Editorial commentary: meeting a paramount challenge
As predicted by the Global Burden of Disease study 2020 (1), lung cancer will continue to be the heaviest burden worldwide to the public health among all malignant diseases (2). With tobacco consumption shifted from the rich to the poor in the recent decade, 50% of smokers are residing in 5 counties, i.e., China, Brazil, Russia, India and Indonesia, underlining where most of the high-risk populations will be to acquire lung cancer (3). Due to the obnoxiously late stage at the time of diagnosis, 5-year survival rate of lung cancer patients remains to be under 20% even in economically advanced countries (4), which signifies the crucial importance of screening and early detection in high risk populations. However, defining and focusing on the at-risk individuals are far from adequate and feasible. For examples, the foremost pitfalls of the currently and publically implemented screening criteria in the United States using low dose CT scan (5) could only identify 30–40% lung cancer in the population, yet accompanying a greater than 90% false positivity. Moreover, substantially high risk individuals were missed by the current definition (6,7). Therefore, a paramount challenge is to establish precision screening scheme with nearly perfect early detection tools. A recently published article published in Cancer (January 15, 2018), entitled “Probability of Cancer in High-Risk Patients Predicted by the Protein-Based Lung Cancer Biomarker Panel in China: LCBP Study”, exemplifies the effort to meet this challenge (8).
This multicenter investigation, led by Dr. C Bai with co-leading authors Drs. D Yang and X Zhang, enrolled 715 participants from 5 regional centers in Beijing, Zhengzhou, Nanjing, Shanghai and Chongqing, analyzed four serum biomarkers (ProGRP, CEA, SCC, and CYFRA21-1) along with relevant clinical information, and developed two prediction models: one for patient risk and one for lung nodule risk (8). Both models demonstrated excellent discrimination for the early diagnosis of lung cancer, with the under the receiver operating characteristic (ROC) curve (AUC) of patient risk model at 0.72, and of the nodule risk model at 0.92 relative to American College of Chest Physicians (ACCP) model (9). The authors compared with and surpassed the Mayo Clinic model suggested by ACCP guidelines (0.92 vs. 0.84) (8), which is consistent with other previously reported studies (Table 1) (10-12). Two key messages could be drawn from the LCPB study based on interpretations of the results: first, to the researchers, the improved power of discrimination illustrated the foreseeing capability to stratify patients with different levels of lung cancer risk although the sensitivity and specificity are not ideal at all settings considered. Second, to the persons at high risk of lung cancer and the care providers, these new models hold high potential to be applicable in high-risk populations upon further evaluation of additional biological markers.
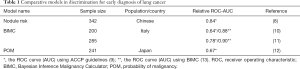
Full table
Acknowledgements
None.
Footnote
Conflicts of Interest: The author has no conflicts of interest to declare.
References
- GBD 2015 Disease and Injury Incidence and Prevalence Collaborators. Global, regional, and national incidence, prevalence, and years lived with disability for 310 diseases and injuries, 1990-2015: a systematic analysis for the Global Burden of Disease Study 2015. Lancet 2016;388:1545-602. [Crossref] [PubMed]
- Wong MC, Lao XQ, Ho KF, et al. Incidence and mortality of lung cancer: global trends and association with socioeconomic status. Sci Rep 2017;7:14300. [Crossref] [PubMed]
- Jha P, Peto R. Global effects of smoking, of quitting, and of taxing tobacco. N Engl J Med 2014;370:60-8. [Crossref] [PubMed]
- US-SEER 2018. Available online: https://seer.cancer.gov/report_to_nation/mortality.html
- Moyer VA. U.S. Preventive Services Task Force. Screening for lung cancer: U.S. Preventive Services Task Force recommendation statement. Ann Intern Med 2014;160:330-8. [PubMed]
- Wang Y, Midthun DE, Wampfler JA, et al. Trends in the proportion of patients with lung cancer meeting screening criteria. JAMA 2015;313:853-5. [Crossref] [PubMed]
- Yang P, Wang Y, Wampfler JA, et al. Trends in Subpopulations at High Risk for Lung Cancer. J Thorac Oncol 2016;11:194-202. [Crossref] [PubMed]
- Yang D, Zhang X, Powell CA, et al. Probability of cancer in high-risk patients predicted by the protein-based lung cancer biomarker panel in China: LCBP study. Cancer 2018;124:262-70. [Crossref] [PubMed]
- Gould MK, Donington J, Lynch WR, et al. Evaluation of individuals with pulmonary nodules: when is it lung cancer? Diagnosis and management of lung cancer, 3rd ed: American College of Chest Physicians evidence-based clinical practice guidelines. Chest 2013;143:e93S-120S.
- Soardi GA, Perandini S, Larici AR, et al. Multicentre external validation of the BIMC model for solid solitary pulmonary nodule malignancy prediction. Eur Radiol 2017;27:1929-33. [Crossref] [PubMed]
- Perandini S, Soardi GA, Motton M, et al. Solid pulmonary nodule risk assessment and decision analysis: comparison of four prediction models in 285 cases. Eur Radiol 2016;26:3071-6. [Crossref] [PubMed]
- Shinohara S, Hanagiri T, Takenaka M, et al. Evaluation of undiagnosed solitary lung nodules according to the probability of malignancy in the American College of Chest Physicians (ACCP) evidence-based clinical practice guidelines. Radiol Oncol 2014;48:50-5. [Crossref] [PubMed]
- Soardi GA, Perandini S, Motton M, et al. Assessing probability of malignancy in solid solitary pulmonary nodules with a new Bayesian calculator: improving diagnostic accuracy by means of expanded and updated features. Eur Radiol 2015;25:155-62. [Crossref] [PubMed]