Computer Aided Nodule Analysis and Risk Yield (CANARY) characterization of adenocarcinoma: radiologic biopsy, risk stratification and future directions
Introduction
Adenocarcinoma of the lung encompasses a varied spectrum of disease with differing behavior, ranging from indolent lesions with essentially 100% post-resection disease-free survival (DFS) to aggressive cancer with poor outcomes despite early and appropriate surgery even in stage 1 disease (1,2). With implementation of the United States Preventative Services Task Force recommendation to screen high risk patients for lung cancer (3) we expect to identify more early-stage cancers—particularly from the adenocarcinoma spectrum (4). Computer Aided Nodule Analysis and Risk Yield (CANARY) is an automated quantitative CT analysis software that allows risk stratification of pulmonary nodules within the adenocarcinoma spectrum (5). Developed at Mayo Clinic, Rochester, MN, CANARY lung nodule characteristics have been shown to predict consensus histopathology (6). Further stratification of the type and volume of whole-nodule CANARY features has been shown to strongly correlate with post-resection DFS (7,8). Therefore, CANARY offers a noninvasive method of risk-stratifying these diverse tumors that may aid in clinical decision making and potentially surgical planning. In the case of multifocal adenocarcinoma of the lung, optimal surgical sequencing based on potential aggressiveness of each tumor may be considered. In this review we will detail CANARY’s development, role, validation and potential for clinical use. Lastly we will explore additional roles for CANARY and planned studies. We detail studies from our lab group that are works in progress, planned for submission or presently existing in abstract form. Please see Table 1 for a brief synopsis of published studies on CANARY to date.
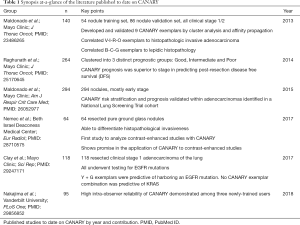
Full table
The need for computer-aided diagnostic tools
The National Lung Screening Trial (NLST) demonstrated a 20% decrease in lung cancer related morality with annual screening via low-dose high resolution CT chest in patients with ≥30 pack years of tobacco use within 15 years of quitting or ongoing smoking, aged 55–74 years old. This came with, however, a false positive rate of 96% (5). Though many of these findings were managed conservatively—it is unclear whether community practice at large would be able to safely maintain the same restraint in nodule follow-up and management. Early data detailing lung cancer screening in the Veterans Health Administration system suggests an even higher false positive rate (9). A computer-aided decision tool such as CANARY may help add decision support in initial management coupled with careful clinical judgement.
Another challenge inherent in lung cancer screening is the relatively high rate of indolent cancers that may never affect the patient during his or her life time (i.e., overdiagnosis). Nodules within the adenocarcinoma spectrum are heterogeneous in histopathology, behavior and radiologic appearance (10,11). Adenocarcinoma is divided into three distinct pathologic subtypes defined by degree of parenchymal invasion: adenocarcinoma in situ (AIS) with no invasion, minimally-invasive adenocarcinoma (MIA) with ≤5 mm invasive focus and invasive adenocarcinoma (IA) with >5 mm invasive focus (12). Lepidic growth describes tumor growth along existing alveolar structures without destruction of the underlying lung architecture. More indolent cancers—AIS and MIA—are characterized by high proportions of lepidic growth which is thought to present radiologically as ground glass opacity and have post-resection DFS approaching 100%—distinctly different than IA (2,11).
Analysis of the NSLT cohort revealed that up to 18% of the screen-detected cancers may be “over-diagnosed (13)”. This typically refers to tumors with a volume doubling time (VDT) of 400 days or more (14). Overdiagnosis is a controversial issue in lung cancer, particularly given that invasive lung cancer can be highly aggressive and cancers diagnosed at advanced stage have a 5-year survival rate of around 18% (15). Nonetheless, identification of cancers with a more indolent clinical course than their clinically-detected counterparts seems to be a relatively uncontroversial feature of lung cancer screening (14,16). Adenocarcinoma has a higher VDT when compared with squamous cell carcinoma (303 versus 77 days from LDCT screening data) and the vast majority of lung cancers that a patient could potentially live with and not die from will fall within the adenocarcinoma spectrum (14,17). This begs the question of how to define overdiagnosis and how to gauge disease-specific mortality from lung cancer against therapeutic morbidity, mortality and all-cause mortality. Lung cancer patients may have extensive tobacco-related comorbidity (18), such as heart disease and COPD/emphysema, so what then is the benefit from a curative surgery to remove an indolent neoplasm? The increased morbidity and potential quality of life changes due to lung resection or other intervention, the low risk that the lung cancer would advance in stage or cause mortality and the cost of therapy vs. active surveillance all raise questions about management strategy. It is probably most acceptable to consider ‘overdiagnosis’ as the subset of indolent adenocarcinomas that many patients will die with—not die from (17), but clinical reality is complex. A uniform approach to treatment of these lesions via standard lobectomy seems accordingly unwarranted hence the impetus for developing biomarkers including non-invasive quantitative imaging tools such as CANARY that can aid in clinical decision support and patient management.
Development of CANARY
CANARY was developed from analysis of historical data at Mayo Clinic, Rochester, MN by the Biomedical Imaging Resource. Robust historical data including patients with resected adenocarcinoma spanning the spectrum from indolent to aggressive histopathology was analyzed. Full imaging, histopathology and survival data for 54 pulmonary nodules of the adenocarcinoma spectrum between 2008–2010 of the adenocarcinoma spectrum served as the training set. Another 86 nodules resected from 80 patients between 2006–2007 served as the independent validation set. All cases had preoperative non-contrast HRCT chest within 3 months prior to resection. Nodule histopathology was scored in detail by two expert lung pathologists for percent lepidic growth present, invasion and histologic subtype (6).
An expert thoracic radiologist (BB) arbitrary selected 774 regions of interest (ROI, size =9×9 voxels) from 37 nodules (of the 54 nodule training set) spanning the radiologic spectrum from pure ground glass to solid density. Density-based histograms from each of the 774 ROIs were compared by a similarity metric and clustered with affinity propagation techniques (19). This process resulted in automatic (independent of human operator influence) sorting of the histograms into nine clusters that represent the spectrum of CT characteristics of an adenocarcinoma. The exemplar at the centroid of each cluster was considered a canonical CANARY feature, and each feature type was arbitrarily color coded Violet (V), Indigo (I), Red (R), Orange (O), Yellow (Y), Pink (P), Blue (B), Cyan (C) and Green (G)—making up the nine CANARY exemplars.
To analyze the entirety of a nodule prospectively, a segmentation tool was used to extract the nodule from the CT volume. This segmentation technique included constrained region growing from a seed in the nodule and manual intervention to correct or exclude structures such as the chest wall, vessels or other anatomy outside of the margin of the nodule. Any regions not automatically included in the nodule volume could be manually added through manual tracing operations on a slice by slice basis. The voxels within the segmentation defined the nodule volume (20,21). Each pixel of the nodule volume is classified by comparing the histogram characteristics of each pixel’s 9×9 voxel neighborhood to the CANARY exemplars (V, I, R, O, Y, P, B, C, and G) in a pairwise fashion and assigning the color code of the most similar exemplar. This process yields a comprehensively color-coded nodule that can be visualized as an overlay on the original images, a 3D representation, quantification of features in a spreadsheet or summarized as a representative glyph that demonstrates the overall volume and relative proportions of each exemplar present in the nodule volume (Figure 1) (6,7).
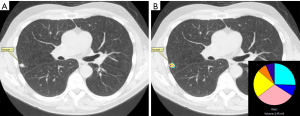
Radiologic biopsy
All of the CANARY features of the nodules in the training set were analyzed for similarity using multidimensional scaling and cluster analysis by affinity propagation. This demonstrated three natural clusters of the CANARY exemplars: V-I-R-O, Y-P and B-C-G. Furthermore it was shown that the V-I-R-O cluster correlated with invasive histopathology and the B-C-G cluster correlated with lepidic histopathology. This allowed creation of a decision tool to classify nodules as AIS, MIA or AI based on the relative proportions of the CANARY features. Using a decision tree primarily driven by the % presence of V-I-R-O, a nodule could be confidently classified as AIS, MIA or IA.
Sixteen nodules from the training set spanning from 0 to 100% lepidic growth were then analyzed by multinomial regression analysis to generate predictive equations for CANARY exemplars to determine invasiveness. Correlation was high in the training set, and remained high in the 38 remaining nodules in the training and validation sets: Spearman R =0.89, CI: 0.83–0.93; P<0.0001. All nodules were scored by thoracic radiologists as AIS, MIA or IA to allow comparison of radiologist opinion with CANARY’s ability to predict underlying histopathology. CANARY demonstrated superior sensitivity and specificity compared to expert radiologist opinion or more detailed analysis of the consolidation/tumor (C/T) ratio (6,10). This supports an intimate connection of the CANARY exemplars to histopathology in adenocarcinoma—a key finding given that histopathology describes tumor behavior in adenocarcinoma of the lung (Figure 2) (2,12).
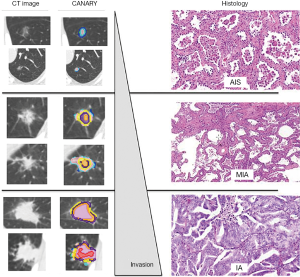
Risk stratification
Given the ability to use nodule CT histogram features to predict histopathology of adenocarcinoma of the lung, we tested CANARY’s ability to determine disease-free survival (DFS) after definitive resection. We identified a total of 306 resected adenocarcinomas of the lung including 264 clinical stage 1 nodules between 2006 and 2009 with 170 nodules (128 of which were clinically stage 1) serving at the training set. All nodules had curative (RO) resection. Each nodule was analyzed by CANARY and the resulting nodule glyphs were allowed to cluster by affinity propagation, sorting into 3 distinct nodule clusters:
- Cluster 1: predominantly B-C-G;
- Cluster 2: mixed;
- Cluster 3: predominantly V-I-R-O.
Next all 264 of the clinical stage 1 nodules were categorized into the aforementioned clusters. DFS free survival was analyzed for each cluster by Kaplan-Meier. Median follow-up was 3.07 years. Clustering was significant by analysis of similarity (ANOSIM R =0.59, P=0.001). DFS information was extracted blinded to the CANARY categorization and we found distinct survival differences between the groups. Cluster 1 had a 5-year survival of 100%, Cluster 2 of 72.7% and Cluster 3 of 51.4%. These were labeled as Good, Intermediate and Poor prognosis groups, respectively. We found the post-resection DFS based on the CANARY classification mirrored what would be predicted by the known histopathology. In addition, the CANARY-based prognosis groups had significantly better outcome prediction compared to pathologic Tumor Node Metastasis (TNM) stage (P<0.0001 versus 0.55, respectively) (7).
Validation within the NSLT cohort
The NLST is the largest study to date of structured lung cancer screening with scheduled low dose CT chest (5). To further validate CANARY’s risk stratification we analyzed the 352 screen-detected cases of adenocarcinoma within the NLST cohort. A total of 294 of the subjects were appropriate for CANARY analysis with exclusion criteria of missing data, tumors embedded in the hilum or mediastinum that could not be segmented from the surrounding tissue or multiple nodules confounding the ability to determine cancer-related survival for an individual nodule (8).
Of 294 screen-detected adenocarcinomas, 23 patients experienced recurrence (7.8%) and 63 died related to their lung cancer (21.4%). CANARY analysis was applied to all 294 nodules including the risk model. Nodules were stratified into the three risk categories: good, intermediate and poor. Kaplan Meier survival curves validated the previous risk stratification results, showing three distinct outcome groups (Figure 3) with near 100% survival in the Good group and fully 100% survival in the Good group when analysis was restricted to the 218 stage 1 adenocarcinomas (P<0.001, P=0.008, respectively) (8).
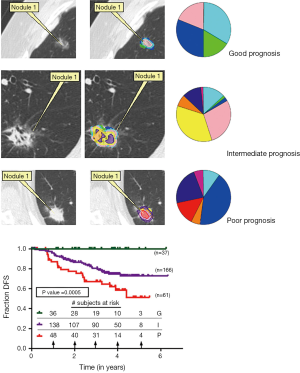
Interobserver agreement
Nodule characterization by CANARY is fully-automated and essentially instantaneous. However, meaningful results from CANARY require that the nodule is captured in its entirety and that non nodule areas such as blood vessels, the hilum and pleural structures as well as adjacent lung are not misclassified as part of the nodule. Prior to CANARY analysis, we utilize a semi-automated segmentation algorithm to generate a mask enveloping the nodule to be analyzed. This mask is generated based upon density thresholds, but since nodule density may vary from subtle ground glass to entirely solid, many automated segmentations based on a simple thresholding will either under or over-estimate the nodule volume. For these reasons, and the complexity of nodules that may be adjacent to or invade anatomy which is not neoplasm, it is essential for the process to be overseen with an expert user manually editing the borders and either adding or removing regions to the volume to be analyzed. Because of the potential for variability in the segmentation process, there is the possibility for error in reproducibility of nodule analysis and thus risk stratification.
To understand the reproducibility and ensure wide applicability of CANARY, three investigators who were not involved in the initial development of CANARY each separately segmented a set of 95 pathologically-determined adenocarcinoma of the lung nodules. In order to explore interobserver agreement, 45 of the nodules were from the Mayo Clinic, Rochester, MN and 50 from Vanderbilt University, Nashville, TN. Interobserver agreement was measured in several ways:
- Segmentation was assessed by dice similarity coefficient (22);
- Intra-class correlation (ICC) was determined for each for each of the nine CANARY exemplars as well as the summed exemplar category V-I-R-O by percent nodule volume (6);
- Agreement among the determination of CANARY-based risk category (good/intermediate/poor) was assessed by Fleiss Kappa coefficient.
Overall interobserver agreement was excellent by all metrics. Dice similarity was 0.79 for the Vanderbilt cohort and 0.81 for the Mayo cohort with a level >0.70 defined as excellent agreement (22). Agreement among exemplar presence was strong with a mean ICC 0.83 (95% CI: 0.76–0.90) for the Vanderbilt cohort and 0.85 (95% CI: 0.80–0.90) for the Mayo cohort. Lastly the Fleiss Kappa score was 0.75 (95% CI: 0.62–0.88) for the Vanderbilt cohort and 0.82 (95% CI: 0.70–0.94) for the Mayo cohort. A Kappa score of 0.61–0.80 signifies significant agreement and 0.81–1.00 signifies perfect agreement. Our group concluded that with adequate training, CANARY can be widely applied with reproducible results (23). Additional internal validation among three independent users (B Bartholmai, R Karwoski, S Rajagopalan) for 283 adenocarcinomas from Mayo historical cases again showed excellent ICC for all exemplars and the summed V-I-R-O category (Table 2). We also analyzed ICC as a function of nodule size for this same validation cohort (n=283) and found that nodule size did not affect ICC for the differing exemplars and the summed V-I-R-O category (Figure 4).
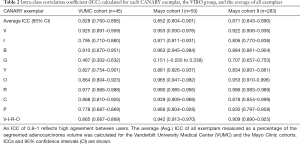
Full table
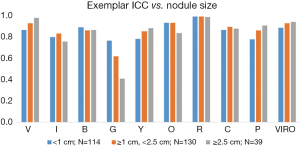
Application of CANARY to other thoracic malignancies
Though both adenocarcinoma of the lung and squamous cell carcinoma are lumped under the umbrella of ‘non-small cell lung cancer (NSCLC),’ due to the role of surgery in early stage disease and similar overall survival (24,25)—they are clinically distinct tumors. Squamous cell carcinoma has a distinctly different molecular profile compared with adenocarcinoma, is typically a more aggressive tumor and has differing radiologic appearance and more rapid VDT (26-29). We initially focused our attention on adenocarcinoma with this tool given the increased prevalence of adenocarcinoma in a screening population and possibility of more nuanced management for indolent adenocarcinoma in which surgical resection may warrant a more individualized approach.
Ultimately CANARY needs prospective study to compare active surveillance with usual care in low-risk nodules in carefully-selected patients. We would only consider nodules stratified into the “good” prognosis for observation. Before undertaking this, however, we needed to prove that were CANARY accidentally fed a nodule not of the adenocarcinoma spectrum—it would not categorize it as good. We want to avoid accidental observation of squamous cell or small cell carcinoma. To test this hypothesis we applied CANARY to screen-detected non-adenocarcinoma cancers within the NLST.
We identified 213 screen-detected non-adenocarcinomas within the NLST—129 of which were able to be analyzed by CANARY (86 squamous cell, 21 large cell, 18 small cell and 4 carcinoid). Only nodules >8 mm were analyzed. Only 2 nodules—both squamous cell carcinoma—were categorized in the “good” prognosis—all others classified as intermediate or poor—demonstrating that CANARY will be unlikely to categorize a non-adenocarcinoma as appropriate for close observation. Of the two cases miscategorized, one was a flat nodule just above the lower size limit cutoff of 8 mm and the other was an 11 mm subsolid opacity in the same location as a subsequent stage 3a squamous cell carcinoma (Figure 5). Due to the structure of the NLST database, we were unable to verify whether this was in fact the subsequent cancer or if it was a nodule within the same location as a cancer, but was the initial reason for this case being in the screen-detected rather than interval cancer arm (30).
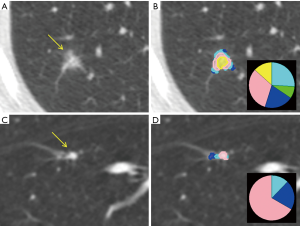
CANARY prognostic categories analyzed by Kaplan Meier survival curves and log rank sum were not statistically significant when applied to the non-adenocarcinoma cohort (P=0.66, P=0.22 for all non-adenocarcinomas and the squamous cell carcinoma subset, respectively) (30). We expected this given that adenocarcinoma represents a well characterized and distinct subset of cancer to which CANARY is calibrated. This informs our caution regarding radiology-based approaches that lump all cancers together.
Independent validation of CANARY
Though CANARY has been externally validated—all studies to date involved at least one of CANARY’s original architects. In the study by Nemec et al., a group with no prior experience with CANARY was able to independently verify its utility in determining histopathology. The prior CANARY studies all had morphologically diverse nodules—whereas Nemec’s group picked a morphologically homogeneous group entirely composed of adenocarcinoma that presented on CT as pure ground glass nodules. Based on radiologic assessment, all 64 tumors analyzed would have been typified as non-invasive (10), however 10 of the 64 were ultimately characterized as IA on histopathology, showing the limitations of visual assessment alone (31).
Notable aspects of this study are:
- 21 (36%) of the studies were contrast enhanced, however linear regression analysis showed no relation between CANARY signature and contrast administration (P=0.331–0.664);
- % B-C-G within the adenocarcinoma negatively correlated with size of the invasive focus (r=−0.406, P=0.005);
- % Y-P within the adenocarcinoma positively correlated with size of the invasive focus (r=0.407, P=0.005);
- % V-I-R-O within the adenocarcinoma positively correlated with the size of the invasive focus (r=0.467, P<0.0001);
- Despite working without an automated segmentation algorithm, CANARY analysis per case was only about 10 minutes (31).
It is promising that with no guidance from our group, CANARY successfully distinguished invasiveness among a radiologically homogeneous group of adenocarcinomas presenting as ground glass nodules. This shows CANARY’s flexibility in application to new clinical questions and opens the door for future groups to work with CANARY to address additional questions in the management of adenocarcinoma of the lung.
Comparison to other quantitative techniques
At present there are multiple techniques coming in to the field of radiomics—a novel area that studies the multitude of raw data involved in a radiologic imaging study to predict different tumor characteristics (32). Hugo Aerts’ group is arguably the most prolific with high quality studies that have demonstrated the possibility of predicting tumor histology (AUC =0.56–0.72) and prognosis (AUC =0.60) (33,34). While the predictive abilities are lower than hoped, the quantitative image features extracted such as tumor heterogeneity, are stable throughout training and validation sets. However, lumping all cancers together may make it difficult to fine-tune a radiologic biomarker—which is one of the strengths in CANARY’s calibration to adenocarcinoma.
Other semi-quantitative tools such as measuring the consolidation/tumor ratio (C/T) offer high specificity but weak sensitivity to determine the invasiveness and thus risk of adenocarcinoma (10). Relying purely on radiologic features diminishes the sensitivity to detect differences in adenocarcinoma. This method reduces the multiple data points available within the quantitative assessment of a tumor (e.g., the nine CANARY exemplars) to a dichotomous variable dependent on the proportion of ground glass present. In that reduction, useful information is lost.
Prediction of driver mutations
Driver mutations such as EGFR, KRAS and ALK determine tumor behavior and ability to treat with targeted chemotherapeutics (35-38). Given the connection between histopathology and tumor behavior, we hypothesized that CANARY exemplars may be able to predict driver mutations as well. We analyzed a subset of our earlier data that had archival tissue and preoperative HRCT within 3 months of adenocarcinoma resection. We applied the AmpliSeq Cancer Hotspot Panel v2 (Thermo Fischer Scientific) to amplify tumor DNA. This panel targets over 2,800 possible somatic mutations within 50 cancer-associated genes (39).
In addition to CANARY analysis we performed quantitative CT analysis of a 10 mm envelope of tumor-free surrounding lung given that tumors likely exert some degree of pathologic change on the adjacent tissue via tumor-mediated cytokines from tumor fibroblasts (21,40). One hundred and eighteen tumors were analyzed by 50 gene panel and CANARY. Of the 118 tumors, 15 harbored EGFR mutations, 47 harbored KRAS mutations and 48 harbored TP53 mutations. KRAS and EGFR mutations were mutually exclusive, but 5 of the 15 EGFR mutations had concurrent TP53 mutations (39).
Increase in the V-I-R-O component of the tumor was correlated with a decreased likelihood of harboring an EGFR mutation with each 10% decrease in percent V-I-R-O present associated with a 23% increase in the odds of being an EGFR mutant (OR 1.23, 95% CI: 1.04–1.46). Y-P was also positively associated with the likelihood of carrying an EGFR mutation (P=0.02 by Wilcoxon rank sum). Each exemplar was examined by stepwise logistical regression and none were found to be predictive of KRAS, nor were quantitative features of the tumor-free surrounding lung. EGFR-containing tumors, however, had less fibrosis in the tumor-free surrounding lung compared with wild type (P=0.007). Both Y and G exemplars were found to be weakly predictive of harboring an EGFR mutation. Finally, a multivariate model of Y and G was predictive of EGFR status with an AUC of 0.77, which was strengthened when considering smoking status to an AUC of 0.85. Our small data set limited our ability to test more than two variables due to the hazard of overfitting the model (39).
Predict response to targeted chemotherapy
In exploratory analysis, CANARY showed promise in predicting EGFR-containing tumors. Theoretically, CANARY along with other radiomic techniques when fully developed and calibrated could be superior to biopsy at predicting response to differing therapeutics. Tumors, including NSCLC, have heterogeneous expression of mutations. Needle biopsies could easily miss a mutation and deliver a false negative result, depending on the region of the tumor sampled. Quantitative CT analysis captures a tumor in its 3-dimentional entirety, and thus can account for that heterogeneity. We are actively studying the role of CANARY to predict response to EGFR-targeted tyrosine kinase inhibitors—and this experimental design can and should be applied to other targeted therapies such as ALK and PD-1 targeted therapies. Additional design elements will be looking at the change in CANARY parametric signature over time to determine which signatures tend to represent a given mutation.
Prospective applications of CANARY
Given CANARY’s robust performance in retrospective use—it needs prospective validation so we can bring it to the forefront to aid in individualized cancer care. Survival after curative resection for stage 1 NSLCLC ranges from 61–77% depending on whether the tumor is >3 cm—the cutoff between stage 1a and stage 1b. Other clinical tools such as more discrete size cutoffs offer more granularity with prognosis (25), but data on adjuvant therapy in stage 1a and 1b still does not show a clear survival benefit for all comers (41). For these reasons the National Comprehensive Cancer network recommends against adjuvant therapy in stage 1a NSCLC and urges an individualized decision in stage 1b NSCLC. In early clinical stage adenocarcinoma, CANARY risk stratification has superior performance when compared to TNM stage (7,8). Given the continued poor outcomes despite curative surgery, CANARY deserves prospective evaluation to more precisely select ‘high risk’ patients for adjuvant therapy despite early stage disease.
CANARY’s other potential prospective role is selecting low-risk early stage tumors that can safely undergo active surveillance. We have seen that CANARY is unlikely to misclassify an aggressive non-adenocarcinoma for observation (30). Lobectomy is a morbid procedure, and if a patient’s life span is not going to be adversely affected by living with a low-risk tumor—similar to how we view many prostate cancers—lobectomy and pneumonectomy clearly offer more harm than benefit (42,43). We can only determine the prospective operating characteristics of CANARY by prospective trial. Figure 6A,B,C demonstrates three different cases of nodule surveillance over time. Case C best represents the ideal nodule selection for prospective active surveillance: a slow-growing nodule followed by serial CT scans for 10 years in the “Good” category. Cases A and B show the change in nodule composition over time in patients who were not ideal surgical candidates due to medical comorbidity. Based on our understanding of indolence and over-diagnosis in adenocarcinoma, this study must be done and can be undertaken safely with proper follow-up interval.
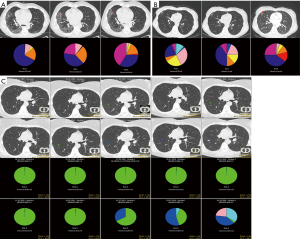
Conclusions
Since its introduction in 2013, CANARY has been shown to reliably provide noninvasive detection of adenocarcinoma histopathology. From this, risk analysis using CANARY has been validated to provide superior post-resection DFS when compared with staging data and CANARY analysis may offer insight into a tumor’s underlying mutational status. The next studies for CANARY are its prospective roles in selecting adenocarcinoma for adjuvant treatment and in selecting indolent adenocarcinomas for structured observation.
Acknowledgements
Funding from the NIH, Mayo Clinic and ACCP facilitated the development and application of CANARY.
Footnote
Conflicts of Interest: CANARY software is currently licensed to Imbio LLC (annual royalties <$5,000). This COI applies to Mayo Clinic and B Bartholmai, F Maldonado, R Karwoski, T Peikert and S Rajagopalan. R Clay has no conflicts of interest to declare.
References
- Patz EF Jr, Pinsky P, Gatsonis C, et al. Overdiagnosis in low-dose computed tomography screening for lung cancer. JAMA Intern Med 2014;174:269-74. [Crossref] [PubMed]
- Boland JM, Froemming AT, Wampfler JA, et al. Adenocarcinoma in situ, minimally invasive adenocarcinoma, and invasive pulmonary adenocarcinoma--analysis of interobserver agreement, survival, radiographic characteristics, and gross pathology in 296 nodules. Hum Pathol 2016;51:41-50. [Crossref] [PubMed]
- Moyer VA, Force USPST. Screening for lung cancer: U.S. Preventive Services Task Force recommendation statement. Ann Intern Med 2014;160:330-8. [PubMed]
- Swensen SJ, Jett JR, Hartman TE, et al. Lung cancer screening with CT: Mayo Clinic experience. Radiology 2003;226:756-61. [Crossref] [PubMed]
- National Lung Screening Trial Research Team, Aberle DR, Adams AM, et al. Reduced lung-cancer mortality with low-dose computed tomographic screening. N Engl J Med 2011;365:395-409. [Crossref] [PubMed]
- Maldonado F, Boland JM, Raghunath S, et al. Noninvasive characterization of the histopathologic features of pulmonary nodules of the lung adenocarcinoma spectrum using computer-aided nodule assessment and risk yield (CANARY)--a pilot study. J Thorac Oncol 2013;8:452-60. [Crossref] [PubMed]
- Raghunath S, Maldonado F, Rajagopalan S, et al. Noninvasive risk stratification of lung adenocarcinoma using quantitative computed tomography. J Thorac Oncol 2014;9:1698-703. [Crossref] [PubMed]
- Maldonado F, Duan F, Raghunath SM, et al. Noninvasive Computed Tomography-based Risk Stratification of Lung Adenocarcinomas in the National Lung Screening Trial. Am J Respir Crit Care Med 2015;192:737-44. [Crossref] [PubMed]
- Kinsinger LS, Anderson C, Kim J, et al. Implementation of Lung Cancer Screening in the Veterans Health Administration. JAMA Intern Med 2017;177:399-406. [Crossref] [PubMed]
- Suzuki K, Koike T, Asakawa T, et al. A prospective radiological study of thin-section computed tomography to predict pathological noninvasiveness in peripheral clinical IA lung cancer (Japan Clinical Oncology Group 0201). J Thorac Oncol 2011;6:751-6. [Crossref] [PubMed]
- Godoy MC, Naidich DP. Subsolid pulmonary nodules and the spectrum of peripheral adenocarcinomas of the lung: recommended interim guidelines for assessment and management. Radiology 2009;253:606-22. [Crossref] [PubMed]
- Travis WD, Brambilla E, Noguchi M, et al. International association for the study of lung cancer/american thoracic society/european respiratory society international multidisciplinary classification of lung adenocarcinoma. J Thorac Oncol 2011;6:244-85. [Crossref] [PubMed]
- Patz EF Jr, Pinsky P, Kramer BS. Estimating overdiagnosis in lung cancer screening--reply. JAMA Intern Med 2014;174:1198-9. [Crossref] [PubMed]
- Veronesi G, Maisonneuve P, Bellomi M, et al. Estimating overdiagnosis in low-dose computed tomography screening for lung cancer: a cohort study. Ann Intern Med 2012;157:776-84. [Crossref] [PubMed]
- Siegel RL, Miller KD, Jemal A. Cancer statistics, 2016. CA Cancer J Clin 2016;66:7-30. [Crossref] [PubMed]
- Thalanayar PM, Altintas N, Weissfeld JL, et al. Indolent, Potentially Inconsequential Lung Cancers in the Pittsburgh Lung Screening Study. Ann Am Thorac Soc 2015;12:1193-6. [PubMed]
- Detterbeck FC, Gibson CJ. Turning gray: the natural history of lung cancer over time. J Thorac Oncol 2008;3:781-92. [Crossref] [PubMed]
- Zhao L, Leung LH, Wang J, et al. Association between Charlson comorbidity index score and outcome in patients with stage IIIB-IV non-small cell lung cancer. BMC Pulm Med 2017;17:112. [Crossref] [PubMed]
- Frey BJ, Dueck D. Clustering by passing messages between data points. Science 2007;315:972-6. [Crossref] [PubMed]
- Bartholmai BJ, Raghunath S, Karwoski RA, et al. Quantitative computed tomography imaging of interstitial lung diseases. J Thorac Imaging 2013;28:298-307. [Crossref] [PubMed]
- Raghunath S, Rajagopalan S, Karwoski RA, et al. Quantitative stratification of diffuse parenchymal lung diseases. PLoS One 2014;9:e93229. [Crossref] [PubMed]
- Zijdenbos AP, Dawant BM, Margolin RA, et al. Morphometric analysis of white matter lesions in MR images: method and validation. IEEE Trans Med Imaging 1994;13:716-24. [Crossref] [PubMed]
- Nakajima EC, Frankland MP, Johnson TF, et al. Assessing the inter-observer variability of Computer-Aided Nodule Assessment and Risk Yield (CANARY) to characterize lung adenocarcinomas. PLoS One 2018;13:e0198118. [Crossref] [PubMed]
- Silvestri GA, Gonzalez AV, Jantz MA, et al. Methods for staging non-small cell lung cancer: Diagnosis and management of lung cancer, 3rd ed: American College of Chest Physicians evidence-based clinical practice guidelines. Chest 2013;143:e211S-50S.
- Ost D, Goldberg J, Rolnitzky L, et al. Survival after surgery in stage IA and IB non-small cell lung cancer. Am J Respir Crit Care Med 2008;177:516-23. [Crossref] [PubMed]
- Rekhtman N, Ang DC, Riely GJ, et al. KRAS mutations are associated with solid growth pattern and tumor-infiltrating leukocytes in lung adenocarcinoma. Mod Pathol 2013;26:1307-19. [Crossref] [PubMed]
- Pinsky PF, Church TR, Izmirlian G, et al. The National Lung Screening Trial: results stratified by demographics, smoking history, and lung cancer histology. Cancer 2013;119:3976-83. [Crossref] [PubMed]
- Mirtcheva RM, Vasquez M, Yankelevitz DF, et al. Bronchioloalveolar carcinoma and adenocarcinoma with bronchioloalveolar features presenting as ground-glass opacities on CT. Clin Imaging 2002;26:95-100. [Crossref] [PubMed]
- Kim TJ, Goo JM, Lee KW, et al. Clinical, pathological and thin-section CT features of persistent multiple ground-glass opacity nodules: comparison with solitary ground-glass opacity nodule. Lung Cancer 2009;64:171-8. [Crossref] [PubMed]
- Clay R, Bartholmai B, Duan F, et al. Canary (computer-Aided Nodule Assessment And Risk Yield) of Non-Adenocarcinoma Pulmonary Nodules in The NLST Cohort. Am J Respir Crit Care Med 2017;195:A4891.
- Nemec U, Heidinger BH, Anderson KR, et al. Software-based risk stratification of pulmonary adenocarcinomas manifesting as pure ground glass nodules on computed tomography. Eur Radiol 2018;28:235-42. [Crossref] [PubMed]
- Aerts HJ, Velazquez ER, Leijenaar RT, et al. Decoding tumour phenotype by noninvasive imaging using a quantitative radiomics approach. Nat Commun 2014;5:4006. [Crossref] [PubMed]
- Wu W, Parmar C, Grossmann P, et al. Exploratory Study to Identify Radiomics Classifiers for Lung Cancer Histology. Front Oncol 2016;6:71. [Crossref] [PubMed]
- Parmar C, Leijenaar RT, Grossmann P, et al. Radiomic feature clusters and prognostic signatures specific for Lung and Head & Neck cancer. Sci Rep 2015;5:11044. [Crossref] [PubMed]
- Shaw AT, Kim DW, Mehra R, et al. Ceritinib in ALK-rearranged non-small-cell lung cancer. N Engl J Med 2014;370:1189-97. [Crossref] [PubMed]
- Lynch TJ, Bell DW, Sordella R, et al. Activating mutations in the epidermal growth factor receptor underlying responsiveness of non-small-cell lung cancer to gefitinib. N Engl J Med 2004;350:2129-39. [Crossref] [PubMed]
- Zhou C, Wu YL, Chen G, et al. Erlotinib versus chemotherapy as first-line treatment for patients with advanced EGFR mutation-positive non-small-cell lung cancer (OPTIMAL, CTONG-0802): a multicentre, open-label, randomised, phase 3 study. Lancet Oncol 2011;12:735-42. [Crossref] [PubMed]
- Massarelli E, Varella-Garcia M, Tang X, et al. KRAS mutation is an important predictor of resistance to therapy with epidermal growth factor receptor tyrosine kinase inhibitors in non-small-cell lung cancer. Clin Cancer Res 2007;13:2890-6. [Crossref] [PubMed]
- Clay R, Kipp BR, Jenkins S, et al. Computer-Aided Nodule Assessment and Risk Yield (CANARY) may facilitate non-invasive prediction of EGFR mutation status in lung adenocarcinomas. Sci Rep 2017;7:17620. [Crossref] [PubMed]
- Bremnes RM, Donnem T, Al-Saad S, et al. The role of tumor stroma in cancer progression and prognosis: emphasis on carcinoma-associated fibroblasts and non-small cell lung cancer. J Thorac Oncol 2011;6:209-17. [Crossref] [PubMed]
- Pignon JP, Tribodet H, Scagliotti GV, et al. Lung adjuvant cisplatin evaluation: a pooled analysis by the LACE Collaborative Group. J Clin Oncol 2008;26:3552-9. [Crossref] [PubMed]
- Berry MF, Hanna J, Tong BC, et al. Risk factors for morbidity after lobectomy for lung cancer in elderly patients. Ann Thorac Surg 2009;88:1093-9. [Crossref] [PubMed]
- Brunelli A, Pompili C, Koller M. Changes in quality of life after pulmonary resection. Thorac Surg Clin 2012;22:471-85. [Crossref] [PubMed]