Tumor mutation burden derived from small next generation sequencing targeted gene panel as an initial screening method
Introduction
Solid tumors with high tumor mutation burden (TMB), including melanoma and non-small cell lung cancer (NSCLC), demonstrate remarkable responses to immune checkpoint inhibitors (1-4). It has been hypothesized that tumors with high TMB are more likely to harbor tumor-specific antigenic peptides or neoantigens, which make them targets of activated immune cells and results in a positive response with immunotherapy (1,5).
Neoantigen load, although not clinically observable, has been shown to be associated with the burden of mutation and immunotherapy response (2,6). TMB was therefore considered an emerging predictive biomarker for the effectiveness of inhibitors of the immune checkpoint (1-3,6). The number of mutations present in a tumor sample is estimated to be TMB or mutation load. It is measured as the number of somatic mutations per megabase (Mb) of the interrogated genome, including single nucleotide and nonsense variants and short insertion-deletion variations.
Whole exome sequencing (WES), spanning about 30 to 50 Mb of coding sequences, has been traditionally used to estimate TMB (3). Advanced NSCLC patients with high TMB estimated by WES who received single-agent or combination immune checkpoint inhibitors were more likely to have improved objective response, more durable clinical benefit and longer progression-free survival than patients with low TMB (2,7,8). In contrast to WES, targeted next-generation sequencing (NGS) with gene panels consisting of 300 to 500 genes, spanning about 1 to 3 Mb of the genome, can accurately sequence genes or regions of interest at a much higher depth to reveal therapeutically-relevant mutations (9,10). The high correlation of TMB derived from WES and large targeted gene panels has then prompted the replacement of WES in the routine clinical assessment of TMB (3,4,11,12); however, its use is still limited by high cost and longer turnaround time. Moreover, despite providing a comprehensive mutation profile, information gathered from sequencing with large targeted panels is not needed in the therapeutic decisions for some patients.
Conversely, smaller targeted gene panels have been increasingly utilized in clinical practice, particularly in lung cancer, due to its cost-effectiveness and faster turnaround time as compared with the use of large targeted gene panels and WES (13). Like large gene panels, targeted sequencing using small gene panel can also simultaneously find genomic alterations in cancer-related genes but only in a limited number of genes. With the more common use of small targeted gene panel, we hypothesized that predicting TMB from it can be clinically useful as a first and cost-effective screening method. In this study, we explored the feasibility of using a 56-gene panel covering 0.26 Mb of the human genome to predict TMB.
To reach this goal, we first simulated the target regions of the small gene panel using sequencing data obtained with the large gene panel and derived an optimal TMB cut-off using a training dataset. We then confirmed the utility of this cut-off using an independent cohort sequenced using the small panel and further confirmed with the large panel.
Methods
Patients
Data derived from targeted sequencing using 520-gene panel (OncoScreen Plus, Burning Rock Biotech, China) from a total of 406 NSCLC patients with varied TMB status were used as the training dataset to identify the genes correlated with high TMB, and derive the optimal TMB cut-off through simulations.
An independent cohort comprised of 30 NSCLC patients with various stages and histological types from our institution who were referred for comprehensive molecular testing at Burning Rock Biotech [College of American Pathologists (CAP)-accredited/Clinical Laboratory Improvement Amendments (CLIA)-certified laboratory] between February 2017 and May 2018 were included for the validation stage of this study. Two independent pathologic examinations confirmed tumor histology. Tumors were staged according to the American Joint Committee on Cancer 7th edition TNM staging system of NSCLC (14).
This study was approved by the relevant Institutional Review Board of the West China Hospital and performed following the ethical standards of West China Hospital and/or national research committee and with the 1964 Helsinki declaration and its later amendments or comparable ethical standard. Prior written informed consent was obtained from each of the recruited patients for the use of their plasma and/or tissue samples in further molecular studies.
Tissue DNA isolation and capture-based targeted DNA sequencing
Tissue DNA was extracted from formalin-fixed, paraffin-embedded (FFPE) tumor tissues using QIAamp DNA FFPE tissue kit (Qiagen). A minimum of 50 ng of DNA is required for NGS library construction. Tissue DNA was sheared using Covaris M220 (Covaris, MA, USA), followed by end repair, phosphorylation, and adaptor ligation. Fragments between 200 to 400 base pairs from the sheared tissue DNA were purified (Agencourt AMPure XP Kit, Beckman Coulter, CA, USA), followed by hybridization with capture probes baits, hybrid selection with magnetic beads and PCR amplification. The quality and the size of the fragments were assessed using Qubit 2.0 Fluorimeter with the dsDNA high-sensitivity assay kit (Life Technologies, Carlsbad, CA). Indexed samples were sequenced on Nextseq500 (Illumina, Inc., USA) with paired end reads and average sequencing depth of 1,000×.
Sequence data analysis
Sequence data were mapped to the reference human genome (hg19) using Burrows-Wheeler Aligner v.0.7.10 (15). Local alignment optimization and variant calling were performed using Genome Analysis Tool Kit v.3.2 (16), and VarScan v.2.4.3 (17). Variants were filtered using the VarScan fpfilter pipeline, loci with depth less than 100 were filtered out. Base-calling in tissue samples required at least 8 and 5 supporting reads for single nucleotide variations (SNVs) and short insertion and deletion variations (INDEL), respectively. Variants with population frequency over 0.1% in the ExAC, 1000 Genomes, dbSNP, or ESP6500SI-V2 databases were grouped as single nucleotide polymorphisms and excluded from further analysis. Remaining variants were annotated with ANNOVAR (18) and SnpEff v.3.6 (19). Analysis of DNA translocation was performed using Factera v.1.4.3 (20).
TMB per patient was computed as a ratio between the total number of mutations detected with the total coding region size of the panel used (i.e., 520-gene OncoScreen Plus panel with 1.26 Mb, 56-gene LungCore panel with 0.25 Mb) using the formula in Eq. [1]. Copy number variations (CNV), fusions, large genomic rearrangements and mutations occurring on the kinase domain of EGFR and ALK were excluded from the mutation count.
| [1] |
Model construction
Various learning methods, including support vector machine (SVM) (21), naive Bayesian, Bayesian network, logistic regression, additive logistic regression (22), random forest, and a multiclass probabilistic classifier were executed to construct the most optimal model in predicting TMB from the small 56-gene panel. These learning algorithms were implemented by WEKA v3.6 (23), with the corresponding packages, including LibSVM, NaïveBayes, BayesNet, Logistic, LogitBoost, RandomForest, MultiClassClassifier, and SVM. Default parameters were obeyed throughout the supervised learning process.
Model performance validation
The sensitivity (Eq. [2]), specificity (Eq. [3]), positive predictive value (PPV) (Eq. [4]) and Matthew’s correlation coefficient (MCC) (Eq. [5]) were calculated accordingly using the formula listed below considering the highest proportion of true positives and the lowest number of false negatives. Sensitivity is defined as the percentage of positive data correctly predicted. Specificity is defined as the percentage of negative data correctly predicted. PPV is defined as the percentage of positive results that are a true positive. MCC is a comprehensive indicator that considers both positive and negative data.
Where TN, TP, FN, FP represent true negative, true positive, false negative, and false positive, respectively.
| [2] |
| [3] |
| [4] |
| [5] |
Statistical analysis
All the data were analyzed using the R statistics package (R v3.4.0; R: The R-Project for Statistical Computing, Vienna, Austria). Differences in the groups were calculated and presented using either Fisher’s exact test or two-tailed Student’s t-test, as appropriate. P value with P<0.05 was considered as statistically significant.
Results
Patient characteristics
A total of 406 NSCLC patients of varied histology and disease stage were included in the training dataset. Among the 406 patients, 62.6% (254/406) of the patients were male, while the remaining 37.4% (152/406) were females. The median age of the cohort was 60 years, ranging from 43 to 88 years. Most of the patients were diagnosed with lung adenocarcinoma (61.8%, 251/406), while 12.3% (50/406) were diagnosed with squamous cell lung carcinoma. Based on the TMB estimated from the large 520-gene panel, 7.9% (32/406) of the patients have TMB ≥20 mutations/Mb, 17.2% (70/406) have TMB between 10–20 mutations/Mb and 75.4% (306/406) had TMB <10 mutations/Mb.
Among the 30 patients included as an independent cohort for the validation stage of the study, a majority were males (86.7%, 26/30). The median age of the validation cohort was 61 years, ranging from 31 to 79 years. About half of the patients (56.7%, 17/30) were diagnosed with lung adenocarcinoma, 40.0% (12/30) were diagnosed with squamous cell lung carcinoma, and a patient was diagnosed with large cell neuroendocrine lung carcinoma. The cohort was comprised of 30% (9/30) patients with early-stage disease, 53.3% (16/30) patients with advanced-stage disease and the remaining 5 patients had unknown disease stage. Early-stage patients included 4 patients with stage IB, 2 patients each with stage IIA and IIB, respectively and 1 patient with stage IIIA. Advanced-stage patients included 4 patients with stage IIIB, 1 patient with stage IIIC, 10 patients with stage IVA, and 1 patient with stage IVB.
Identifying the optimal cut-off for the small panel
In order to investigate the use of the small 56-gene panel to estimate TMB, by using data simulation, we first extracted the target regions covered by the small gene panel from the sequencing data obtained using the large gene panel for the training cohort composed of 406 NSCLC patients. TMB for both large and small panel is similarly calculated as the ratio of the number of SNVs per coding region of the genome covered by the gene panel as expressed in Eq.1 in the Methods section. We then analyzed the correlation of actual TMB calculated from the large gene panel and the simulated TMB for the small gene panel. Furthermore, we also derived an optimal TMB cut-off that could effectively identify patients with low and high TMB.
The large targeted gene panel (OncoScreen Plus, Burning Rock Biotech, China) includes 520 cancer development-related genes spanning 1.64 Mb of the human genome (1.26 Mb excluding the regions not included in TMB estimation). Previous validation of the 520-gene panel revealed high correlation between actual TMB estimated from the WES data of 8,092 patient samples with 35 types of cancers from the Cancer Genome Atlas (TCGA) and the simulated TMB from target regions included in the 520-gene panel derived from the WES data (Pearson correlation coefficient R2=0.976; data not shown). In contrast, the small targeted gene panel (LungCore, Burning Rock Biotech, China) includes 56 lung cancer-related genes spanning 0.28 Mb of the human genome (0.25 Mb excluding the regions not included in TMB estimation). The fitted curve illustrating the correlation between the TMB estimated from the large gene panel and the small gene panel is shown in Figure 1A. The TMB estimated from small gene panel, and large gene panel is highly correlated, with a regression coefficient (R2) of 0.821 and a Pearson correlation coefficient of 0.906. The genes included in the large and small gene panels are listed in Tables S1 and S2, respectively.
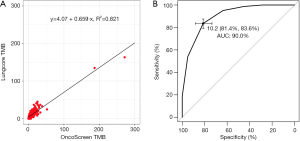
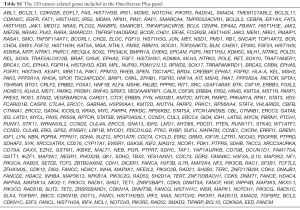
Full table

Full table
Based on the TMB cut-off of 10 mutations/Mb for large gene panel, we divided the data for the small gene panel into TMB ≥10 and TMB <10. According to the receiver operating characteristic (ROC) curve illustrated in Figure 1B, the most optimal cut-off was 10.2 mutations/Mb for the small panel achieving a specificity of 81.4%, sensitivity of 83.6%, and area under the curve (AUC) of 90.0%. Hence, we have set the cut-off as 10 mutations/Mb.
Statistical performance indicators for TMB estimation using the small gene panel
After identifying the optimal TMB cut-off that could distinguish between low and high TMB, we next determined the impact of the TMB cut-off value of 10 mutations/Mb on the performance of TMB estimation using the small panel by calculating statistical performance indicators including sensitivity, specificity and PPV. Furthermore, seven different machine learning algorithms were employed to analyze the performance of the small gene panel in distinguishing and stratifying the TMB using 10 mutations/Mb as the cut-off.
Table 1 summarizes the statistical indicators for the performance of the small gene panel in estimating TMB. At a TMB cut-off of 10 mutations/Mb, the specificity and PPV were 83.6% and 62.4%, respectively. Both the specificity and PPV had an increasing trend with the increase in TMB achieving 100% when the TMB was at 21 mutations/Mb (Figure S1, Table 1). Meanwhile, the sensitivity had an opposite trend, with the highest sensitivity of 81.4% achieved at 10 mutations/Mb cut-off and concomitantly decreased with the increase in TMB (Table 1). The cut-off was confirmed as 10 mutations/Mb considering the highest proportion of true positives and the lowest number of false negatives.
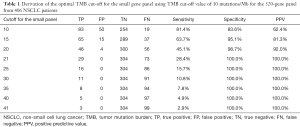
Full table
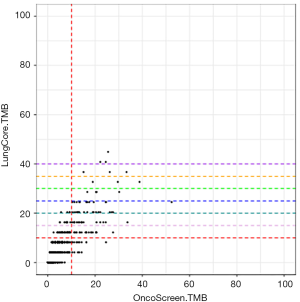
Further analysis of the analytical performance with seven different learning algorithms using a TMB cut-off of 10 mutations/Mb achieved specificity between 92.1% and 96.4% (Table 2). Among the algorithms, BayesNet revealed the highest sensitivity of 70.6%, followed by the sensitivity of 67.6% from both Logistic and MultiClassClassifier. Meanwhile, Logistic revealed the highest sensitivity, PPV and MCC of the model achieving 96.4%, 85.9%, and 68.3%, respectively. Moreover, specificity, PPV and MCC of 95.1%, 82.1%, and 67.1% were respectively achieved by both Logistic and MultiClassClassifier (Table 2).
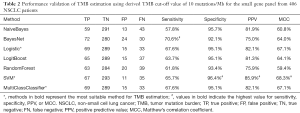
Full table
The inverse relationship between the sensitivity and TMB cut-off strongly supports the fact that small targeted gene panels are not dependable for the estimation of high TMB. In contrast, the increasing trend in both specificity and PPV with the corresponding increase in TMB demonstrated that TMB is likely to be overestimated by the small gene panel, but the likelihood of false-positive results is very low. Since TMB is overestimated, samples with TMB values above the cut-off (>10 mutations/Mb) requires revalidation with a larger gene panel, while samples with TMB values below the cut-off (<10 mutations/Mb) are more likely to be accurate and does not need further validation.
Identifying known genes and alterations associated with increased TMB
We further identified the specific genes associated with high TMB within the large 520-gene panel by performing statistical analysis on the sequencing data from 406 NSCLC patients with varied TMB status. This step aims to identify the genes that are associated with high TMB from the large gene panel and determine if they are present in the small gene panel.
At a TMB cut-off of 10 mutations/Mb, patients with high TMB had significantly more mutations in a total of 106 genes than patients with low TMB. Of these, 26 of these genes were part of the small gene panel and covered 46.4% (26/56) of the genes in the small panel (Table S3).
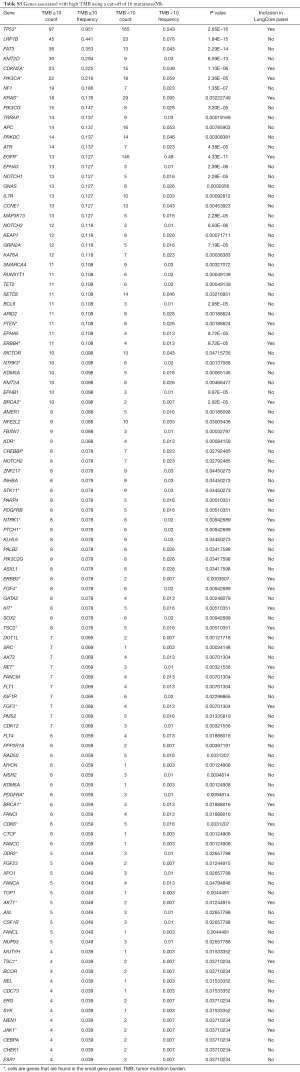
Full table
Interestingly, TP53 mutations were the most predominant mutation among patients with high TMB (P<0.001, Table S3, Figure S2). Among all the other actionable mutations, ALK and ROS1 fusions were also more likely to be detected among patients with low TMB (ALK fusion P=0.0095; ROS1 fusion P=0.043).
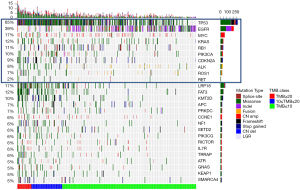
Validation of TMB estimation with small gene panel using an independent cohort
After identifying the optimal cut-off and establishing the feasibility of TMB estimation with the small gene panel using simulated data from the training cohort, we next aimed to validate our findings with the use of an independent cohort consisting of an additional 30 NSCLC patients. This cohort was sequenced using both the small and the large gene panels to compare the TMB estimated from both panels. Furthermore, the statistical performance of the small gene panel was also evaluated with learning algorithms.
The mutation detection rate of TP53 was 67%, with 91.7% (11/12) of the patients having TMB ≥20 mutations/Mb, 72.7% (8/11) having TMB between 10 to 20 mutations/Mb and 14.3% (1/7) having TMB <10 mutations/Mb (Figure S3).
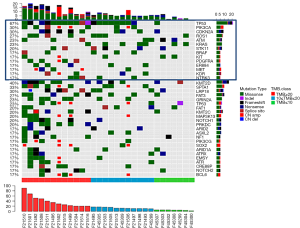
Table 3 lists the TMB estimated with the small (LungCore) and further validated with the large (OncoScreen Plus) gene panel for each of the 30 patients. Most of the patients (66.7%, 20/30) had 5 or more mutations detected with an estimated TMB of ≥20 mutations/Mb. Four patients had TMB between 10–20 mutations/Mb, while the remaining 6 patients had TMB <10 mutations/Mb. In contrast, based on the TMB validated with the 520-gene panel, 40.0% (12/30) of the patients had TMB ≥20 mutations/Mb, 36.7% (11/30) had TMB between 10–20 mutations/Mb and the remaining 23.3% (7/30) had TMB <10 mutations/Mb.
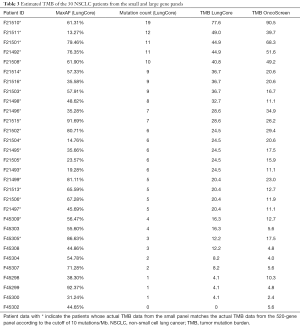
Full table
The analytical performance of the small gene panel in TMB estimation using a cut-off value of 10 mutations/Mb achieved specificity of 71.4%, PPV of 91.7%, and sensitivity of 95.7% (Table 4, Figure S4). Consistent with the trend observed in the training dataset (Table 1), the specificity and PPV also had an increasing trend, with both specificity and PPV reaching 100% at a TMB of 20 mutations/Mb. Meanwhile, the sensitivity also had a decreasing trend with the concomitant increase in the TMB in the validation cohort (Table 4). Consistently, the analytical performance evaluated by the seven different learning algorithms revealed the sensitivity of 91.3%, specificity, and PPV of 100% and MCC of 84.3% in both LogitBoost and SVM models (Table 5).
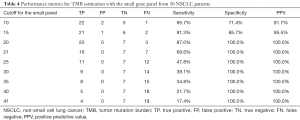
Full table
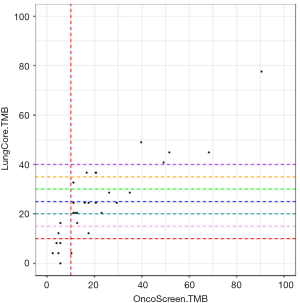
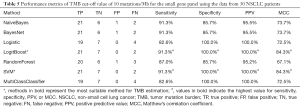
Full table
These data taken together indicate that the cut-off of 10 mutations/Mb estimated from the small 56-gene panel could reliably stratify patients with low (<10) and high (≥10) TMB. Furthermore, by being overestimated, the TMB of the patients having low TMB were accurately estimated by the small panel, while the patients with high TMB required further validation with larger targeted gene panel.
Discussion
To the best of our knowledge, this is the first study to evaluate the utility of a small 56-gene panel to derive TMB as a first screening method. Our results suggest that at a cut-off of 10 mutations/Mb, TMB derived from the small 56-gene panel can reliably identify the subset of patients with low TMB (<10 mutations/Mb). Also, the results were able to identify the individuals who would likely not benefit from TMB estimation using large targeted gene panel and the subset of patients with high TMB (≥10 mutations/Mb). Additionally, it was able to identify those who require further evaluation with large gene panel. Since smaller gene panels, such as the 56-gene panel analyzed in our study, are the more frequently used targeted panel in clinical practice, the inclusion of this TMB estimation into the clinical reports can provide a more meaningful contribution for making a timely treatment decision for lung cancer patients.
Previous studies have published that there is a highly imprecise estimate of TMB from small gene panels covering approximately 0.5 Mb of the human genome (10). With the inclusion of about a third of the genes more likely associated with high TMB within the small LungCore panel (46.4%, 26/56), despite being limited, TMB estimation with the small panel still has the potential to be informative and clinically relevant. Using both simulated and actual TMB from the small panel, performance validation consistently revealed the same trend of increasing specificity and PPV, and decreasing sensitivity with a concomitant increase in TMB, strongly suggesting the likelihood of overestimating TMB with the small panel. Consistent with the results from the simulated data, the actual TMB estimated from both the small panel and the 520-gene panel in 30 patients in the independent validation cohort proved this concept. With a cut-off of 10 mutations/Mb, all of the patients with TMB ≥10 mutations/Mb (22/22) estimated by the 520-gene panel similarly had TMB ≥10 mutations/Mb from the small panel; however, only 91.7% (22/24) of the patients with TMB ≥10 mutations/Mb from the small panel had TMB ≥10 mutations/Mb estimated by the 520-gene panel. This data strongly shows the overestimation of TMB with the small gene panel, thus requiring further validation with a larger gene panel for the correct TMB estimation.
On the contrary, all the patients (6/6) with TMB <10 mutations/Mb estimated from the small panel consistently had TMB <10 mutations/Mb estimated by the 520-gene panel, indicating that estimation of low TMB is very accurate with no false-negative calls. These data further suggest that the TMB cut-off of 10 mutations/Mb from the small panel can accurately stratify the patients with low TMB who would not likely benefit from immunotherapy and does not require sequencing with a large gene panel. In addition, 5 of the patients who have more than 10 mutations detected and have estimated TMB of >40 mutations/Mb with the small gene panel consistently had TMB between 39.7 to 90.5 mutations/Mb from the 520-gene panel. These findings indicate that TMB estimation from the small gene panel, although not entirely accurate, can still serve as a valuable reference.
The increased use of immune checkpoint inhibitor therapy in advanced NSCLC patients whose tumors do not harbor actionable EGFR or ALK mutations have driven the need to establish a biomarker in predicting therapeutic benefit. TMB, although still controversial, has now been adopted as a predictive biomarker for immunotherapy response. Traditionally, TMB was assessed using WES until data simulation studies have demonstrated the feasibility of using targeted NGS with gene panels consisting of 300 to 500 genes (3,4,11). Several reports have since proven the utility of large targeted gene panels in accurately predicting TMB (3,4,8,10,11). Although large targeted gene panels providing a more comprehensive mutational profile of solid tumors, they are still substantially limited by their high cost and longer turnaround time. Recent reports have demonstrated that smaller targeted gene panels interrogating about 150 genes from blood samples were sufficient for estimating TMB.
Moreover, TMB estimated from the 150-gene panel were correlated with immune checkpoint inhibitor response in Chinese NSCLC patients, with patients having blood TMB (bTMB) of more than 6 mutations/Mb, considered as high bTMB, correlated with longer progression-free survival than those with low bTMB (P=0.001) (24). By providing a more concise but informative mutation profile, small targeted panels can serve as practical alternatives to large panels in clinical practice. Thus, the inclusion of TMB estimation into the analysis pipeline and clinical reports for small targeted gene panel could extend its utility as an initial TMB screening method, which can easily be integrated into the hospital-based diagnostic sequencing laboratories. Generally, NSCLC patients with actionable mutations benefit most from using small gene panels by revealing actionable mutations and allowing them prompt access to targeted drugs. On the other hand, patients with no actionable mutations revealed by testing with the small gene panel would either be treated with cytotoxic chemotherapy regimen or be recommended to undergo further molecular testing to explore other treatment options. By including TMB estimation in the small gene panel workflow, it extends the utility of small gene panels, particularly in patients with no actionable mutations, and ensures the timely treatment decisions for the patients that do not need further TMB validation with the larger gene panel.
Since our study is a proof of concept study in the utility of small gene panel in TMB prediction, we believe that the use of patient samples with various histologies and disease stage until mutations were no longer detected, and TMB could not be estimated, does not affect the study results.
Despite the limited number of patients and the inclusion of patients with various disease stage and histologies in both the training and validation cohorts, our findings demonstrate the reliability of the small targeted gene panel as an initial TMB screening method to distinguish the subset of patients with low TMB who will not benefit from sequencing with a large panel, thus providing a cost-effective and convenient screening method that provides meaningful application in clinical practice. Our study is also limited by the lack of clinical outcomes from the patients. Prospective studies with a larger cohort are needed to validate the predictive value of data from small gene panel as well as the clinical outcome.
Conclusions
Our study demonstrated that a small targeted gene panel could provide additional information on the TMB of patients, albeit limited, indicating its potential as a cost-effective and convenient screening method and adding to its utility in the timely diagnosis and management of lung cancer patients.
Acknowledgments
The authors thank all the patients and their families. We also thank the investigators, study coordinators, operation staff, and the whole project team who worked on this study.
Funding: This work was supported by Sichuan Provincial Research Foundation for Basic Research (grant number 2018SZ0023 to M Huang).
Footnote
Conflicts of Interest: A Lizaso and T Hou are employees of Burning Rock Biotech. The other authors have no conflicts of interest to declare.
Ethical Statement: The authors are accountable for all aspects of the work in ensuring that questions related to the accuracy or integrity of any part of the work are appropriately investigated and resolved. This study was approved by the relevant Institutional Review Board of the West China Hospital. Prior written informed consent was obtained from each of the recruited patients.
Data Sharing Statement: No additional data available.
Open Access Statement: This is an Open Access article distributed in accordance with the Creative Commons Attribution-NonCommercial-NoDerivs 4.0 International License (CC BY-NC-ND 4.0), which permits the non-commercial replication and distribution of the article with the strict proviso that no changes or edits are made and the original work is properly cited (including links to both the formal publication through the relevant DOI and the license). See: https://creativecommons.org/licenses/by-nc-nd/4.0/.
References
- Snyder A, Makarov V, Merghoub T, et al. Genetic basis for clinical response to CTLA-4 blockade in melanoma. N Engl J Med 2014;371:2189-99. [Crossref] [PubMed]
- Rizvi NA, Hellmann MD, Snyder A, et al. Cancer immunology. Mutational landscape determines sensitivity to PD-1 blockade in non-small cell lung cancer. Science 2015;348:124-8. [Crossref] [PubMed]
- Goodman AM, Kato S, Bazhenova L, et al. Tumor Mutational Burden as an Independent Predictor of Response to Immunotherapy in Diverse Cancers. Mol Cancer Ther 2017;16:2598-608. [Crossref] [PubMed]
- Tomasini P, Greillier L. Targeted next-generation sequencing to assess tumor mutation burden: ready for prime-time in non-small cell lung cancer? Transl Lung Cancer Res 2019;8:S323-6. [Crossref] [PubMed]
- Schumacher TN, Schreiber RD. Neoantigens in cancer immunotherapy. Science 2015;348:69-74. [Crossref] [PubMed]
- Miller A, Asmann Y, Cattaneo L, et al. High somatic mutation and neoantigen burden are correlated with decreased progression-free survival in multiple myeloma. Blood Cancer J 2017;7:e612. [Crossref] [PubMed]
- Zarogoulidis P, Papadopoulos V, Maragouli E, et al. Nivolumab as first-line treatment in non-small cell lung cancer patients-key factors: tumor mutation burden and PD-L1 ≥50. Transl Lung Cancer Res 2018;7:S28-30. [Crossref] [PubMed]
- Hellmann MD, Nathanson T, Rizvi H, et al. Genomic Features of Response to Combination Immunotherapy in Patients with Advanced Non-Small-Cell Lung Cancer. Cancer Cell 2018;33:843-52.e4. [Crossref] [PubMed]
- Frampton GM, Fichtenholtz A, Otto GA, et al. Development and validation of a clinical cancer genomic profiling test based on massively parallel DNA sequencing. Nat Biotechnol 2013;31:1023-31. [Crossref] [PubMed]
- Buchhalter I, Rempel E, Endris V, et al. Size matters: Dissecting key parameters for panel-based tumor mutational burden analysis. Int J Cancer 2019;144:848-58. [Crossref] [PubMed]
- Campesato LF, Barroso-Sousa R, Jimenez L, et al. Comprehensive cancer-gene panels can be used to estimate mutational load and predict clinical benefit to PD-1 blockade in clinical practice. Oncotarget 2015;6:34221-7. [Crossref] [PubMed]
- Chalmers ZR, Connelly CF, Fabrizio D, et al. Analysis of 100,000 human cancer genomes reveals the landscape of tumor mutational burden. Genome Med 2017;9:34. [Crossref] [PubMed]
- Endris V, Penzel R, Warth A, et al. Molecular diagnostic profiling of lung cancer specimens with a semiconductor-based massive parallel sequencing approach: feasibility, costs, and performance compared with conventional sequencing. J Mol Diagn 2013;15:765-75. [Crossref] [PubMed]
- Goldstraw P, Chansky K, Crowley J, et al. The IASLC Lung Cancer Staging Project: Proposals for Revision of the TNM Stage Groupings in the Forthcoming (Eighth) Edition of the TNM Classification for Lung Cancer. J Thorac Oncol 2016;11:39-51. [Crossref] [PubMed]
- Li H, Durbin R. Fast and accurate short read alignment with Burrows-Wheeler transform. Bioinformatics 2009;25:1754-60. [Crossref] [PubMed]
- McKenna A, Hanna M, Banks E, et al. The Genome Analysis Toolkit: a MapReduce framework for analyzing next-generation DNA sequencing data. Genome Res 2010;20:1297-303. [Crossref] [PubMed]
- Koboldt DC, Zhang Q, Larson DE, et al. VarScan 2: somatic mutation and copy number alteration discovery in cancer by exome sequencing. Genome Res 2012;22:568-76. [Crossref] [PubMed]
- Wang K, Li M, Hakonarson H. ANNOVAR: functional annotation of genetic variants from high-throughput sequencing data. Nucleic acids research 2010;38:e164-e.
- Cingolani P, Platts A. A program for annotating and predicting the effects of single nucleotide polymorphisms, SnpEff: SNPs in the genome of Drosophila melanogaster strain w1118; iso-2; iso-3. Fly (Austin) 2012;6:80-92. [Crossref] [PubMed]
- Newman AM, Bratman SV, Stehr H, et al. FACTERA: a practical method for the discovery of genomic rearrangements at breakpoint resolution. Bioinformatics 2014;30:3390-3. [Crossref] [PubMed]
- Pirooznia M, Deng Y. SVM Classifier - a comprehensive java interface for support vector machine classification of microarray data. BMC Bioinformatics 2006;7 Suppl 4:S25. [Crossref] [PubMed]
- Friedman J, Hastie T, Tibshirani R. Additive logistic regression: a statistical view of boosting (With discussion and a rejoinder by the authors). The Annals of Statistics 2000;28:337-407. [Crossref]
- Frank E, Hall M, Trigg L, et al. Data mining in bioinformatics using Weka. Bioinformatics 2004;20:2479-81. [Crossref] [PubMed]
- Wang Z, Duan J, Cai S, et al. Assessment of Blood Tumor Mutational Burden as a Potential Biomarker for Immunotherapy in Patients With Non-Small Cell Lung Cancer With Use of a Next-Generation Sequencing Cancer Gene Panel. JAMA Oncol 2019;5:696-702. [Crossref] [PubMed]