A quantitative method for assessing smoke associated molecular damage in lung cancers
Introduction
Tobacco exposure is the number one risk factor for lung cancer. In the United States, cigarette smoking is linked to about 80% to 90% of lung cancers. However, the use of other tobacco products such as cigars or pipes also increases the risk for lung cancer. Tobacco smoke is a toxic mix of more than 7,000 chemicals, many of which are poisons, and more than 70 are known to be carcinogenic (CDC.gov). Cigarette smokers are 15–30 times more likely to get lung cancer or die from lung cancer than lifetime never smokers.
Former smokers have a lower risk of lung cancer than if they had continued to smoke, but their risk is higher than the risk for people who never smoked. Smoking cessation at any age lowers the risk of lung cancer. Cigarette smoking can cause cancer at multiple other sites in the body (1). Exposure to secondhand smoke also causes lung cancer, although the relative risk is much lower than that of a heavy smoker (2,3).
As definitions for persons with smoke exposure are not uniform, standardized approaches to estimation and reporting are essential to ensure comparability of results in different studies and sources.
We shall use the following definitions for tobacco usage and exposure:
- Current smoker. An adult who has smoked at least 100 cigarettes in their lifetime and who currently smokes cigarettes or has quit within the previous 12 months.
- Former (“reformed”) smoker. An adult who has smoked at least 100 cigarettes in their lifetime but has quit smoking for longer than the previous 12 months.
- Never smoker. An adult who has never smoked, or has smoked less than 100 cigarettes in their lifetime.
- Ever smoker. An adult who has smoked at least 100 cigarettes in their lifetime (irrespective of whether they are currently smoking).
- Environmental tobacco smoke (ETS). Usually refers to cigarette smoke in the environment of a nonsmoker. ETS is also called second-hand smoke. Inhaling ETS is called passive smoking.
- Pack year measure of tobacco exposure. Calculated by multiplying the number of packs of cigarettes smoked per day by the number of years the person has smoked. For example, 1 pack-year is equal to smoking 20 cigarettes (1 pack) per day for 1 year, and 1/2 pack year is equal to smoking 10 cigarettes per day for 1 year.
Because quitting is relatively easy to initiate but difficult to maintain, many smokers repeatedly attempt to quit, usually for short or moderate periods (<1 year). Thus, a former smoker is defined as one who has quit for at least one year. As the risk of lung cancer decreases with the duration of quitting (4), former smokers may also be subdivided into those that quit (reformed) less than 15 years and those that quit (reformed) for longer than 15 years. However, for heavy former smokers, an increased risk of developing lung cancer remains for their lifetime, and never returns to the baseline levels of a lifetime never smoker (5).
Measurement of tobacco exposure is important for tobacco prevention and control monitoring systems (6) and for determining the risk of various tobacco products. Pack years of exposure is the most widely used quantitative measure of overall tobacco use. However, the use of this subjective parameter has major limitations: (I) as daily tobacco usage often varies during the lifetime of a smoker, often interspersed with repeated attempts at quitting for varying times, accurate estimates of smoke exposure over the smoker’s lifetime are difficult; (II) ETS exposure, which often occurs during childhood, is even more difficult to estimate and likely to be a rough approximation; (III) obtaining the most accurate smoke exposure history often requires a trained health care professional and a carefully crafted questionnaire; (IV) an individual’s genetic susceptibility may vary with a number of factors including family history, gender and ethnicity, resulting in different degrees of smoke induced damage from similar exposures; (V) the risk from usage of different tobacco products (such as cigars, pipes, beedis etc.) may vary, making estimates of exposure and their possible harmful effects difficult; (VI) finally, a lung cancer patient may deliberately misstate his or her tobacco exposure for a number of reasons.
Smoke exposure results in characteristic genetic changes in the resultant lung cancers. These findings are consistent with the proposition that tobacco exposure results in cancer risk by increasing the somatic mutation load, both in number and type (1). The tumor types with the highest odds ratio for developing cancer in individuals who smoke an average of more than 30 cigarettes a day are those that occur in tissues directly exposed to tobacco smoke (such as lung cancer and larynx). In fact, it is estimated that smoking a pack of cigarettes a day causes 150 mutations in each lung cell every year (1). These mutations represent the start of a cascade of genetic damage that can eventually cause cancer. However, the molecular changes associated with smoking in other tissues not directly exposed to tobacco smoke is more complex and variable.
One of these molecular changes is an increased number of somatic mutations (of all types) (7). The three common types of lung cancer [lung adenocarcinoma (LUAD), lung squamous cell (LUSC), and small cell lung cancer (SCLC)] are among the five cancer types having the highest tumor mutation burden (TMB). The average TMB is more than several fold lower in never-smokers than in smokers (8,9).
The other major molecular difference between cancers arising in ever and never smokers involves the pattern of DNA substitution mutations. DNA substitution mutations are of two types: transitions are interchanges between two-ring purines (A or G) or of one-ring pyrimidines (C or T)—they therefore involve bases of similar shape. Transversions are interchanges of purine for pyrimidine bases, which therefore involve exchange between one-ring and two-ring structures. Although there are twice as many possible transversions (8 transversions, 4 transitions), the molecular mechanisms by which they are generated result in transition mutations usually occurring at higher frequencies than transversions (“transition bias”) (10). In addition, transitions are less likely to result in amino acid substitutions, and are more likely to cause silent single nucleotide polymorphisms (SNPs).
In smoker associated cancers, C to A/G to T transversions are the commonest form of DNA substitution mutations (8,9,11). This specific transversion is relatively rare in most tumor types, and has been attributed to the mutagenic action of carcinogens in tobacco smoke, in particular to polycyclic aromatic hydrocarbons (11). By contrast, the commonest DNA substitution mutation present in never smoker lung cancers is C to T/G to A transitions (8,9).
In this report, we have combined the two DNA mutation changes that distinguish smoker and never smoker associated cancers to generate and validate a quantitative score for assessing smoke associated damage in lung cancers.
Methods
Datasets of the three major lung cancer types (LUAD, LUSC and SCLC) were used in our study, and an additional one for bronchial carcinoids.
LUAD datasets
Two independent datasets of LUAD were downloaded for training and validation. Genomic Data Commons (GDC) data was downloaded through the public GDC Data Portal (https://docs.gdc.cancer.gov/Data/) [which utilizes data from The Cancer Genome Atlas (https://cancergenome.nih.gov)]. The somatic variants of the whole exome sequencing (WXS) data were measured with MuTect variant calling pipeline. Data from 567 samples were available. More details are available from the GDC website.
The other LUAD dataset was the Broad dataset that was downloaded from a published report (9). The mutation variations of its 183 LUAD samples were examined with a combination of WXS or whole genome sequencing (WGS): 159 WXS, 23 WXS and WGS, and 1 WGS only. Because in this study, we focused on the WXS mutations, we used the 159 WXS samples as the validation data.
LUSC dataset
A GDC LUSC dataset with 492 available samples was also used as another validation dataset. It also included the WES somatic variants measured with MuTect variant calling pipeline.
SCLC datasets
Two independent SCLC datasets were downloaded from published reports and were also used as validation datasets. SCLC I consisted of 30 WXS of primary tumors, published by Rudin et al. (12) and SCLC II contained WXS of 27 tumors, downloaded from Peifer et al. (13).
Bronchial carcinoid dataset
WXS of 13 carcinoid samples in Fernandez-Cuesta were downloaded for this report (14). The somatic mutations were detected by their in-house method.
Trend analysis among different groups
Mann-Kendall (MK) test was used to statistically assess if there was a monotonic upward or downward trend of the tumor mutation burden (TMB) and the transversion/transition ratio (TTR) (see below) for different smoking history groups (15,16).
Classification analysis
To test whether TTR and TMB can be used to quantify the smoking exposure, we used them as variables to classify samples into ever/never smoker groups with maximum entropy discriminant (MED) classification algorithm (17,18).
The GDC samples were separated into four smoking exposure groups: never, reformed longer than 15 years, reformed less than 15 years and current. However, pack year exposure history was available for both LUAD data sets. Therefore, we also separated samples into three groups according to their pack year information:
- Heavy smoker: pack year ≥60;
- Light-moderate smoker: 0< pack year <60;
- Never smoker: pack year =0.
We realize that these are not standard definitions for exposure, but reflect useful categories for our analyses.
GDC LUAD contained the most samples. Therefore, it was used as the training set. The Broad LUAD, GDC LUSC and the two SCLC datasets were used for validation data sets. The details of these sets are shown in Table S1. For additional indirect validation, we compared the F scores from these samples with the presence of KRAS or EGFR mutations. The F scores of bronchial carcinoids were also calculated.

Full table
where xi is the nornalized log2 (TMB) of sample i and yi is the normalized TTR of sample i.
All samples were normalized by the mean value [mean (log2TMB) =7.1848, mean (TTR) =1.0255] and standard deviation [STD (log2TMB) =1.8573, STD (TTR) =0.9077] of the training samples. To improve the classification accuracy, the training model used only heavy smokers as positive samples and never smokers as negative samples. The prediction performance of the lung cancer data sets was used for optimizing the final classification model. All analyses were performed in MATLAB and the scripts are available upon request.
Methods for quantitation of molecular smoke damage in lung cancers
- TMB. The TMBs were considerably lower (>6 fold) in never smokers than in current smokers, suggesting that the TMB could be used as an indicator of smoke damage in lung cancers. We used the mean value of never smokers plus two standard deviations as a cut off value to separate the two groups.
- TTR. Based on the different patterns of DNA substitution mutations observed in lung cancers arising in smokers and never smokers, we reasoned that the ratio of C to A/G to T transversions: C to T/G to A transitions would be a numerical indicator of smoke exposure. We refer to this as the TTR. Values greater than one would reflect smoke associated mutational damage, while values less than one would indicate little or no smoke associated damage.
- The F score as determined by maximum entropy (MaxEnt). This method is described in detail below.
The MaxEnt classification method
In statistics, multinomial logistic regression also known as MaxEnt classifier is a classification method that generalizes logistic regression to multiclass problems. It is a model that is used to predict the probabilities of the different possible outcomes of a categorically distributed dependent variable, given a set of independent variables. We implemented a MATLAB code of a discriminative MaxEnt classifier (17-19).
Performance measurements
To evaluate the classification performance of the classification of the analysis, prediction accuracy (ACC), specificity (SP) and sensitivity (SN) are defined as follows:
where TP, FP, TN and FN denote true positive, false positive, true negative, and false negative, respectively. For example, sensitivity is the proportion of heavy smokers correctly classified, specificity is the proportion of never smokers correctly classified, and accuracy is the proportion of both.
Results
The data sets and gender and smoke exposure demographics
Of the two adenocarcinoma data sets studied, the GDC data from The Cancer Genome Atlas (TCGA) portal contained the more detailed smoking history and separated the samples into four smoke exposure categories. Thus a greater number of our analyses utilized this set. Of the 478 cases with gender and smoking history available, 54% were women (Table S1). However, as expected, there were more women among the never smoker category (72.5%), while the gender distribution among the ever smokers was almost equal (50.9% women) (Figure S1).
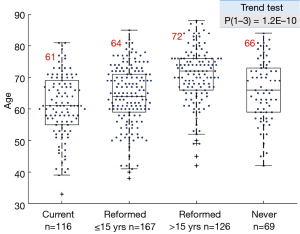
The smoke exposure subgroups also showed a highly significant downward trend in pack year history, with a sharp drop between the reformed ≤15 years and reformed >15 years’ subgroups (Figure S2).
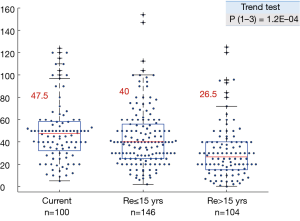
Establishment and validation of the F score method
Since the TTR and TMB were strongly associated with tobacco exposure, we decided to use them as combined variables to measure smoking damage. MaxEnt was used as a classifier. The classification results are shown in Figure 1. The accuracies of training and validation data were 86.3% and 89.8%, respectively.
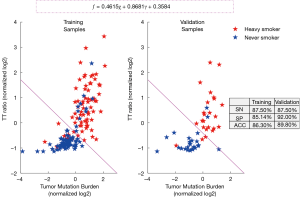
In the training step, we first grouped the lung cancer cases into ever or never smoker groups and developed a F (fi) score {shown in Eq. [1]} as a quantitative indicator of smoking associated molecular damage in lung cancers (Figures 2,S1). F score values >0 were considered to show evidence of tobacco related molecular damage, while values ≤0 were considered to lack evidence of tobacco related molecular damage. When compared to the smoking histories, F scores had sensitivity, specificity and accuracy values in in the 85–87% range.
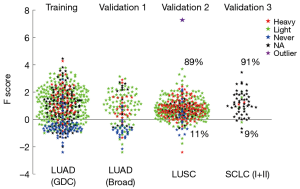
Results of quantitation methods for detection of molecular smoke damage in lung cancers
Results for the three quantitation methods are shown in Table 1. For both the TMB and TTR methods, a surprisingly high percentage of current smokers were found to lack evidence of smoke exposure. For the TMB method, 29.2% of LUAD, 31.6% of LUSC and 64.9% of SCLC tumors arising in current smokers lacked evidence of smoke damage. For the TTR method, 24.2% of LUAD, 40.6% of LUSC and 21% of SCLC tumors arising in current smokers lacked evidence of smoke damage. By contrast, for the F score, method 10.8% of LUAD, 6% of LUSC and 9.8% of SCLC tumors in current smokers lacked evidence of smoke damage. Thus, the data from the F score method were more consistent with the smoke exposure histories and with the knowledge that the vast majority of LUSC and SCLC cases are smoke exposure related (20,21).
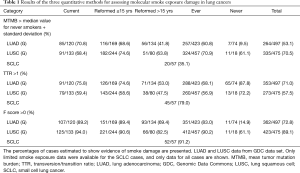
Full table
F scores for the four smoke exposure subgroups are shown in Figure 3. While the mean values for current and recently reformed (≤15 years) subgroups were identical (1.34), there was a sharp drop in value for the longer term reformed (>15 years) smokers (0.53) and the never smokers had a negative value (−0.60). The Trend Test was highly significant. The tobacco pack year exposure decreased with length of smoking cessation. However, for all smoking subtypes, the pack year exposure varied widely. There was no direct correlation between smoke exposure and the F score (data not shown).
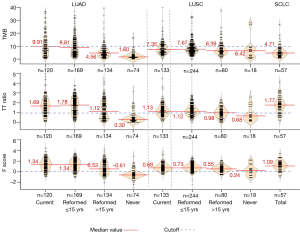
Women constituted more than 50% of the learning data set, and more than 70% of the never smoker subgroup (Table S1). Also, there was a highly significant increasing mean age of the reformed smokers’ subgroups compared to current smokers, accompanied by a decreasing pack year exposure (Figures S1,S2).
Bronchial carcinoids are relatively rare low grade (NETs) of the lung that are not associated with smoking and have a pathogenesis distinct from the common types of lung cancer (14,22). We calculated the F scores of 42 bronchial carcinoids (Figure 4). Of these, 37 (88%) had negative scores, including all 10 with known smoking histories (8 ever smokers, 2 never smokers).
For further indirect validation, we examined the F scores for LUADs having EGFR or KRAS mutations, pooling data from both LUAD data sets (Figure 5). Approximately one third of the EGFR and 90% of the KRAS mutations had F scores >0. Most KRAS mutations, which target ever smokers (23,24), occurred in ever smokers. By contrast, EGFR mutations, which target never smokers and reformed smokers >15 years (24), were more frequent in never smokers and in tumors with F scores <0.
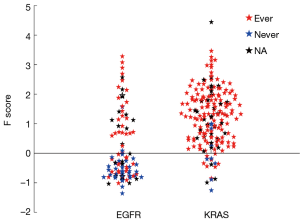

Full table
We attempted to correlate the F score with clinical parameters. The only clinical parameter presents in the two adenocarcinoma data bases we utilized was overall survival. There was no correlation between the F score and overall survival.
Discussion
The molecular changes between lung cancers arising in smokers and never smokers are reflected in differences in the somatic mutation load and in the type of mutations. LUADs have been divided into transversion high and transversion low subgroups as an indicator of smoke damage (25). However, this proposed division is a binary division and not a numerical one, and ignores the other major molecular change, the somatic mutation load, which shows multifold differences between tumors arising in smokers and never smokers. We also altered the Transversion high and Low groups into a ratio comparing the transversion characteristic of smoker tumors and the transition characteristic of never smoker tumors to form the TTR. By combining both molecular changes that differentiate smoker and never smoker tumors, we generated and validated a F score where values >0 were predicted to show evidence of tobacco related molecular damage, while values ≤0 were predicted to lack such evidence. We compared the three numerical scoring systems (TMB, TTR and the F score). Both the TMB and TTR had large fractions of current smoker tumors that lacked evidence of molecular damage, and were not considered as useful. By contrast the F score system had a much smaller fraction of ever smoker tumors without lack of smoke associated damage, comparable to the expected figures.
While most lung cancers are smoking related, about 15% of all lung cancers in the USA arise in lifetime never smokers (20,26). Lung cancers arising in smokers and never smokers appear to be very different diseases at the clinical, epidemiologic and molecular levels (20,27,28). In the clear majority of cases, lung cancers in never smokers have adenocarcinoma or large cell carcinoma histology (20,26). As large cell carcinoma is no longer considered to be an independent entity but may represent poorly or undifferentiated forms of the other major forms of lung cancer (29,30), it may be presumed that LUAD is the major form of lung cancer associated with never smokers. Consistent with these observations, we found that the majority (~90%) of LUSC and SCLC had positive F scores, predicting smoke associated molecular damage, while LUADs demonstrated a much higher frequency of negative F scores. The latter observation reflects the fact that most lung cancers arising in never smokers have adenocarcinoma histology (20). Thus, the use of these scores to assess tobacco associated mutation damage may have limited utility in LUSC and SCLC. However, the F scores may be more useful in LUADs, which show wide ranges of tobacco associated mutational damage, varying with length of smoking cessation, and compromising most cancers arising in never smokers. A fraction of ever smokers in all lung cancer histologies showed negative scores, indicating lack of evidence for smoke induced molecular damage. The mean pack years of exposure varied in the three ever smoker adenocarcinoma groups from 26.5 to 47.5, and it is highly unlikely that modest amounts of smoke exposure contribute to lung cancer pathogenesis. The definition of an ever smoker is a person who has smoked 100 or more cigarettes during his or her lifetime, a minute fraction of the exposure required to induce lung cancers. Approximately 15% of current smokers had exposures less than 20 pack years, and their exposure may have had modest or even no contribution to cancer pathogenesis. However, we were unable to demonstrate any direct relationship between pack year exposure and the F score.
Because ETS is a weak carcinogen, we predicted that lung cancers arising in lifetime never smokers would have very different molecular changes than those arising in ever smokers, irrespective of the extent of ETS exposure, and that long term former (reformed) smokers would have changes intermediate between those of current and never smokers. As described in the Results, our predictions have largely been confirmed. While no estimates of ETS exposure were available for the patient cohorts we studied, the F scores of most never smokers were ≤0, indicating little or no molecular damage that could be attributed to tobacco related mutagens in ETS. However, nearly 15% of never smokers had positive F scores, possibly indicating ETS, or incorrect smoking histories. While ETS is a major health hazard, it is a weak carcinogen for lung cancer compared to the direct exposure of smokers (2,20,31). Of interest, a recent study found no effects of ETS on the molecular profile of lung cancers in never smokers (2). Thus, the F score may also be useful for assessing the contribution of ETS in individual LUADs.
Two of the major driver mutations frequently present in LUADs are KRAS and EGFR mutations. In the largest series of cases reported, Riely et al. (23) found that KRAS mutations in LUADs occurred at a frequency of 25% in smokers and at a frequency of 15% in never smokers. Of interest, the mutations in smokers and never smokers showed the same patterns of transversions and transitions that are characteristic these two groups. By contrast, EGFR mutations in NSCLC are more common in never smokers. In a large meta-analysis study, Ren et al. found that EGFR mutations in NSCLC were approximately 5 times more common in never smokers than in smokers (32). Our findings are consistent with these reports. EGFR mutations frequently occurred in LUADs having scores <0 (68%), and almost all (92%) of the mutations occurring in LUADs with scores >0 were in smokers. By contrast, 89% of the KRAS mutations occurred in LUADs having F scores >0, and the clear majority of mutations occurred in ever smokers (94%).
Bronchial carcinoids are low grade NETs of the lung which are not associated with smoking, and which arise independently of the high grade NETs [SCLC and large cell neuroendocrine carcinoma (LCNEC)] (22). Consistent with this, the F score indicated that most of the carcinoid tumors examined had F scores <0 and, thus, demonstrated no evidence of smoking associated molecular damage.
As most LUSC and SCLC tumors have positive F scores indicating that they have evidence of tobacco associated molecular damage, the greatest value of the F score system appears to be in assessing molecular damage in LUADs. Some LUADs arising in never smokers had F scores >0, suggesting that they had some degree of smoking associated molecular damage. Whether this damage was due to incorrect smoking histories or reflected ETS induced damage remains to be determined. In addition, some smokers lacked evidence of smoking associated molecular damage in their tumors, indicating that smoking was unlikely to be the major causative factor. A previous study found varying frequencies of misclassification of smoking histories, with up to 15% being misclassified (33), consistent with our findings.
The F score was not correlated with overall patient survival. Apparently, tobacco damage is related to tumor pathogenesis, but once a tumor arises, the degree of smoke damage may not contribute to its further clinical or biological progression.
Of interest, women constituted more than 50% of the learning data set, and more than 70% of the never smoker subgroup. This is consistent with multiple observations that the fraction of never smokers among women is relatively high, more so among East Asians. Another observation was the highly significant increasing mean age of the reformed smokers’ subgroups compared to current smokers. This could reflect the fact that smoke cessation decreases the rate of further mutations compared to current smokers. The reformed subgroups also had lower pack year histories compared to current smokers.
In summary, we have developed and validated a robust, non-subjective, quantitative scoring system for assessing smoke associated molecular damage in lung cancers. The scoring system will be of use in assessing the presence and degree of smoke associated molecular damage in lung cancers arising in ever and never smokers.
Acknowledgments
Funding: This work was supported by a grant from the National Cancer Institute, Bethesda, MD, USA: “Specialized Program in Research Excellence in Lung Cancer”, P50 CA70907, and by a generous donation from the Margot A. Johnson Lung Cancer Research Fund.
Footnote
Conflicts of Interest: The authors have no conflicts of interest to declare.
Ethical Statement: The authors analyzed data from already published citations. Thus there is no necessity for obtaining Institutional review Board Permission or Patient Consent.
References
- Alexandrov LB, Ju YS, Haase K, et al. Mutational signatures associated with tobacco smoking in human cancer. Science 2016;354:618-22. [Crossref] [PubMed]
- Couraud S, Debieuvre D, Moreau L, et al. No impact of passive smoke on the somatic profile of lung cancers in never-smokers. Eur Respir J 2015;45:1415-25. [Crossref] [PubMed]
- Krishnan VG, Ebert PJ, Ting JC, et al. Whole-genome sequencing of asian lung cancers: second-hand smoke unlikely to be responsible for higher incidence of lung cancer among Asian never-smokers. Cancer Res 2014;74:6071-81. [Crossref] [PubMed]
- Peto R, Darby S, Deo H, et al. Smoking, smoking cessation, and lung cancer in the UK since 1950: combination of national statistics with two case-control studies. BMJ 2000;321:323-9. [Crossref] [PubMed]
- Halpern MT, Gillespie BW, Warner KE. Patterns of absolute risk of lung cancer mortality in former smokers. J Natl Cancer Inst 1993;85:457-64. [Crossref] [PubMed]
- Ryan H, Trosclair A, Gfroerer J. Adult current smoking: differences in definitions and prevalence estimates--NHIS and NSDUH, 2008. J Environ Public Health 2012;2012. [Crossref] [PubMed]
- Alexandrov LB, Nik-Zainal S, Wedge DC, et al. Signatures of mutational processes in human cancer. Nature 2013;500:415-21. [Crossref]
- Govindan R, Ding L, Griffith M, et al. Genomic landscape of non-small cell lung cancer in smokers and never-smokers. Cell 2012;150:1121-34. [Crossref] [PubMed]
- Imielinski M, Berger AH, Hammerman PS, et al. Mapping the hallmarks of lung adenocarcinoma with massively parallel sequencing. Cell 2012;150:1107-20. [Crossref] [PubMed]
- Stoltzfus A, Norris RW. On the Causes of Evolutionary Transition:Transversion Bias. Mol Biol Evol 2016;33:595-602. [Crossref] [PubMed]
- Pfeifer GP, Hainaut P. On the origin of G --> T transversions in lung cancer. Mutat Res 2003;526:39-43. [Crossref] [PubMed]
- Rudin CM, Durinck S, Stawiski EW, et al. Comprehensive genomic analysis identifies SOX2 as a frequently amplified gene in small-cell lung cancer. Nat Genet 2012;44:1111-6. [Crossref] [PubMed]
- Peifer M, Fernandez-Cuesta L, Sos ML, et al. Integrative genome analyses identify key somatic driver mutations of small-cell lung cancer. Nat Genet 2012;44:1104-10. [Crossref] [PubMed]
- Fernandez-Cuesta L, Peifer M, Lu X, et al. Frequent mutations in chromatin-remodelling genes in pulmonary carcinoids. Nat Commun 2014;5:3518. [Crossref] [PubMed]
- Mann HB. Nonparametric Tests Against Trend. Econometrica 1945;13:245-59. [Crossref]
- Kendall MG. Rank correlation methods. Rank correlation methods. Oxford, England: Griffin, 1948.
- Langmead CJ, McClung CR, Donald BR. A maximum entropy algorithm for rhythmic analysis of genome-wide expression patterns. Proc IEEE Comput Soc Bioinform Conf 2002;1:237-45. [Crossref] [PubMed]
- Burkoff NS, Várnai C, Wild DL. Predicting protein β-sheet contacts using a maximum entropy-based correlated mutation measure. Bioinformatics 2013;29:580-7. [Crossref] [PubMed]
- Acharya UR, Raghavendra U, Fujita H, et al. Automated characterization of fatty liver disease and cirrhosis using curvelet transform and entropy features extracted from ultrasound images. Comput Biol Med 2016;79:250-8. [Crossref] [PubMed]
- Sun S, Schiller JH, Gazdar AF. Lung cancer in never smokers - a different disease. Nat Rev Cancer 2007;7:778-90. [Crossref] [PubMed]
- Pelosof L, Ahn C, Gao A, et al. Proportion of Never-Smoker Non-Small Cell Lung Cancer Patients at Three Diverse Institutions. J Natl Cancer Inst 2017.109. [PubMed]
- Swarts DR, Ramaekers FC, Speel EJ. Molecular and cellular biology of neuroendocrine lung tumors: evidence for separate biological entities. Biochim Biophys Acta 2012;1826:255-71. [PubMed]
- Riely GJ, Kris MG, Rosenbaum D, et al. Frequency and distinctive spectrum of KRAS mutations in never smokers with lung adenocarcinoma. Clin Cancer Res 2008;14:5731-4. [Crossref] [PubMed]
- Dogan S, Shen R, Ang DC, et al. Molecular epidemiology of EGFR and KRAS mutations in 3,026 lung adenocarcinomas: higher susceptibility of women to smoking-related KRAS-mutant cancers. Clin Cancer Res 2012;18:6169-77. [Crossref] [PubMed]
- Cancer Genome Atlas Research Network. Comprehensive molecular profiling of lung adenocarcinoma. Nature 2014;511:543-50. [Crossref]
- Rivera GA, Wakelee H. Lung Cancer in Never Smokers. Adv Exp Med Biol 2016;893:43-57. [Crossref] [PubMed]
- Couraud S, Souquet PJ, Paris C, et al. BioCAST/IFCT-1002: epidemiological and molecular features of lung cancer in never-smokers. Eur Respir J 2015;45:1403-14. [Crossref] [PubMed]
- Planchard D, Besse B. Lung cancer in never-smokers. Eur Respir J 2015;45:1214-7. [Crossref] [PubMed]
- Rossi G, Mengoli MC, Cavazza A, et al. Large cell carcinoma of the lung: clinically oriented classification integrating immunohistochemistry and molecular biology. Virchows Arch 2014;464:61-8. [Crossref] [PubMed]
- Pardo J, Martinez-Penuela AM, Sola JJ, et al. Large cell carcinoma of the lung: an endangered species? Appl Immunohistochem Mol Morphol 2009;17:383-92. [Crossref] [PubMed]
- Kim CH, Lee YC, Hung RJ, et al. Secondhand Tobacco Smoke Exposure and Lung Adenocarcinoma In Situ/Minimally Invasive Adenocarcinoma (AIS/MIA). Cancer Epidemiol Biomarkers Prev 2015;24:1902-6. [Crossref] [PubMed]
- Ren JH, He WS, Yan GL, et al. EGFR mutations in non-small-cell lung cancer among smokers and non-smokers: a meta-analysis. Environ Mol Mutagen 2012;53:78-82. [Crossref] [PubMed]
- Wells AJ, English PB, Posner SF, et al. Misclassification rates for current smokers misclassified as nonsmokers. Am J Public Health 1998;88:1503-9. [Crossref] [PubMed]