DNA methylation markers that correlate with occult lymph node metastases of non-small cell lung cancer and a preliminary prediction model
Introduction
The TNM classification is an essential foundation of staging and treatment strategies in non-small cell lung cancer (NSCLC) patients. Lymph node (LN) metastasis is a significant factor compromising prognosis; 5-year overall survival with LNM is only 26–53% (1). Occult LN metastasis accounts for 22.4% of the total occult metastases in resectable NSCLC, and LN metastasis is associated with significantly worse disease-free survival and overall survival (2). The current imaging approaches are not sufficiently precise to diagnose occult LN metastasis before surgery, and invasive biopsy is not widely used due to its technical complexity and high probability of a false negative result. Therefore, more precise and non-invasive methods are warranted to determine the LN status preoperatively and lead to improved treatment strategy.
DNA methylation is an important epigenetic modification that involves differentiation and development, aging, and tumorigenesis. Abnormal DNA methylation is considered to be a hallmark of cancer development, causing instability of chromatin by inactivating gene transcription or suppressing gene transcription (3). Promoter hypermethylation and global DNA hypomethylation are rather common in cancer, and methylation alterations are often the first choice for tracing the signals from early stage tumors due to their early existence in cancer development. Previous studies have demonstrated specific DNA methylation signals for early NSCLC and early recurrence of the curative stage I NSCLC (4-7).
To our knowledge, there is currently no related research exploring the association between DNA methylation and occult LN metastasis in NSCLC. Herein, we aimed to screen and identify methylation biomarkers that could be used for occult LN metastasis diagnosis.
Methods
Patient selection
The research protocol was approved by the Ethics Committee of the First Affiliated Hospital of Guangzhou Medical University, and written informed consent was obtained from all patients before the operation using cancer tissue and blood samples. From October 2015 to January 2016, a total of 119 NSCLC patients with primary lesions less than 3.0 cm in diameter undergoing radical resection with or without adjuvant chemotherapy were performed in the Department of Thoracic Surgery of the First Affiliated Hospital of Guangzhou Medical University, of which 44 plasma samples matched. We retrospectively investigated these patients. The inclusion criteria were as follows: complete surgical resection; systemic LN dissection or sampling (at least three N2 stations or complete LN dissection); negative resection margins observed under a microscope; pathologically proven stages of pT1-3N1-2M0 NSCLC (according to the TNM classification of the 8th edition of AJCC); and the follow-up period was more than 4 months. Factors assessed included age, gender, smoking status, and pathological data (histology, primary tumor stage, and LN stage)
High throughput targeted methylation sequencing
A cohort of 119 age-matched malignant lung nodule tissue samples (27 pN+ and 92 pN0) were used for screening and identifying methylation markers specific for LN metastasis. High throughput targeted methylation sequencing was performed on plasma and tissue samples from patients with malignant lung nodules. We previously described the methods in detail (6), mainly including the isolation of tissue genomic DNA and plasma cell-free DNA (cfDNA), Bisulfite conversion and the AnchorIRISTM targeted methylation sequencing.
LN status-related feature selection and model establishment
The methylation profiles were compared between patients with and without occult LN metastases. We used beta binomial distribution base tool DSS package to detect differentially methylated markers from tissue samples. We obtained 878 differential methylation markers that passed FDR cutoff of 0.2 from tissue samples. Two preliminary prediction models were built by random forest with differentially methylated markers shared by plasma and tissue samples and markers present either in plasma or tissue samples respectively. The performance of these models was then evaluated using receiver operating characteristic (ROC) statistics derived from ten folds cross validation repeated ten times. Bootstraps resample methods with 100 repetitions were used for these activities.
Statistical analyses
Categorical data were analyzed by Pearson’s chi-squared test (SPSS, version 22.0, Chicago, IL, USA), a two-sided P value of less than 0.05 was considered statistically significant. We used the LASSO logistic regression model with penalty parameter tuning that had been conducted using 10-fold cross-validation based on minimum criteria. The likelihood ratio test with backward stepdown selection was applied to the multivariate logistic regression model. Detailed descriptions of the LASSO algorithm and DCA are provided in the supplementary data. R statistical software (v3.4, Bell Laboratories, Murray Hill, NJ, USA) was used for all statistical tests. We used the “glmnet” package to perform the LASSO logistic regression model analysis. Random forest implemented in Random Forest R package was used to build the predictive model.
The VIFs were calculated using the “car” package. The ROC curves were plotted using the “pROC” package.
Results
Patient baseline characteristics and study design
The study flowchart and AnchorMonarch-based deep learning system is shown in Figure 1. Retrospective study was performed on a total of 119 cases with NSCLC at pathological stages I–III. The 119 cases included 13 patients with squamous cell carcinoma (SCC), 101 patients with adenocarcinoma, and 5 patients with all other cancer types. Of the total cases, 85.7% were under 70 years old and 52.1% were female. The distribution of adenocarcinoma subtypes was as follows: AIS, 7 (6.9%); MIA, 20 (19.8%); and IA, 74 (73.3%). The distribution of pN stage was as follows: pN0, 91 (76.5%); pN1, 8 (6.7%); pN2, 19 (16.0%); and others, 1 (0.8%). The baseline characteristics of the patients in this study are shown in Table 1.
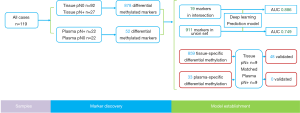
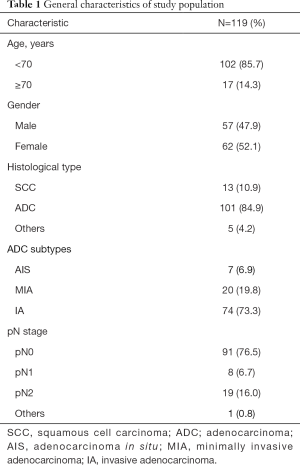
Full table
General characteristics of pN0 group and pN+ group in 119 cases
The 119 cases with pN0 consisted of 29% males and 48% females (pN+ in males: 18% vs. pN+ in females: 4%; P=0.000). The pathology in pN0 and pN+ with AIS, MIA, IA, SCC and other were as follows: 6%, 17%, 47%, 6% and 2% vs. 0%, 0%, 15%, 5% and 3%, respectively, P=0.003. General characteristics of the pN0 group and pN+ group are shown in Table 2.
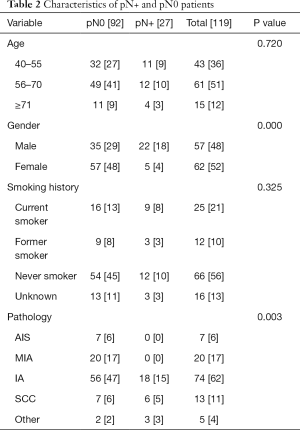
Full table
Differential methylation of pN0 group and pN+ group based on tissue samples
A cohort of 119 age-matched malignant lung nodule tissue samples, pN+ 27 cases vs. pN0 92 cases, respectively. Analysis of significantly different signals after AnchorIRISTM targeted methylation sequencing, we obtained a total of 878 differential methylation markers. Two hundred twenty nine genes, such as (ALDH7A1, ALG10, ALOX5, etc.) were hypermethylated and 649 genes, such as (ABCB1, ABCB4, ABCG1, etc.) were hypomethylated (Figure 2A,B).
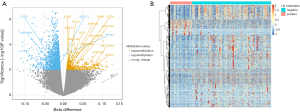
Differential methylation of pN0 group and pN+ group based on plasma samples
With plasma samples, we detected 52 differentially methylated markers associated with LN metastasis. Hypermethylation on 32 genes, such as TNFRSF1B, DMRTA2, VAV3-AS1, HIST3H2A, PALD1, NKX2-3, FAM24B-CUZD1, LRRC32, MAP3K12 and WSCD2, etc. were identified between the two groups. Hypomethylation on 20 genes, such as RAB42, KCNN3, UBE2L6, RARG, CRY1, MTUS2, TMEM179, MEGF11, MIR548H4 and NAGS, etc. were also detected (Figure 3A). 19 out of 52 markers discovered in plasma samples were also detected in tissue samples. The overlapped cluster of 19 genes in occult LN metastasis included CIRBP, CHGB, MEGF11, MIR548H4, EPHA4, DMRTA2, CCDC140, NKX2-3, HIST1H2AG, NT5C, LINC00620, WSCD2, COCH, ARHGAP10, LRRC74B, ICAM1, GNB4, HIST3H2A and FCHO1 (Figures 2A,3B).
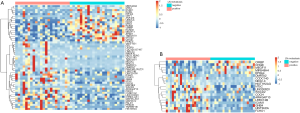
LN metastasis ROC categorized by plasma samples
Random Forest established two preliminary prediction models with differential methylation markers shared by plasma and tissue samples and markers present separately in plasma or tissue samples. The performance of these models was then assessed by ROC statistics stemmed from ten folds cross validation repeated ten times. ROC of the model built with a cluster of the 19 overlapped genes differentially methylated markers shared by tissue and plasma samples (Figure 4), the AUC of the preliminary prediction model is 88.6% (95% CI, 87.8–89.4%). ROC of the model built with 911 genes differentially methylated markers present in either tissue or plasma samples, and the preliminary prediction model is 74.9% (95% CI, 72.2–77.6%).
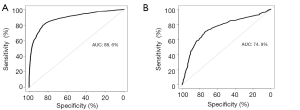
Tissue and plasma specific methylation differential markers in 9 matched occult LN metastasis of NSCLC patients
We sought to find out the differential methylated markers specifically in plasma samples and in tissue samples. In the whole dataset, we discovered 33 markers specifically methylated in plasma samples and 859 markers specifically methylated in tissue samples. In this cohort, there were 9 cases of occult LN metastasis with matching plasma samples. Significantly different methylation signals (Figure S1) were extracted and matched with the differential panel for occult LN metastases. 48 tissue-specific differential methylation markers were identified, included ALDH7A1, ALG10, BACH2, BCAS4, BCL11A, BCL6B, C14orf37 (Table S1). However, none of the 33 plasma-specific differential methylation markers were validated by matching the plasma 52 differentially methylated markers.
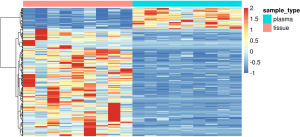
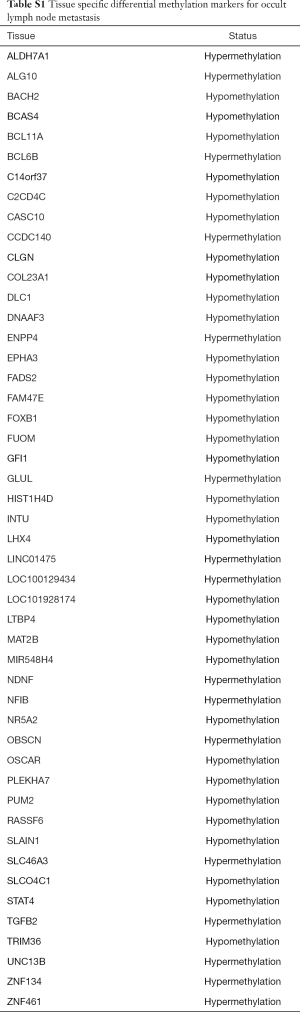
Full table
Discussion
To the best of our knowledge, this is the first study to show a specific non-invasive blood diagnostic tool cfDNA methylation markers in occult LN metastasis of NSCLC and to observe its predictive value. Previously, it was assumed that adenocarcinoma, upper or middle lobe located lesions, tumor size >3.0 cm, and primary tumor FDG-PET/CT SUVmax >4.0 g/mL were significant risk factors for occult mediastinal LN metastasis, and 68% of these metastatic foci were smaller than 4.0 mm (8). Furthermore, papillary-predominant and solid-predominant subtypes were significantly higher in occult nodal metastasis rate (9). We previously performed a radiomics nomogram to predict LN metastasis in solid lung adenocarcinoma, and showed that fourteen radiomics features were significantly correlated with LNM. Excellent calibration and discrimination in the training cohort and validation cohort were achieved, with an AUC of 0.871 and 0.856, respectively (10). However, the radiomics model was based on relatively large tumors and none of the above has been found to direct occult metastasis of LNs. Considering the difficulty in obtaining occult LN metastasis tissue, we still need desirable diagnostic tools to judge preoperative occult LN metastasis and postoperative residual LN metastasis.
LN metastases are driven by high tumor burden and specific biology. Greater tumor size is associated with higher risk for LN metastases (11). On the other hand, highly motivated tumor cells and a promoting environment contribute to this process especially in the early phase of disease. Occult metastasis of LNs is featured by small primary tumor and small LN burden, where biology plays a key role. Aberrant methylation is common in NSCLC and represents a promising marker for the molecular staging of these patients (12).
In this study, high-throughput targeted methylation sequencing was performed on plasma and matched tissue samples from a cohort of 119 lung cancer patients with a primary lesion less than 3.0 cm in diameter. The methylation profiles were compared between cases with and without occult LN metastases. There were no significant differences in age, gender, and smoking status between the two groups, but there were significant differences in pathological types. Invasive adenocarcinoma and other tumors (carcinoid, large cell carcinoma, mucoepidermoid carcinoma and lymphoepithelioma like carcinoma) significantly increased occult LN metastasis (P=0.003).
We obtained 878 differential methylation markers that passed FDR cutoff of 0.2. The selected methylation markers were subsequently used in building a robust statistical model for predicting LN metastasis in 22 pN+ and 22 pN0 plasma samples of NSCLC patients. 37% (19 out of 52 genes) of these cluster markers could be found in the matched tissue samples. We built a random forest predictive model with 19 markers shared by tissue and plasma samples and achieved an AUC of 88.6% (95% CI, 87.8–89.4%). We also attempted to build another predictive model with 911 markers present in either tissue or plasma samples and achieved an AUC of 74.9% (95% CI, 72.2–77.6%).
In summary, we have identified some DNA methylation markers for occult LN metastasis of NSCLC and developed a model for predicting occult LN metastasis with cell free DNA. Future clinical studies with larger sample size are needed to confirm this promising result.
Acknowledgments
Funding: This work was supported by the following funding: China National Science Foundation (Grant No. 81871893 & No. 81501996); Key Project of Guangzhou Scientific Research Project (Grant No. 201804020030); National Key Technology R&D Program (2018YFC1311900), Guangdong Science and Technology Foundation (2019B030316028).
Footnote
Conflicts of Interest: All authors have completed the ICMJE uniform disclosure form (available at http://dx.doi.org/10.21037/tlcr.2020.03.13). WL serves as an unpaid Associate Editor-in-Chief of Translational Lung Cancer Research. The other authors have no conflicts of interest to declare.
Ethical Statement: The authors are accountable for all aspects of the work in ensuring that questions related to the accuracy or integrity of any part of the work are appropriately investigated and resolved. The protocol of this study was approved by the Ethics Committee of the First Affiliated Hospital of Guangzhou Medical University (No. Kls2015-25).
Open Access Statement: This is an Open Access article distributed in accordance with the Creative Commons Attribution-NonCommercial-NoDerivs 4.0 International License (CC BY-NC-ND 4.0), which permits the non-commercial replication and distribution of the article with the strict proviso that no changes or edits are made and the original work is properly cited (including links to both the formal publication through the relevant DOI and the license). See: https://creativecommons.org/licenses/by-nc-nd/4.0/.
References
- Goldstraw P, Chansky K, Crowley J, et al. The IASLC lung cancer staging project: proposals for revision of the TNM stage groupings in the forthcoming (eighth) edition of the TNM classification for lung cancer. J Thorac Oncol 2016;11:39-51. [Crossref] [PubMed]
- Tang W, Lei Y, Su J, et al. TNM stages inversely correlate with the age at diagnosis in ALK-positive lung cancer. Transl Lung Cancer Res 2019;8:144-54. [Crossref] [PubMed]
- Cheng Y, He C, Wang M, et al. Targeting epigenetic regulators for cancer therapy: mechanisms and advances in clinical trials. Signal Transduct Target Ther 2019;4:62. [Crossref] [PubMed]
- Ma Y, Bai Y, Mao H, et al. A panel of promoter methylation markers for invasive and noninvasive early detection of NSCLC using a quantum dots-based FRET approach. Biosens Bioelectron 2016;85:641-8. [Crossref] [PubMed]
- Chen HF, Lei L, Wu LX, et al. Effect of icotinib on advanced lung adenocarcinoma patients with sensitive EGFR mutation detected in ctDNA by ddPCR. Transl Cancer Res 2019;8:2858-63. [Crossref]
- Liang W, Zhao Y, Huang W, et al. Non-invasive diagnosis of early-stage lung cancer using high-throughput targeted DNA methylation sequencing of circulating tumor DNA (ctDNA). Theranostics 2019;9:2056-70. [Crossref] [PubMed]
- Sun K. Clonal hematopoiesis: background player in plasma cell-free DNA variants. Ann Transl Med 2019;7:S384. [Crossref] [PubMed]
- Kanzaki R, Higashiyama M, Fujiwara A, et al. Occult mediastinal lymph node metastasis in NSCLC patients diagnosed as clinical N0-1 by preoperative integrated FDG-PET/CT and CT: Risk factors, pattern, and histopathological study. Lung Cancer 2011;71:333-7. [Crossref] [PubMed]
- Song CY, Kimura D, Sakai T, et al. Novel approach for predicting occult lymph node metastasis in peripheral clinical stage I lung adenocarcinoma. J Thorac Dis 2019;11:1410-20. [Crossref] [PubMed]
- Yang X, Pan X, Liu H, et al. A new approach to predict lymph node metastasis in solid lung adenocarcinoma: a radiomics nomogram. J Thorac Dis 2018;10:S807-S819. [Crossref] [PubMed]
- Yu X, Li Y, Shi C, et al. Risk factors of lymph node metastasis in patients with non-small cell lung cancer ≤2 cm in size: A monocentric population-based analysis. Thorac Cancer 2018;9:3-9. [Crossref] [PubMed]
- Harden SV, Tokumaru Y, Westra WH, et al. Gene promoter hypermethylation in tumors and lymph nodes of stage I lung cancer patients. Clin Cancer Res 2003;9:1370-5. [PubMed]