Immunotherapy to Treat Cancer. Oncology (Williston Park) 2016;30:289, 292.
- Binder DC, Fu YX, Weichselbaum RR. Radiotherapy and immune checkpoint blockade: potential interactions and future directions. Trends Mol Med 2015;21:463-5. [Crossref] [PubMed]
- Formenti SC, Demaria S. Combining radiotherapy and cancer immunotherapy: a paradigm shift. J Natl Cancer Inst 2013;105:256-65. [Crossref] [PubMed]
- Lee Y, Auh SL, Wang Y, et al. Therapeutic effects of ablative radiation on local tumor require CD8+ T cells: changing strategies for cancer treatment. Blood 2009;114:589-95. [Crossref] [PubMed]
- Kerns SL, Ostrer H, Rosenstein BS. Radiogenomics: using genetics to identify cancer patients at risk for development of adverse effects following radiotherapy. Cancer Discov 2014;4:155-65. [Crossref] [PubMed]
- Jelonek K, Pietrowska M, Widlak P. Systemic effects of ionizing radiation at the proteome and metabolome levels in the blood of cancer patients treated with radiotherapy: the influence of inflammation and radiation toxicity. Int J Radiat Biol 2017;93:683-96. [Crossref] [PubMed]
- Rühle PF, Wunderlich R, Deloch L, et al. Modulation of the peripheral immune system after low-dose radon spa therapy: Detailed longitudinal immune monitoring of patients within the RAD-ON01 study. Autoimmunity 2017;50:133-140. [Crossref] [PubMed]
- Yuan X, Liao Z, Liu Z, et al. Single nucleotide polymorphism at rs1982073:T869C of the TGFbeta 1 gene is associated with the risk of radiation pneumonitis in patients with non-small-cell lung cancer treated with definitive radiotherapy. J Clin Oncol 2009;27:3370-8. [Crossref] [PubMed]
- Xiong H, Liao Z, Liu Z, et al. ATM polymorphisms predict severe radiation pneumonitis in patients with non-small cell lung cancer treated with definitive radiation therapy. Int J Radiat Oncol Biol Phys 2013;85:1066-73. [Crossref] [PubMed]
- Yin M, Liao Z, Liu Z, et al. Functional polymorphisms of base excision repair genes XRCC1 and APEX1 predict risk of radiation pneumonitis in patients with non-small cell lung cancer treated with definitive radiation therapy. Int J Radiat Oncol Biol Phys 2011;81:e67-73. [Crossref] [PubMed]
- Yin M, Liao Z, Liu Z, et al. Genetic variants of the nonhomologous end joining gene LIG4 and severe radiation pneumonitis in nonsmall cell lung cancer patients treated with definitive radiotherapy. Cancer 2012;118:528-35. [Crossref] [PubMed]
- Dadrich M, Nicolay NH, Flechsig P, et al. Combined inhibition of TGFβ and PDGF signaling attenuates radiation-induced pulmonary fibrosis. Oncoimmunology 2015;5:e1123366. [Crossref] [PubMed]
- Kong FM, Wang S. Non-dosimetric risk factors for radiation-induced lung toxicity. Semin Radiat Oncol 2015;25:100-9. [Crossref] [PubMed]
- Pang Q, Wei QY, Xu T, et al. Functional Promoter Variant rs2868371 of HSPB1 Is Associated With Risk of Radiation Pneumonitis After Chemoradiation for Non-Small Cell Lung Cancer. Int J Radiat Oncol Biol Phys 2013;85:1332-9. [Crossref] [PubMed]
- Guerra JLL, Gomez D, Wei Q, et al. Association between single nucleotide polymorphisms of the transforming growth factor β1 gene and the risk of severe radiation esophagitis in patients with lung cancer. Radiother Oncol 2012;105:299-304. [Crossref] [PubMed]
- Tucker SL, Li M, Xu T, et al. Incorporating single-nucleotide polymorphisms into the Lyman model to improve prediction of radiation pneumonitis. Int J Radiat Oncol Biol Phys 2013;85:251-7. [Crossref] [PubMed]
- Li H, Liu G, Xia L, et al. A polymorphism in the DNA repair domain of APEX1 is associated with the radiation-induced pneumonitis risk among lung cancer patients after radiotherapy. Br J Radiol 2014;87:20140093. [Crossref] [PubMed]
- Guan X, Yin M, Wei Q, et al. Genotypes and haplotypes of the VEGF gene and survival in locally advanced non-small cell lung cancer patients treated with chemoradiotherapy. BMC Cancer 2010;10:431. [Crossref] [PubMed]
- Vinogradskiy Y, Tucker SL, Bluett JB, et al. Prescribing radiation dose to lung cancer patients based on personalized toxicity estimates. J Thorac Oncol 2012;7:1676-82. [Crossref] [PubMed]
- Voets AM, Oberije C, Struijk RB, et al. No association between TGF-beta1 polymorphisms and radiation-induced lung toxicity in a European cohort of lung cancer patients. Radiother Oncol 2012;105:296-8. [Crossref] [PubMed]
- Wang L, Bi N. TGF-β1 Gene Polymorphisms for Anticipating Radiation-Induced Pneumonitis in Non–Small-Cell Lung Cancer: Different Ethnic Association. J Clin Oncol 2010;28:e621-2. [Crossref] [PubMed]
- Chanock SJ, Manolio T, Boehnke M, et al. Replicating genotype-phenotype associations. Nature 2007;447:655-60. [Crossref] [PubMed]
- Anscher MS, Kong FM, Andrews K, et al. Plasma transforming growth factor β1 as a predictor of radiation pneumonitis. Int J Radiat Oncol Biol Phys 1998;41:1029-35. [Crossref] [PubMed]
- Anscher MS, Kong FM, Jirtle RL. The relevance of transforming growth factor β1 in pulmonary injury after radiation therapy. Lung Cancer 1998;19:109-20. [Crossref] [PubMed]
- Anscher MS, Kong FM, Marks LB, et al. Changes in plasma transforming growth factor beta during radiotherapy and the risk of symptomatic radiation-induced pneumonitis. Int J Radiat Oncol Biol Phys 1997;37:253-8. [Crossref] [PubMed]
- Fu XL, Huang H, Bentel G, et al. Predicting the risk of symptomatic radiation-induced lung injury using both the physical and biologic parameters V 30 and transforming growth factor β. Int J Radiat Oncol Biol Phys 2001;50:899-908. [Crossref] [PubMed]
- Zhao L, Sheldon K, Chen M, et al. The predictive role of plasma TGF-β1 during radiation therapy for radiation-induced lung toxicity deserves further study in patients with non-small cell lung cancer. Lung Cancer 2008;59:232-9. [Crossref] [PubMed]
- Kim JY, Kim YS, Kim YK, et al. The TGF-β1 dynamics during radiation therapy and its correlation to symptomatic radiation pneumonitis in lung cancer patients. Radiat Oncol 2009;4:59. [Crossref] [PubMed]
- Kong FM, Washington MK, Jirtle RL, et al. Plasma transforming growth factor-β1 reflects disease status in patients with lung cancer after radiotherapy: a possible tumor marker. Lung Cancer 1996;16:47-59. [Crossref] [PubMed]
- Kong FM, Anscher MS, Sporn TA, et al. Loss of heterozygosity at the mannose 6-phosphate insulin-like growth factor 2 receptor (M6P/IGF2R) locus predisposes patients to radiation-induced lung injury. Int J Radiat Oncol Biol Phys 2001;49:35-41. [Crossref] [PubMed]
- De Jaeger K, Seppenwoolde Y, Kampinga HH, et al. Significance of plasma transforming growth factor-β levels in radiotherapy for non–small-cell lung cancer. Int J Radiat Oncol Biol Phys 2004;58:1378-87. [Crossref] [PubMed]
- Novakova-Jiresova A, van Gameren MM, Coppes RP, et al. Transforming growth factor-β plasma dynamics and post-irradiation lung injury in lung cancer patients. Radiother Oncol 2004;71:183-9. [Crossref] [PubMed]
- Chen Y, Rubin P, Williams J, et al. Circulating IL-6 as a predictor of radiation pneumonitis. Int J Radiat Oncol Biol Phys 2001;49:641-8. [Crossref] [PubMed]
- Hart JP, Broadwater G, Rabbani Z, et al. Cytokine profiling for prediction of symptomatic radiation-induced lung injury. Int J Radiat Oncol Biol Phys 2005;63:1448-54. [Crossref] [PubMed]
- Stenmark MH, Cai XW, Shedden K, et al. Combining physical and biologic parameters to predict radiation-induced lung toxicity in patients with non-small-cell lung cancer treated with definitive radiation therapy. Int J Radiat Oncol Biol Phys 2012;84:e217-22. [Crossref] [PubMed]
- Crohns M, Saarelainen S, Laine S, et al. Cytokines in bronchoalveolar lavage fluid and serum of lung cancer patients during radiotherapy—association of interleukin-8 and VEGF with survival. Cytokine 2010;50:30-6. [Crossref] [PubMed]
- Rübe CE, Palm J, Erren M, et al. Cytokine plasma levels: reliable predictors for radiation pneumonitis? PLoS One 2008;3:e2898. [Crossref] [PubMed]
- Siva S, MacManus M, Kron T, et al. A pattern of early radiation-induced inflammatory cytokine expression is associated with lung toxicity in patients with non-small cell lung cancer. PLoS One 2014;9:e109560. [Crossref] [PubMed]
- Cai XW, Shedden K, Ao X, et al. Plasma proteomic analysis may identify new markers for radiation-induced lung toxicity in patients with non-small-cell lung cancer. Int J Radiat Oncol Biol Phys 2010;77:867-76. [Crossref] [PubMed]
- Oh JH, Craft JM, Townsend R, et al. A bioinformatics approach for biomarker identification in radiation-induced lung inflammation from limited proteomics data. J Proteome Res 2011;10:1406-15. [Crossref] [PubMed]
- Kong FM, Ao X, Wang L, et al. The use of blood biomarkers to predict radiation lung toxicity: a potential strategy to individualize thoracic radiation therapy. Cancer Control 2008;15:140-50. [Crossref] [PubMed]
- Luo J, Shen L, Zheng D. Diagnostic value of circulating free DNA for the detection of EGFR mutation status in NSCLC: a systematic review and meta-analysis. Sci Rep 2014;4:6269. [Crossref] [PubMed]
- Deig CR, Mendonca MS, Lautenschlaeger T. Blood-Based Nucleic Acid Biomarkers as a Potential Tool to Determine Radiation Therapy Response in Non-Small Cell Lung Cancer. Radiat Res 2017;187:333-8. [Crossref] [PubMed]
- Bettegowda C, Sausen M, Leary RJ, et al. Detection of circulating tumor DNA in early- and late-stage human malignancies. Sci Transl Med 2014;6:224ra24. [Crossref] [PubMed]
- Newman AM, Bratman SV, To J, et al. An ultrasensitive method for quantitating circulating tumor DNA with broad patient coverage. Nat Med 2014;20:548-54. [Crossref] [PubMed]
- Guo N, Lou F, Ma Y, et al. Circulating tumor DNA detection in lung cancer patients before and after surgery. Sci Rep 2016;6:33519. [Crossref] [PubMed]
- Jin JY, Wang W, Ten Haken RK, et al. Use a survival model to correlate single-nucleotide polymorphisms of DNA repair genes with radiation dose-response in patients with non-small cell lung cancer. Radiother Oncol 2015;117:77-82. [Crossref] [PubMed]
- Liao C, Yu Z, Guo W, et al. Prognostic value of circulating inflammatory factors in non-small cell lung cancer: a systematic review and meta-analysis. Cancer Biomark 2014;14:469-81. [Crossref] [PubMed]
- Ryan BM, Pine SR, Chaturvedi AK, et al. A combined prognostic serum interleukin-8 and interleukin-6 classifier for stage 1 lung cancer in the prostate, lung, colorectal, and ovarian cancer screening trial. J Thorac Oncol 2014;9:1494-503. [Crossref] [PubMed]
- Meniawy TM, Lake RA, McDonnell AM, et al. PD-L1 on peripheral blood T lymphocytes is prognostic in patients with non-small cell lung cancer (NSCLC) treated with EGFR inhibitors. Lung Cancer 2016;93:9-16. [Crossref] [PubMed]
- Aguiar PN Jr, Santoro IL, Tadokoro H, et al. The role of PD-L1 expression as a predictive biomarker in advanced non-small-cell lung cancer: a network meta-analysis. Immunotherapy 2016;8:479-88. [Crossref] [PubMed]
- Mori S, Motoi N, Ninomiya H, et al. High expression of programmed cell death 1 ligand 1 in lung adenocarcinoma is a poor prognostic factor particularly in smokers and wild-type epidermal growth-factor receptor cases. Pathol Int 2017;67:37-44. [Crossref] [PubMed]
- Inamura K, Yokouchi Y, Sakakibara R, et al. Relationship of tumor PD-L1 expression with EGFR wild-type status and poor prognosis in lung adenocarcinoma. Jpn J Clin Oncol 2016;46:935-41. [Crossref] [PubMed]
- Suzuki K, Kachala SS, Kadota K, et al. Prognostic immune markers in non-small cell lung cancer. Clin Cancer Res 2011;17:5247-56. [Crossref] [PubMed]
- Creelan BC, Antonia SJ, Bepler G, et al. Indoleamine 2, 3-dioxygenase activity and clinical outcome following induction chemotherapy and concurrent chemoradiation in Stage III non-small cell lung cancer. Oncoimmunology 2013;2:e23428. [Crossref] [PubMed]
- Suzuki Y, Suda T, Furuhashi K, et al. Increased serum kynurenine/tryptophan ratio correlates with disease progression in lung cancer. Lung Cancer 2010;67:361-5. [Crossref] [PubMed]
- Chan KC, Jiang P, Zheng YW, et al. Cancer genome scanning in plasma: detection of tumor-associated copy number aberrations, single-nucleotide variants, and tumoral heterogeneity by massively parallel sequencing. Clin Chem 2013;59:211-24. [Crossref] [PubMed]