Selecting lung cancer screenees using risk prediction models—where do we go from here
Introduction
This report provides a description of where we currently are with regard to identifying individuals at high risk for lung cancer and their selection for low dose computed tomography (LDCT) lung cancer screening. Some relevant issues are discussed, and directions on how to move forward in this area are suggested. Although much of this report reviews existing literature, it also includes personal observations made in the lung cancer screening field, and in attempt to address some current issues, fresh analyses have been carried out and are presented.
Older age, and greater number of comorbidities and competing causes of death in screenees are expected to decrease beneficial lung cancer screening outcomes, such as years of life gained, quality-adjusted life years (QALY) gained, and deaths averted. Compared to individuals selected for screening using a validated lung cancer risk prediction model, the PLCOm2012, National Lung Screening Trial (NLST) criteria-selected individuals are younger and have fewer comorbidities and competing causes of death. These observations suggest that screening using NLST criteria may yield better outcomes than using PLCOm2012 risk. However, a sizeable proportion of NLST criteria selected individuals are at low lung cancer risk. We evaluated whether the overall favourable numbers for age, comorbidities and competing causes of death for those meeting NLST criteria are driven by inclusion of low risk individuals who would not benefit from screening.
Methods
Search and review of literature
PubMed, Medline, and the Cochrane Library were searched from 1 January 1980 to 22 April 2018 using combinations of words or terms that included lung cancer risk prediction, risk models, and lung cancer screening. Reference lists from articles were reviewed and relevant articles were identified. Non-English language articles and abstracts were excluded.
Evaluating adverse outcome indicators in individuals selected for screening by NLST and PLCOm2012 risk criteria
Using Prostate Lung Colorectal and Ovarian Cancer Screening Trial (PLCO) smoker data, we carried out contingency table analysis stratifying by NLST versus PLCOm2012 (≥1.5% 6-year risk) eligibility and evaluated number of lung cancers occurring in 6 years, mean age, comorbidity count and competing causes of death in 5 years. The 1.5% risk threshold for selecting lung cancer screenees has been identified to be an appropriate cut-point for this model (1). Comorbidity count was the sum of the following diseases where 1 was assigned if each was present and 0 was assigned if the disease was not reported: heart disease, stroke, history of cancer, hypertension, chronic obstructive pulmonary disease/emphysema and diabetes. This list is not comprehensive, but does include 5 of the 7 leading causes of death in the United States (2). Excluded were death by accident, which is an acute event and cannot be a predictor of subsequent death, and Alzheimer’s disease, which presumably prevented individuals from participating in the PLCO. Statistical significance was not reported for the contingency table analysis, because with the large sample size, small trivial differences were significant. Emphasis was placed on potentially clinically meaningful differences.
Review and results
The NLST demonstrated that LDCT lung cancer screening can reduce lung cancer mortality by 20% in high-risk individuals (3). Critically important to the success of lung cancer screening is application of screening to high-risk individuals. In NLST, high risk was defined by ≥30 pack-years smoked, quit-time in former smokers of ≤15 years, and age 55 to 74 years. As a consequence, many institutions have recommended lung cancer screening of high risk individuals and most of them recommend selecting individuals using the NLST criteria or a variant of it. In the U.S., because they control reimbursement costs of screening for eligible individuals, the United States Preventive Services Task Force (USPSTF) and Centers for Medicare and Medicaid Services (CMS) recommendations are particularly influential (4,5). Their definition of high-risk is identical to the NLST criteria except that they apply to ages 55–80 and 55–77 years, respectively.
NLST criteria includes some low-risk individuals and excludes some high-risk individuals
Application of accurate risk prediction models to NLST participants demonstrates that their lung cancer risk is heterogenous and many individuals are at lung cancer risks so low that they are unlikely to benefit from screening. There was no mortality benefit of screening in NLST participants with low model-estimated risks (1,6). Figure 1 illustrates that individuals in the lowest 30th percentile of PLCOm2012 model risks have no screening benefit. Screening-related harms in the low-risk group exceed benefits, and it is not possible that screening this low risk group who are NLST criteria positive could be cost-effective. In addition, evaluation of NLST and risk model criteria in PLCO smokers shows that the NLST criteria exclude some high-risk individuals. In 74,218 PLCO ever-smokers, 4,929 would be screened with PLCOm2012, but not by NLST-criteria, and 3.2% of them were diagnosed with lung cancers (Table 1). Overall, it has been shown in retrospective and cost-effectiveness analyses that selection of screenees by accurate risk model has statistically significantly higher sensitivity and positive predictive value (PPV) for identifying individual who are diagnosed with lung cancer, and averts more deaths and is more cost-effective (1,7-10).
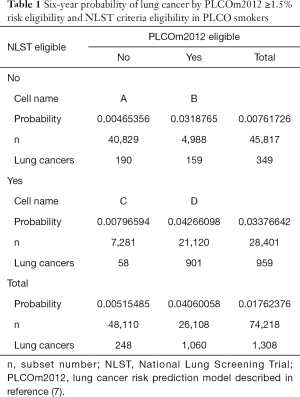
Full table
Which model to choose for screening
In a previous review, the count of lung cancer risk prediction models to 2014 was 18 (11). Since then additional models have been published, and four have come to the attention of the author, taking the count to 22. This list is not all inclusive.
Wilson and Wessfeld published the Pittsburgh Predictor which was designed to be a user-friendly, short, four-factor model (12). Predictors include duration of smoking, smoking status, smoking intensity, and age. The model was trained on NLST data and validated on Pittsburgh Lung Screening Study data, both of which were pre-selected to be high-risk samples and do not represent the general population of smokers. We comment on the predictive performance of this model later.
Katki and colleagues developed and validated the Lung Cancer Risk Assessment Tool (LCRAT) and Lung Cancer Death Risk Assessment Tool (LCDRAT) (8), which replaces their earlier lung cancer death model (6). The incidence model (LCRAT) was validated in PLCO and NLST data and the death model was also validated in National Health Interview Survey data (NHIS; 1997–2001). They found a greater number of lung-cancer deaths were prevented over 5 years along with a lower number needed to screen (NNS) to prevent one lung cancer death. Model predictors include age, education, sex, race, smoking intensity/duration/quit-years, body mass index, family history of lung-cancer, and self-reported emphysema. LCRAT is similar to the PLCOm2012 in that is a regression model that was developed using PLCO control smoker data and includes the same predictors except it adds sex (non-significant) and excludes personal history of cancer and current smoking status. We found that the LCRAT and PLCOm2012 perform similarly when evaluated for lung cancer incidence and mortality in PLCO and NLST control and intervention groups.
Muller and colleagues developed the UK Biobank 2-year lung cancer incidence model which includes as predictors sex, smoking history variables, nicotine addiction, medical history, family history of lung cancer, and lung function (forced expiratory volume in 1 second) (13). The model was reported to have excellent discrimination, however, it included a large number of never-smokers in analysis, which inflates the area under the receiver operator characteristic curve (AUC) considerably over what would be observed in ever-smokers only. The PLCOall2014 model, which is an adaption of the PLCOm2012 that includes never-smokers but does not require biomarker or pulmonary function test data, had an AUC of 0.84 in external validation (1). In contrast, the internally validated UK Biobank model AUC was 0.84. The UK Biobank model was not externally validated. These AUC’s may not be comparable because the test samples differ. External comparative validation is required. Regardless, at this time, it appears that the Biobank model has no strong advantage over other good models which do not require in-person testing.
Markaki and colleagues developed and validated the HUNT Lung Cancer Model, a Cox model based on Norwegian data (9). Model predictors include age, pack-years, smoking intensity, years since smoking cessation, body mass index, daily cough, and hours of daily indoors exposure to smoke. The model AUC demonstrated excellent discrimination in development and validation data. However, individuals >20 years of age were included in the model, and inclusion of younger low-risk individuals is expected to inflate the AUC. Screening of individuals younger than 55 years will need substantial supportive evidence before being accepted in public health practice, as biological reasoning (14) and microsimulation modeling (15,16) does not support screening individuals under the age of 50 or 55 years at this time. The HUNT model includes daily cough as a predictor. This is a complex predictor as it may be due to smoking-related inflammation (“smokers cough”), smoking-related non-lung cancer diseases, or lung cancer. Cancer screening dogma states that asymptomatic individuals should be screened, and symptomatic individuals should be referred to a clinical diagnostic evaluation rather than into a screening program. The inclusion of symptoms in prediction models improves prediction and inflates AUC, as was observed in previous clinical prediction models (17,18). These clinical models were intended to help guide triaging individuals to diagnostic evaluation of lung cancer and not entry into screening programs.
Not all models have similar predictive performance. Ten Haaf and colleagues found that the PLCOm2012, Bach and Two-Stage Clonal Expansion incidence models performed better than other models tested (19). Katki and colleagues showed that the Bach, LCRAT and PLCOm2012 models were superior lung cancer incidence models (20). The PLCOm2012 externally validated well in large German, Australian, and Canadian samples (21-23).
Convincing policymakers to accept screenee selection using model-estimated risk
The USPSTF and CMS do not recommend using model-estimated risk to determine eligibility for lung cancer screening. The National Comprehensive Cancer Network 2018 guidelines are the first to move in this direction and approve selection based on PLCOm2012 estimated risk (24). To date, there is substantial evidence demonstrating superiority of model-based risk for determining eligibility over NLST-based criteria. The evidence includes superior sensitivity and PPV for detecting lung cancer and greater cost-effectiveness. However, this evidence comes primarily from retrospective analyses of existing trial data and microsimulation modeling (1,6-8,10). It is possible that some public health policy decision-makers will be more convinced of the superiority of using model-estimated risk for selection of screenees if evidence comes from prospective studies or from demonstration of success in programmatic implementation of risk-based screening.
Currently, the International Lung Screen Trial (ILST) is prospectively enrolling over 4,000 participants in Canada, Australia and elsewhere, based on being eligible by either PLCOm2012 6-year risk ≥1.5% or USPSTF criteria (25). Rather than using a randomized controlled trial design, the ILST is using a more efficient design in which individuals are matched to themselves. Table 2 describes the sampling design. Individuals in Table 2, cells B, C and D, receive two annual LDCT scans and are followed for 6 years. Individuals who were invited to participate in the study and are negative by both criteria (Table 2, cell A) will not receive LDCT screening, but samples of A will be followed for the occurrence of lung cancer. In comparing the detection of lung cancers and the number enrolled by USPSTF versus PLCOm2012 criteria, both criteria agree on excluding individuals in cell A and including individuals in cell D. The informative data for comparison are in cells B and C. McNemar’s method can be used to compare if the number of participants or lung cancers differs between cells B and C. The NLST and CMS eligibility criteria are nested in the USPSTF criteria, so comparative evaluation of those criteria will also be made. In addition, sensitivity, specificity and PPVs will be compared. Interim results will be presented in 2018 and are expected to help clarify advantages of each enrollment strategy.
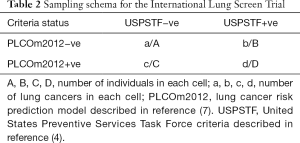
Full table
Cancer Care Ontario (CCO) in Canada has gone one step further. Their Lung Cancer Screening Pilot for People at High Risk (HR_LCSP) is currently enrolling 3,000 individuals in three Ontario centers for annual screening during a 2-year period to evaluate how to optimize implementation of a province-wide lung cancer screening program. Enrollment is based on PLCOm2012 risk ≥2% (24). The decision to use PLCOm2012 risk to determine eligibility came from a multidisciplinary Expert Panel who reviewed the evidence. The HR_LCSP will provide insights regarding practical application of a risk model for selection and should also demonstrate successful identification of individuals who are diagnosed with early stage lung cancer. The final HR_LCSP report is due in 2020, but preliminary findings appear to support use of the PLCOm2012.
Some policy makers are rigid in their thinking about what constitutes “best evidence”. For example, the Canadian Task Force on Preventive Health Care (CTFPHC) in their 2016 Recommendations on Screening for Lung Cancer state: “For adults aged 55–74 years with at least a 30 pack-year smoking history who currently smoke or quit less than 15 years ago, we recommend annual screening with LDCT up to three consecutive times.” (26). This recommendation mimics the NLST protocol exactly, including three consecutive screens. However, risk of lung cancer or benefit from screening does not suddenly dissipate after 2 years or three screens. Randomized control trials (RCT) on effective therapeutic medications for chronic diseases, such as hypertension, last only a few years. Are patients told to stop medications after the few years are up?—of course not! Strict adherence to trial protocols in the belief that it represents “best evidence” needs reappraisal (the CTFPHC is planning to reassess their recommendation in a timely fashion).
Many people believe that the best epidemiological demonstration of a causal relationship comes from RCTs and prospectively collected data. Randomization in the RCT makes the comparison groups roughly equal in the distribution of confounders and minimizes selection bias, thus increasing study validity. In many situations, the RCT study design is superior to alternative epidemiological study designs, but not always. In this paper, there are two examples in which alternative study designs have similar validity to the RCT. The ILST has all participants receive both interventions, so does not depend on the assumption that randomization has made the comparison groups similar. Retrospective analyses of PLCO or NLST data, comparing NLST versus model-based risk selection criteria, have high validity. Data measurement and collection, and sample selection, were made without awareness of the hypothesis of interest. Outcomes occurred after baseline predictor data collection. Consider the data reported in Table 1, cell C, that 58 lung cancers were diagnosed in 7,281 individuals during follow-up (risk =0.008) represents real observations and does identify true low risk in this NLST+ve group. This finding is as valid as the finding that LDCT screening in the NLST led to a 20% lung cancer mortality reduction and should be considered to be strong enough evidence on which to base public health decisions.
Overcoming the belief that risk models select excessive numbers of individuals who are old and sick and die of non-lung cancer causes
The 2018 Screening for Lung Cancer: CHEST Guideline and Expert Panel Report does not recommend using model-estimated risk for selecting screenees. This is because of apprehension that people with high model-estimated risk are more likely to be elderly, in poor health and less likely to benefit from screening compared to those chosen by NLST criteria (27). Screening older individuals is expected to lead to fewer life-years gained than screening younger individuals. Screening individuals with more comorbidity is expected to lead to fewer QALY gained and more competing causes of death than screening individuals with fewer comorbidities. Many individuals who meet NLST criteria are at too low a risk to benefit from screening. We hypothesized that it was the low risk NLST+ve individuals who were weighting the NLST+ve group as a whole to being notably younger and healthier than the PLCOm2012+ve group as a whole. In PLCO smokers, we compared the number of lung cancers, mean age and comorbidity count, and number of competing causes of death in 5 years, stratified by NLST versus PLCOm2012 eligibility status.
Individuals who are NLST+ve but PLCOm2012−ve are at low risk: the cumulative 6-year incidence of lung cancer was 0.8% (Table 1). In contrast, those individuals who are NLST−ve and PLCOm2012+ve have a cumulative 6-year incidence of lung cancer of 3.2%. The number of lung cancers occurring in these groups were 58 and 159 (odds ratio =2.74; 95% CI, 2.02–3.77; P<0.0001). Thus, 7,281 of 28,401 NLST+ve individuals (25.6%) are at low risk and are unlikely to benefit from lung cancer screening.
On average, individuals who were selected for screening by NLST criteria were 61.8 years and those selected by PLCOm2012 criteria were 64.1 years (Table 3). The lower mean age for the NLST+ve group was in large part driven by the young age occurring in those who were at low risk (i.e., were PLCOm2012−ve and had 0.8% lung cancers in 6 years): 58.3 years. Excluding them, the average age for the NLST+ve group at high risk was 63.1 years, only 1 year younger than the mean for all PLCOm2012+ves.
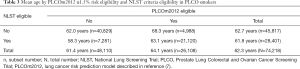
Full table
On average, individuals who were NLST+ve had 0.90 comorbidities and those who were PLCOm2012+ve had 1.02 comorbidities, a difference of 0.12 (Table 4). When low risk individuals, with mean comorbidity count of 0.63, are removed from the NLST+ve group the mean comorbidity count is 0.99, and the mean difference from all PLCOm2012+ves is only 0.03 comorbidities.
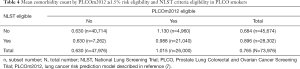
Full table
Of the individuals who were NLST+ve, 5.4% died of competing causes (non-lung cancer) during the 5 years of follow-up, compared to 6.7% of those who were PLCOm2012+ve (Table 5). When low-risk NLST+ve individuals, who have 2.5% competing cause deaths, are excluded, the remaining high-risk NLST-eligible group has 6.5% competing causes deaths, which is not substantially different from the 6.7% observed for all PLCOm2012+ves.
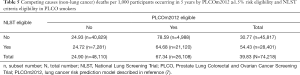
Full table
In summary, the risk of lung cancer in the NLST+ve/PLCO−ve individuals was low (0.8% in 6 years), well below the 1.5%, 1.9% and 2.0% threshold that have been suggested as being appropriate for screening (1,8,28). It was this group that was the youngest, and had the least comorbidities and competing causes of death. The high-risk NLST+ve group had ages, and numbers of comorbidity and competing causes of death that were more similar to those observed in the PLCOm2012+ve group as a whole (Figure 2). These observations suggest two options: screen individuals who are PLCOm2012+ve or alternatively those who are both NLST+ve and PLCOm2012+ve. The latter option would lead to screening fewer individuals who on average would be slightly younger and have slightly fewer comorbidities and competing causes of death. The latter option is recommended to accommodate existing public health guidelines (USPSTF and CMS recommendations), rather than being based on best evidence.
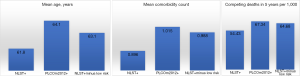
Kumar and colleagues’ cost-effectiveness analysis attempted to demonstrate that the superiority of selection by risk model versus NLST criteria was less when measured by life years saved or QALY then when measured by deaths averted (10). Their analysis accounted for age and comorbidities and, although the difference in life years and QALYs were diminished compared to deaths averted, the superiority of model-based risk assessment remained substantial.
Models that predict competing mortality (non-lung cancer deaths) in the lung cancer screening setting are under development but may only provide limited guidance. Clinical judgment may over-ride model estimated risks and clinicians will likely play an important role in diverting individuals from screening who may not benefit from it.
An additional issue that needs to be considered and dealt with is the ethics of ageism: Should older individuals have less opportunity to receive lung cancer screening because of their age—they have less years of potential life to be saved?
At what risk threshold should we screen?
It is unclear at what threshold of risk screening should be recommended. PLCOm2012 ≥1.5% has been proposed as an appropriate threshold for screening when using this model (1). Other thresholds may be suitable for different models and in different settings. Katki and colleagues found that a 1.9% risk threshold using their LCRAT model led to the same number of individuals being selected for screening in a U.S. population-based sample as did the USPSTF criteria (8). In preparation for the HR_LCSP, Cancer Care Ontario prepared a Health Technology Assessment which included a MISCAN microsimulation modeling-based cost effectiveness analysis (CEA). As part of the CEA, 576 different NLST-like and NELSON-like selection criteria were evaluated (16). Ten models were identified which were on the efficiency frontier, that is, saved the most life-years per a given cost. A preferred model was chosen which was believed to be acceptable to government budgets. The preferred model had an incremental cost effectiveness ratio of just under $50,000 Canadian. The PLCOm2012 model was compared to the MISCAN preferred model and at a ≥2% risk threshold it would lead to the same number of individuals being screened but had significantly higher sensitivity and PPV when evaluated in PLCO control smokers. Thus, the CCO’s HR_LCSP selects individuals for screening using PLCOm2012 ≥2% risk and this approach was considered to be most efficient while being affordable to the health care system.
Different models have been calibrated in different study populations and are expected to have different thresholds from each other while yielding roughly comparable sensitivities and specificities for detecting lung cancer. Also, when applying models to novel populations the optimal risk threshold needs to be re-assessed. The higher the risk threshold for eligibility, the greater the specificity and cost effectiveness. Some jurisdictions have limited budgets for screening and the threshold for a given model can be adjusted to accommodate available resources.
Challenges with implementation of risk models
Some potential users are concerned that complex prediction models are too onerous to be applied in clinical practice and would prefer simpler models with fewer predictors. Some simplified parsimonious models, such as the Pittsburgh Predictor (12), have not performed as well in external validation as more comprehensive models (20). Ten Haaf and colleagues evaluated full and abbreviated versions of several prediction models (19). Although several abbreviated models performed well, they never predicted as well as the full models. In CCO’s HR_LCSP, navigators applied the PLCOm2012 risk calculator over the phone, and they have not reported major concerns.
Avoiding exacerbating race/ethnic lung cancer disparities
In the United States, African Americans have a higher incidence of lung cancer than whites (29), and this disparity remains after adjustment for important predictors, including smoking. NLST-like criteria do not take this disparity into consideration, whereas the PLCOm2012 and LCRAT (8) do incorporate race/ethnicity and potentially avoid adding to health disparities. Whether this phenomenon exists and to what extent in other countries is unclear. Researchers in France and Canada, felt that it did not or that this association was not clearly established, and they requested a version of the PLCOm2012 that was re-parameterized with race/ethnicity removed. This model is available from the author upon request.
In many world regions, indigenous or First Nations men and women have higher lung cancer incidence and mortality compared to non-indigenous people (30,31). But these findings are inconsistent, in particular, indigenous individuals in non-Alaskan United States appear to have lower lung cancer rates than non-indigenous individuals (31). Sarfati and colleagues have described the many difficulties in measuring cancer rates in indigenous populations (32). Whether the reported lower incidence of lung cancer in indigenous people in non-Alaskan United States reported by Moore and colleagues (31) is a valid finding is unclear. The correct PLCOm2012 odds ratio for lung cancer risk for “American Indian or Alaskan Native” compared to whites is 2.79 (95% CI, 0.99–7.86). This finding is inconsistent with the report of Moore and colleagues but was based on small numbers (31). The PLCOm2012 suggests that adjusted for other factors, indigenous individuals are at increased risk and would benefit from risk assessment with an accurate model including an indigenous category, such as is present in the PLCOm2012. Note that the original data provided for preparation of the PLCOm2012 had the labels for “Native Hawaiian or Pacific Islander” and “Indian or Alaskan Native” reversed and they are incorrectly labeled in reference (7). The error was later corrected in Table S1 of reference (1).
It appears that in many jurisdictions, indigenous people have important proportions of individuals who are at high enough risk to benefit from lung cancer screening (31). Anecdotal reports from First Nations community members in Ontario suggest that a sizeable number of them develop lung cancer before the age of 55 years, and they have asked whether lung cancer screening in their community could begin at an earlier age (personal communication). Some analysis suggests that harms from radiation exposure when starting screening before age 50 can exceed the benefits of lung cancer mortality reduction from screening (14). Microsimulation cost-effectiveness analyses generally have not found screening before age 55 years to be as cost-effective as when starting at age 55 years or older (15,16,33). However, none of these analyses focused on high risk populations in which lung cancer occurred frequently at an early age. Further research in indigenous populations is required to accurately estimate lung cancer risks, the age distribution of lung cancers, and cost-effectiveness of lung cancer screening programs starting at an age younger than 55 years. Prediction models need to evaluate inclusion of predictors accounting for indigenous peoples’ risks when these models are intended for use in such populations.
Enhancing lung cancer risk prediction models—inclusion of screening results and biomarker data
Screening results are an important independent predictor of future lung cancer risk (23,34). Incorporation of screening results into risk models can further improve decision making regarding future screening of individuals. Risk prediction models incorporating screening results need to be developed, validated and implemented. The one complicating factor is added complexity. The impact of the screening results from the last screen can be different than from the previous two or three screens. Including three dichotomous screening results leads to eight possible permutations. A version of the PLCOm2012 has been prepared which incorporates the results of three rounds of screening into risk prediction (submitted). Computer computation of such risks is relatively easy. The challenge is the application interface between model and user. Retrieving the relevant data and manipulating it into the risk calculator can be an impediment, and work will have to be done to streamline such processes. Given the large amount of improvement in predictive ability added by incorporation of screening results and the low cost of screening results information once an individual has been in a screening program, this approach to risk prediction is worthwhile and may well outperform addition of biomarkers to prediction models.
When preparing a lung cancer risk model which incorporates screening results, the following thoughts should be borne in mind. Lung cancer risk prediction models predict who is at high risk of developing lung cancer and therefore can help identify who would be suitable candidates for screening. In contrast, pulmonary nodule malignancy probability models, such as the Brock model (35), inform the likelihood that a nodule detected on screening is a cancer. The two types of models should not be confused or mixed because they apply to different populations, have different purposes, and lead to different actions: high risk by the former model would lead to screening, and high risk by the later model would lead to clinical investigation and not continuance in routine lung cancer screening. Thus, it is important that for lung cancer risk prediction modeling, inclusion of screening results is not detecting an existing cancer but is predicting a future cancer that may be detected by future lung cancer screening and not a current diagnostic evaluation.
Improving screenee selection by combining prediction model risk with biomarker data is an area of active research. For example, one research team is using PLCO biorepository specimens to study how information from a panel of 14 blood-based biomarkers can supplement PLCOm2012 estimated risk to improve early lung cancer detection and risk prediction (36). Although some biomarkers will technically be shown to improve predictive discrimination, the decision to incorporate them into widespread public health screening programs will require cost-effectiveness analyses to determine whether the added time and cost required to obtain and analyze biospecimens is justified. It is possible that validated biomarkers may be cost-effective in novel two-stage decision making protocols, for example applying a risk prediction model with a sensitive threshold, followed by applying a specific biomarker to reduce false-positives. In addition, proven biomarkers may be of particular utility in special populations.
Conclusions
Lung cancer screening science is in its infancy but is rapidly evolving. One important aspect of it concerns determination of high risk and selection of individuals for screening. It is generally accepted that good risk prediction models are better at identifying high-risk individuals than NLST-like criteria. A plethora of risk prediction models have become available, and some models have been shown to predict better than others in comparative studies. The concern of some policy makers that risk prediction models excessively select older individuals who have more comorbidities and competing causes of death should not deter use of good prediction models for selecting screenees. We have shown that a sizeable proportion of those selected by NLST criteria who are younger, have fewer comorbidities and competing causes of death are at too low a risk to benefit from screening. Those remaining who are NLST criteria positive and are at high risk have similar ages, number of comorbidities and competing causes of death as those selected by the PLCOm2012 model. Unlike the NLST or NLST-like criteria, good comprehensive models can take African American and indigenous peoples’ elevated lung cancer risk into account and avoid exacerbating race/ethnic disparities. Evidence is accumulating that may help guide health policy decision makers in the direction of recommending use of good lung cancer risk prediction models for determining risk and who should be offered lung cancer screening. It is anticipated that advances in risk prediction models in the next few years, in particular by inclusion of screening results and biomarker data, will improve identification of individuals who may benefit from lung cancer screening.
Acknowledgements
None.
Footnote
Conflicts of Interest: The author has no conflicts of interest to declare.
References
- Tammemägi MC, Church T, Hocking W, et al. Evaluation of the Lung Cancer Risks at which to Screen Ever- and Never-Smokers: Screening Rules Applied to the PLCO and NLST Cohorts. PLoS Med 2014;11:e1001764. [Crossref] [PubMed]
- Murphy SL, Xu J, Kochanek KD, et al. Deaths: Final Data for 2015. Natl Vital Stat Rep 2017;66:1-75. [PubMed]
- Aberle DR, Adams AM, Berg CD, et al. Reduced lung-cancer mortality with low-dose computed tomographic screening. N Engl J Med 2011;365:395-409. [Crossref] [PubMed]
- Moyer VA. U. S. Preventive Services Task Force. Screening for lung cancer: U.S. Preventive Services Task Force recommendation statement. Ann Intern Med 2014;160:330-8. [PubMed]
- Centers for Medicare & Medicaid Services (CMS). Decision Memo for Screening for Lung Cancer with Low Dose Computed Tomography (LDCT) (CAG-00439N). Available online: https://www.cms.gov/medicare-coverage-database/details/nca-decision-memo.aspx?NCAId=274&NcaName=Screening+for+Lung+Cancer+with+Low+Dose+Computed+Tomography+(LDCT)&MEDCACId=68&IsPopup=y&bc=AAAAAAAAAgAAAA%3d%3d&. Accessed 31 August 2015.
- Kovalchik SA, Tammemagi M, Berg CD, et al. Targeting of low-dose CT screening according to the risk of lung-cancer death. N Engl J Med 2013;369:245-54. [Crossref] [PubMed]
- Tammemagi MC, Katki HA, Hocking WG, et al. Selection criteria for lung-cancer screening. N Engl J Med 2013;368:728-36. [Crossref] [PubMed]
- Katki HA, Kovalchik SA, Berg CD, et al. Development and Validation of Risk Models to Select Ever-Smokers for CT Lung Cancer Screening. JAMA 2016;315:2300-11. [Crossref] [PubMed]
- Markaki M, Tsamardinos I, Langhammer A, et al. A Validated Clinical Risk Prediction Model for Lung Cancer in Smokers of All Ages and Exposure Types: A HUNT Study. EBioMedicine 2018;31:36-46. [Crossref] [PubMed]
- Kumar V, Cohen JT, van Klaveren D, et al. Risk-Targeted Lung Cancer Screening: A Cost-Effectiveness Analysis. Ann Intern Med 2018;168:161-9. [Crossref] [PubMed]
- Tammemägi MC. Application of risk prediction models to lung cancer screening: a review. J Thorac Imaging 2015;30:88-100. [Crossref] [PubMed]
- Wilson DO, Weissfeld J. A simple model for predicting lung cancer occurrence in a lung cancer screening program: The Pittsburgh Predictor. Lung Cancer 2015;89:31-7. [Crossref] [PubMed]
- Muller DC, Johansson M, Brennan P. Lung Cancer Risk Prediction Model Incorporating Lung Function: Development and Validation in the UK Biobank Prospective Cohort Study. J Clin Oncol 2017;35:861-9. [Crossref] [PubMed]
- Berrington de González A, Kim KP, Berg CD. Low-dose lung computed tomography screening before age 55: estimates of the mortality reduction required to outweigh the radiation-induced cancer risk. J Med Screen 2008;15:153-8. [Crossref] [PubMed]
- de Koning HJ, Meza R, Plevritis SK, et al. Benefits and harms of computed tomography lung cancer screening strategies: a comparative modeling study for the U.S. Preventive Services Task Force. Ann Intern Med 2014;160:311-20. [Crossref] [PubMed]
- Ten Haaf K, Tammemägi MC, Bondy SJ, et al. Performance and Cost-Effectiveness of Computed Tomography Lung Cancer Screening Scenarios in a Population-Based Setting: A Microsimulation Modeling Analysis in Ontario, Canada. PLoS Med 2017;14:e1002225. [Crossref] [PubMed]
- Hippisley-Cox J, Coupland C. Identifying patients with suspected lung cancer in primary care: derivation and validation of an algorithm. Br J Gen Pract 2011;61:e715-23. [Crossref] [PubMed]
- Iyen-Omofoman B, Tata LJ, Baldwin DR, et al. Using socio-demographic and early clinical features in general practice to identify people with lung cancer earlier. Thorax 2013;68:451-9. [Crossref] [PubMed]
- Ten Haaf K, Jeon J, Tammemagi MC, et al. Risk prediction models for selection of lung cancer screening candidates: A retrospective validation study. PLoS Med 2017;14:e1002277. [Crossref] [PubMed]
- Katki HA, Kovalchik SA, Petito SC, et al. Implications of nine risk prediction models for selecting ever-smokers for computed tomography lung-cancer screening. Ann Intern Med 2018. [Epub ahead of print]. [Crossref] [PubMed]
- Li K, Husing A, Sookthai D, et al. Selecting High-Risk Individuals for Lung Cancer Screening: A Prospective Evaluation of Existing Risk Models and Eligibility Criteria in the German EPIC Cohort. Cancer Prev Res (Phila) 2015;8:777-85. [Crossref] [PubMed]
- Weber M, Yap S, Goldsbury D, et al. Identifying high risk individuals for targeted lung cancer screening: Independent validation of the PLCOm2012 risk prediction tool. Int J Cancer 2017;141:242-53. [Crossref] [PubMed]
- Tammemagi MC, Schmidt H, Martel S, et al. Participant selection for lung cancer screening by risk modelling (the Pan-Canadian Early Detection of Lung Cancer [PanCan] study): a single-arm, prospective study. Lancet Oncol 2017;18:1523-31. [Crossref] [PubMed]
- National Comprehensive Cancer Network 2017. Available online: [Access date: 6 October 2017].www.nccn.org
- International Lung Screen Trial. Available online: (accessed on 04 April 2018).https://clinicaltrials.gov/ct2/show/NCT02871856
- Lewin G, Morissette K, Dickinson J, et al. Recommendations on screening for lung cancer. CMAJ 2016;188:425-32. [Crossref] [PubMed]
- Mazzone PJ, Silvestri GA, Patel S, et al. Screening for Lung Cancer: CHEST Guideline and Expert Panel Report. Chest 2018;153:954-85. [Crossref] [PubMed]
- Tammemagi M, Miller B, Yurcan M, et al. Determination of the Eligibility Criteria for Cancer Care Ontario’s Lung Cancer Screening Pilot for People at High Risk (Abstract #9432). American Thoracic Society International Conference. San Diego, CA; 21 May 2018.
- Centers for Disease Control and Prevention (CDC). Racial/Ethnic disparities and geographic differences in lung cancer incidence --- 38 States and the District of Columbia, 1998-2006. MMWR Morb Mortal Wkly Rep 2010;59:1434-8. [PubMed]
- Chiefs of Ontario, Cancer Care Ontario, Institute for Clinical Evaluative Sciences. Cancer in First Nations People in Ontario: Incidence, Mortality, Survival and Prevalence. Toronto, 2017.
- Moore SP, Antoni S, Colquhoun A, et al. Cancer incidence in indigenous people in Australia, New Zealand, Canada, and the USA: a comparative population-based study. Lancet Oncol 2015;16:1483-92. [Crossref] [PubMed]
- Sarfati D, Garvey G, Robson B, et al. Measuring cancer in indigenous populations. Ann Epidemiol 2018;28:335-42. [Crossref] [PubMed]
- Meza R, Ten Haaf K, Kong CY, et al. Comparative analysis of 5 lung cancer natural history and screening models that reproduce outcomes of the NLST and PLCO trials. Cancer 2014;120:1713-24. [Crossref] [PubMed]
- Patz EF Jr, Greco E, Gatsonis C, et al. Lung cancer incidence and mortality in National Lung Screening Trial participants who underwent low-dose CT prevalence screening: a retrospective cohort analysis of a randomised, multicentre, diagnostic screening trial. Lancet Oncol 2016;17:590-9. [Crossref] [PubMed]
- McWilliams A, Tammemagi MC, Mayo JR, et al. Probability of cancer in pulmonary nodules detected on first screening CT. N Engl J Med 2013;369:910-9. [Crossref] [PubMed]
- Feng Z, Hanash SM, Tammemagi MC. Incorporating Biomarkers to Improve Lung Cancer Risk Prediction. Available online: (accessed on 24 April, 2018).http://grantome.com/grant/NIH/U01-CA194733-02