Targeted sequencing analysis of cell-free DNA from metastatic non-small-cell lung cancer patients: clinical and biological implications
Introduction
Evidence suggests that the analysis of circulating cell-free DNA (cfDNA) isolated from plasma represents a valuable alternative for biomarker testing in patients with metastatic non-small-cell lung cancer (mNSCLC) when a tissue specimen is not available (1-3). Indeed, a number of studies have demonstrated that EGFR mutation testing in cfDNA has a quite high specificity, although the sensitivity ranges between 50% and 80% depending on the technology that is employed (4-6). Importantly, the overall response rate (ORR) and the progression free survival (PFS) of patients treated on the basis of the EGFR test performed on cfDNA are comparable to those observed for tissue testing (7-9).
In the above-described studies, analysis of cfDNA was performed using techniques that allow to investigate few loci per analysis, such as real-time PCR or droplet digital PCR (ddPCR). However, driver alterations that offer possibility of targeted treatment with approved drugs or within clinical trials have been described in over 70% of lung adenocarcinomas (10). For this reason, targeted sequencing panels with improved sensitivity have been developed for the analysis of cfDNA. Preliminary data suggest that patients treated with targeted agents based on the results of next generation sequencing (NGS) testing of cfDNA have a high response rate (11,12).
Despite these encouraging preliminary data, there is an increasing skepticism in the use of NGS-based techniques for the analysis of cfDNA. This uncertainty is mainly due to the observation that the concordance rate between tissue and plasma mutation profiles is often low (13). Whereas it might be expected to find mutations in tissue that are not detected in cfDNA for the low sensitivity of the test, the identification in plasma of mutations not found in tissue has raised concerns on the specificity and the clinical utility of the test (11). In this respect, results of a pilot external quality assessment (EQA) scheme for testing EGFR and KRAS/NRAS mutations in cfDNA showed that laboratory using NGS had the highest error rate (14). These concerns have been further increased by a recent study demonstrating a high discordant rate between different NGS-based cfDNA platforms, with an unexpected wide range of sensitivity (38% to 89%) and positive predictive value (PPV) (36% to 80%) (15). Although sequencing artifacts were reported as the main cause of such discordance, we must take into account that other phenomena such as clonal hematopoietic mutations of indeterminate potential (CHIP) and tumor heterogeneity might also contribute to the different results observed between tissue and cfDNA testing.
The availability on the market of NGS-based panels for cfDNA analysis has led to a significant increase in laboratories offering this test in routine clinical practice. However, the above-described findings highlight the need for an appropriate validation and verification of NGS testing methods before they are employed in the clinical practice. In this study, we first optimized a targeted sequencing-based cfDNA assay by using plasma samples from healthy individuals and next applied this test to cfDNA obtained from mNSCLC. By using this approach, we assessed the influence of sequencing artifacts, tumor heterogeneity and CHIP on the discordance between tissue- and plasma-based tests.
Methods
Patient population
The study included 107 patients with mNSCLC, for which a tumor specimen and a matched plasma sample was available at diagnosis, before starting anticancer therapy. Plasma obtained from 30 healthy donors with no personal history of cancer was also included in this study. The study has been approved by the Ethical Committee of the Pascale Institute (Protocol n16/14 OSS and 21/14 OSS).
Genomic DNA extraction from tumor samples
Genomic DNA (gDNA) was isolated from two 10 µm FFPE tissue sections using the QIAamp DNA FFPE Tissue kit (Qiagen, Hilden, Germany) according to the automated protocol performed in the QIAcube apparatus (Qiagen). The gDNA from cytological samples was extracted using the QIAamp DNA Micro kit (Qiagen) according to manufacturer’s protocol.
The gDNA quantity was assessed with the dsDNA HS assay kit using the Qubit 2.0 Fluorometer (Invitrogen, Monza, Italy).
cfDNA extraction from plasma samples
Peripheral blood was collected into 10.0 mL BD Vacutainer® plastic tubes containing EDTA (BD Diagnostics, Milan, Italy). The plasma fraction was obtained and stored as previously described (16). cfDNA was extracted from 2 mL of plasma from 107 mNSCLC patients and 30 healthy volunteers with the QIAamp Circulating Nucleic Acid Kit (Qiagen), with the exception of six healthy donors in which volumes of plasma ranging between 1 and 1.5 mL were available (Table S1). The cfDNA quantity was assessed with the dsDNA HS assay kit on the Qubit 2.0 Fluorometer (Invitrogen, Monza, Italy).
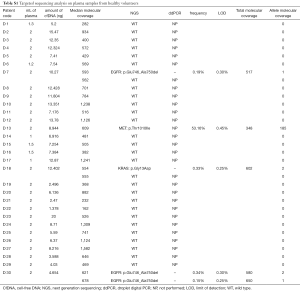
Full table
Targeted sequencing of tumor samples
Tumor samples were analyzed with the Oncomine Solid Tumour DNA kit (Thermo Fisher Scientific, Waltham, MA, USA) covering hotspot variants and actionable mutations of 22 genes involved in colon and lung cancers (Figure S1). Ten ng of gDNA or a maximum volume of 12 µL of extracted gDNA were used as input to prepare libraries according to the manufacturer’s instructions. The amplified libraries were sequenced on the Ion Torrent PGM semiconductor as previously described (16). The data were analyzed using the torrent suite software v5.0 (Thermo Fisher Scientific) and the obtained variants confirmed by the integrative genome viewer (IGV) from the Broad Institute. The limit of mutations detection (LOD) of tissue NGS approach is 2% allelic frequency. The Heterogeneity Score (HS) was calculated by normalizing the VAF for the fraction of neoplastic cells (17).
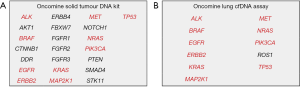
Targeted sequencing of cfDNA
cfDNA was analyzed with the Oncomine™ Lung cfDNA Assay (Thermo Fisher Scientific). The panel consists of a single pool of primers to perform multiplex PCR for sequencing of 35 amplicons across 11 NSCLC-related genes (Figure S1). Twenty nanograms of cfDNA input or a maximum volume of 13 µL per sample were used for libraries construction according to the manufacturer’s instructions. This protocol includes a Tagging Technology for rare mutations detection. Each cfDNA molecule is assigned with unique molecular tags (UMI) by PCR using fusion primers that contain both gene specific and UMI sequences. True hotspot variant can be distinguished from workflow errors counting the frequency of the variants in families that have the same UMI (18). The amplified libraries were quantified with the Ion Library TaqMan Quantitation Kit (Thermo Fisher Scientific). Fifty pM of each library were multiplexed and the pool was loaded on Ion 540 chip using the Ion Chef System (Thermo Fisher Scientific). Sequencing was performed on the Ion S5 XL platform and raw data were analyzed using the Torrent Suite Software v5.6 and the Ion Reporter Software v5.6 (Thermo Fisher Scientific). Three or more families with the same UMI are needed to define a mutation as true, according to company’s recommendations. The LOD of the method correlates with the amount of cfDNA used for library preparation. To obtain 0.1% LOD (1 mutant copy in a background of 1,000 wild-type copies), 20 ng of cfDNA input are required. A different LOD per sample is thus calculated based on the input cfDNA and on the coverage obtained in the region for sample of the mutation. Each variant was verified using the IGV visualization tool (http://www.broadinstitute.org/igv/).
ddPCR analysis
Samples with mutant allele fraction (MAF) close to the lowest sensitivity of NGS and tissue/plasma discordant cases were analyzed using the QX200 Droplet Digital PCR (ddPCR) System (Bio-Rad, Milan, Italy). The EGFR p.L858R Mutation Assay, the KRAS Screening Multiplex kit and the EGFR Exon 19 Deletions Screening Kit (Bio-Rad) were used according to manufacturers’ instructions (see Supplementary).
Results
Targeted sequencing analysis of cfDNA from healthy volunteers
We first analyzed cfDNA from 30 healthy donors in order to develop an optimized workflow of analysis. Plasma from healthy donors usually contains much lower levels of cfDNA as compared with cancer patients and, therefore, these cases are highly challenging. Indeed, in 29/30 cases the cfDNA input for the library preparation step was below the 20 ng recommended by the manufacturer protocol (range, 3 to 20 ng of total cfDNA) (Table S1). The amount of cfDNA extracted from six cases with <2 mL of plasma was similar to the cases with 2 mL of plasma available (Table S1). As expected, the median molecular coverage obtained for each case and the LOD were negatively influenced by the amount of cfDNA used.
Unexpectedly, one MET p.T1010I polymorphism, two EGFR exon 19 deletions and one KRAS p.G13D mutation were detected in cfDNA from 4/30 healthy volunteers (Table S1). Only one EGFR deletion was confirmed in a second independent NGS analysis, although at an allelic frequency below the LOD for the specific mutation, which is calculated according to the quality of the specific sample sequencing run and to the coverage obtained in the region of the mutation. None of the variants in EGFR and KRAS genes were confirmed by ddPCR, thus suggesting that they are sequencing artifacts probably linked to the suboptimal cfDNA input.
In order to define appropriate cutoffs for mutation detection and to avoid potential false positive cases, we developed our own workflow of analysis based on 2 crucial parameters (Figure 1). We defined a variant as true when the allele molecular coverage (number of families with a specific mutation) was ≥3 and the allele frequency of the mutation was higher than the LOD for the specific mutation. We also established that when 1 of the 2 parameters does not comply with the set benchmarks, an independent confirmatory analysis with a highly sensitive approach is needed. When none of these parameters were verified, the variant was defined as a false positive result and thus it was not called. When this workflow of analysis was applied to the sequencing data, only the p.T1010I polymorphism in the MET gene was confirmed.
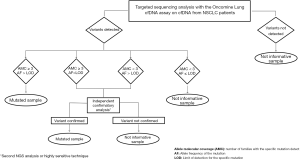
Targeted sequencing analysis of tumor and matched cfDNA samples from mNSCLC patients
Once identified the algorithm and the parameters for data analysis, we performed targeted sequencing analysis of tumor and matched cfDNA samples from a cohort of 107 patients with mNSCLC. Table 1 summarizes the demographic and clinical characteristics of the patients. In most cases (48.6%), a cytological sample was available as tumor material source for genotyping testing. Approximately 90% of patients had a diagnosis of lung adenocarcinoma, while other subtypes were less represented (Table 1).
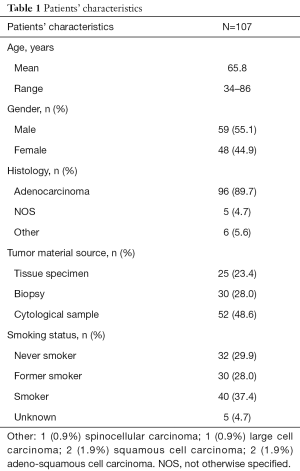
Full table
The cfDNA input for the library preparation was below the recommended amount of 20 ng in 67/107 (62.6%) cases (range of cfDNA: 1.56 to 20 ng; mean 12.3 and median 10.4). Using our workflow of analysis, we identified at least one variant in 64/107 cfDNA samples (59.8%). Tissue testing revealed the presence of at least one variant in the same coding areas covered by the plasma panel in 75/107 corresponding tumor specimens (70.1%). In 30/107 cases no mutation was detected by NGS analysis in both tumor and plasma samples. Two cases showed a mutation in plasma not present in the tumor tissue. The most frequently altered genes in tumor and plasma specimens were EGFR (30.8% vs. 26.2%), KRAS (24.2% vs. 19.6%), TP53 (29.9% vs. 20.6%) and BRAF (3.7% in both tumor and plasma) (Figure 2).
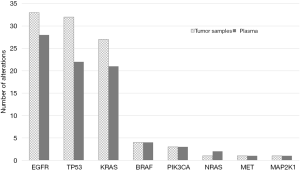
The overall concordance between plasma and matched tumor specimens was relatively low. Only 68/108 (63%) genomic variants identified in tumor specimens were also detected in the matched plasma samples. In addition, 14 variants found in plasma were not detected in the tumor specimens. However, the discordance rate differed importantly among the investigated genes.
EGFR mutations were found in 23/30 plasma samples from patients with EGFR-mutant tumor (sensitivity 76.7%) and in 2/77 plasma samples from EGFR-wild type patients (specificity 97.4%), thus resulting in an acceptable concordance rate of 91.6% (Table S2). Four of the seven false-negative cases (EGFR mutant-tissue/WT-plasma) had an input of cfDNA <20 ng. Interestingly, ddPCR analysis could detect in the cfDNA the expected EGFR mutation only in 1/7 cases at an allelic frequency close to the LOD of the NGS panel (VAF =0.29%) (Table 2). Therefore, the majority of these tumors should be classified as non-shedders.
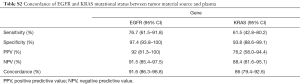
Full table
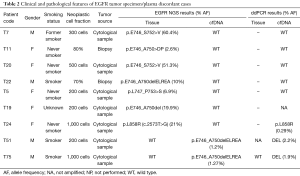
Full table
In the 2 false-positive cases (EGFR WT-tissue/mutant-plasma), ddPCR confirmed the EGFR mutations in both plasma samples but could not detect the mutation in the tissue samples. Importantly, in both cases only a cytology specimen was available for tissue testing.
By contrast, we observed a higher discordance between cfDNA and tissue testing for KRAS variants (Table S2). In particular, 26 tumor samples carried a mutation in KRAS, of which only 16 (sensitivity: 61.5%) were also confirmed in the matched plasma sample. Additional cfDNA material for an independent confirmatory analysis by ddPCR was available for 6 of 10 discordant cases. KRAS mutation was detected by ddPCR in 4 cfDNA samples at VAF ranging between 0.03% and 0.2%, very close or below the LOD of the NGS cfDNA-panel.
Next, we tried to assess whether the difference in sensitivity for EGFR and KRAS mutations could be due to clonal or sub-clonal features of these variants. We previously reported that the HS can provide an approximate estimate of the fraction of neoplastic cells carrying a specific variant (17). Interestingly, the HS for KRAS mutations in the 21 cases with known rate of neoplastic cells ranged between 3.5 and 642, with a mean value of 125 and a median value of 69.3 (Figure 3). Ten out of 21 cases (47.6%) showed HS levels <50, suggesting possible sub-clonal mutations. By contrast, only 15.4% of the EGFR mutant cases had a HS for EGFR mutations <50 (data not shown).
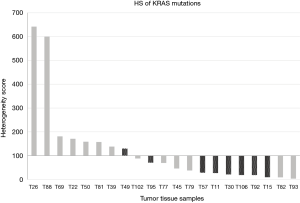
Surprisingly, we observed two EGFR mutant cases (T26 and T27) that carried also KRAS variants in plasma confirmed by ddPCR analysis. We had no available peripheral blood cells from these patients in order to assess the possible origin of these mutations from hematopoietic cells. However, for both cases we had available serial plasma samples collected during treatment with EGFR tyrosine kinase inhibitors (TKIs). A quite constant allelic frequency of the KRAS variants was detected over the time in the cfDNA of both samples, while the levels of the EGFR mutations were significantly affected by TKI treatment (Figure 4). Analysis of tumor tissue from patient T27 did not show any KRAS variant. This patient had a partial response to first-line afatinib that lasted 32 months. Overall, these findings suggest for this case a possible origin of the KRAS variant from clonal hematopoiesis. In contrast, case T26 was found to carry in the tumor tissue the same KRAS variant identified in cfDNA. The allelic frequency of the tumor KRAS variant was significantly higher than the EGFR mutation, which was much more represented in the cfDNA (Figure 4). Interestingly, we observed after five months of treatment a concomitant increase in the EGFR activating p.L858R and resistance p.T790M mutations without any appreciable variation of the levels of the KRAS variant. Patient T26 had stable disease as best response to first-line gefitinib with a PFS of 8 months. At progression, patient T26 was treated with osimertinib. However, the patient died after 4 weeks due to a rapid progression of the disease with massive pleural effusion and decay of general conditions. The short response to first-line gefitinib and the fast progression with lack of response to osimertinib suggest that this tumor indeed carry both KRAS and EGFR mutant clones. Importantly, these data were confirmed by ddPCR analyses that overall showed allelic frequencies of the identified variants similar to NGS testing at the different time points in both cases T26 and T27 (Tables S3,S4). However, in the cfDNA sample obtained at two months of treatment from patient T26, ddPCR detected a p.T790M variant at low allelic frequency not identified by the NGS panel (Table S3).
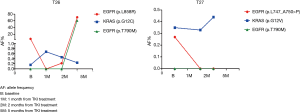
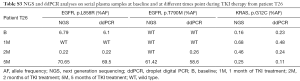
Full table
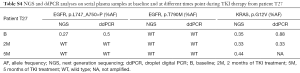
Full table
Five additional cases were negative for KRAS mutations on tissue and mutated on plasma. The plasma-ddPCR analysis confirmed all the KRAS variants identified in cfDNA, thus demonstrating the specificity of the calls, and detected KRAS mutations in 2/5 matched tumor samples at allelic frequencies below the LOD of the NGS tissue panel.
Discussion
The use of NGS-based methods for the analysis of cfDNA is a valuable alternative for tumor molecular profiling for those patients who have not available tissue specimens or whose tissue is not adequate for testing. In addition, in patients with metastatic disease and multiple localizations of the tumor, liquid biopsy might also provide a more comprehensive profiling of the tumor as compared with the analysis of a small fragment of a single tumor site. Finally, the liquid biopsy for its non-invasive nature is the only tool that can be adopted for a molecular monitoring of the disease (19,20). Nevertheless, the high discordance between tissue and liquid biopsy raised concerns on its use in the clinical scenario.
Our findings confirm the high discordance between tissue and cfDNA genetic profiling and highlight the possible involvement of different mechanisms, i.e. technical artifacts, CHIP and tumor heterogeneity.
Technical artifacts of NGS-based testing of cfDNA might depend on different factors. First, the quantity of the cfDNA isolated from plasma samples is usually low. Indeed, in a significant number of cases included in this study the amount of input cfDNA for the NGS test was lower than the recommended quantity. In our experience, the extraction of cfDNA from larger volumes of plasma might solve only in part this issue. In fact, the concentration of cfDNA obtained from 4 mL of plasma was similar to 2 mL in two independent cohorts of 50 mNSCLC patients observed within our clinical practice (data not shown). The low concentration rather than the total amount limits the quantity of cfDNA that can be added in the reaction. In addition, because of the high dilution of tumor-derived DNA in DNA from normal cells, the coverage of NGS analysis of cfDNA is usually high, thus favoring potential sequencing artifacts. A recent study provided a comprehensive analysis of sequencing error sources in conventional NGS workflows (21). Different substitution errors were found to be due to sample handling, library preparation, enrichment PCR, and sequencing. In agreement with these findings, a recent report described a high rate of false positive calls from four vendors using different platforms for cfDNA testing, with vendor’s specific signatures of false positive calls (15). Our approach to sequence a cohort of healthy donors to set an appropriate workflow for data interpretation allowed us to significantly reduce the incidence of sequencing artifacts. Indeed, all the variants identified in mNSCLC patients by using this approach were confirmed by independent ddPCR analysis, thus confirming the specificity of the calls when the optimized workflow is applied. Our data also highlight the importance to use for the validation of NGS tests for cfDNA clinical samples that resemble the complexity of the routine clinical practice rather than reference material, as already recommended for tissue testing with NGS panels (22).
Both CHIP and tumor heterogeneity might lead to discordant tissue-plasma results. CHIP is more frequent in older individuals and might affect a number of genes, including genes that are relevant in the pathogenesis of human solid tumors such as TP53, JAK2 and KRAS (23,24). A recent study from Hu and co-workers showed that most KRAS and JAK2 mutations found in cfDNA but not in tumor tissue of NSCLC patients are likely to derive from CHIP (24). However, another study by Liu et al. (25) did not find any KRAS mutation in cfDNA from healthy donors, while it identified rare EGFR variants in peripheral blood cells. Unfortunately, we had no peripheral blood cells available from patients with EGFR or KRAS plasma-mutant/tissue-wild type. However, at least in some cases we could demonstrate the presence of low levels of the same KRAS variant identified in cfDNA also in the tumor specimens of apparently false positive cases, thus suggesting that tumor heterogeneity contributes to the discordance between tumor and plasma testing. In this respect, it has been demonstrated that lung adenocarcinoma contains on average 4 to 7 different clones, with tumors showing >15 clones (26). In agreement with these findings, heterogeneous driver alterations that occurred later in tumor evolution were found in more than 75% of lung cancer (27), suggesting that analysis of cfDNA might better recapitulate tumor heterogeneity as compared with tissue testing (28). Rare NSCLC cases carrying both EGFR and KRAS variants have been previously described (29). Analysis of serial samples collected during TKIs treatment did not show any kinetics that could allow to distinguish between tumor heterogeneity or CHIP. The lack of clinical response to treatment is a criterion that might aid in the interpretation of similar findings.
We also found 2 cases EGFR plasma-mutant/tissue-wild type. EGFR variants have been reported up to now to be involved in CHIP in a single study (25). In addition, EGFR mutations have been described to be almost always clonal in the seminal paper by Jamal-Hanjani and co-workers (27). Nevertheless, two cases showed a late-clonal EGFR variant in this study. McGranahan et al. (30) also described two NSCLC cases with possible sub-clonal EGFR mutations. We have previously reported two EGFR tissue-wild type NSCLC patients in which EGFR mutations were identified in plasma samples and next confirmed to be present at a very low allelic frequency in the matched tissue samples (16). These data imply that in rare cases EGFR mutations might be late clonal or sub-clonal. However, we must also acknowledge that both discordant cases described in this study had available only cytological material of poor quality for tumor testing, thus raising the possibility of a false negative result on tissue.
Tumor heterogeneity might also in part explain the relatively lower sensitivity for KRAS variant in the cfDNA samples as compared with EGFR mutations. By using the HS classification, we found that KRAS variants were more frequently sub-clonal as compared with EGFR mutations in our cohort. Interestingly, the median HS values were lower among cases with discordant tissue/plasma KRAS mutation status as compared with concordant status (Mann Whitney test P value =0.039). Although we acknowledge that the HS provides only a rough estimate of the clonal nature of a variant, this approach has been previously shown to correlate with outcome in colorectal carcinoma patients (17).
In agreement with previous studies (24), we also found a quite high level of discordance between tissue and plasma testing for TP53 variants, suggesting again the possible contribution of both CHIP and tumor heterogeneity to this phenomenon. The discordance rate for other variants was apparently lower. However, it is difficult to make such comparison due to the significantly lower frequency of variants in genes other than KRAS, TP53 and EGFR in our cohort of patients. Finally, we want to highlight the importance to test germline DNA isolated from peripheral blood cells, when available, in order to assess whether pathogenic variants are due to CHIP, at least for genes frequently mutant in CHIP such as TP53 or JAK2 (24).
Conclusions
In conclusion, technical artifacts of NGS testing in challenging samples with suboptimal cfDNA input can be solved by using appropriate algorithms of analysis of sequencing data. Nevertheless, CHIP and tumor heterogeneity might both affect the concordance between plasma and tumor testing. While the true contribution of CHIP to this phenomenon needs to be further demonstrated, the implications of tumor heterogeneity for treatment of patients with targeted agents require appropriate clinical experimentation.
Supplementary
Droplet digital PCR (ddPCR) analysis
The ddPCR EGFR Exon 19 Deletions Screening Kit is a primer-probe mix able to detect 15 deletions in EGFR Exon 19 (c.2235_2252>AAT, c.2235_2249del15, c.2236_2250del15, c.2238_2252>GCA, c.2238_2255del18, c.2239_2253>CAA, c.2239_2251>C, c.2239_2258>CA, c.2239_2252>CA, c.2239_2256del18, c.2239_2248TTAAGAGAAG>C, c.2239_2253del15, c.2240_2257del18, c.2240_2254del15 and c.2239_2247delTTAAGAGAA, plus wild-type). Other EGFR Exon 19 deletions present in this region may also be detected. The KRAS Screening Multiplex Kit includes a primer-probe mix (9 µM primers and 5 µM of each probe) able to detect 7 mutations (p.Gly12Ala, p.Gly12Cys, p.Gly12Asp, p.Gly12Arg, p.Gly12Ser, p.Gly12Val, p.Gly13Asp) in codon 12 and 13 of the KRAS gene (Bio-Rad, Milan, Italy). ddPCR reaction mixtures contained a final concentration of 250 nM for each of the probes, 450 nM for the forward and reverse primers, 1× ddPCR Supermix for Probes (No dUTP) (Bio-Rad) and 15 ng of genomic DNA or cfDNA, when available, in a final volume of 20 µL. The entire volume of the ddPCR reaction was loaded in appropriate wells of a DG8 cartridge with 70 µL of generator oil (Bio-Rad). Samples are partitioned into approximately 20,000 water-oil emulsion droplets using the QX200 Droplet generator (Bio-Rad). Forty microliters of the water-oil emulsion were used for the ddPCR reaction that was performed with a Veriti Thermal cycler (Thermo Fisher) under the following conditions: 1 cycle of 95 °C for 10 min, 40 cycles of 94 °C for 30 s and 55 °C for 1 min, and 1 cycle of 98 °C for 10 min. After thermal cycling, the plates were transferred to a QX200 Droplet reader. The digital PCR data were analyzed using the QuantaSoft analytical software v1.7.4 (Bio-Rad).
Acknowledgments
We thank Dr. Nicoletta Chicchinelli for technical support. We also thank Drs. Vincenzo Iervolino and Catia Addolorata Di Macchia for their help in collecting the peripheral blood samples from healthy donors.
Funding: This work was supported by grants from the Associazione Italiana per la Ricerca sul Cancro (AIRC; grant number: IG17135).
Footnote
Conflicts of Interest: The authors have no conflicts of interest to declare.
Ethical Statement: The authors are accountable for all aspects of the work in ensuring that questions related to the accuracy or integrity of any part of the work are appropriately investigated and resolved. The use of the data for this study was approved by the Ethical Committee of the Pascale Institute (Protocol n16/14 OSS and 21/14 OSS). All patients provided written informed consent before enrollment in the study.
Data Sharing Statement: No additional data available.
Open Access Statement: This is an Open Access article distributed in accordance with the Creative Commons Attribution-NonCommercial-NoDerivs 4.0 International License (CC BY-NC-ND 4.0), which permits the non-commercial replication and distribution of the article with the strict proviso that no changes or edits are made and the original work is properly cited (including links to both the formal publication through the relevant DOI and the license). See: https://creativecommons.org/licenses/by-nc-nd/4.0/.
References
- Goto K, Ichinose Y, Ohe Y, et al. Epidermal growth factor receptor mutation status in circulating free DNA in serum: from IPASS, a phase III study of gefitinib or carboplatin/paclitaxel in non-small cell lung cancer. J Thorac Oncol 2012;7:115-21. [Crossref] [PubMed]
- Douillard JY, Ostoros G, Cobo M, et al. Gefitinib treatment in EGFR mutated caucasian NSCLC: circulating-free tumor DNA as a surrogate for determination of EGFR status. J Thorac Oncol 2014;9:1345-53. [Crossref] [PubMed]
- Fenizia F, De Luca A, Pasquale R, et al. EGFR mutations in lung cancer: from tissue testing to liquid biopsy. Future Oncol 2015;11:1611-23. [Crossref] [PubMed]
- Douillard JY, Ostoros G, Cobo M, et al. First-line gefitinib in Caucasian EGFR mutation-positive NSCLC patients: a phase-IV, open-label, single-arm study. Br J Cancer 2014;110:55-62. [Crossref] [PubMed]
- Reck M, Hagiwara K, Han B, et al. ctDNA Determination of EGFR Mutation Status in European and Japanese Patients with Advanced NSCLC: The ASSESS Study. J Thorac Oncol 2016;11:1682-9. [Crossref] [PubMed]
- Sacher AG, Paweletz C, Dahlberg SE, et al. Prospective Validation of Rapid Plasma Genotyping for the Detection of EGFR and KRAS Mutations in Advanced Lung Cancer. JAMA Oncol 2016;2:1014-22. [Crossref] [PubMed]
- Mayo-de-Las-Casas C, Jordana-Ariza N, Garzon-Ibanez M, et al. Large scale, prospective screening of EGFR mutations in the blood of advanced NSCLC patients to guide treatment decisions. Ann Oncol 2017;28:2248-55. [Crossref] [PubMed]
- Mok T, Wu YL, Lee JS, et al. Detection and Dynamic Changes of EGFR Mutations from Circulating Tumor DNA as a Predictor of Survival Outcomes in NSCLC Patients Treated with First-line Intercalated Erlotinib and Chemotherapy. Clin Cancer Res 2015;21:3196-203. [Crossref] [PubMed]
- Oxnard GR, Hu Y, Mileham KF, et al. Assessment of Resistance Mechanisms and Clinical Implications in Patients With EGFR T790M-Positive Lung Cancer and Acquired Resistance to Osimertinib. JAMA Oncol 2018;4:1527-34. [Crossref] [PubMed]
- Tsao AS, Scagliotti GV, Bunn PA Jr, et al. Scientific Advances in Lung Cancer 2015. J Thorac Oncol 2016;11:613-38. [Crossref] [PubMed]
- Aggarwal C, Thompson JC, Black TA, et al. Clinical Implications of Plasma-Based Genotyping With the Delivery of Personalized Therapy in Metastatic Non-Small Cell Lung Cancer. JAMA Oncol 2019;5:173-80. [Crossref] [PubMed]
- Remon JL, Jovelet L, Caramella C, et al. Real-World Utility of an Amplicon-Based Next-Generation Sequencing Liquid Biopsy for Broad Molecular Profiling in Patients With Advanced Non–Small-Cell Lung Cancer. JCO Precis Oncol 2019;3:1-14.
- Chae YK, Davis AA, Carneiro BA, et al. Concordance between genomic alterations assessed by next-generation sequencing in tumor tissue or circulating cell-free DNA. Oncotarget 2016;7:65364-73. [Crossref] [PubMed]
- Keppens C, Dequeker EMC, Patton SJ, et al. International pilot external quality assessment scheme for analysis and reporting of circulating tumour DNA. BMC Cancer 2018;18:804. [Crossref] [PubMed]
- Stetson D, Ahmed A, Xu X, et al. Orthogonal Comparison of Four Plasma NGS Tests With Tumor Suggests Technical Factors are a Major Source of Assay Discordance. JCO Precis Oncol 2019;3:1-9.
- Rachiglio AM, Esposito Abate R, Sacco A, et al. Limits and potential of targeted sequencing analysis of liquid biopsy in patients with lung and colon carcinoma. Oncotarget 2016;7:66595-605. [Crossref] [PubMed]
- Normanno N, Rachiglio AM, Lambiase M, et al. Heterogeneity of KRAS, NRAS, BRAF and PIK3CA mutations in metastatic colorectal cancer and potential effects on therapy in the CAPRI GOIM trial. Ann Oncol 2015;26:1710-4. [Crossref] [PubMed]
- Li BT, Janku F, Jung B, et al. Ultra-deep next-generation sequencing of plasma cell-free DNA in patients with advanced lung cancers: results from the Actionable Genome Consortium. Ann Oncol 2019;30:597-603. [Crossref] [PubMed]
- Siravegna G, Marsoni S, Siena S, et al. Integrating liquid biopsies into the management of cancer. Nat Rev Clin Oncol 2017;14:531-48. [Crossref] [PubMed]
- Wang J, Chang S, Li G, et al. Application of liquid biopsy in precision medicine: opportunities and challenges. Front Med 2017;11:522-7. [Crossref] [PubMed]
- Ma X, Shao Y, Tian L, et al. Analysis of error profiles in deep next-generation sequencing data. Genome Biol 2019;20:50. [Crossref] [PubMed]
- Deans ZC, Costa JL, Cree I, et al. Integration of next-generation sequencing in clinical diagnostic molecular pathology laboratories for analysis of solid tumours; an expert opinion on behalf of IQN Path ASBL. Virchows Arch 2017;470:5-20. [Crossref] [PubMed]
- Genovese G, Kahler AK, Handsaker RE, et al. Clonal hematopoiesis and blood-cancer risk inferred from blood DNA sequence. N Engl J Med 2014;371:2477-87. [Crossref] [PubMed]
- Hu Y, Ulrich BC, Supplee J, et al. False-Positive Plasma Genotyping Due to Clonal Hematopoiesis. Clin Cancer Res 2018;24:4437-43. [Crossref] [PubMed]
- Liu J, Chen X, Wang J, et al. Biological background of the genomic variations of cf-DNA in healthy individuals. Ann Oncol 2019;30:464-70. [Crossref] [PubMed]
- Andor N, Graham TA, Jansen M, et al. Pan-cancer analysis of the extent and consequences of intratumor heterogeneity. Nat Med 2016;22:105-13. [Crossref] [PubMed]
- Jamal-Hanjani M, Wilson GA, McGranahan N, et al. Tracking the Evolution of Non-Small-Cell Lung Cancer. N Engl J Med 2017;376:2109-21. [Crossref] [PubMed]
- Abbosh C, Birkbak NJ, Wilson GA, et al. Phylogenetic ctDNA analysis depicts early-stage lung cancer evolution. Nature 2017;545:446-51. [Crossref] [PubMed]
- Rachiglio AM, Fenizia F, Piccirillo MC, et al. The Presence of Concomitant Mutations Affects the Activity of EGFR Tyrosine Kinase Inhibitors in EGFR-Mutant Non-Small Cell Lung Cancer (NSCLC) Patients. Cancers (Basel) 2019. [Crossref] [PubMed]
- McGranahan N, Favero F, de Bruin EC, et al. Clonal status of actionable driver events and the timing of mutational processes in cancer evolution. Sci Transl Med 2015;7:283ra54. [Crossref] [PubMed]