Blood based biomarkers beyond genomics for lung cancer screening
Introduction
A major contributing factor to high mortality in lung cancer is that most cases are diagnosed at advanced stages with limited benefits from currently available therapies, hence the interest in implementing screening strategies to detect lung cancer at an early stage. The findings from the National Lung Screening Trial (NLST) study, which demonstrated a 20% reduction in lung cancer mortality with low-dose CT (LDCT) screening of subjects aged 55–74 years with a 30-pack-year smoking history, have generated interest in lung cancer screening (1). Based on these findings the US Preventive Services Task Force recommended LDCT screening of subjects that meet the NLST criteria. However the adoption of lung cancer screening has been quite modest and a cautious approach to screening has been advocated (2). Concerns about the high percentage of false positives and potential health hazards resulting from exposure to radiation with repeat LDCTs as well as concern about the fact that a majority of subjects destined to be diagnosed with lung cancer are currently not eligible for screening have heightened the need to identify subjects at risk for developing lung cancer that do not meet current eligibility criteria. Biomarkers offer the potential for precision medicine across the cancer continuum from risk assessment to early detection and tumor classification (3). For lung cancer in particular, approaches in which a biomarker based initial screen is followed by LDCT or in which a biomarker test is combined with LDCT have merit for early detection applications (4,5).
Sources and types of biomarkers for lung cancer detection
In principle, biomarkers that may aid in identifying subjects that may benefit from LDCT or that complement LDCT such as for assessment of indeterminate nodules could be found in various biological fluids notably blood, urine, saliva or sputum (6-8). Exhaled breath has also been explored as a source of biomarkers (9-11) as well airway brushing (12). Blood in particular, with its cellular, micro-particulate, and plasma constituents, represents a rich source of biomarkers.
Although considerable attention is focused on liquid biopsy approaches for lung cancer detection (13), it is likely that no single marker or approach will be useful alone, hence the need to explore combinations of markers and approaches to determine their performance. This undertaking represents a daunting effort. There are thousands of publications in the literature pertaining to potential lung cancer biomarkers. Testing biomarker candidates singly or in combination with informative biospecimens relevant to the intended application is generally beyond the means of individual laboratories and requires a collaborative effort as currently being undertaken through the National Cancer Institute Early Detection Research Network. Here, we limit the review to circulating protein, metabolite and humoral immune response based biomarkers that have potential for screening and early detection of lung cancer.
Autoantibodies
A humoral immune response in the form of autoantibodies to tumor antigens occurs early during tumor development. Identification of antigens that induce a selective autoantibody response in lung cancer has the potential to yield markers useful for early detection applications. A recent PubMed search of autoantibodies in lung cancer yielded 1,090 publications. A sampling of autoantibody-based biomarker combinations that have been published is provided in Table 1. A review of the literature found that the most commonly used detection method was enzyme-linked immunoassay (ELISA), applied in 31 out of 38 tests for single autoantibodies and in 33 out of 49 tests for multiple autoantibodies. Multiplex assays are exemplified in a panel of seven autoantibodies to tumor antigens which yielded improved sensitivity compared to individual autoantibodies or smaller panels (32).
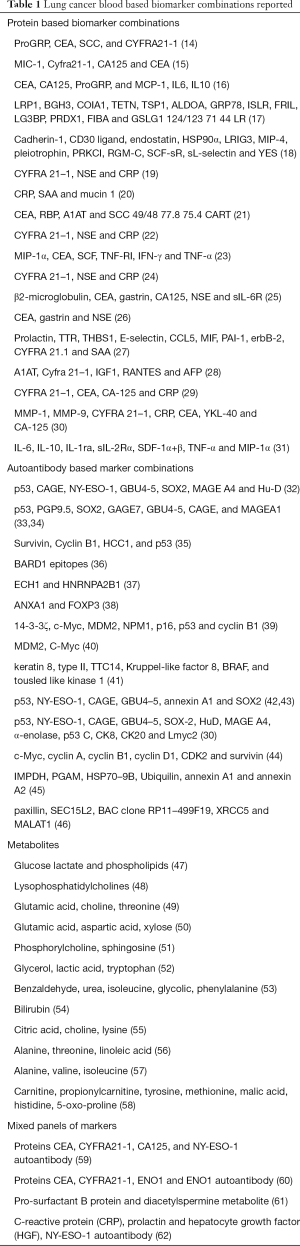
Full table
Another study involved a panel of 25 serum autoantibodies associated with non-small cell lung cancer (NSCLC) that were tested in a protein microarray format containing the autoantigens using sera from 125 patients with NSCLC and 125 matched controls with a benign nodule (63). In the training data set the logistic regression c-index statistic was 0.691 and 0.490 in the test set. The relationship between the score and outcome was not statistically significant (P=0.460). A meta-analysis of 31 articles with single autoantibodies and 39 with multiplex autoantibodies was recently done, supporting improved diagnostic accuracy with multiplex panels (64).
An ongoing study is intended to determine whether a panel of autoantibodies can identify subjects at high risk for lung cancer, followed by X-ray and computed tomography (CT) scanning, resulting in reduced incidence of patients with late-stage lung cancer (III & IV) or unclassified presentation at diagnosis, compared to standard practice (65). The study involves 12,000 participants aged 50–75 who are at high risk for lung cancer in Scotland. The intervention is a seven-autoantibody test, followed by X-ray and CT for subjects with a positive result. The primary outcome is the difference, after 24 months, between the rates of patients with stage III, IV or unclassified lung cancer at diagnosis. Participants with a positive test result but for whom the CT scan does not lead to a lung cancer diagnosis will be offered follow-up CTs for 24 months. An initial chest X-ray will be used to determine the urgency and the need for intravenous contrast in the first screening CT. Participants who are found to have lung cancer will be followed to assess both time to diagnosis and stage of disease at diagnosis.
From a practical perspective a biomarker approach based on autoantibodies is appealing as antigenic targets could be printed or synthesized on a chip. Hybridization to detect autoantibody reactivity would require no more blood volume than a drop of blood. Additionally, with the availability of autoantibody marker panels, each having specificity to one of the common cancers, a chip could be produced that would allow screening across cancer types. However, at present, the definitive validation of the utility of autoantibody biomarkers for screening either alone or in combination with other markers has not been demonstrated.
Protein based biomarker panels
A wide world of technologies is currently available for the discovery of protein markers as well as for the development of corresponding assays with applications to lung cancer (Table 1). Widely used approaches consist of multiple forms of mass spectrometry for both discovery and assay development and the use of antibody arrays for discovery and ELISA for assay development either singly or in multiplex formats.
At present time, several protein-based biomarkers are available in the clinic that are primarily used for diagnosis or for patient monitoring. These assays are particularly suited for subjects with symptoms or for imaging-based findings including stratification of indeterminate nodules. Guidelines for the management of subjects with nodules detected through CT screening are evolving. The National Comprehensive Cancer Network (NCCN) provides guidelines that avoid invasive follow-up for small nodules with low probably of malignancy (NCCN.org). The guidelines incorporate recommendations based on the NLST, the International Early Lung Cancer Action Program protocols and the Fleischner Society guidelines. Reporting systems have been suggested that classify nodules observed with screening CTs based on the risk of malignancy which is linked to suggested follow-up [American College of Radiology. Lung CT Screening Reporting and Data System (Lung-RADS). Available at: http://www.acr.org/Quality-Safety/Resources/LungRADS] (66). A recent study encompassed 691 patients with pulmonary lesions from three centers in China and was intended to develop and validate a nomogram model based on clinically available tumor markers to assess the likelihood of cancer for patients with pulmonary lesions (67). The predictive performance of the nomogram was measured by concordance index and calibrated with 1,000 bootstrap samples to reduce over-fitting. A multivariate logistic regression model identified tumor size, CEA, SCC, CYFRA21-1, pro-GRP, and HE4 as independent risk factors for lung cancer. The nomogram developed showed concordance index of 0.901 (95% CI: 0.842–0.960; P<0.001) for lung cancer in the training set and 0.713 (95% CI: 0.599–0.827; P<0.001) in the validation set.
A multitude of other studies have explored the contribution of existing protein lung cancer biomarkers as well as additional markers resulting from discovery studies. One group utilizing mass spectrometry developed a 13-protein blood-based classifier that differentiated malignant and benign nodules with high confidence (17). The group identified 371 protein candidates and developed a mass spectrometry based multiple reaction monitoring assay for each. The classifier was validated on an independent set of plasma samples with good performance. The source and the relationship of the tumor markers to tumor characteristics was not clear. In a subsequent study, an integrated model incorporating clinical risk factors and proteomic molecular markers was developed and its performance assessed on a subset of 222 lung nodules, between 8 and 20 mm in diameter (68). Whereas the protein markers were found to be most predictive, the integration of protein markers with clinical features was found to be superior. Rigorous independent validation of these markers is required before such a test is adopted.
Metabolites as lung cancer biomarkers
Comprehensive metabolomic profiling using high performance liquid chromatography coupled with tandem mass spectrometry (LC-MS/MS), encompassing a few thousand identified metabolites covering metabolic pathways relevant to health and disease has become well established. Metabolomic profiling offers a dynamic view of cell tissue and organismal function. Active effort is ongoing in the search for metabolomics biomarkers that have relevance to lung cancer detection (69) (Table 1).
Phospholipids are important constituents of the cell membrane. Phospholipid metabolic pathways are upregulated in lung cancer yielding distinct signatures (70). A blood based metabolomics study was conducted as part of lung cancer screening (71). Analysis encompassed 31 patients with screen detected lung cancer and a matched group of 92 healthy controls. Several metabolites in serum discriminated patients from controls. Most were downregulated in cancer samples, including amino acids, carboxylic acids and tocopherols. Benzaldehyde was the only compound found to be significantly upregulated. A follow-up study based on lipid profiling encompassed 100 patients with early stage lung cancer, including 31 screen-detected cases and a matched group of 300 healthy screened subjects (48). Downregulation of lysophosphatidylcholines (LPC18:2, LPC18:1 and LPC18:0) distinguished cases from controls. Other studies have uncovered a diversity of metabolites that distinguished cases from controls (Table 1) thus necessitating rigorous validation studies that address specific intended clinical application(s) for their validation.
Modeling lung cancer risk based on subject’s characteristics and biomarker profile
An intended goal of molecular testing is to identify subjects that would benefit from LDCT. There is not a currently recommended risk prediction model for lung cancer for general use. Several models based on subject’s characteristics have been proposed (72-74). The addition of pulmonary function has been shown to improve risk prediction (75,76). Cotinine, a circulating metabolite of nicotine has been found to be associated with lung cancer risk (77), and may represent a more sensitive assessment of smoking intensity than self-reporting.
The contribution of inflammatory markers to lung cancer risk has been explored. Circulating levels of CRP, SAA, sTNFRII, and CXCL9/MIG were found to be associated with lung cancer risk in the Prostate, Lung, Colorectal, and Ovarian Cancer Screening Trial (78). However, these markers did not improve lung cancer risk stratification beyond models based on subject characteristics. Cytokine profiling has revealed an increased risk of lung cancer associated with high levels of IL6 and IL8 (79). A causal role of fasting insulin and low-density lipoprotein cholesterol in lung cancer etiology, and for BMI in squamous cell and small cell carcinoma, has been suggested (80).
Vitamin status has been associated with risk of numerous diseases including cancer (81,82). A nested case-control study within the European Prospective Investigation into Cancer and Nutrition provided strong evidence that increased vitamin B6 catabolism is independently associated with a higher risk of future lung cancer (83). A recent study of circulating folate, vitamin B6, and methionine found that subjects with higher circulating concentrations of vitamin B6 and folate had a modestly decreased risk of lung cancer (82). The kynurenine/tryptophan ratio has also been found to be associated with increased risk of lung cancer (84). A study that involved a prospective cohort of 395,875 participants in Taiwan was conducted to improve risk prediction models for lung cancer by incorporating biomarkers (85). Age, gender, smoking pack-years, family history of lung cancer, personal cancer history, BMI, lung function, and serum biomarkers were included as variables. The area under the curve (AUC) in overall population was 0.851 (95% CI: 0.840–0.862), with never smokers 0.806 (95% CI: 0.790–0.819), light smokers 0.847 (95% CI: 0.824–0.871), and heavy smokers 0.732 (95% CI: 0.708–0.752). By integrating risk factors together with CEA and AFP for light smokers, and lung function testing, AFP, and CEA for light and never smokers, cancer risks as high as those for heavy smokers could be identified. The risk model for heavy smokers allowed stratification of heavy smokers into subgroups with distinct risks.
Few studies have assessed the contribution of biomarkers based on rigorous validation studies using lung cancer screening cohorts. Proteomic studies have led to the identification of several candidate markers associated with the development of lung cancer including pro-surfactant protein B (pro-SFTPB), a target of NKX2-1, a lineage survival oncogene in lung cancer (86). Multivariable logistic regression models were used to evaluate the predictive ability of pro-SFTPB among subjects undergoing LDCT screening. The AUC values of the full model with and without pro-SFTPB were 0.741 (95% CI: 0.696 to 0.783) and 0.669 (95% CI: 0.620 to 0.717), respectively (difference in AUC, P=0.0002) (87). (Figure 1A) In a related study, a mass spectrometry method designed to profile a wide range of metabolites was applied to pre-diagnostic serum samples from a high-risk cohort that encompassed 100 subjects who subsequently developed NSCLC and 199 matched controls. A separate aliquot was used to quantify levels of pro-SFTPB (61). N1, N12-diacetylspermine was identified as a metabolite with significant increase in concentration in samples from cases compared to controls. A validation study of DAS and pro-SFTPB was performed using an independent set of pre-diagnostic sera from 108 patients with NSCLC and 216 matched controls. DAS significantly complemented performance of pro-SFTPB in both the discovery and validations sets, with a combined area under the curve in the validation set of 0.808 (P=0.001 DAS + pro-SFTPB vs. pro-SFTPB alone) (Figure 1B).
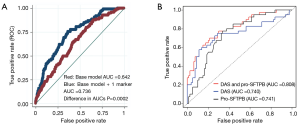
While these risk marker studies show promise for identifying subjects with increased risk based on their biomarker profiles that otherwise may not be eligible for screening, much work remains to be done to determine an optimal, cost effective approach to assess risk based on a combination of biomarkers and subject characteristics.
Conclusions
It is likely that biomarker panels will emerge in the near future that have relevance to lung cancer early detection that will be useful in clinical practice. As experience is gained, it is also likely that improvement in biomarker testing will ensue through better biomarker combinations or through increased understanding of the population(s) that would best benefit from tailoring of biomarkers, for instance patients with COPD, light smokers ineligible for LDCT-based screening, or those with indeterminate nodules discovered incidentally or after LDCT-based screening. Biomarker panels will need careful validation among populations relevant to their intended application, which will be greatly facilitated by multi-center cooperation between lung cancer screening programs, ongoing clinical trials, and speciality diagnostic clinics.
Acknowledgements
This work was supported through the MD Anderson Moonshot Program.
Footnote
Conflicts of Interest: The authors have no conflicts of interest to declare.
References
- Ostrowski M, Marjanski T, Rzyman W. Low-dose computed tomography screening reduces lung cancer mortality. Adv Med Sci 2018;63:230-6. [Crossref] [PubMed]
- Reich JM, Kim JS. Five reasons for caution in advocating low-dose computerized tomographic lung cancer screening. J Thorac Dis 2017;9:3433-6. [Crossref] [PubMed]
- Vargas AJ, Harris CC. Biomarker development in the precision medicine era: lung cancer as a case study. Nat Rev Cancer 2016;16:525-37. [Crossref] [PubMed]
- Hasan N, Kumar R, Kavuru MS. Lung cancer screening beyond low-dose computed tomography: the role of novel biomarkers. Lung 2014;192:639-48. [Crossref] [PubMed]
- Holdenrieder S. Biomarkers along the continuum of care in lung cancer. Scand J Clin Lab Invest Suppl 2016;245:S40-5. [Crossref] [PubMed]
- Carozzi FM, Bisanzi S. Molecular biomarkers and early diagnosis of lung cancer: state of knowledge and future perspectives. Epidemiol Prev 2016;40:56-63. [PubMed]
- Cameron SJ, Lewis KE, Beckmann M, et al. The metabolomic detection of lung cancer biomarkers in sputum. Lung Cancer 2016;94:88-95. [Crossref] [PubMed]
- Haznadar M, Cai Q, Krausz KW, et al. Urinary Metabolite Risk Biomarkers of Lung Cancer: A Prospective Cohort Study. Cancer Epidemiol Biomarkers Prev 2016;25:978-86. [Crossref] [PubMed]
- Peralbo-Molina A, Calderon-Santiago M, Priego-Capote F, et al. Identification of metabolomics panels for potential lung cancer screening by analysis of exhaled breath condensate. J Breath Res 2016;10:026002. [Crossref] [PubMed]
- Chen X, Wang F, Lin L, et al. Association of Smoking with Metabolic Volatile Organic Compounds in Exhaled Breath. Int J Mol Sci 2017.18. [PubMed]
- López-Sánchez LM, Jurado-Gamez B, Feu-Collado N, et al. Exhaled breath condensate biomarkers for the early diagnosis of lung cancer using proteomics. Am J Physiol Lung Cell Mol Physiol 2017;313:L664-76. [Crossref] [PubMed]
- Beane J, Mazzilli SA, Tassinari AM, et al. Detecting the Presence and Progression of Premalignant Lung Lesions via Airway Gene Expression. Clin Cancer Res 2017;23:5091-100. [Crossref] [PubMed]
- Hofman P. Liquid biopsy for early detection of lung cancer. Curr Opin Oncol 2017;29:73-8. [Crossref] [PubMed]
- Yang D, Zhang X, Powell CA, et al. Probability of cancer in high-risk patients predicted by the protein-based lung cancer biomarker panel in China: LCBP study. Cancer 2018;124:262-70. [Crossref] [PubMed]
- Wang X, Zhi X, Yang Z, et al. A novel serum based biomarker panel has complementary ability to preclude presence of early lung cancer for low dose CT (LDCT). Oncotarget 2017;8:45345-55. [PubMed]
- Pan YW, Zhou ZG, Wang M, et al. Combination of IL-6, IL-10, and MCP-1 with traditional serum tumor markers in lung cancer diagnosis and prognosis. Genet Mol Res 2016;15. [PubMed]
- Li XJ, Hayward C, Fong PY, et al. A blood-based proteomic classifier for the molecular characterization of pulmonary nodules. Sci Transl Med 2013;5:207ra142. [Crossref] [PubMed]
- Ostroff RM, Bigbee WL, Franklin W, et al. Unlocking biomarker discovery: large scale application of aptamer proteomic technology for early detection of lung cancer. PLoS One 2010;5:e15003. [Crossref] [PubMed]
- Schneider J, Bitterlich N, Velcovsky HG, et al. Fuzzy logic-based tumor-marker profiles improved sensitivity in the diagnosis of lung cancer. Int J Clin Oncol 2002;7:145-51. [Crossref] [PubMed]
- Gao WM, Kuick R, Orchekowski RP, et al. Distinctive serum protein profiles involving abundant proteins in lung cancer patients based upon antibody microarray analysis. BMC Cancer 2005;5:110. [Crossref] [PubMed]
- Patz EF Jr, Campa MJ, Gottlin EB, et al. Panel of serum biomarkers for the diagnosis of lung cancer. J Clin Oncol 2007;25:5578-83. [Crossref] [PubMed]
- Schneider J, Bitterlich N, Kotschy-Lang N, et al. A fuzzy-classifier using a marker panel for the detection of lung cancers in asbestosis patients. Anticancer Res 2007;27:1869-77. [PubMed]
- Borgia JA, Basu S, Faber LP, et al. Establishment of a multi-analyte serum biomarker panel to identify lymph node metastases in non-small cell lung cancer. J Thorac Oncol 2009;4:338-47. [Crossref] [PubMed]
- Schneider J. Detection of lung cancer in silicosis patients using a tumor-marker panel. Cancer Biomark 2010;6:137-48. [Crossref] [PubMed]
- Wu Y, Wu Y, Wang J, et al. An Optimal tumor marker group-coupled artificial neural network for diagnosis of lung cancer. Experts Systems with Applications 2011;38:11329-34. [Crossref]
- Feng F, Wu Y, Wu Y, et al. The effect of artificial neural network model combined with six tumor markers in auxiliary diagnosis of lung cancer. J Med Syst 2012;36:2973-80. [Crossref] [PubMed]
- Bigbee WL, Gopalakrishnan V, Weissfeld JL, et al. A multiplexed serum biomarker immunoassay panel discriminates clinical lung cancer patients from high-risk individuals found to be cancer-free by CT screening. J Thorac Oncol 2012;7:698-708. [Crossref] [PubMed]
- Lee HJ, Kim YT, Park PJ, et al. A novel detection method of non-small cell lung cancer using multiplexed bead-based serum biomarker profiling. J Thorac Cardiovasc Surg 2012;143:421-7. [Crossref] [PubMed]
- Flores-Fernández JM, Herrera-López EJ, Sánchez-Llamas F, et al. Development of an optimized multi-biomarker panel for the detection of lung cancer based on principal component analysis and artificial neural network modeling. Experts Systems with Applications 2012;39:10851-6. [Crossref]
- Flores JM, Herrera-López E, Leal G, et al. Artificial Neural Network-Based Serum Biomarkers Analysis Improves Sensitivity in the Diagnosis of Lung Cancer. CLAIB 2011 IFMBE Proceedings 2013;33:882-5. [Crossref]
- Daly S, Rinewalt D, Fhied C, et al. Development and validation of a plasma biomarker panel for discerning clinical significance of indeterminate pulmonary nodules. J Thorac Oncol 2013;8:31-6. [Crossref] [PubMed]
- Chapman CJ, Healey GF, Murray A, et al. EarlyCDT(R)-Lung test: improved clinical utility through additional autoantibody assays. Tumour Biol 2012;33:1319-26. [Crossref] [PubMed]
- Du Q, Yu R, Wang H, et al. Significance of tumor-associated autoantibodies in the early diagnosis of lung cancer. Clin Respir J 2018. [Epub ahead of print]. [Crossref] [PubMed]
- Ren S, Zhang S, Jiang T, et al. Early detection of lung cancer by using an autoantibody panel in Chinese population. Oncoimmunology 2017;7:e1384108. [Crossref] [PubMed]
- Li P, Shi JX, Xing MT, et al. Evaluation of serum autoantibodies against tumor-associated antigens as biomarkers in lung cancer. Tumour Biol 2017;39:1010428317711662. [Crossref] [PubMed]
- Pilyugin M, Descloux P, Andre PA, et al. BARD1 serum autoantibodies for the detection of lung cancer. PLoS One 2017;12:e0182356. [Crossref] [PubMed]
- Dai L, Li J, Tsay JJ, et al. Identification of autoantibodies to ECH1 and HNRNPA2B1 as potential biomarkers in the early detection of lung cancer. Oncoimmunology 2017;6:e1310359. [Crossref] [PubMed]
- Wang W, Zhong W, Chen C, et al. Circulating Antibodies to Linear Peptide Antigens Derived from ANXA1 and FOXP3 in Lung Cancer. Anticancer Res 2017;37:3151-5. [PubMed]
- Dai L, Tsay JC, Li J, et al. Autoantibodies against tumor-associated antigens in the early detection of lung cancer. Lung Cancer 2016;99:172-9. [Crossref] [PubMed]
- Li P, Shi JX, Dai LP, et al. Serum anti-MDM2 and anti-c-Myc autoantibodies as biomarkers in the early detection of lung cancer. Oncoimmunology 2016;5:e1138200. [Crossref] [PubMed]
- Wang J, Shivakumar S, Barker K, et al. Comparative Study of Autoantibody Responses between Lung Adenocarcinoma and Benign Pulmonary Nodules. J Thorac Oncol 2016;11:334-45. [Crossref] [PubMed]
- Boyle P, Chapman CJ, Holdenrieder S, et al. Clinical validation of an autoantibody test for lung cancer. Ann Oncol 2011;22:383-9. [Crossref] [PubMed]
- Lam S, Boyle P, Healey GF, et al. EarlyCDT-Lung: an immunobiomarker test as an aid to early detection of lung cancer. Cancer Prev Res (Phila) 2011;4:1126-34. [Crossref] [PubMed]
- Rom WN, Goldberg JD, Addrizzo-Harris D, et al. Identification of an autoantibody panel to separate lung cancer from smokers and nonsmokers. BMC Cancer 2010;10:234. [Crossref] [PubMed]
- Farlow EC, Patel K, Basu S, et al. Development of a multiplexed tumor-associated autoantibody-based blood test for the detection of non-small cell lung cancer. Clin Cancer Res 2010;16:3452-62. [Crossref] [PubMed]
- Zhong L, Coe SP, Stromberg AJ, et al. Profiling tumor-associated antibodies for early detection of non-small cell lung cancer. J Thorac Oncol 2006;1:513-9. [Crossref] [PubMed]
- Louis E, Adriaensens P, Guedens W, et al. Detection of Lung Cancer through Metabolic Changes Measured in Blood Plasma. J Thorac Oncol 2016;11:516-23. [Crossref] [PubMed]
- Ros-Mazurczyk M, Jelonek K, Marczyk M, et al. Serum lipid profile discriminates patients with early lung cancer from healthy controls. Lung Cancer 2017;112:69-74. [Crossref] [PubMed]
- Puchades-Carrasco L, Jantus-Lewintre E, Perez-Rambla C, et al. Serum metabolomic profiling facilitates the non-invasive identification of metabolic biomarkers associated with the onset and progression of non-small cell lung cancer. Oncotarget 2016;7:12904-16. [Crossref] [PubMed]
- Fahrmann JF, Kim K, DeFelice BC, et al. Investigation of metabolomic blood biomarkers for detection of adenocarcinoma lung cancer. Cancer Epidemiol Biomarkers Prev 2015;24:1716-23. [Crossref] [PubMed]
- Chen Y, Ma Z, Min L, et al. Biomarker identification and pathway analysis by serum metabolomics of lung cancer. Biomed Res Int 2015;2015:183624. [PubMed]
- Miyamoto S, Taylor SL, Barupal DK, et al. Systemic Metabolomic Changes in Blood Samples of Lung Cancer Patients Identified by Gas Chromatography Time-of-Flight Mass Spectrometry. Metabolites 2015;5:192-210. [Crossref] [PubMed]
- Louis E, Cantrelle FX, Mesotten L, et al. Metabolic phenotyping of human plasma by (1) H-NMR at high and medium magnetic field strengths: a case study for lung cancer. Magn Reson Chem 2017;55:706-13. [Crossref] [PubMed]
- Wen CP, Zhang F, Liang D, et al. The ability of bilirubin in identifying smokers with higher risk of lung cancer: a large cohort study in conjunction with global metabolomic profiling. Clin Cancer Res 2015;21:193-200. [Crossref] [PubMed]
- Deja S, Porebska I, Kowal A, et al. Metabolomics provide new insights on lung cancer staging and discrimination from chronic obstructive pulmonary disease. J Pharm Biomed Anal 2014;100:369-80. [Crossref] [PubMed]
- Wen T, Gao L, Wen Z, et al. Exploratory investigation of plasma metabolomics in human lung adenocarcinoma. Mol Biosyst 2013;9:2370-8. [Crossref] [PubMed]
- Maeda J, Higashiyama M, Imaizumi A, et al. Possibility of multivariate function composed of plasma amino acid profiles as a novel screening index for non-small cell lung cancer: a case control study. BMC Cancer 2010;10:690. [Crossref] [PubMed]
- Klupczynska A, Derezinski P, Garrett TJ, et al. Study of early stage non-small-cell lung cancer using Orbitrap-based global serum metabolomics. J Cancer Res Clin Oncol 2017;143:649-59. [Crossref] [PubMed]
- Mazzone PJ, Wang XF, Han X, et al. Evaluation of a Serum Lung Cancer Biomarker Panel. Biomark Insights 2018;13:1177271917751608. [Crossref] [PubMed]
- Dai L, Qu Y, Li J, et al. Serological proteome analysis approach-based identification of ENO1 as a tumor-associated antigen and its autoantibody could enhance the sensitivity of CEA and CYFRA 21-1 in the detection of non-small cell lung cancer. Oncotarget 2017;8:36664-73. [Crossref] [PubMed]
- Wikoff WR, Hanash S, DeFelice B, et al. Diacetylspermine Is a Novel Prediagnostic Serum Biomarker for Non-Small-Cell Lung Cancer and Has Additive Performance With Pro-Surfactant Protein B. J Clin Oncol 2015;33:3880-6. [Crossref] [PubMed]
- Ma S, Wang W, Xia B, et al. Multiplexed Serum Biomarkers for the Detection of Lung Cancer. EBioMedicine 2016;11:210-8. [Crossref] [PubMed]
- Campa MJ, Gottlin EB, Herndon JE 2nd, et al. Rethinking Autoantibody Signature Panels for Cancer Diagnosis. J Thorac Oncol 2017;12:1011-4. [Crossref] [PubMed]
- Tang ZM, Ling ZG, Wang CM, et al. Serum tumor-associated autoantibodies as diagnostic biomarkers for lung cancer: A systematic review and meta-analysis. PLoS One 2017;12:e0182117. [Crossref] [PubMed]
- Sullivan FM, Farmer E, Mair FS, et al. Detection in blood of autoantibodies to tumour antigens as a case-finding method in lung cancer using the EarlyCDT(R)-Lung Test (ECLS): study protocol for a randomized controlled trial. BMC Cancer 2017;17:187. [Crossref] [PubMed]
- Manos D, Seely JM, Taylor J, et al. The Lung Reporting and Data System (LU-RADS): a proposal for computed tomography screening. Can Assoc Radiol J 2014;65:121-34. [Crossref] [PubMed]
- Du Q, Yan C, Wu SG, et al. Development and validation of a novel diagnostic nomogram model based on tumor markers for assessing cancer risk of pulmonary lesions: a multicenter study in Chinese population. Cancer Lett 2018;420:236-41. [Crossref] [PubMed]
- Kearney P, Hunsucker SW, Li XJ, et al. An integrated risk predictor for pulmonary nodules. PLoS One 2017;12:e0177635. [Crossref] [PubMed]
- Yu L, Li K, Zhang X. Next-generation metabolomics in lung cancer diagnosis, treatment and precision medicine: mini review. Oncotarget 2017;8:115774-86. [Crossref] [PubMed]
- Rocha CM, Barros AS, Gil AM, et al. Metabolic profiling of human lung cancer tissue by 1H high resolution magic angle spinning (HRMAS) NMR spectroscopy. J Proteome Res 2010;9:319-32. [Crossref] [PubMed]
- Roś-Mazurczyk M, Wojakowska A, Marczak L, et al. Panel of serum metabolites discriminates cancer patients and healthy participants of lung cancer screening - a pilot study. Acta Biochim Pol 2017;64:513-8. [Crossref] [PubMed]
- Tammemägi MC. Application of risk prediction models to lung cancer screening: a review. J Thorac Imaging 2015;30:88-100. [Crossref] [PubMed]
- Spitz MR, Etzel CJ, Dong Q, et al. An expanded risk prediction model for lung cancer. Cancer Prev Res (Phila) 2008;1:250-4. [Crossref] [PubMed]
- Marcus MW, Chen Y, Raji OY, et al. LLPi: Liverpool Lung Project Risk Prediction Model for Lung Cancer Incidence. Cancer Prev Res (Phila) 2015;8:570-5. [Crossref] [PubMed]
- Tammemagi MC, Lam SC, McWilliams AM, et al. Incremental value of pulmonary function and sputum DNA image cytometry in lung cancer risk prediction. Cancer Prev Res (Phila) 2011;4:552-61. [Crossref] [PubMed]
- Muller DC, Johansson M, Brennan P. Lung Cancer Risk Prediction Model Incorporating Lung Function: Development and Validation in the UK Biobank Prospective Cohort Study. J Clin Oncol 2017;35:861-9. [Crossref] [PubMed]
- Timofeeva MN, McKay JD, Smith GD, et al. Genetic polymorphisms in 15q25 and 19q13 loci, cotinine levels, and risk of lung cancer in EPIC. Cancer Epidemiol Biomarkers Prev 2011;20:2250-61. [Crossref] [PubMed]
- Shiels MS, Katki HA, Hildesheim A, et al. Circulating Inflammation Markers, Risk of Lung Cancer, and Utility for Risk Stratification. J Natl Cancer Inst 2015;107. [PubMed]
- Brenner DR, Fanidi A, Grankvist K, et al. Inflammatory Cytokines and Lung Cancer Risk in 3 Prospective Studies. Am J Epidemiol 2017;185:86-95. [Crossref] [PubMed]
- Carreras-Torres R, Johansson M, Haycock PC, et al. Obesity, metabolic factors and risk of different histological types of lung cancer: A Mendelian randomization study. PLoS One 2017;12:e0177875. [Crossref] [PubMed]
- Johansson M, Relton C, Ueland PM, et al. Serum B vitamin levels and risk of lung cancer. JAMA 2010;303:2377-85. [Crossref] [PubMed]
- Fanidi A, Muller DC, Yuan JM, et al. Circulating Folate, Vitamin B6, and Methionine in Relation to Lung Cancer Risk in the Lung Cancer Cohort Consortium (LC3). J Natl Cancer Inst 2018;110. [PubMed]
- Zuo H, Ueland PM, Midttun O, et al. Results from the European Prospective Investigation into Cancer and Nutrition Link Vitamin B6 Catabolism and Lung Cancer Risk. Cancer Res 2018;78:302-8. [Crossref] [PubMed]
- Chuang SC, Fanidi A, Ueland PM, et al. Circulating biomarkers of tryptophan and the kynurenine pathway and lung cancer risk. Cancer Epidemiol Biomarkers Prev 2014;23:461-8. [Crossref] [PubMed]
- Wu X, Wen CP, Ye Y, et al. Personalized Risk Assessment in Never, Light, and Heavy Smokers in a prospective cohort in Taiwan. Sci Rep 2016;6:36482. [Crossref] [PubMed]
- Taguchi A, Politi K, Pitteri SJ, et al. Lung cancer signatures in plasma based on proteome profiling of mouse tumor models. Cancer Cell 2011;20:289-99. [Crossref] [PubMed]
- Sin DD, Tammemagi CM, Lam S, et al. Pro-surfactant protein B as a biomarker for lung cancer prediction. J Clin Oncol 2013;31:4536-43. [Crossref] [PubMed]