Heterogeneity of neoantigen landscape between primary lesions and their matched metastases in lung cancer
Introduction
Tumor neoantigens are peptide antigens derived from somatic mutations of the tumor genome and presented by major histocompatibility complex (MHC) on the surface of malignant cells (1-3). Unlike tumor-associated antigens (TAA), tumor neoantigens are unique to the tumor cells that are not expressed on normal cells. It therefore could be differentially identified by the host immune system to generate robust tumor-specific T cell responses (4-6). With the advancement of genomics and bioinformatics, including high-throughput sequencing and computational algorithms for neoantigen prediction, personalized vaccines for cancer immunotherapy based on neoantigens has been proven to be promising (7-11). In a syngeneic mouse model, using vaccine with a computationally designed synthetic mRNA generated by syngeneic tumor, a complete rejection of established tumors along with potent CD4+ and CD8+ T cell responses were observed (12). Such findings were recently validated by three first-in-human studies in advanced melanoma (13-15). These findings suggest that tumor neoantigens are attractive immune targets for therapeutic vaccines that hold promise for the long-term control of cancer (16).
However, a series of questions remain to be answered before such strategy can be widely applied in the clinical settings (17,18). As an example, personalized cancer vaccines based on neoantigens are mainly leveraged in patients with locally advanced or metastatic solid tumors. Since patients are suffering not only from the primary lesions but also the metastases, it is therefore important to know whether there is discrepancy in either the counts or types of neoantigens between primary lesions and their metastases, especially considering their differences in biological evolution as well as tumor immune microenvironment, cell-cell or cell-stroma interactions, etc. (19,20). Herein, it is an emergent need to clarify whether the tumor specific neoantigens identified from primary tumors could well represent those in the matched metastasis.
In this study, we aimed to investigate and compare the tumor neoantigens between primary lesions and matched metastases using 79 samples from 24 patients with lung cancer, including 10 with matched liver metastasis (LM), 10 with matched brain metastasis (BM) and 4 with sole BM. We hope our investigation will help in understanding the biology of tumor neoantigens, and developing better neoantigen-based vaccines to simultaneously target primary and metastatic tumors.
Methods
Sample collection
Primary lung cancers, matched metastases and peripheral blood were collected before any systemic therapy as part of the standard clinical care between January 2014 and December 2015 in three Chinese medical centers. Additional metastatic samples including sole BM (primary sites were not available) and matched peripheral blood were also identified. The surgical specimens and biopsy tissues were snap-frozen in liquid nitrogen within 30–45 minutes of harvest. Then 8–10 µm fresh frozen or formalin-fixed paraffin-embedded (FFPE) sections were made and hematoxylin-eosin (HE) stained slides were reviewed by pathologists to confirm the histological types and estimate the proportion of malignant cells relative to non-tumor cells. The criteria for further sequencing analysis are the presence of at least 50% tumor nuclei and less than 20% necrosis on histological review of each sample (21). Genomic DNA was extracted from all samples. Germline DNA control and human leukocyte antigen (HLA) typing was extracted and analyzed from the matched peripheral blood leukocytes. The study protocol was approved by the Institutional Review Board of Shanghai Pulmonary Hospital (No. FK-18-109). Informed consent was obtained before sample collection. This study was conducted in accordance with the Declaration of Helsinki.
DNA extraction and library construction
DNA was extracted using DNeasy Blood and Tissue Kit or the QIAamp DNA FFPE Tissue Kit (Qiagen, Hilden, Germany) and quantified with the Qubit dsDNA HS Assay kit (Life Technologies, USA) according to manufacturer’s instructions. Genomic DNA was sheared into 150–200 base pairs (bp) fragments with Covaris M220 Focused-ultrasonicator TM Instrument (Covaris, Massachusetts, USA). Fragmented DNAs were constructed using KAPA Hyper Prep Kit (Illumina platforms) (KAPA Biosystems, Massachusetts, USA) per manufacturer’s recommended protocol. Multiple indexing adaptors were ligated to the ends of the DNA fragments to prepare them for hybridization onto a flow cell. Purification and size selection of the library were conducted by using AMPure XP magnetic beads (Beckman Coulter, Brea, CA, USA). The concentration and quality of the library was determined using the Qubit 3.0 system and Bioanalyzer 2100 (Agilent, Agilent HS DNA Reagent, 5067-4627).
Whole-exome sequencing
DNA libraries were subjected to whole-exome capture with xGen Exome Research Panel v1.0 (Integrated DNA Technologies), which spans a 39 Mb target region (19,396 genes) of the human genome and covers 51 Mb of end-to-end tiled space. Human Cot-1 DNA (Life Technologies) and xGen universal blocking oligos (Integrated DNA Technologies) were added as blocking reagents to reduce non-specific hybridization. The capture reaction was conducted with NimbleGen SeqCap EZ Hybridization and Wash Kit (Roche) and Dynabeads M-270 (Life Technologies) according to manufacturer’s recommended protocol. The captured samples were sequenced on an Illumina HiSeq X-TEN platform with a paired-end run of 2×150 bp. The quality of each read was initially verified using the software embedded in the HiSeq X-TEN sequence. A FASTQ file was generated for each tested sample for sequence alignment and converted to a BAM file for further analysis.
Data filtering and variant calling
The generated sequencing reads were initially parsed with FLEXBAR for adapter trimming and low quality bases were filtered out (22). Obtained reads were aligned to hg19 human genome reference, using BWA aligner v0.7.12 (23). Duplicated reads were then removed from the aligned and sorted BAM files by using Picard 2.2.1. GATK v3.8 was used to do local realignment around potential small insertions and deletions (indels) and base recalibration for next step mutation calling procedures. We used MuTect v1.1.7 to detect single nucleotide variants (SNVs) and Strelka v1.0.14 to call small indels (24,25). Tumor-normal paired sample calling was processed during the mutation calling procedure, in order to filter out the personal germline mutations. ANNOVAR was run to screen the nonsynonymous mutations in the exonic region for further study. The candidate variants with a variant allele frequency (VAF) above 0.2% were recorded in the population database EXAC (The Exome Aggregation Consortium) v.03 and Genome Aggregation Database (gnomAD) were filtered out for further identification of the somatic mutations.
Neoantigen prediction
We used the pVAC-Seq pipeline with the NetMHCpan version 4.0 binding strength predictor and Pickpocket to identify potential tumor neoantigens (26). The major criteria for tumor neoantigen identification included: (I) derived from tumor-specific (missense, frameshift, inframe indels, and fusions); (II) high predicted affinity to patients specific HLA class I alleles (IC50 <500 nmol/L) with k-mer of 9–11 length; (III) fold change
Statistical analysis
Comparisons of neoantigen counts between paired primary tumors and metastases were based on Student’s t-test. Non-parametric Mann-Whitney U test was applied for comparison of neoantigen counts between different groups. The categorical variables were compared using Chi-square test, or Fisher’s exact test. The continuous variables were analyzed by ANOVA and Tukey’s multiple comparison tests. The associations between tumor neoantigen burden (TNB, defined as the number of predicted neoantigens) and clinicopathologic features were assessed using Chi square tests. The correlations of TNB between primary lesions and matched metastases were evaluated using Pearson’s correlation analysis. All tests were performed with GraphPad Prism 6.0. P<0.05 was considered statistically significant.
Results
The baseline feature and comparison of TNB in primaries and their metastases
We firstly conducted WES on 60 sample from 20 cases, including 10 with primary lesions and LM, and 10 with primary lesions and BM, yielding a mean sequencing depth of 155× (104×–247×). Among them (Figure 1), 12 (60.0%) patients were male and 8 (40.0%) had smoking history with a median age of 57 years old. There were 16 patients with lung adenocarcinoma, 2 with lung squamous cell carcinoma and 2 with small-cell lung cancer, respectively. Neoantigen predictions were performed according to the identified somatic mutations per tumor. No specific neoantigens were found to be particularly associated with LM or BM. There was a significant correlation between TNB and tumor mutational burden (TMB) in both the primary lesions and metastases (Figure S1A,B). A wide range of TNB were identified in both the primary lesions (median 214, range 54–2,992) and metastases (median 178, range 34–2,488) (Figure 1). The TNB of primary lesions was similar to matched metastases (P=0.632) (Figure 2A). Moreover, the TNB in metastases was significantly associated with those in primary lesions (R2=0.872, P<0.001) (Figure 2B). Although gene fusions seem to contribute a higher percentage of neoantigens in metastases than in primary lesions (e.g., in LM10, LM16 and BM14), the overall distribution pattern of neoantigens based on genetic variant types and predicted HLA binding affinity showed high concordance between matched primary and metastatic lesions (Figure 2C,D). Interestingly, it seems that neoantigens of BM group were mainly derived from gene fusions, and much of them had the stronger affinity than those of LM group, but it did not reach the statistical significance (Figure S2). Additionally, gender, smoking history, histological types and metastatic sites had no impact on TNB of primary lesions or metastases (Figure S3). Never-smoking female patients just had numerically lower TNB than male smokers without statistical significance (Figure S4), which might be due to limited sample size.
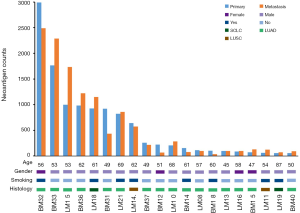
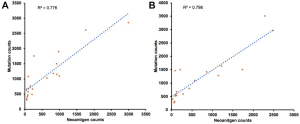

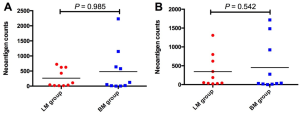
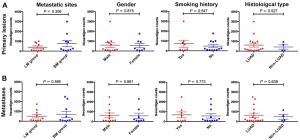
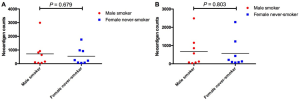
Neoantigens landscape in primaries and their metastases
To investigate the landscape of neoantigens between primary lesions and matched metastases, identified neoantigens were classified as shared (present in both primary and metastatic lesions) and private (present only in the primary or metastatic lesions) according to the previous reports (30,31). We observed a relatively low percentage of neoantigens identified as shared, with a median of 19.6% (range, 2.1–32.7%) (Figure 3A). There was no difference in the percentage of shared neoantigens between primaries and matched metastases (median 28.3% vs. 28.8%, P=0.934) (Figure S5). Considering the clinical significance of shared neoantigens in personalized vaccine therapy, we further surveyed the distribution and predicted HLA binding affinity of shared neoantigens based on genetic variant types. The shared neoantigen details of each case are available on request. As shown in Figure 3B, shared neoantigens of each sample were formed by SNVs, indels and fusions. SNVs and fusions contributed most of the shared neoantigens in these samples, with a median percentage of 85.2% (range, 33.0–100.0%). Most shared neoantigens were strong binders and a similar rate of neoantigens with strong affinity was also observed in patients with LM than with BM (Figure 3C). We further compared the corresponding genes of the top 10 shared neoantigens with highest HLA binding affinity among different patients. Not surprisingly, significant variation was observed across all cases (Figure 3D), echoing the concept that cancer is a very “personal” disease. However, we did observe interesting pattern although more samples are needed for confirmation. For example, mucin (MUC) family genes were found commonly involved (35.0%, 7/20) including MUC4, MUC6, MUC16 and MUC17, followed by CACNA1E (20.0%, 4/20), FHOD3 (20.0%, 4/20), PABPC3 (20.0%, 4/20) and LUC7L2 (20.0%, 4/20) (Figure 3D).
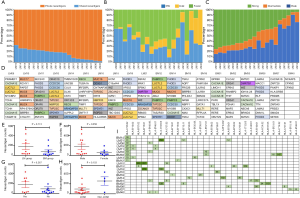
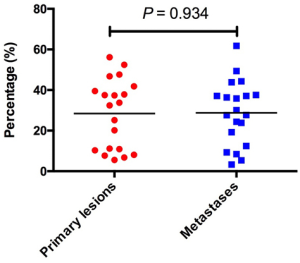
Impact of clinical parameters on shared neoantigens counts and corresponding HLA genotypes
Patients with LM had a numerically higher percentage of shared neoantigens than those with BM (mean 176 vs. 80, P=0.174; Figure 3E). Male patients seemed to have higher shared neoantigens than females but it did not reach the statistical significance (P=0.058; Figure 3F), whereas smoking history and histological type had no effect on the percentage of shared neoantigens (Figure 3G,H). We further determined the corresponding HLA genotypes of shared neoantigens. Intriguingly, a variety of HLA genotypes were identified. Most of them belonged to HLA-B and HLA-C, especially HLA-C*06:02 allele was found in 10 cases (50.0%) (Figure 3I). Of note, a large number of shared neoantigens were identified to be restricted to HLA-B*15:01 and HLA-C*03:03 alleles (Figure 3I).
Neoantigen intratumor homogeneity and heterogeneity in sole BMs
Since BM presented higher degree of genetic evolution (unpublished data from our group), we then asked if it plays potent role in shaping the landscape of neoantigens. As a proof of concept, we decided to seek the answer by studying the neoantigen intratumor (multi-regions of a single brain metastasis) heterogeneity as previously described (32). We collected BM specimens from another four patients. A single BM lesion was divided into 3–4 pieces from patients BM91, BM92, BM93 and BM94 for intratumor analysis (Figure S6). Based on neoantigen counts, we found the intratumor homogeneity and heterogeneity in all four patients (Figure 4A). The distribution of neoantigen type was almost identical among distinct lesions (Figure S7). We further analyzed the ratio of shared neoantigens. Interestingly, the percentage of shared neoantigens among different pieces of the same BM lesion was also found nearly 20.0% across all specimens, which was similar to those in the paired primaries and metastases (Figure 4B). Again, we compared the corresponding genes of top 20 shared neoantigens with highest HLA binding affinity among different pieces of all cases. Interestingly, we found FRG2B presented in all cases, and WIZ and NBPF1 were found in 75% of cases (Figure 4C), suggesting the existence of both intratumoral and intertumoral homogeneity in BM. The shared neoantigens were derived from SNVs, indels and fusions, and SNVs and fusions contributed most of the shared neoantigens, with a median percentage of 92.0% (range, 85.4–97.3%) (Figure 4D). Neoantigens with high HLA binding affinity were dominant (Figure 4E). The corresponding HLA genotypes were polymorphic and HLA-C*14:02 allele was found in two cases (50.0%). Intriguingly, shared neoantigens are mainly restricted to HLA-B*15:01 and HLA-C*03:03 alleles (Figure 4E).
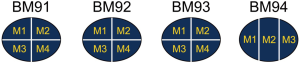
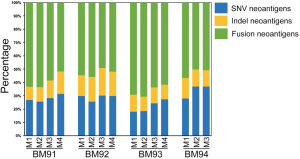
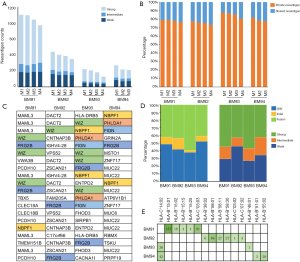
Discussion
Although personalized cancer vaccines based on tumor-derived neoantigens have shown strong and long-lasting antitumor effect in patients with melanoma, there are a series of undetermined questions before it can be clinically widely applied in advanced solid tumors including lung cancer. One major question is whether neoantigens identified from primary lesions could represent their metastases. To our knowledge, this is the first study to investigate the neoantigen landscape of primary lesions and matched metastases in advanced lung cancer. Through series of analysis, although we have observed the similarity of neoantigen composition (i.e., the proportions of SNV vs. Indel vs. fusions) between primary lung cancer and the matched metastatic lesions, only about 20% shared neoantigen was observed. While supporting the concept of clonal evolution, it is also reasonable to speculate that vaccines developed based on primary tumor neoantigen alone may not have optimal effect on metastatic lesions. We have also observed that gender, smoking history, histological types and metastatic sites had no impact on TNB. In addition, using the sole BM specimen, we observed both the inter- and intra-tumoral neoantigen homogeneity. It is mysterious that the percentage of shared neoantigen remains at ~20% between the primary and matched metastases, as well as among different pieces of the sole BM specimen. Whether this is a coincidence, or rather the result of evolution needs to be further explored. Nevertheless, our data suggested that simultaneous biopsy of primary and metastatic lesions might provide better information for the design of neoantigen-based vaccines in patients with advanced cancers.
Neoantigens could be derived from the nonsynonymous somatic mutations including SNVs, indels and gene fusions. Accumulating evidences suggest that distinct genetic variant types could generate various neoantigens with different immunogenicity and HLA-binding affinity (1,2). We therefore deconstructed the composition of shared neoantigens in these samples. Our results showed that identified shared neoantigens were mainly derived from SNVs and fusions, while indels just had a slight contribution to generation of neoantigens. However, recent evidence demonstrated indels are a class of highly immunogenic mutations which could trigger an increased abundance of neoantigens and greater mutant-binding specificity (33), it therefore suggested that the quantity/proportion of certain types of neoantigen may not be a key factor. Whether there are dominant neoantigens for effective cancer vaccine response remains to be deciphered.
Successful T-cell-mediated antitumor effect requires efficient presentation of tumor antigens by HLA class I (HLA-I) molecules (34). Previous study reported that specific HLA genotype was associated with extended overall survival in patients received immune checkpoint inhibitors (35). This may provide an opportunity for the development of therapeutic vaccines that potentially target immunodominant specific HLA-restricted neoantigens expressed by tumors. Herein, we surveyed the corresponding HLA genotypes of clonal neoantigens. Unexpectedly, a variety of HLA genotypes were identified. Most of them belonged to HLA-B and -C. This could be explained because HLA-B is generally expressed at higher levels than HLA-A and HLA-C on the cell surface, and they bind to a greater diversity of peptides (36). Antigen-presenting cells express higher levels of HLA-C on the cell surface than other cell types (37). Interestingly, a large number neoantigens were identified to match HLA-B*15:01 allele. But a recent study indicated that ICI-treated melanoma patients with HLA-B*15:01 had poor survival (35). Molecular dynamics simulations showed that dynamical elements of HLA-B*15:01 may impair the total strength of the interaction with T-cell receptor for effective neoantigen recognition. These findings suggested that we shall take into consideration the HLA genotypes upon designing the optimal neoantigen-based therapeutic vaccines.
Brain is one of the most common metastatic sites of patients with solid tumors including lung cancer (38). Although driver mutation-guided clinical studies have been successfully in matching patients with BM to novel targeted agents, there is still an unmet need in the current therapeutic strategies for a large proportion of BM patients without oncogenic alterations. Strikingly, a recent study reported that T cell-based therapy targeting shared neoantigen derived from histone 3 variant H3.3K27M mutation showed the strong antitumor effect on the glioma xenografts in mice (39). A phase I trial to assess the safety of repeated administration of the H3.3K27M specific vaccine in patients with glioma is ongoing (details in NCT02960230). These data offer the rational to develop neoantigen-based vaccines for patients with BM. However, considering the unique evolution of BM and its subsequent effect on genomic landscape (32), we need to comprehensively investigate the neoantigen landscape across multi-regions of BM. Unexpectedly, we observed the intratumor homogeneity in all cases. Further analysis suggested that the percentage of shared neoantigens was also nearly 20% across all specimens, which was similar to those in the paired primaries and metastases, suggesting certain clonal neoantigens are necessary for intrametastases maintenance and progression. These findings are similar to previous genomic characterization of BM from various solid tumors, which showed spatially and temporally separated BM sites were genetically homogenous (32). However, more samples are needed to conclude whether a single biopsy of BM is sufficient to represent the neoantigens of the whole BMs.
There are several limitations that should be pointed out. First, the number of cases included in the current study is relatively small to draw a solid conclusion and RNA-seq should be performed to further investigate identified alterations especially fusions. Second, it is desirable to directly compare the neoantigen landscape among the primary, LM and BM lesions in the same patients. However, it is technically challenging to collect such a spectrum of specimens since autopsy is not widely accepted by Chinese patients. Third, it would be more desirable to analyze the multiple metastatic lesions obtained at different stages of tumor progression over time or under the selective pressure of ICIs to better address the evolution of neoantigen landscape. Forth, we did not look at the specific CD8+ T cell in blood using MHC multimers and TCR repertoires due to the limited samples. Last but not least, we did not predict the binding to HLA class II and determine whether the loss of many predicted neo-antigens in the metastases was due to immuno-editing caused by a strong T cell response against these neoantigens or by spontaneous genetic instability. Nevertheless, we have observed meaningful results, based on which we are planning to collect more cases at different stages of tumor progression to validate our current findings.
In conclusion, this study suggested that the neoantigen landscape in terms of the number, type and predicted HLA binding affinity is similar between primary lesion and matched metastases. However, the percentage of shared neoantigens is only modest. To develop neoantigen-based cancer vaccine for lung patients with metastatic disease, whether we shall take the shared neoantigens into full consideration, or focus solely on the neoantigens of the primary lesion needs to be further explored.
Acknowledgments
Funding: This study was supported in part by grants from the National Natural Science Foundation of China (No. 81772467, 81871865, 81874036 and 81972167), the Backbone Program of Shanghai Pulmonary Hospital (No. FKGG1802), “Shuguang Program” supported by Shanghai Education Development Foundation and Shanghai Municipal Education Commission (No. 16SG18).
Footnote
Conflicts of Interest: All authors have completed the ICMJE uniform disclosure form (available at http://dx.doi.org/10.21037/tlcr.2020.03.03). CZ serves as an unpaid Editor-in-Chief of Translational Lung Cancer Research. The other authors have no conflicts of interest to declare.
Ethical Statement: The authors are accountable for all aspects of the work in ensuring that questions related to the accuracy or integrity of any part of the work are appropriately investigated and resolved. The study protocol was approved by the Institutional Review Board of Shanghai Pulmonary Hospital (No. FK-18-109). Informed consent was obtained before sample collection.
Open Access Statement: This is an Open Access article distributed in accordance with the Creative Commons Attribution-NonCommercial-NoDerivs 4.0 International License (CC BY-NC-ND 4.0), which permits the non-commercial replication and distribution of the article with the strict proviso that no changes or edits are made and the original work is properly cited (including links to both the formal publication through the relevant DOI and the license). See: https://creativecommons.org/licenses/by-nc-nd/4.0/.
References
- Schumacher TN, Schreiber RD. Neoantigens in cancer immunotherapy. Science 2015;348:69-74. [Crossref] [PubMed]
- Napoletano C, Bellati F. Neoantigens from the bench to the bedside: new prospective for ovarian cancer immunotherapy. Ann Transl Med 2019;7:S305. [Crossref] [PubMed]
- Desrichard A, Snyder A, Chan TA. Cancer Neoantigens and Applications for Immunotherapy. Clin Cancer Res 2016;22:807-12. [Crossref] [PubMed]
- Grunewald CM, Schulz WA, Skowron MA, et al. Tumor immunotherapy—the potential of epigenetic drugs to overcome resistance. Transl Cancer Res 2018;7:1151-60. [Crossref]
- Akbay EA, Kim J. Autochthonous murine models for the study of smoker and never-smoker associated lung cancers. Transl Lung Cancer Res 2018;7:464-86. [Crossref] [PubMed]
- Yarchoan M, Johnson BA 3rd, Lutz ER, et al. Targeting neoantigens to augment antitumour immunity. Nat Rev Cancer 2017;17:209-22. [Crossref] [PubMed]
- Capietto AH, Jhunjhunwala S, Delamarre L. Characterizing neoantigens for personalized cancer immunotherapy. Curr Opin Immunol 2017;46:58-65. [Crossref] [PubMed]
- Aurisicchio L, Pallocca M, Ciliberto G, et al. The perfect personalized cancer therapy: cancer vaccines against neoantigens. J Exp Clin Cancer Res 2018;37:86. [Crossref] [PubMed]
- Lee CH, Yelensky R, Jooss K, et al. Update on Tumor Neoantigens and Their Utility: Why It Is Good to Be Different. Trends Immunol 2018;39:536-48. [Crossref] [PubMed]
- Nogueira C, Kaufmann JK, Lam H, et al. Improving Cancer Immunotherapies through Empirical Neoantigen Selection. Trends Cancer 2018;4:97-100. [Crossref] [PubMed]
- Jiang T, Shi T, Zhang H, et al. Tumor neoantigens: from basic research to clinical applications. J Hematol Oncol 2019;12:93. [Crossref] [PubMed]
- Kreiter S, Vormehr M, van de Roemer N, et al. Mutant MHC class II epitopes drive therapeutic immune responses to cancer. Nature 2015;520:692-6. [Crossref] [PubMed]
- Carreno BM, Magrini V, Becker-Hapak M, et al. Cancer immunotherapy. A dendritic cell vaccine increases the breadth and diversity of melanoma neoantigen-specific T cells. Science 2015;348:803-8. [Crossref] [PubMed]
- Ott PA, Hu Z, Keskin DB, et al. An immunogenic personal neoantigen vaccine for patients with melanoma. Nature 2017;547:217-21. [Crossref] [PubMed]
- Sahin U, Derhovanessian E, Miller M, et al. Personalized RNA mutanome vaccines mobilize poly-specific therapeutic immunity against cancer. Nature 2017;547:222-6. [Crossref] [PubMed]
- Sahin U, Tureci O. Personalized vaccines for cancer immunotherapy. Science 2018;359:1355-60. [Crossref] [PubMed]
- Finn OJ. The dawn of vaccines for cancer prevention. Nat Rev Immunol 2018;18:183-94. [Crossref] [PubMed]
- Hu Z, Ott PA, Wu CJ. Towards personalized, tumour-specific, therapeutic vaccines for cancer. Nat Rev Immunol 2018;18:168-82. [Crossref] [PubMed]
- Binnewies M, Roberts EW, Kersten K, et al. Understanding the tumor immune microenvironment (TIME) for effective therapy. Nat Med 2018;24:541-50. [Crossref] [PubMed]
- Wan L, Pantel K, Kang Y. Tumor metastasis: moving new biological insights into the clinic. Nat Med 2013;19:1450-64. [Crossref] [PubMed]
- Shi J, Hua X, Zhu B, et al. Somatic Genomics and Clinical Features of Lung Adenocarcinoma: A Retrospective Study. PLoS Med 2016;13:e1002162. [Crossref] [PubMed]
- Dodt M, Roehr JT, Ahmed R, et al. FLEXBAR-Flexible Barcode and Adapter Processing for Next-Generation Sequencing Platforms. Biology (Basel) 2012;1:895-905. [Crossref] [PubMed]
- Li H, Durbin R. Fast and accurate short read alignment with Burrows-Wheeler transform. Bioinformatics 2009;25:1754-60. [Crossref] [PubMed]
- Cibulskis K, Lawrence MS, Carter SL, et al. Sensitive detection of somatic point mutations in impure and heterogeneous cancer samples. Nat Biotechnol 2013;31:213-9. [Crossref] [PubMed]
- Saunders CT, Wong WS, Swamy S, et al. Strelka: accurate somatic small-variant calling from sequenced tumor-normal sample pairs. Bioinformatics 2012;28:1811-7. [Crossref] [PubMed]
- Hundal J, Carreno BM, Petti AA, et al. pVAC-Seq: A genome-guided in silico approach to identifying tumor neoantigens. Genome Med 2016;8:11. [Crossref] [PubMed]
- Vita R, Overton JA, Greenbaum JA, et al. The immune epitope database (IEDB) 3.0. Nucleic Acids Res 2015;43:D405-12. [Crossref] [PubMed]
- Liu C, Yang X, Duffy B, et al. ATHLATES: accurate typing of human leukocyte antigen through exome sequencing. Nucleic Acids Res 2013;41:e142. [Crossref] [PubMed]
- Tang H, Zhu J, Du W, et al. CPNE1 is a target of miR-335-5p and plays an important role in the pathogenesis of non-small cell lung cancer. J Exp Clin Cancer Res 2018;37:131. [Crossref] [PubMed]
- Jamal-Hanjani M, Wilson GA, McGranahan N, et al. Tracking the Evolution of Non-Small-Cell Lung Cancer. N Engl J Med 2017;376:2109-21. [Crossref] [PubMed]
- McGranahan N, Furness AJ, Rosenthal R, et al. Clonal neoantigens elicit T cell immunoreactivity and sensitivity to immune checkpoint blockade. Science 2016;351:1463-9. [Crossref] [PubMed]
- Brastianos PK, Carter SL, Santagata S, et al. Genomic Characterization of Brain Metastases Reveals Branched Evolution and Potential Therapeutic Targets. Cancer Discov 2015;5:1164-77. [Crossref] [PubMed]
- Turajlic S, Litchfield K, Xu H, et al. Insertion-and-deletion-derived tumour-specific neoantigens and the immunogenic phenotype: a pan-cancer analysis. Lancet Oncol 2017;18:1009-21. [Crossref] [PubMed]
- Tran E, Robbins PF, Lu YC, et al. T-Cell Transfer Therapy Targeting Mutant KRAS in Cancer. N Engl J Med 2016;375:2255-62. [Crossref] [PubMed]
- Chowell D, Morris LGT, Grigg CM, et al. Patient HLA class I genotype influences cancer response to checkpoint blockade immunotherapy. Science 2018;359:582-7. [Crossref] [PubMed]
- Snary D, Barnstable CJ, Bodmer WF, et al. Molecular structure of human histocompatibility antigens: the HLA-C series. Eur J Immunol 1977;7:580-5. [Crossref] [PubMed]
- Schaefer MR, Williams M, Kulpa DA, et al. A novel trafficking signal within the HLA-C cytoplasmic tail allows regulated expression upon differentiation of macrophages. J Immunol 2008;180:7804-17. [Crossref] [PubMed]
- Jiang T, Su C, Li X, et al. EGFR TKIs plus WBRT Demonstrated No Survival Benefit Other Than That of TKIs Alone in Patients with NSCLC and EGFR Mutation and Brain Metastases. J Thorac Oncol 2016;11:1718-28. [Crossref] [PubMed]
- Chheda ZS, Kohanbash G, Okada K, et al. Novel and shared neoantigen derived from histone 3 variant H3.3K27M mutation for glioma T cell therapy. J Exp Med 2018;215:141-57. [Crossref] [PubMed]