Biomarker for personalized immunotherapy
Introduction
In 2018, Nobel Prize in Physiology or Medicine was awarded to two famous scientists for their discovery of two checkpoints, cytotoxic T lymphocyte antigen-4 (CTLA-4) and programmed cell death 1 (PD-1) (1). Immunotherapy has emerged as a powerful weapon in multiple cancer types, with checkpoint blockade (CPB) blocking the PD-1 and programmed death ligand 1 (PD-L1) pathway (2). By releasing the ability of cytotoxic T cells, the innate immune system could recognize and kill cancer cells and prevent cancer metastasis. Wide application in cancer disease, overall survival (OS) greatly prolonged. Anti-PD-1/PD-L1 therapy proceed by steady steps from second line to first line setting in advanced stage non-small cell lung cancer (NSCLC) patients (3-6). No matter as monotherapy or combined therapy with platinum-based doublets, CPB have brought promising results with durable responses, improved OS and favorable tolerability in published phase III studies (7,8). Undoubtedly, one of the key points to the success is the biomarker design that should screen out suitable patients for CPB.
PD-L1 immunohistochemistry (IHC) testing with Dako 22C3 antibodies have been approved by Food and Drug Administration (FDA) as companion diagnostic for the treatment of pembrolizumab in NSCLC patients at first line and second line setting. Dako 28-8 and Ventana SP142 have been approved as complementary diagnostic for the treatment of nivolumab and atezolizumab in NSCLC patients at second line setting. Recently, FDA extend review period for frontline nivolumab plus ipilimumab in TMB-high NSCLC, because tumor mutation burden (TMB) is uncertain for the predictive value. Patients with driver gene variation are excluded from immunotherapy in multiple cancer guidelines. EGFR mutant or ALK rearranged patients would not be recommended CPB, but still clinical trials seek for possibility in progression with EGFR-TKIs but T790M negative patients. An increasing number of researches are in an attempt to explode a more favorable predictor of CPB response than PD-L1 expression alone. Thus, combined biomarkers, such as PD-L1 with tumor infiltrating lymphocytes (TILs), KRAS mutation with TP53 mutation, and other immune-related gene profile emerged, which not only could be a potential biomarker to predict efficacy of CPB, but give an illustration to understand the background of tumor microenvironment (TME). Here, we give an overview on the available and potential biomarkers for personalized immunotherapy.
PD-L1 expression
PD-1 is a receptor which expressed on the surface of cytotoxic T cells. Its ligands PD-L1 and PD-L2 express on the cell surface of macrophages, epithelial cells and other normal cells (9). The combination of PD1 and PD-L1 could protect normal cells from immune recognition and inhibiting the destruction by cytotoxic T cells. In recent years, increasing research indicated that tumors cells could evade immune surveillance by up-regulation of PD-L1 expression. When PD-L1 binds to the PD-1, activated T cells become inactivated and depleted, resulted in the prevention of tumor cells (TCs) from host immunity. So far, 2 PD-1 (nivolumab, pembrolizumab) and 2 PD-L1 blocking antibodies (durvalumab, atezolizumab) were approved by the FDA for the treatment of lung cancer (1).
Undoubtedly, PD-L1 expression is the only selected biomarker used for clinical practice. Tumor proportion score (TPS) should be calculated by IHC analysis with PD-L1 antibodies. In first line setting, NSCLC patients with PD-L1 expression (TPS ≥1%) could be treated with pembrolizumab monotherapy, and so as patients with positive PD-L1 expression (TPS ≥1%) in second line (5,6). Nivolumab and atezolizumab choosing unselected patients also achieved great success and are approved by FDA in second line setting (3,4,10).
Recent years, combined immunotherapy made great progress with astonishing speed, having stepped into first line treatment for unselected NSCLC patients. The series of Keynote clinical trials announced their results in New England Journal Medicine (11,12). Regardless of PD-L1 expression, pembrolizumab plus chemotherapy favored in longer survival than chemotherapy alone. Although the incidence of adverse events is comparable in two groups, toxicity in pembrolizumab-combination group is well tolerated. Nevertheless, in the sub-analysis, the survival differences in PD-L1 high group (TPS ≥50%) is bigger than PD-L1 low group (50%> TPS ≥1%) and PD-L1 negative group (TPS <1%). Altogether, these data indicate a new therapeutic strategy: (I) in the first line setting, TPS of PD-L1 expression is more than 50%. Pembrolizumab is recommended for NSCLC patients. When TPS is less than 50%, pembrolizumab plus platinum-based chemotherapy will be recommended. (II) In the second line setting, TPS is more than 1%. Pembrolizumab is recommended. When PD-L1 expression unknown or negative, nivolumab and atezolizumab is recommended (13).
However, as an imperfect biomarker, IHC analysis of PD-L1 expression remains a few questions to be settled on:
- Four IHC antibodies (Dako, 28-8; Dako, 22C3; Ventana, SP142; Ventana, SP263) are used for assessment of the positivity of PD-L1 expression in clinical trials evaluating clinical efficacy of different CPIs. Previous studies showed that the assessment of PD-L1 expression on TCs was comparable with 22C3, 28-8 and SP263 assays, while SP142 assay exhibiting lower positive expression. Immune cells (ICs) staining across four assays appears to be consistent, but more variable than for TC staining (14). Meanwhile, different cutoff values are applied for the evaluation of PD-L1 expression. Thus, standardization and harmonization of PD-L1 testing assays is urgently needed to be work on. Different international and national initiatives have been conducted for PD-L1 IHC harmonization and validation.
- Although at least two pathologists completed evaluation on PD-L1 expression on TCs by IHC independently, the proportion of inconsistent outcomes should be given more attention. After all, the assessment is indeed too subjective and empirical. In phase II results of the project Blueprint, strong reliability among pathologists in TC PD-L1 scoring with all five assays of PD-L1 IHC (22C3, 28-8, SP142, SP263, and 73-10), but poor reliability in IC PD-L1 scoring (15).
- Objective response rate (ORR) is not as high as expected. Only PD-L1 expression could not screen out most potential benefited patients, since low or negative stained tumor could also response to CPB. Comprehensive genomic signature is urgently needed to be explored.
- IHC staining need to be performed on tumor sample through invasive operations at the baseline of immunotherapy. Dynamic monitoring of clinical efficacy or prediction of primary resistance is impossible for IHC analysis of PD-L1 staining on tissue sections. However, relevant research has been initiated with exosomal PD-L1 expression or CTC PD-L1 expression obtaining from peripheral blood (16,17).
Altogether, PD-L1 expression has been accepted for clinic practice to identify if patients could be treated with CPB but it is still not enough. Combined with other biomarkers, such as TILs, tumor neoantigen, other checkpoints, such as Lag3, TIMs and TIGIT is the future direction (18,19).
TMB
TMB varies greatly between different cancer types, among which melanoma, lung adenocarcinoma and lung squamous carcinoma are the top three (20,21). Till now, four CPIs were approved by FDA for the treatment of melanoma and NSCLC in different stages, with PD-L1 expression as patients selected biomarker (1). After the failure of Checkmate 026, BMS used TMB as biomarker to conduct a retrospective analysis (22). The results showed that compared with PD-L1 expression, TMB could better identify benefited NSCLC patients for the treatment of nivolumab plus ipilimumab. TMB ≥10 Mut/Mb is selected as the cutoff value to definite TMB high population. Clinical efficacy was indeed more outstanding in patients with TMB ≥10 Mut/Mb, regardless of PD-L1 expression. Another study by Ready et al. (23) showed when PD-L1 ≥1%, the proportion of TMB ≥10 Mut/Mb and TMB <10 Mut/Mb is 70% versus 30%; when PD-L1 <1%, the proportion of two subgroups is 90% versus 10%. In previous study, patients with TMB results was less overlapped with PD-L1 expression (24). It seems that these two biomarkers could identify independent and unique population that could get benefit from CPB (25).
In 2018 AACR, the initial outcomes of CheckMate 227 indicated that median PFS was 7.2 months in TMB high patients who were treated with nivolumab plus ipilimumab at first line setting, compared with 5.4 months in chemo cohort (HR 0.75, 95% CI: 0.53–1.07). The results were consistent in PD-L1 <1% subgroups. Last year, FDA announced that review period will be extended for another 3 months for frontline nivolumab combined with ipilimumab in TMB-high NSCLC. Additional survival data was required in TMB-low patients. The final results showed that in TMB ≥10 Mut/Mb group, median OS is 23.0 versus 16.7 months (HR 0.77, 95% CI: 0.56 to 1.06); in TMB <10 Mut/Mb group, median OS is 16.2 months versus 12.4 months (HR 0.78, 95% CI: 0.61 to 1.00). In 2018, Devarakonda et al. reported the result of the Lung Adjuvant Cisplatin Evaluation (LACE)-Bio-II study and found that high nonsynonymous TMB appears to be a favorable prognostic biomarker for patients with resected NSCLC (26). A recent research by Samstein et al. demonstrated that higher TMB (top 20%) was associated with better OS after immunotherapy across multiple cancer types. The HR in NSCLC was 2.08 (95% CI: 1.61–2.68; P<0.001) (27). TMB cut-points associated with improved survival varied markedly, with 13.8 Mut/Mb in NSCLC. Regarding the predictive or prognostic role of TMB, available evidence is inconsistent (27,28). We need to wait and see the outcomes of other ongoing trials and research, avoiding to jump to an uncertain conclusion. Nevertheless, progress has been achieved by blood-based TMB (bTMB) as biomarker to predict PFS in patients receiving atezolizumab in NSCLC (24). The correlation between tumor tissue-based TMB and bTMB is positive. The proportion of shared variants is 59%, and the tissue only and blood only variants are 25% and 26%, respectively. It was confirmed in two clinical trial POPLAR and OAK that bTMB ≥16 Mut/Mb is a clinically meaningful cut-point in NSCLC patients. Another study published on the journal of JAMA Oncology, identified bTMB of 6 or higher correlated with favorable PFS in patients treated with CPIs (29). Meanwhile, 150 genes panel was used to illustrate that NGS has high consistence with WES for TMB evaluation. This is promising results and we hope that in the future, tissue biopsy for guiding the immunological therapeutic strategy is less needed.
TMB has a few limitations, although FDA has authorized FoundationOne CDx and MSK-IMPAC for clinical use. As is known, whole exome sequencing (WES) could better calculate TMB theoretically. However, owing to its great cost, long time consuming and complicated process, WES is only applied in scientific research (30). Available data showed that the consistence of comprehensive genomic profiling by next generation sequencing (NGS) and WES is strong and both could reflect the predictive role of TMB for CPIs (21). However, various platforms of NGS results has no standardization of TMB testing and no unified cutoff value, ranging from 7.4 to 10.0 Mut/Mb. In addition, analytical and preanalytical factors could affect the quantity and quality of DNA and thus influence the TMB values, which include sequencing depth, sample collection, input material quality and quantity and so on (30).
Tumor infiltrating cells
The existence of TILs is necessary for anti-PD-1/PD-L1 antibodies to restore the immunity of host to destroy TCs (31). Thus, the density of TILs by IHC analysis is another important predictor for clinical efficacy with CPB. Based on the infiltrating T cells and PD-L1 expression, TME could be divided into four groups: TILs+/PD-L1+; TILs−/PD-L1+; TILs+/PD-L1−; TILs−/PD-L1− (32,33). Another method for clustering the context of the immune TME is inclusion with many immune-related factors as immune profiles to predict responses to immunotherapy. One subtype with presence of multiple TILs subpopulations and positive PD-L1 expression, regarded as adaptive immune resistance or inflamed tumor, is the most likely to respond to immunotherapy (34). In fact, the effects of the immune infiltrate on the prognosis of patients with cancer is much different. CD8+ T cells, tertiary lymphoid structures and M1 macrophages have a positive prognostic association with OS (35,36).
Not only the density but also the location of TILs plays a predictive role on clinical efficacy of CPB. High infiltration of cytotoxic T cells, within a certain distance to cancer cells, significantly correlated with prolonged patient survival. It seems that the cytotoxic T cells near to cancer cells has important immune biological function (37). Accompanied by the emergence of single-cell sequencing, profiling single ICs in tumor samples of patients treated with CPB is no longer an impossible thing (38).
Defining certain phenotypes of ICs associated with response to CPB could be achieved by bioinformatic analysis on clustering infiltrated T cells (39-41). In melanoma, researchers found that the presence of TCF7 protein on CD8+ T cells was related to clinical response to CPB. Although CD8+ T cells is regarded as immune effector cells with the function to recognize and kill TCs, diverse phenotypes of CD8+ T cells are undefined. Last year, Simoni and his colleges identified a subtype of CD8+ TILs with the absence of CD39, named as bystander CD8+ T cells, which are not specific for tumor antigens (42). It greatly broadens our knowledge of immune TME and the ability of TILs subpopulations. Further study still needs to find something else about the function of T cells and unknown T cells phenotypes.
Genetic variations
Gene testing gives us much information to guide clinic decision. In addition to TMB, somatic mutations assistant to classify tumors into different molecular subtypes. Clinical trials with CPB mostly exclude NSCLC patients with EGFR mutation and ALK rearrangement. In addition, patients with ROS1 rearrangement and BRAF mutations should also be excluded, owing to better clinical efficacy of targeted therapy. It has been furtherly proved that these genetic variation lead to unfavorable efficacy to immunotherapy (43). Less PD-L1 expression and fewer CD8+ TILs in patients with driver gene mutations could be the underlying mechanism of the lower response rate to CPB (44). Dong et al. brought an insight into the background of immune context and PD-L1 expression in patients with EGFR mutation. Uninflamed phenotype and weak immunogenicity could interpret the impaired response to PD-1 blockade. Targeted therapy with EGFR-TKIs is the standard treatment for EGFR mutation with advanced stage NSCLC. However, 7% patients with primary resistance could not get clinical benefit from TKIs. An interesting finding from Su et al. demonstrated that PD-L1 expression would give an interpretation of the poor response and de novo resistance to EGFR-TKIs (45). Almost half of the patients with de novo resistance have PD-L1 and CD8 co-expression. These patients may have potential therapeutic sensitivity to PD-1 blockade therapy. It seems that when EGFR-TKIs is not suitable for EGFR mutant patients, PD-L1 and CD8 IHC analysis maybe recommended to give guidance for the treatment of CPB.
So far, no effective inhibitors targeting KRAS mutation is available in the clinic. Previous studies found that KRAS and TP53 mutation have potential predictive value for response to PD-1 blockade therapy (46-48). Dong et al. illustrated that positive PD-L1 expression, CD8+T cell infiltration and high mutation burden maybe the reason of favorable clinical benefit from CPB, comparing with other genetic mutation. Mutations in the LKB1 (STK11) are the third most frequent genetic variation in NSCLC, which often presents with KRAS mutations. STK11 is a tumor suppressor gene that could exerts a negative regulation on the mTOR pathway. Recent studies showed that KRAS G12D, combined with STK11 mutations could result in inferior prognosis in NSCLC patients (48). However, combined with STK11, KRAS mutation would be resulted in immune invasion and primary resistance to anti-PD-1 therapy (49,50). Additionally, patients with JAK1/2 mutations may not respond to CPB. Because this genetic alteration would inhibit the downstream pathway of IFN-gamma and the favorable effects of anti-tumor immunity (2). Other mutations would lead to primary or adaptive resistant mechanism, such as B2M truncating mutation and loss of PTEN (51,52). However, these claims indeed make sense theoretically, but need to be confirmed by clinical data. Thus, resistance mechanisms and relevant biomarkers are under intensive investigation and development.
TCR repertoire
T lymphocytes play a vital role in immune-protection mechanism. The diversity of T-cell antigen receptors (TCR) is dependent on the randomization of VDJ rearrangement, which furtherly results in the diversity of CDR3 region (53). With the advent of TCR sequencing, the internal immunity triggered by tumor neoantigens could be better understood. This new technology, combined with single-cell sequencing, could comprehensively analyze the antigen-specific T cells response. TCR repertoire reflect adaptive immune system in cancer patients after the treatment of immunotherapy, which could be a potential predictive biomarker for immune responses (54).
In recent years, TCR sequencing has already conquered a few challenges and make it possible not only to track the T cell subtypes that infiltrating in tumor microenvironment, but also to identify the dominant TCR clonotypes in tumor lesion and peripheral blood (55). TCR repertoire analysis could give two dimensions of information, namely T cells diversity and clonality. However, relevant research regarding lung cancer is scarce. Previous studies showed that the dominant T lymphocytes clones could be greatly different from primary tumor lesions to normal margins, and even to peripheral blood (56-58). This could be illustrated by the heterogeneity of TILs in tumor microenvironment and the dynamic changes in peripheral circulation. In addition, another important data is that if the diversity of T cells in tumor lesions or blood could be predictive to the response to immunotherapy, such CPIs. Similarly, limited data indicated that conclusions of available findings regarding prediction of response or survival to CPB are inconsistent (58-60). Altogether, TCR sequencing still have a long way to go.
Predictive biomarkers for HPD
A proportion of patients will present primary resistance to CPB therapy, which origins from lack of antigenic mutations, de-differentiation with loss of tumor antigen expression, constitutive PD-L1 expression and loss of HLA expression (52,61). Recent studies told us that immune CPB sometimes could make things worse.
Hyperprogressive disease (HPD) defined as double tumor growth rates between baseline and treatment. A small subtype of NSCLC patients could develop HPD, which likely emerges in older patients with a shorter median OS (62). This new pattern of disease progression in cancer patients treated with PD-1/PD-L1 blockade also happens on head and neck squamous cell carcinoma and other cancer types (63). Previous study performed analysis on genomic alteration associated with CPB treatment. Patients with MDM2 amplification and EGFR aberrations maybe the potential reasons of HPD patterns (64). Recently, Lo Russo et al. found that enrichment of tumor-associated macrophages (TAM) could be one mechanism for HPD in NSCLC patients followed by PD-1 blockade (65). Due to the limited evidence, further investigation is urgently needed.
CTC PD-L1 expression
Circulating TCs (CTCs) is derived from primary or metastatic lesions, which could reflect the genetic or epigenetic variations in tumor tissue. Non-invasive liquid biopsy of circulating tumor DNA (ctDNA) for gene testing is achieved by NGS. Since PD-L1 expression in tumor tissue is an imperfect biomarker for CPB prediction, efforts have been made to investigate the performance of CTCs as an alternative biomarker to evaluated PD-L1 expression.
Cell Search CXC Kit, Vortex HT chip, or digital PCR detection following microfluidic enrichment process are applied for capture CTCs from blood when patients under treatment (17,66-68). Detectable rate, which means the proportion of peripheral blood contains at least one CTC, is around 83–97%, depending on the sensitivity of capture methods. On the basis of limited data, PD-L1 expression on CTCs and concordance rate of PD-L1 expression on tumor tissue and CTCs vary markedly in different studies (17,69). Nevertheless, the prognostic trending of PD-L1 on CTCs in NSCLC patients has been verified by use of various PD-L1 primary antibodies, and various thresholds. And a few case reports showed dynamic PD-L1 + CTC count may help to identify changes in response to treatment, and these cells could be a readily obtainable source to understand tumor evolution (17,67).
However, current studies on association of CTCs with PD-L1 positive expression and response to CPB is not enough for blood-based monitoring. Hong et al. combined microfluidic enrichment for CTCs together with RNA based droplet digital PCR quantitation. Molecular signatures of CTCs score in melanoma was used to predict early response to CPB. Outcomes presented that decrease in CTC score made a good correlation with marked improvement in PFS (HR 0.17; P=0.008) and OS (HR 0.12; P=0.04) (70).
Although there are several advantages of the PD-L1 expression on CTCs, such as minimally invasive inspection method without high risk of complications; dynamic monitor at different points during the CPB treatment; providing important complementary information on therapeutic responses; refection of heterogeneity of tumor tissue. Uncertain questions remain: (I) the stability and specificity of CTCs capture methods vary greatly; (II) which IHC antibodies should by applied for PD-L1 staining; (III) how to define PD-L1 positive expression; (IV) what is the optimal cutoff points of PD-L1 to correlate with clinical efficacy of immunotherapy with CPB.
Comprehensive signature
Tremendous progress has been made in checkpoints blockade. Current potential predictive markers have been mentioned above separately. However, the background of immune TME and the tumor-IC interaction are very complicated (35). To identify the determinants of tumor immunogenicity, increasing efforts have been made by incorporating all the immune-related biomarkers in TME and peripheral blood. Comprehensive analysis is increasingly performed, using genomic data and bioinformatics tools (71). Charoentong and his colleges analyzed the data from TCGA database and clinical trials with CPIs in 20 solid cancers and named the great project as The Cancer Immunome Atlas (TCIA). Combining the TILs, cancer antigenomes, tumor heterogeneity and immunophenotypes, the immunophenoscore was developed that could be a predictor of response to CPIs in melanoma (AUC =1.00, 95% CI: 0.99–1.00). In addition, they open the database to researchers for further data mining from the immunological insights, having successfully developed web-accessible resource (72). Using immuno-gram or immune-score, researchers aim to better understand why some patients respond to immunotherapy and why some not, ultimately aiming at improving anticancer therapy (73,74).
Karasaki et al. performed WES and RNA sequencing in 20 resected NSCLC patients. Three immune-gram patterns characterized by gene signatures were identified. However, there are no results on association between three immune-gram patterns with CPB efficacy (75). An increasing number of researches on machine learning emerged in different fields. Charoentong and his colleges using machine learning developed a superior predictor, termed as immunophenoscore, and verify the response to checkpoint antibodies in two independent validation cohorts. The interactive big data resource includes 28 TIL subpopulation, neoantigens, mutation load, and tumor heterogeneity. Authors illustrated a lot of associations suggesting important biological conclusions with implications for cancer immunotherapy. In this year, Zhang et al. published their results on the journal of Nature Communication which is the first report with multiple sequencing data from Chinese NSCLC patients (76). Racial differences between Chinese and Westerner could be greatly achieved by the comparing the genomic and immunological characteristics from surgical tissue and TCGA database. Although there are no data regarding the clinical efficacy of CPIs, this report provides theoretical basis for the development of future immunotherapy strategies.
Available data online provides a good opportunity for many scientists to do relevant immunological research. But the premise is that they must have a strong bioinformatic analysis team to interpret these huge and complicated data repositories and find the key determinants of favorable efficacy of CPIs in lung cancer patients.
In conclusion, PD-L1 expression by IHC testing is recommended for CPB treatment in first line and beyond line settings. With the advent of NGS, gene status could give a new sight for the guidance of immunotherapy. It should be cautious when patients with EGFR mutation, ALK rearrangement, or KRAS and STK11 or LKB1 co-mutation was treated with PD-1/PD-L1 blockade. TMB ≥10 Mut/Mb as a selected biomarker still lack sufficient evidence and further study need to confirm the predictive or prognostic role. Comprehensive analysis with multiple immune-related biomarkers is a promising research orientation on prediction of CPB (Figure 1). In the future, the role of liquid biopsy with CTC and exosomes, and gut microbiota on CPB prediction still need further exploration. Altogether, there is a long way for searching optimal biomarkers in anti-PD-1/PD-L1 blockade.
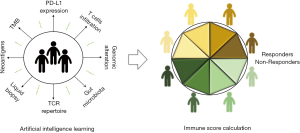
Acknowledgments
Funding: This work was supported by the following grants: National Key R&D Program of China (Grant No. 2016YFC1303800); Key Lab System Project of Guangdong Science and Technology Department – Guangdong Provincial Key Lab of Translational Medicine in Lung Cancer (Grant No. 2012A061400006/2017B030314120); Special Fund of Public Interest by National Health and Family Control Committee (Grant No. 201402031).
Footnote
Conflicts of Interest: The authors have no conflicts of interest to declare.
Ethical Statement: The authors are accountable for all aspects of the work in ensuring that questions related to the accuracy or integrity of any part of the work are appropriately investigated and resolved.
References
- Liu SY, Wu YL. An immunological storm for cancer therapy: 2018 Nobel Prize in Physiology or Medicine. Sci Bull 2018;63:1608-10. [Crossref]
- Ribas A, Wolchok JD. Cancer immunotherapy using checkpoint blockade. Science 2018;359:1350-5. [Crossref] [PubMed]
- Borghaei H, Paz-Ares L, Horn L, et al. Nivolumab versus Docetaxel in Advanced Nonsquamous Non-Small-Cell Lung Cancer. N Engl J Med 2015;373:1627-39. [Crossref] [PubMed]
- Brahmer J, Reckamp KL, Baas P, et al. Nivolumab versus Docetaxel in Advanced Squamous-Cell Non-Small-Cell Lung Cancer. N Engl J Med 2015;373:123-35. [Crossref] [PubMed]
- Herbst RS, Baas P, Kim DW, et al. Pembrolizumab versus docetaxel for previously treated, PD-L1-positive, advanced non-small-cell lung cancer (KEYNOTE-010): a randomised controlled trial. Lancet 2016;387:1540-50. [Crossref] [PubMed]
- Reck M, Rodriguez-Abreu D, Robinson AG, et al. Pembrolizumab versus Chemotherapy for PD-L1-Positive Non-Small-Cell Lung Cancer. N Engl J Med 2016;375:1823-33. [Crossref] [PubMed]
- Galon J, Bruni D. Approaches to treat immune hot, altered and cold tumours with combination immunotherapies. Nat Rev Drug Discov 2019;18:197-218. [Crossref] [PubMed]
- Hellmann MD, Ciuleanu TE, Pluzanski A, et al. Nivolumab plus Ipilimumab in Lung Cancer with a High Tumor Mutational Burden. N Engl J Med 2018;378:2093-104. [Crossref] [PubMed]
- Boussiotis VA. Molecular and Biochemical Aspects of the PD-1 Checkpoint Pathway. N Engl J Med 2016;375:1767-78. [Crossref] [PubMed]
- Rittmeyer A, Barlesi F, Waterkamp D, et al. Atezolizumab versus docetaxel in patients with previously treated non-small-cell lung cancer (OAK): a phase 3, open-label, multicentre randomised controlled trial. Lancet 2017;389:255-65. [Crossref] [PubMed]
- Gandhi L, Rodriguez-Abreu D, Gadgeel S, et al. Pembrolizumab plus Chemotherapy in Metastatic Non-Small-Cell Lung Cancer. N Engl J Med 2018;378:2078-92. [Crossref] [PubMed]
- Paz-Ares L, Luft A, Vicente D, et al. Pembrolizumab plus Chemotherapy for Squamous Non-Small-Cell Lung Cancer. N Engl J Med 2018;379:2040-51. [Crossref] [PubMed]
- Wu YL, Planchard D, Lu S, et al. Pan-Asian adapted Clinical Practice Guidelines for the management of patients with metastatic non-small-cell lung cancer: a CSCO-ESMO initiative endorsed by JSMO, KSMO, MOS, SSO and TOS. Ann Oncol 2019;30:171-210. [Crossref] [PubMed]
- Hirsch FR, McElhinny A, Stanforth D, et al. PD-L1 Immunohistochemistry Assays for Lung Cancer: Results from Phase 1 of the Blueprint PD-L1 IHC Assay Comparison Project. J Thorac Oncol 2017;12:208-22. [Crossref] [PubMed]
- Tsao MS, Kerr KM, Kockx M, et al. PD-L1 Immunohistochemistry Comparability Study in Real-Life Clinical Samples: Results of Blueprint Phase 2 Project. J Thorac Oncol 2018;13:1302-11. [Crossref] [PubMed]
- Chen G, Huang AC, Zhang W, et al. Exosomal PD-L1 contributes to immunosuppression and is associated with anti-PD-1 response. Nature 2018;560:382-6. [Crossref] [PubMed]
- Ilié M, Szafer-Glusman E, Hofman V, et al. Detection of PD-L1 in circulating tumor cells and white blood cells from patients with advanced non-small-cell lung cancer. Ann Oncol 2018;29:193-9. [Crossref] [PubMed]
- Thorsson V, Gibbs DL, Brown SD, et al. The Immune Landscape of Cancer. Immunity 2018;48:812-30.e14. [Crossref] [PubMed]
- Anderson AC, Joller N, Kuchroo VK. Lag-3, Tim-3, and TIGIT: Co-inhibitory Receptors with Specialized Functions in Immune Regulation. Immunity 2016;44:989-1004. [Crossref] [PubMed]
- Alexandrov LB, Nik-Zainal S, Wedge DC, et al. Signatures of mutational processes in human cancer. Nature 2013;500:415-21. [Crossref] [PubMed]
- Chalmers ZR, Connelly CF, Fabrizio D, et al. Analysis of 100,000 human cancer genomes reveals the landscape of tumor mutational burden. Genome Med 2017;9:34. [Crossref] [PubMed]
- Carbone DP, Reck M, Paz-Ares L, et al. First-Line Nivolumab in Stage IV or Recurrent Non-Small-Cell Lung Cancer. N Engl J Med 2017;376:2415-26. [Crossref] [PubMed]
- Ready N, Hellmann MD, Awad MM, et al. First-Line Nivolumab Plus Ipilimumab in Advanced Non-Small-Cell Lung Cancer (CheckMate 568): Outcomes by Programmed Death Ligand 1 and Tumor Mutational Burden as Biomarkers. J Clin Oncol 2019;37:992-1000. [Crossref] [PubMed]
- Gandara DR, Paul SM, Kowanetz M, et al. Blood-based tumor mutational burden as a predictor of clinical benefit in non-small-cell lung cancer patients treated with atezolizumab. Nat Med 2018;24:1441-8. [Crossref] [PubMed]
- Hellmann MD, Nathanson T, Rizvi H, et al. Genomic Features of Response to Combination Immunotherapy in Patients with Advanced Non-Small-Cell Lung Cancer. Cancer Cell 2018;33:843-52.e4. [Crossref] [PubMed]
- Devarakonda S, Rotolo F, Tsao MS, et al. Tumor Mutation Burden as a Biomarker in Resected Non-Small-Cell Lung Cancer. J Clin Oncol 2018;36:2995-3006. [Crossref] [PubMed]
- Samstein RM, Lee CH, Shoushtari AN. Tumor mutational load predicts survival after immunotherapy across multiple cancer types. Nat Genet 2019;51:202-6. [Crossref] [PubMed]
- Yarchoan M, Hopkins A, Jaffee EM. Tumor Mutational Burden and Response Rate to PD-1 Inhibition. N Engl J Med 2017;377:2500-1. [Crossref] [PubMed]
- Wang Z, Duan J, Cai S, et al. Assessment of Blood Tumor Mutational Burden as a Potential Biomarker for Immunotherapy in Patients With Non-Small Cell Lung Cancer With Use of a Next-Generation Sequencing Cancer Gene Panel. JAMA Oncol 2019;5:696-702. [Crossref] [PubMed]
- Chan TA, Yarchoan M, Jaffee E, et al. Development of tumor mutation burden as an immunotherapy biomarker: utility for the oncology clinic. Ann Oncol 2019;30:44-56. [Crossref] [PubMed]
- Havel JJ, Chowell D, Chan TA. The evolving landscape of biomarkers for checkpoint inhibitor immunotherapy. Nat Rev Cancer 2019;19:133-50. [Crossref] [PubMed]
- Zhang Y, Chen L. Classification of Advanced Human Cancers Based on Tumor Immunity in the MicroEnvironment (TIME) for Cancer Immunotherapy. JAMA Oncol 2016;2:1403-4. [Crossref] [PubMed]
- Teng MW, Ngiow SF, Ribas A, et al. Classifying Cancers Based on T-cell Infiltration and PD-L1. Cancer Res 2015;75:2139-45. [Crossref] [PubMed]
- Chen DS, Mellman I. Elements of cancer immunity and the cancer-immune set point. Nature 2017;541:321-30. [Crossref] [PubMed]
- Fridman WH, Zitvogel L, Sautes-Fridman C, et al. The immune contexture in cancer prognosis and treatment. Nat Rev Clin Oncol 2017;14:717-34. [Crossref] [PubMed]
- Donnem T, Hald SM, Paulsen EE, et al. Stromal CD8+ T-cell Density-A Promising Supplement to TNM Staging in Non-Small Cell Lung Cancer. Clin Cancer Res 2015;21:2635-43. [Crossref] [PubMed]
- Carstens JL, Correa de Sampaio P, Yang D, et al. Spatial computation of intratumoral T cells correlates with survival of patients with pancreatic cancer. Nat Commun 2017;8:15095. [Crossref] [PubMed]
- Azizi E, Carr AJ, Plitas G, et al. Single-Cell Map of Diverse Immune Phenotypes in the Breast Tumor Microenvironment. Cell 2018;174:1293-308.e36. [Crossref] [PubMed]
- Zheng C, Zheng L, Yoo JK, et al. Landscape of Infiltrating T Cells in Liver Cancer Revealed by Single-Cell Sequencing. Cell 2017;169:1342-56.e16. [Crossref] [PubMed]
- Lavin Y, Kobayashi S, Leader A, et al. Innate Immune Landscape in Early Lung Adenocarcinoma by Paired Single-Cell Analyses. Cell 2017;169:750-65.e17. [Crossref] [PubMed]
- Lambrechts D, Wauters E, Boeckx B, et al. Phenotype molding of stromal cells in the lung tumor microenvironment. Nat Med 2018;24:1277-89. [Crossref] [PubMed]
- Simoni Y, Becht E, Fehlings M, et al. Bystander CD8(+) T cells are abundant and phenotypically distinct in human tumour infiltrates. Nature 2018;557:575-9. [Crossref] [PubMed]
- Gainor JF, Shaw AT, Sequist LV, et al. EGFR Mutations and ALK Rearrangements Are Associated with Low Response Rates to PD-1 Pathway Blockade in Non-Small Cell Lung Cancer: A Retrospective Analysis. Clin Cancer Res 2016;22:4585-93. [Crossref] [PubMed]
- Liu SY, Dong ZY, Wu SP, et al. Clinical relevance of PD-L1 expression and CD8+ T cells infiltration in patients with EGFR-mutated and ALK-rearranged lung cancer. Lung Cancer 2018;125:86-92. [Crossref] [PubMed]
- Su S, Dong ZY, Xie Z, et al. Strong Programmed Death Ligand 1 Expression Predicts Poor Response and De Novo Resistance to EGFR Tyrosine Kinase Inhibitors Among NSCLC Patients With EGFR Mutation. J Thorac Oncol 2018;13:1668-75. [Crossref] [PubMed]
- Dong ZY, Zhong WZ, Zhang XC, et al. Potential Predictive Value of TP53 and KRAS Mutation Status for Response to PD-1 Blockade Immunotherapy in Lung Adenocarcinoma. Clin Cancer Res 2017;23:3012-24. [Crossref] [PubMed]
- Biton J, Mansuet-Lupo A, Pecuchet N, et al. TP53, STK11, and EGFR Mutations Predict Tumor Immune Profile and the Response to Anti-PD-1 in Lung Adenocarcinoma. Clin Cancer Res 2018;24:5710-23. [Crossref] [PubMed]
- Aredo JV, Padda SK, Kunder CA, et al. Impact of KRAS mutation subtype and concurrent pathogenic mutations on non-small cell lung cancer outcomes. Lung Cancer 2019;133:144-50. [Crossref] [PubMed]
- Skoulidis F, Byers LA, Diao L, et al. Co-occurring genomic alterations define major subsets of KRAS-mutant lung adenocarcinoma with distinct biology, immune profiles, and therapeutic vulnerabilities. Cancer Discov 2015;5:860-77. [Crossref] [PubMed]
- Rizvi H, Sanchez-Vega F, La K, et al. Molecular Determinants of Response to Anti-Programmed Cell Death (PD)-1 and Anti-Programmed Death-Ligand 1 (PD-L1) Blockade in Patients With Non-Small-Cell Lung Cancer Profiled With Targeted Next-Generation Sequencing. J Clin Oncol 2018;36:633-41. [Crossref] [PubMed]
- Zaretsky JM, Garcia-Diaz A, Shin DS, et al. Mutations Associated with Acquired Resistance to PD-1 Blockade in Melanoma. N Engl J Med 2016;375:819-29. [Crossref] [PubMed]
- Sharma P, Hu-Lieskovan S, Wargo JA, Ribas A. Primary, Adaptive, and Acquired Resistance to Cancer Immunotherapy. Cell 2017;168:707-23. [Crossref] [PubMed]
- van der Merwe PA, Dushek O. Mechanisms for T cell receptor triggering. Nat Rev Immunol 2011;11:47-55. [Crossref] [PubMed]
- Schrama D, Ritter C, Becker JC. T cell receptor repertoire usage in cancer as a surrogate marker for immune responses. Semin Immunopathol 2017;39:255-68. [Crossref] [PubMed]
- Nielsen SCA, Boyd SD. Human adaptive immune receptor repertoire analysis-Past, present, and future. Immunol Rev 2018;284:9-23. [Crossref] [PubMed]
- Sherwood AM, Emerson RO, Scherer D, et al. Tumor-infiltrating lymphocytes in colorectal tumors display a diversity of T cell receptor sequences that differ from the T cells in adjacent mucosal tissue. Cancer Immunol Immunother 2013;62:1453-61. [Crossref] [PubMed]
- Chen Z, Zhang C, Pan Y, et al. T cell receptor beta-chain repertoire analysis reveals intratumour heterogeneity of tumour-infiltrating lymphocytes in oesophageal squamous cell carcinoma. J Pathol 2016;239:450-8. [Crossref] [PubMed]
- Kudo Y, Haymaker C, Zhang J, et al. Suppressed immune microenvironment and repertoire in brain metastases from patients with resected non-small cell lung cancer. Ann Oncol 2019. [Epub ahead of print]. [Crossref] [PubMed]
- Postow MA, Manuel M, Wong P, et al. Peripheral T cell receptor diversity is associated with clinical outcomes following ipilimumab treatment in metastatic melanoma. J Immunother Cancer 2015;3:23. [Crossref] [PubMed]
- Robert L, Tsoi J, Wang X, et al. CTLA4 blockade broadens the peripheral T-cell receptor repertoire. Clin Cancer Res 2014;20:2424-32. [Crossref] [PubMed]
- Syn NL, Teng MWL, Mok TSK, et al. De-novo and acquired resistance to immune checkpoint targeting. Lancet Oncol 2017;18:e731-41. [Crossref] [PubMed]
- Champiat S, Dercle L, Ammari S, et al. Hyperprogressive Disease Is a New Pattern of Progression in Cancer Patients Treated by Anti-PD-1/PD-L1. Clin Cancer Res 2017;23:1920-8. [Crossref] [PubMed]
- Saâda-Bouzid E, Defaucheux C, Karabajakian A, et al. Hyperprogression during anti-PD-1/PD-L1 therapy in patients with recurrent and/or metastatic head and neck squamous cell carcinoma. Ann Oncol 2017;28:1605-11. [Crossref] [PubMed]
- Kato S, Goodman A, Walavalkar V, et al. Hyperprogressors after Immunotherapy: Analysis of Genomic Alterations Associated with Accelerated Growth Rate. Clin Cancer Res 2017;23:4242-50. [Crossref] [PubMed]
- Lo Russo G, Moro M, Sommariva M, et al. Antibody-Fc/FcR Interaction on Macrophages as a Mechanism for Hyperprogressive Disease in Non-small Cell Lung Cancer Subsequent to PD-1/PD-L1 Blockade. Clin Cancer Res 2019;25:989-99. [Crossref] [PubMed]
- Nicolazzo C, Raimondi C, Mancini M, et al. Monitoring PD-L1 positive circulating tumor cells in non-small cell lung cancer patients treated with the PD-1 inhibitor Nivolumab. Sci Rep 2016;6:31726. [Crossref] [PubMed]
- Dhar M, Wong J, Che J, et al. Evaluation of PD-L1 expression on vortex-isolated circulating tumor cells in metastatic lung cancer. Sci Rep 2018;8:2592. [Crossref] [PubMed]
- Yue C, Jiang Y, Li P, et al. Dynamic change of PD-L1 expression on circulating tumor cells in advanced solid tumor patients undergoing PD-1 blockade therapy. OncoImmunology 2018;7:e1438111. [Crossref] [PubMed]
- Guibert N, Delaunay M, Lusque A, et al. PD-L1 expression in circulating tumor cells of advanced non-small cell lung cancer patients treated with nivolumab. Lung Cancer 2018;120:108-12. [Crossref] [PubMed]
- Hong X, Sullivan RJ, Kalinich M, et al. Molecular signatures of circulating melanoma cells for monitoring early response to immune checkpoint therapy. Proc Natl Acad Sci U S A 2018;115:2467-72. [Crossref] [PubMed]
- Li B, Severson E, Pignon JC, et al. Comprehensive analyses of tumor immunity: implications for cancer immunotherapy. Genome Biol 2016;17:174. [Crossref] [PubMed]
- Charoentong P, Finotello F, Angelova M, et al. Pan-cancer Immunogenomic Analyses Reveal Genotype-Immunophenotype Relationships and Predictors of Response to Checkpoint Blockade. Cell Rep 2017;18:248-62. [Crossref] [PubMed]
- Mezquita L, Auclin E, Ferrara R, et al. Association of the Lung Immune Prognostic Index With Immune Checkpoint Inhibitor Outcomes in Patients With Advanced Non-Small Cell Lung Cancer. JAMA Oncol 2018;4:351-7. [Crossref] [PubMed]
- Zahoor H, Grivas P. The Cancer Immunogram: A Pledge for a Comprehensive Biomarker Approach for Personalized Immunotherapy in Urothelial Cancer. Eur Urol 2019;75:445-7. [Crossref] [PubMed]
- Karasaki T, Nagayama K, Kuwano H, et al. An Immunogram for the Cancer-Immunity Cycle: Towards Personalized Immunotherapy of Lung Cancer. J Thorac Oncol 2017;12:791-803. [Crossref] [PubMed]
- Zhang XC, Wang J, Shao GG, et al. Comprehensive genomic and immunological characterization of Chinese non-small cell lung cancer patients. Nat Commun 2019;10:1772. [Crossref] [PubMed]