Circulating tumor DNA clearance predicts prognosis across treatment regimen in a large real-world longitudinally monitored advanced non-small cell lung cancer cohort
Introduction
The treatment of patients with advanced non-small cell lung cancer (NSCLC) has been revolutionized by the development of therapies targeting specific genetic alterations. The characterization of NSCLC into subtypes according to their genetic alterations has significantly improved the efficacy of targeted therapies and disease outcomes in subgroup of patients (1-4). However, their efficacies are compromised by development of resistance mechanisms (namely clonal evolution), which inevitably emerge in all patients with a median progression-free survival (PFS) ranging from a few months to a year (5-7). Response assessment primarily relies on imaging modalities, which may not reflect clonal evolution at molecular level (8). Therefore, there is a compelling need to develop improved modalities for monitoring clonal evolution.
The genomic profile of circulating tumor DNA (ctDNA), predominantly released by apoptosis and necrosis of cancer cells, has been shown to closely match that of tumor samples (9,10), and has now been validated as surrogate means for detecting mutations in NSCLC (11-13). For instance, plasma and tissue-based genotyping for EGFR T790M yielded equivalent clinical outcomes of osimertinib, thus supporting the use of plasma genotyping as an alternative diagnostic option (14). Much effort has been invested in exploring the potential of ctDNA in monitoring responses and assessing the emergence of drug resistance (15-17). Among patients undergoing epidermal growth factor receptor (EGFR) tyrosine kinase inhibitor (TKI) treatment, a reduction in the allelic fraction (AF) of EGFR mutation reflects sensitivity to these inhibitors (18). In addition, ctDNA has been instrumental in revealing novel resistance mechanisms, such as acquired EGFR C797S to osimertinib (5), MET Y1248H and D1246N to c-Met inhibitors, etc. (19).
Patients harboring the same mutation may exhibit marked differences in response to treatment (2). Circulating tumor DNA has been proposed as a noninvasive real-time biomarker to provide prognostic and predictive information for monitoring treatment (20-22). The prognostic value of ctDNA has been well-established in detecting minimal residual disease following surgery or treatment with curative intent, and is currently being explored in treatment responses of patients with advanced cancer (23-26). A recent study has shown that the detectable ctDNA at time of the diagnosis and identification of residual ctDNA at first evaluation were both associated with a poor prognosis (21). However, more work is needed to comprehensively examine its prognostic and predictive values in cohorts consisting of different treatment history.
In this prospective, real-world study, we performed capture-based ultra-deep targeted sequencing on longitudinal plasma samples to investigate the potential of ctDNA analysis in predicting clinical outcomes. We explored the genomic landscape of 1,336 Chinese patients with advanced NSCLC and subsequently focused on 248 of them with a minimum of 2 monitoring points for analyzing the predictive and prognostic value of ctDNA, as well as for investigating the dynamics of ctDNA upon pharmacological intervention by using a panel consisting of 168 NSCLC-related genes, covering 170KB of human genome.
Methods
Patient selection
From September 2015 to October 2016, advanced NSCLC (stage IIIB to IV) patients with specific mutations in at least one of the following genes EGFR, ALK, ROS 1, RET, KRAS, PIK3CA, ERBB2, MET and BRAF were enrolled. Their longitudinal plasma samples were collected at baseline and at various points throughout the ensuing treatment in multiple participating institutions. Detailed inclusion criteria were listed in supplemental methods. This study was approved by a central ethic committee at Nanjing General Hospital of Nanjing Command (2016NZKY-003-02). All other centers were covered by this protocol except for First Affiliated Hospital of Guangzhou Medical University (IRB2016-26) and Tianjin Medical School Affiliated General Hospital (IRB2016-050-01). All patients gave informed consent to participate in the study and gave permission for use of their peripheral blood.
Next generation sequencing (NGS) library preparation and capture-based targeted DNA sequencing
Fragments of size 200–400 bp were selected by AMPure beads (Agencourt AMPure XP Kit), followed by hybridization with capture probe baits, hybrid selection with magnetic beads and PCR amplification. Indexed samples were sequenced on Nextseq500 sequencer (Illumina, Inc., USA) with pair-end reads. An average depth of 11,816x was reached.
Statistical analysis
All statistical tests were conducted in R (version 3.3.1), using two-sided tests, unless otherwise specified. For patient characteristics, the differences in distribution of continuous and categorical variables across groups were assessed using Wilcoxon and Fisher exact tests, respectively. Survival tests were conducted using log-rank tests or Cox regression models when a co-variant was included.
Results
Patient demographics and study design
Within the screened population, 949 (71.03%) harbored driver mutations, 245 (18.34%) had no mutation detected, whilst the remaining 142 (10.63%) patients had non-driver mutations. Approximately 16% (n=207) patients were treatment-naïve; 71% (n=949) were previously treated and the remaining 13.5% (n=181) had no treatment history information available. Thirty-one percent of patients (n=410) had one line of previous treatment; 18.2% (n=244) had two lines; 11.1% (n=149) had three lines and the remaining 10.9% (n=146) had more than three lines of treatment (Figure 1A). The median follow-up time for patients enrolled in this study was 322 days (25–75%: 258–426 days). The median interval for ctDNA analysis was 95 days (25–75%: 82–120 days). Figure 1B shows detailed treatment history (outer ring) and treatment information during this study (inner ring). Among the 949 patients harboring driver mutations at baseline assessment, 376 patients received matched targeted therapy (MTT) according to sequencing results. Limited drug accessibility was the primary reason responsible for patients with driver mutation but unable to receive MTT. In addition, a significant number of patients had only EGFR sensitizing mutation upon progression on 1st generation EGFR-TKI, thus undergoing chemotherapy subsequently. A detailed view of their treatment prior to and during our study is shown in Figure S1. Detailed survival analysis was performed on 248 patients (longitudinal cohort), with 2 or more evaluation time points beyond the baseline. A total of 280 patients had 2 or more follow-up tests and 32 of them were excluded due to various reasons. The selection of patients enrolled in the follow-up cohort is shown in Figure 1A. The remaining patients, with either baseline assessment or only one time follow-up subsequent to baseline, had limited information for detailed survival analysis. Therefore, they were excluded in such analyses.
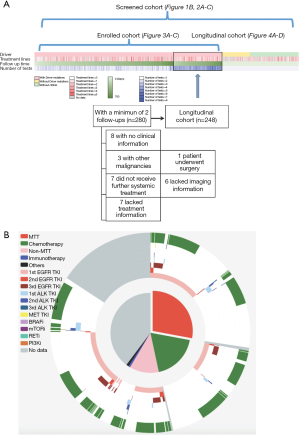
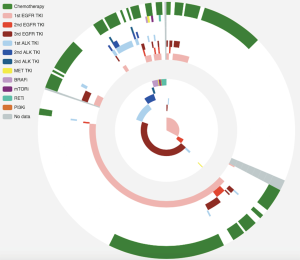
We first compared and contrasted baseline clinical parameters, including gender, age, smoking history, histology, stage, treatment history and metastatic sites between longitudinal and screened cohorts. Our data demonstrated that the two cohorts were similar in most of the parameters, except for gender, presence of bone metastasis and EGFR mutation status (Table S1). The longitudinal cohort had female predominance, a larger number of bone metastasis and EGFR mutations. These associations are due in part to EGFR being the most frequent driver mutation in Asian NSCLC patients, in particular female patients. However, such differences do not skew analyses performed in this study.
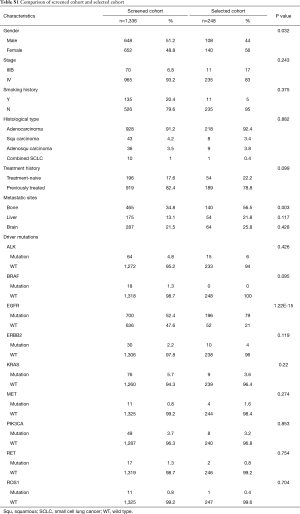
Full table
Landscape of baseline mutation
We performed capture-based ultra-deep targeted sequencing on all baseline plasma samples using a panel consisting of 168 genes, spanning 170KB of human genome. The design and validation of this panel, earlier described by Mao et al. (9), achieved 95% and 87% by-variant sensitivity for identifying mutations from matched tissue and plasma samples, respectively, excluding copy number variations (CNVs) (9). DNA obtained from white blood cells (WBCs) was used as a reference to sort out germline mutations. Overall, an average of 11,816× sequencing depth was achieved.
At baseline, we identified 3,503 aberrations spanning 132 genes, including 2,204 single-nucleotide variants (SNVs), 693 insertions or deletions (Indels), 412 copy-number amplifications (CNAs), 80 copy number deletions, and 114 translocations. Approximately 18% patients (245/1,336) had no mutations detected from this panel. EGFR was the most frequently mutated gene, followed by TP53, occurring in 55% and 41% of patients, respectively. Among all genetic aberrations identified, well-established NSCLC driver mutations, including EGFR, KRAS, BRAF, ERBB2, ALK, RET and ROS1, comprised 46.9% of all variants. The overview of mutation spectrum is shown in Figure 2A.
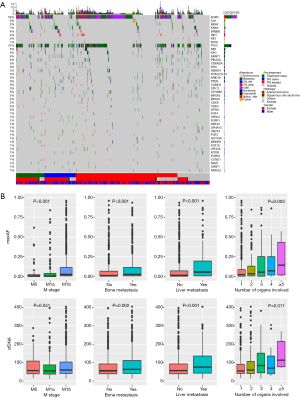
We then investigated the clinical relevance of baseline maximum allelic fraction (maxAF) and total cell-free DNA (cfDNA). MaxAF was defined as the maximum allelic fraction among all somatic mutations identified in a plasma sample. Higher maxAF and cfDNA were associated with more advanced M stage, a higher likelihood of bone/liver metastasis and more organs with secondary lesions (Figure 2B). Interestingly, maxAF showed a more significant correlation with all clinical features than the amount of cfDNA.
Overall survival (OS) is correlated with baseline ctDNA abundance and mutation load
We performed detailed analysis on the longitudinal cohort to assess the predictive and prognostic value of ctDNA. We first investigated the correlation between OS and baseline parameters, including ctDNA abundance and mutation load. Previous studies exploring the correlation between mutation load at baseline and OS provided controversial or inconsistent results (27,28). Our data revealed an inverse correlation between baseline ctDNA amount (expressed as the product of maxAF and total amount of cfDNA and OS (P=0.012). The mutation count was also inversely correlated with OS, independent of baseline ctDNA amount (P=8.5×10−4) (Figure 3A,B). We then derived a molecular signature for OS prediction using multivariate stepwise regression, starting from 6 genes that individually associated with OS: CDKN2A, EGFR, KEAP1, KRAS, MET and POM121L12. The final molecular signature consisted of KEAP1, KRAS and MET. Patients with no mutations in these genes had longer OS (P<0.0001) (Figure 3C).
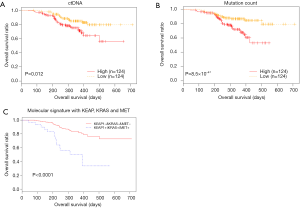
ctDNA clearance predicts longer PFS and OS
In clinical settings, treatment response is typically monitored on a regular interval by radiological imaging, which will not mirror clonal evolution. We analyzed the potential use of ctDNA as a surrogate marker for monitoring treatment response in our longitudinal cohort, which had at least 2 ctDNA tests. After a median follow-up of 157 days, disease progression occurred in 166 (66.9%) patients. During the course of treatment, 123 patients treated with either MTT or chemotherapy had a minimum of one time ctDNA clearance occurring from 1 to 15 months after commencement of treatment, with a median PFS of 8.6 months. CtDNA clearance is defined as lack of detectable mutation from this panel covering 168 lung cancer-related genes, with an average sequencing depth of 11,816× and 0.2% detection limit. Fifty patients achieved partial response (PR), 67 had stable disease (SD) and 3 had progressive disease (PD) as the best response, thus yielding to an overall response rate (ORR) of 41.7% and a disease control rate (DCR) of 97.5%. Up to June 25, 2017, the median OS of this group has not been reached. Conversely, 125 patients with consistent detectable ctDNA throughout the course of treatment had a median PFS of 4.1 months and a median OS of 16.7 months. Among them, 14 achieved PR, 64 had SD and 38 had PD as the best response, thus yielding to an ORR of 12.1% and a DCR of 67.2%. Taken together, this data reveals that patients with a minimum of one time ctDNA clearance experienced longer PFS (P=2.2×10−16; HR 0.28) and longer OS (P=4.5×10−6, HR 0.19) independent of baseline ctDNA amount, regardless of type of treatment and time of evaluation (Figure 4A). The baseline clinical parameters including gender, smoking history, stage, treatment history of patients with the minimum of one time ctDNA clearance and those with consistently detectable ctDNA were comparable, except for gender. More male patients experienced ctDNA clearance (Table S2). Furthermore, patients with the minimum of one time ctDNA clearance had better ORR (P=3.9×10−7) and DCR (P=1.4×10−10) compared to those with detectable ctDNA throughout the course of treatment. A similar trend was observed in patients undergoing MTT (Figure 4B), but not in those treated with chemotherapy (Figure S2). Overall, ctDNA clearance was a significant predictor of PFS (P=0.022) but not OS (P=0.22) in chemotherapy-treated patients after adjustment for baseline ctDNA amount. Collectively, this data shows ctDNA may be a valuable real-time biomarker for monitoring therapeutic response, whilst its clearance at any point of treatment may efficiently predict treatment response. This aspect actually mirrors clonal response, thus allowing uncovering the biological nature beyond the clinical response.
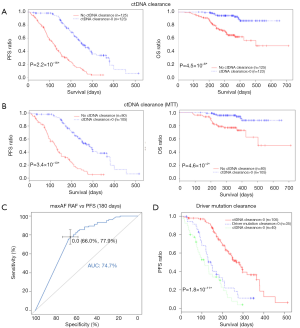
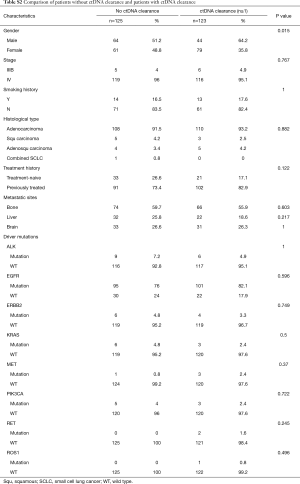
Full table
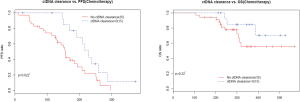
Previous studies showed that decreasing ctDNA levels during treatment may be associated with favorable therapeutic efficacy (21). We hence investigated whether or not ctDNA clearance or certain degree of ctDNA reduction reflected by maxAF would better correlate with treatment efficacy. To derive a binary classifier which can differentiate the population according to treatment efficacy, we performed receiver operating curve (ROC) analysis of changes in maxAF during the course of treatment. A decrease of maxAF to zero was identified as the optimal cutoff, characterized by area under curve (AUC) of 0.75 (95% CI: 0.691–0.802) (Figure 4C). Change in maxAF was defined as the ratio of smallest maxAF detectable in follow-up evaluations and baseline. Therefore, ctDNA clearance, but not ctDNA decrease in response to pharmacological interventions, could be identified as a predictive marker.
We then evaluated whether or not the clearance of driver mutation may provide similar predictive power as ctDNA clearance for predicting PFS. We compared PFS among 3 groups of patients, with all mutation clearance, only driver mutation clearance, and with presence of driver mutations. No difference in PFS was observed in patients with only driver mutation clearance and those with driver mutation, thus suggesting that monitoring only driver mutations will not efficiently predict PFS (Figure 4D).
Discussion
Therapeutic response in cancer is conventionally assessed with imaging techniques, which are however unable to identify clonal dynamics and evolutionary changes during therapeutic management. Recent studies showed that ctDNA may be a reliable tool for real-time tracking of molecular dynamics and hence for predicting treatment response defined by residual disease (21,22). In this study, we investigated the diagnostic value of ctDNA by performing capture-based ultra-deep sequencing on longitudinal plasma samples obtained at baseline and multiple efficacy evaluation time points from 248 patients with advanced NSCLC. Our real-world study, comprising both treatment-naïve and previously treated patients, shows that ctDNA may be a valuable real-time biomarker for monitoring therapeutic response, whilst ctDNA clearance at any point of treatment may also be used for efficiently predicting treatment benefits. CtDNA clearance was defined as no detectable mutations using this panel that covers 168 lung cancer-related genes, characterized by average sequencing depth of 11,816× and 0.2% detection limit. Albeit we cannot rule out the possibility that patients with “ctDNA clearance” had mutations with AFs below the detection limit (i.e., 0.2%), patients with the minimum of one time ctDNA clearance during the course of treatment had statistically significant longer PFS and OS compared to those with detectable ctDNA during therapy.
The prognostic value of ctDNA at first assessment (baseline) has been previously described in a prospective study comprising only newly diagnosed patients undergoing first-line treatment (21). Our study, consisting of a heterogeneous population and diverse evaluation schedules, not only confirmed the finding of this earlier investigation, but also extended the significance of ctDNA analysis for predicting treatment benefits in all patients, regardless of treatment history and time of evaluation. We also demonstrated that the assessment of ctDNA clearance may generate valuable clinical benefits, namely longer PFS and OS. However, our findings warrant additional studies aimed to define the value of ctDNA clearance as surrogate endpoint of therapeutic efficacy and as a risk stratification factor, especially for differentiating poor from favorable patient outcomes.
The prognostic and predictive value of ctDNA level before treatment remains a controversial issue. Many studies showed that increased ctDNA level at baseline is associated with unfavorable PFS and OS, while others failed to find a significant correlation with clinical outcomes (21,29,30). We observed a significant inverse correlation between baseline ctDNA amount and OS, while we also identified a molecular signature predictive of OS. A recent study has identified new determinants of ctDNA in NSCLC, including necrosis degree, lymph node involvement, lymphovascular invasion, pathological tumor size, Ki-67 labelling indices and tumor histology (31). Therefore, large cohort studies controlling for these factors would be necessary to accurately define the prognostic and predictive value of the baseline ctDNA assessment. There are some potential limitations associated with this study, including heterogeneity in treatments and time points in ctDNA evaluation.
Conclusions
To the best of our knowledge, this is the largest real-world study consisting of Chinese patients with advanced NSCLC to evaluate the value of ctDNA in monitoring treatment response. Taken together, our study showed the predictive and prognostic value of ctDNA clearance during treatment in a heterogeneous population with diverse treatment regiments and evaluation schedules.
Supplementary
Methods
Preparation of plasma cell-free DNA
Ten milliliters of peripheral blood were collected in struck tubes and centrifuged for 10 min at 2,000×g at 4 °C. The supernatant was transferred to a new tube and centrifuged again at 16,000×g at 4 °C for 10 min. Subsequently, circulating free DNA extraction was performed using the QIAamp Circulating Nucleic Acid kit (Qiagen). Quantification of cfDNA was performed using the Qubit 2.0 Fluorimeter with the dsDNA HS assay kits (Life Technologies, Carlsbad, CA). A minimum of 50 ng of cfDNA is required for NGS library construction.
NGS library preparation and Capture-based targeted DNA sequencing
DNA was subjected to end repair, phosphorylation and adaptor ligation. Fragments of size 200–400 bp were selected by AMPure beads (Agencourt AMPure XP Kit), followed by hybridization with capture probe baits, hybrid selection with magnetic beads and PCR amplification. A bioanalyzer high-sensitivity DNA assay was subsequently performed to assess the quality and size of the fragments. Indexed samples were sequenced on Nextseq500 sequencer (Illumina, Inc., USA) with pair-end reads. An average depth of 11,816× was reached with a limit of detection of 0.2%.
Patient selection
Our inclusion criteria were: (I) patients with advanced NSCLC-stage IIIB to IV; (II) both treatment-naïve and previously treated patients are qualified; (III) all histological types are eligible; (IV) patients must have at least one of the following mutations detected in their baseline plasma sample: EGFR (any mutations in exons 18–21 that are recorded by COSMIC or TCGA), ALK (any rearrangements in intron 19), ROS 1 (any rearrangements in intron 31–34), RET (any rearrangements in intron 11), KRAS (any mutations in exon2–4 that are recorded by COSMIC or TCGA), PIK3CA (any mutations in exon 10 and 21 that are recorded by COSMIC or TCGA), ERBB2 (exon 20 insertion) and BRAF (any mutations in exon 15 that are recorded by COSMIC or TCGA); (V) at least 18 years old and physically capable of undergoing systematic treatment.
Sequence data analysis
Sequence data were mapped to the human genome (hg19) using BWA aligner 0.7.10. Local alignment optimization, variant calling and annotation were performed using GATK 3.2, MuTect, and VarScan. Variants were filtered using the VarScan fpfilter pipeline, with loci with depth less than 100 filtered out. At least 2 supporting reads were needed for INDELs; while 8 supporting reads were needed for SNVs to be called. According to the ExAC, 1000 Genomes, dbSNP, ESP6500SI-V2 database, variants with population frequency over 0.1% were grouped as SNP and excluded from further analysis. Remaining variants were annotated with ANNOVAR and SnpEff v3.6. DNA translocation analysis was performed using both Tophat2 and Factera 1.4.3. White blood cells were used as a reference to filter out germline mutations.
Acknowledgments
We thank Dr. Haiyan Xu for valuable discussion and proofreading. We thank the patients and their families for participation. This study has been presented at ASCO Annual Meeting 2017 in Chicago, USA.
Funding: None.
Footnote
Conflicts of Interest: All authors have completed the ICMJE uniform disclosure form (available at http://dx.doi.org/10.21037/tlcr.2020.03.17). HHZ, XM, LZ, JY, HL, SC, and YH are employed by Burning Rock Biotech. YS and RR serves as the unpaid Editors-in-Chief of Translational Lung Cancer Research. MMK serves as the unpaid editorial board member of Translational Lung Cancer Research from Jul 2019 to Jul 2021. JKF serves as the unpaid editorial board member of Translational Lung Cancer Research from Dec 2019 to Nov 2020. The other authors have no conflicts of interest to declare.
Ethical Statement: The authors are accountable for all aspects of the work in ensuring that questions related to the accuracy or integrity of any part of the work are appropriately investigated and resolved. This study was approved by a central ethic committee at Nanjing General Hospital of Nanjing Command (2016NZKY-003-02) and written informed consent was obtained from all patients.
Open Access Statement: This is an Open Access article distributed in accordance with the Creative Commons Attribution-NonCommercial-NoDerivs 4.0 International License (CC BY-NC-ND 4.0), which permits the non-commercial replication and distribution of the article with the strict proviso that no changes or edits are made and the original work is properly cited (including links to both the formal publication through the relevant DOI and the license). See: https://creativecommons.org/licenses/by-nc-nd/4.0/.
References
- Mok TS, Wu YL, Thongprasert S, et al. Gefitinib or carboplatin-paclitaxel in pulmonary adenocarcinoma. N Engl J Med 2009;361:947-57. [Crossref] [PubMed]
- Hur JY, Lee JS, Kim IA, et al. Extracellular vesicle-based EGFR genotyping in bronchoalveolar lavage fluid from treatment-naive non-small cell lung cancer patients. Transl Lung Cancer Res 2019;8:1051-60. [Crossref] [PubMed]
- Mazières J, Zalcman G, Crino L, et al. Crizotinib therapy for advanced lung adenocarcinoma and a ROS1 rearrangement: results from the EUROS1 cohort. J Clin Oncol 2015;33:992-9. [Crossref] [PubMed]
- Maione P, Gridelli C. Crizotinib in ROS1-positive non-small cell lung cancer: an Asian large phase II trial confirms its key-role. Transl Cancer Res 2018;7:S792-5. [Crossref]
- Thress KS, Paweletz CP, Felip E, et al. Acquired EGFR C797S mutation mediates resistance to AZD9291 in non-small cell lung cancer harboring EGFR T790M. Nat Med 2015;21:560-2. [Crossref] [PubMed]
- Engelman JA, Zejnullahu K, Mitsudomi T, et al. MET amplification leads to gefitinib resistance in lung cancer by activating ERBB3 signaling. Science 2007;316:1039-43. [Crossref] [PubMed]
- Liam CK. Central nervous system activity of first-line osimertinib in epidermal growth factor receptor-mutant advanced non-small cell lung cancer. Ann Transl Med 2019;7:61. [Crossref] [PubMed]
- Colombi D, Di Lauro E, Silva M, et al. Non-small cell lung cancer after surgery and chemoradiotherapy: follow-up and response assessment. Diagn Interv Radiol 2013;19:447-56. [PubMed]
- Mao X, Zhang Z, Zheng X, et al. Capture-Based Targeted Ultradeep Sequencing in Paired Tissue and Plasma Samples Demonstrates Differential Subclonal ctDNA-Releasing Capability in Advanced Lung Cancer. J Thorac Oncol 2017;12:663-72. [Crossref] [PubMed]
- Jovelet C, Ileana E, Le Deley MC, et al. Circulating Cell-Free Tumor DNA Analysis of 50 Genes by Next-Generation Sequencing in the Prospective MOSCATO Trial. Clin Cancer Res 2016;22:2960-8. [Crossref] [PubMed]
- Bettegowda C, Sausen M, Leary RJ, et al. Detection of circulating tumor DNA in early- and late-stage human malignancies. Sci Transl Med 2014;6:224ra24. [Crossref] [PubMed]
- Spellman PT, Gray JW. Detecting cancer by monitoring circulating tumor DNA. Nat Med 2014;20:474-5. [Crossref] [PubMed]
- Yong E. Cancer biomarkers: Written in blood. Nature 2014;511:524-6. [Crossref] [PubMed]
- Oxnard GR, Thress KS, Alden RS, et al. Association Between Plasma Genotyping and Outcomes of Treatment With Osimertinib (AZD9291) in Advanced Non-Small-Cell Lung Cancer. J Clin Oncol 2016;34:3375-82. [Crossref] [PubMed]
- Siravegna G, Marsoni S, Siena S, et al. Integrating liquid biopsies into the management of cancer. Nat Rev Clin Oncol 2017;14:531-48. [Crossref] [PubMed]
- Crowley E, Di Nicolantonio F, Loupakis F, et al. Liquid biopsy: monitoring cancer-genetics in the blood. Nat Rev Clin Oncol 2013;10:472-84. [Crossref] [PubMed]
- Alix-Panabières C, Pantel K. Clinical Applications of Circulating Tumor Cells and Circulating Tumor DNA as Liquid Biopsy. Cancer Discov 2016;6:479-91. [Crossref] [PubMed]
- Thress KS, Brant R, Carr TH, et al. EGFR mutation detection in ctDNA from NSCLC patient plasma: A cross-platform comparison of leading technologies to support the clinical development of AZD9291. Lung Cancer 2015;90:509-15. [Crossref] [PubMed]
- Li A, Yang J, Zhang XC, et al. Acquired MET Y1248H and D1246N mutations mediate resistance to MET inhibitors in non-small cell lung cancer. Clin Cancer Res 2017;23:4929-37. [Crossref] [PubMed]
- Parkinson CA, Gale D, Piskorz AM, et al. Exploratory Analysis of TP53 Mutations in Circulating Tumour DNA as Biomarkers of Treatment Response for Patients with Relapsed High-Grade Serous Ovarian Carcinoma: A Retrospective Study. PLoS Med 2016;13:e1002198. [Crossref] [PubMed]
- Pécuchet N, Zonta E, Didelot A, et al. Base-Position Error Rate Analysis of Next-Generation Sequencing Applied to Circulating Tumor DNA in Non-Small Cell Lung Cancer: A Prospective Study. PLoS Med 2016;13:e1002199. [Crossref] [PubMed]
- Wan JC, Massie C, Garcia-Corbacho J, et al. Liquid biopsies come of age: towards implementation of circulating tumour DNA. Nat Rev Cancer 2017;17:223-38. [Crossref] [PubMed]
- Klco JM, Miller CA, Griffith M, et al. Association Between Mutation Clearance After Induction Therapy and Outcomes in Acute Myeloid Leukemia. JAMA 2015;314:811-22. [Crossref] [PubMed]
- Tie J, Wang Y, Tomasetti C, et al. Circulating tumor DNA analysis detects minimal residual disease and predicts recurrence in patients with stage II colon cancer. Sci Transl Med 2016;8:346ra92. [Crossref] [PubMed]
- Reinert T, Scholer LV, Thomsen R, et al. Analysis of circulating tumour DNA to monitor disease burden following colorectal cancer surgery. Gut 2016;65:625-34. [Crossref] [PubMed]
- Beaver JA, Jelovac D, Balukrishna S, et al. Detection of cancer DNA in plasma of patients with early-stage breast cancer. Clin Cancer Res 2014;20:2643-50. [Crossref] [PubMed]
- Shi W, Jiang T, Nuciforo P, et al. Pathway level alterations rather than mutations in single genes predict response to HER2-targeted therapies in the neo-ALTTO trial. Ann Oncol 2017;28:128-35. [Crossref] [PubMed]
- Rosenberg JE, Hoffman-Censits J, Powles T, et al. Atezolizumab in patients with locally advanced and metastatic urothelial carcinoma who have progressed following treatment with platinum-based chemotherapy: a single-arm, multicentre, phase 2 trial. Lancet 2016;387:1909-20. [Crossref] [PubMed]
- Pietrasz D, Pecuchet N, Garlan F, et al. Plasma Circulating Tumor DNA in Pancreatic Cancer Patients Is a Prognostic Marker. Clin Cancer Res 2017;23:116-23. [Crossref] [PubMed]
- Bidard FC, Madic J, Mariani P, et al. Detection rate and prognostic value of circulating tumor cells and circulating tumor DNA in metastatic uveal melanoma. Int J Cancer 2014;134:1207-13. [Crossref] [PubMed]
- Abbosh C, Birkbak NJ, Wilson GA, et al. Phylogenetic ctDNA analysis depicts early-stage lung cancer evolution. Nature 2017;545:446-51. [Crossref] [PubMed]