Novel genetic variants in genes of the Fc gamma receptor-mediated phagocytosis pathway predict non-small cell lung cancer survival
Introduction
Global cancer statistics in 2018 indicated that lung cancer was the most common cancer and the leading cause of cancer-related deaths, with an estimated 2,093,876 new cases and 1,761,007 cancer deaths worldwide (1). Non-small cell lung cancer (NSCLC) accounts for 85% of lung cancer cases, which mainly includes squamous cell carcinomas and adenocarcinomas. Recently developed immunotherapy has become a clinical research focus for the treatment of lung cancer, especially clinical application of the check-point inhibitors, such as PD-1/PD-L1 inhibitors (2). However, predicting the response to these therapies, has been challenging, because individuals’ response to the same treatment remains heterogeneous, suggesting that host factors including genetic variants also play an important role in variable treatment response. For example, single-nucleotide polymorphisms (SNPs) have been shown to be associated with both short-term response and long-term prognosis of cancer patients (3-5). Therefore, identifying the role of these genetic variants may help us generate a better model to predict outcomes and optimize therapies in the treatment of NSCLC patients.
Antibodies, produced in response to foreign antigens, are the key components that are responsible for innate and adaptive immunity. For example, immunoglobulin G (IgG) antibodies recognize tumor cells and form immune complexes to mediate effector activities by interactions between the Fc domains of IgG antibodies and the Type I and type II Fc gamma receptors (FCGRs) (6,7). In addition, the FCGR-mediated phagocytosis plays a central role in tumor immunity by tyrosine phosphorylation of multiple immunoreceptors, resulting in phagocytosis of target tumor cells (8). Furthermore, antibody-coated tumor cells interact with FCGRs and engage monocytes, macrophages or natural killer cells, leading to phagocytosis or cell-mediated cytotoxicity of tumor cells (9). Recent research has showed that antibody-dependent cellular phagocytosis (ADCP) is an important immune mechanism by which antibodies attack foreign antigens. For example, FCGR2A (CD32a) is thought to be a dominant player in the induction of ADCP by macrophages (10).
Several studies have reported that SNPs in the coding regions of some FCGR-related genes may have an effect on clinical response to mAbs. For instance, genetic variants in FCGR2A and FCGR3A predicted the response to trastuzumab in both metastatic HER2-positive breast cancer patients and gastric cancer patients (11,12), and the FCGR2A H/H genotype was associated with a better overall survival (OS) in cetuximab-treated colorectal cancer patients with a wild-type KRAS (13). But there are no reports about the effect of SNPs in the FCGR-related genes on survival of NSCLC. Thus, we hypothesize that genetic variants in the FCGR-mediated phagocytosis pathway genes are associated with a heterogeneous anti-tumor immune response, resulting in variable survival of NSCLC patients. In the present study, we tested this hypothesis by using publicly available genome-wide association study (GWAS) datasets to evaluate associations between genetic variants of genes in the FCGR-mediated phagocytosis pathway and NSCLC survival.
Methods
Study populations
We used one GWAS dataset for 1,185 NSCLC patients from the Prostate, Lung, Colorectal and Ovarian (PLCO) Cancer Screening Trial as the discovery and another for 984 NSCLC patients from the Harvard Lung Cancer Susceptibility (HLCS) study as the validation. The PLCO dataset had additional data on OS and disease-free survival (DSS) but not progression-free survival (PFS), while the HLCS dataset had data only on OS for analysis. The access to the PLCO GWAS dataset was approved by the dbGAP from the National Cancer Institute (the approval number: PLCO-95 and Project #6404), for which genomic DNA samples extracted from the whole blood were genotyped with Illumina HumanHap240Sv1.0, Human- Hap300v1.1 and HumanHap550v3.0 (dbGaP accession: phs000093.v2.p2 and phs000336.v1.p1) (14,15), while the DNA samples from HLCS patients extracted from the whole blood were genotyped with Illumina Humanhap610-Quad arrays. Details of data collection and participants’ characteristics in both GWAS datasets have been described elsewhere (16,17). The two original studies were approved by the institutional review boards of the National Cancer Institute and Massachusetts General Hospital, respectively, with a written informed consent obtained from each participant. The distributions of population characteristics in the PLCO and HLCS studies are shown in Table S1. Because all the data used in the present study were de-identified and previously collected, this exempt research was approved by Duke Internal Review Board (Pro00054575).
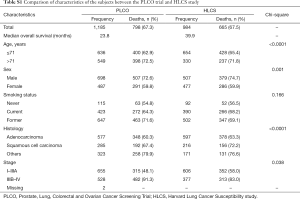
Full table
Gene and SNP selection
In total, 97 genes in the FCGR-mediated phagocytosis pathway were selected as the candidate gene-set after excluding duplicate genes and pseudo genes, according to the online datasets the Molecular Signatures Database (http://software.broadinstitute.org/gsea/msigdb/index.jsp) and the Human Biological Pathway Unification Database (http://pathcards.genecards.org) by the keyword “Fc gamma R” (Table S2). We imputed additional SNPs for these genes and their ±500 kb flanking buffer regions with IMPUTE2 and the 1,000 Genomes Project data (phase 3). SNPs in these genes and within their ±2 kb flanking regions were selected for further analysis based on the following criteria: a minor allele frequency ≥0.05, a genotyping rate ≥95%, a Hardy-Weinberg equilibrium P value ≥1×10−5, and imputation infor score ≥0.8.
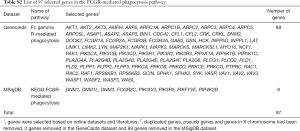
Full table
In silico functional analysis
We used three online bioinformatics tools to identify potential functional SNPs, including RegulomeDB (http://regulomedb.org/), HaploReg (https://pubs.broadinstitute.org/mammals/haploreg/haploreg.php) and SNPinfo (https://snpinfo.niehs.nih.gov/snpinfo/snpfunc.html). We further performed expression quantitative trait loci (eQTL) analysis to assess correlations between SNPs and mRNA expression levels. These mRNA expression data were obtained from lymphoblastoid cell lines derived from 373 European descendants included in the 1,000 Genomes Project (18) as well as from the whole blood and normal lung tissues in the genotype-tissue expression (GTEx) project (19). In addition, we compared the differences in mRNA expression levels for the paired tumor and adjacent normal tissues using the data from the Cancer Genome Atlas (TCGA) database (dbGaP Study Accession: phs000178.v9.p8) (20).
Statistical analysis
In the PLCO dataset, multivariate Cox proportional hazards regression analysis was used to evaluate associations between each of the SNPs and OS (in an additive model) with adjustment for age, sex, smoking status, histology, tumor stage, chemotherapy, radiotherapy, surgery and the first four principal components of the population structures. In the single-locus analysis, we estimated the hazards ratio (HR) and its 95% confidence interval (CI) by using the GenABEL package of R software (21). The false discovery rate (FDR) with a cut-off value of 0.20 was used to reduce the probability of false-positives findings (22), and the recommended Bayesian false-discovery probability (BFDP) with a cutoff value of 0.800 was also used for multiple test corrections under the consideration that the majority of SNPs were imputed with a high level of linkage disequilibrium (LD) among the SNPs under investigation (23). Then, SNPs with P≤0.005 were chosen for validation using the HLCS GWAS dataset. Meta-analysis was performed to combine the results of both discovery and validation datasets. If the Cochran’s Q-test P>0.100 and the heterogeneity statistic (I2) <50%, a fixed-effects model was applied; otherwise, a random-effects model was employed. In the PLCO dataset, different genetic models were performed to explore the associations between the validated SNPs and OS/DSS, and Kaplan-Meier curves and log-rank tests were used to compare the effects of genotypes on OS/DSS. To observe the combined effect of significant SNPs, we used the number of risk genotypes to estimate cumulative effects of the identified SNPs. Finally, we performed the receiver operating characteristic (ROC) curve and time-dependent ROC analysis with the time ROC package of R software to illustrate the prediction ability of the genetic model (24). In the eQTL analysis, we calculated correlations between SNPs and mRNA expression levels by using a general linear regression model. Additionally, we compared the mRNA expression levels between paired tumor and adjacent normal lung tissues available in the TCGA database by the paired t test. Statistical analyses were performed using PLINK (version 1.9), SAS software (version 9.4; SAS Institute, Cary, NC, USA) and R software (version 3.5.1). The Manhattan plots were generated by Haploview v4.2 and regional association plots were constructed by LocusZoom (http://locuszoom.sph.umich.edu/locuszoom/).
Results
Associations between SNPs in the FCGR-mediated phagocytosis pathway genes and NSCLC OS in both PLCO and HLCS datasets
Since only OS was available in both PLCO and HLCS datasets, we used OS to identified independent SNPs as NSCLC survival predictors. Basic demographic and clinical characteristics of the 1,185 NSCLC patients in the PLCO discovery dataset have been described elsewhere (25), with the results of multivariate Cox regression analysis adjusted for age, sex, smoking status, histology, tumor stage, chemotherapy, radiotherapy, surgery and first four principal components (detailed information on principal components was provided in Table S3). As shown in the flowchart (Figure 1), after imputation and quality control, we used 24,734 SNPs (including 2,009 genotyped and 22,725 imputed SNPs) in the multivariate Cox regression analysis. As a result, we identified 1,084 SNPs to be significantly associated with NSCLC OS after multiple test correction by BFDP ≤0.800. A Manhattan plot showing the associations between the SNPs and NSCLC OS is presented in Figure S1. Only the top 292 SNPs with P≤0.005 in multivariate Cox regression analysis in the PLCO datasets were included for further validation by the HLCS dataset. Two SNPs in intron regions of two different genes (i.e., rs9673682 in PLCG2 and rs115613985 in PLPP1, both of which were imputed) were validated after multivariate Cox regression analysis with adjustment for age, sex, histology, stage, smoking status, chemotherapy, radiotherapy, surgery and first three principal components. In the subsequent combined-analysis of these two datasets, the PLCG2 rs9673682 G allele was found to be associated with a better NSCLC OS (HR 0.87, 95% CI: 0.81–0.94, P=5.90×10−4), while the PLPP1 rs115613985 A allele was associated with a poorer NSCLC OS (HR 1.18, 95% CI: 1.08–1.29, P=1.32×10−4), and no heterogeneity was observed between the two datasets (Table 1).
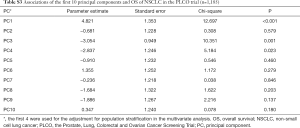
Full table
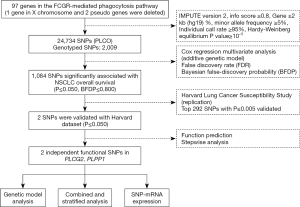
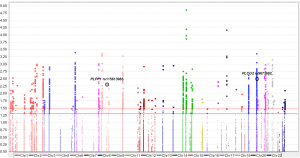
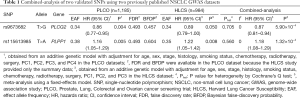
Full table
Identification of potentially functional and independent SNPs as NSCLC survival predictors
First, we used three bioinformatics tools (i.e., SNPinfo, RegulomeDB and HaploReg) to search for potential functions of the two SNPs and found that both SNPs were located in the intron regions of their genes with some considerable evidence of functions. As indicated by RegulomeDB, PLCG2 rs9673682 and PLPP1 rs115613985 had a score of 4 and 6, respectively (Table S4), but neither had functions based on the SNPinfo. In HaploReg, however, PLCG2 rs9673682 and PLPP1 rs115613985 were predicted to be located in histone marks, DNAse or motifs, which may have an effect on transcriptional activity. Therefore, these two SNPs were included in the further stepwise Cox regression analysis. As the HLCS dataset only provided the summary data, we had to use the PLCO dataset to perform the multivariate stepwise Cox regression analysis with adjustment for clinical variables and the first four principal components. The results suggest that both of the SNPs were significant and independent predictors of NSCLC OS (Table 2). In addition, we also conducted an independent test for all previously published SNPs identified in the present study populations in the PLCO dataset; as a result, both of these SNPs remained associated with NSCLC OS (HR 0.89, 95% CI: 0.80–1.00, and P=0.040 for PLCG2 rs9673682 and HR 1.23, 95% CI: 1.10–1.37 and P<0.001 for PLPP1 rs115613985), independent of other previously published SNPs (Table S5).
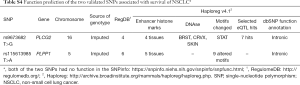
Full table
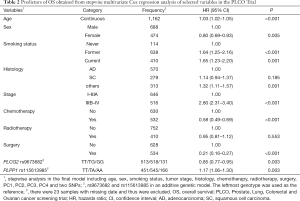
Full table
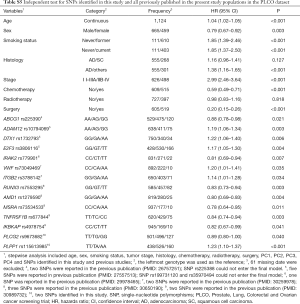
Full table
In the PLCO dataset, multivariate Cox regression analyses with different genetic models were also used to evaluate the effect of each SNP on risk of death. The PLCG2 rs9673682 G allele was significantly associated with a decrease in risk of death [for OS: HR 0.90, 95% CI: 0.77–1.04 for TG, 0.70 (0.55–0.90) for GG compared with TT, Ptrend=0.005; for DSS: 0.88 (0.75–1.03) for TG, 0.70 (0.54–0.90) for GG compared with TT, Ptrend=0.004], while the PLPP1 rs115613985 A allele was significantly associated with an increase in risk of death [for OS: 1.20 (1.03–1.40) for TA, 1.33 (1.06–1.65) for AA compared with TT, Ptrend=0.005; for DSS: 1.19 (1.01–1.41) for TA; 1.32 (1.05–1.67) for AA compared with TT, Ptrend=0.009; Table 3]. Kaplan-Meier curves of the associations between these SNPs and NSCLC OS and DSS are presented in Figure S2, and the regional association plot of each SNP is shown in Figure S3. In a dominant genetic model, PLCG2 rs9673682 TG + GG genotypes were consistently associated with better OS (HR 0.85, 95% CI: 0.74–0.99; P=0.030) and DSS (HR 0.84, 95% CI: 0.72–0.97; P=0.021), while PLPP1 rs115613985 TA + AA were associated with poorer OS (HR 1.23, 95% CI: 1.06–1.42; P=0.008) and DSS (HR 1.22, 95% CI: 1.04–1.43; P=0.013) (Table 3).
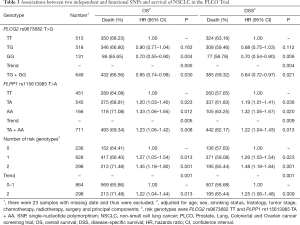
Full table
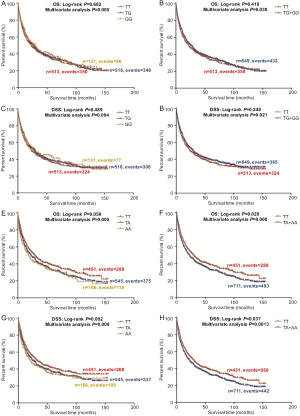
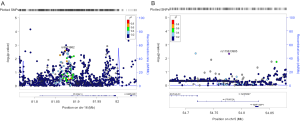
Combined and stratified analyses of the two independent and functional SNPs in the PLCO dataset
To better estimate the joint effect of these two SNPs on risk of death, we combined their risk genotypes (i.e., rs9673682 TT and rs115613985 TA + AA) into a genetic score as the number of risk genotypes. The trend test indicated that an increased genetic score was associated with a poorer survival (Ptrend=0.001 for OS and Ptrend=0.001 for DSS; Table 3). Based on similar survival rates of the subgroups, we divided all patients into a low-risk group (0–1 risk score) and a high-risk group (2 risk scores). Compared with the low-risk group, patients in the high-risk group had a higher death risk in the PLCO dataset (OS: HR 1.22, 95% CI: 1.04–1.44 and P=0.013; DSS: HR 1.25, 95% CI: 1.06–1.48 and P=0.009, Table 3). Moreover, when we created a genetic score by combining these two SNPs with all other previously reported SNPs identified in the present study populations, we found that the effect on NSCLC OS/DSS increased as the genetic score increased (Ptrend<0.001) after adjustment for other covariates and that the high-risk group (i.e., 8–13 vs. 3–7 risk genotypes) was associated with a poorer survival (OS: HR 1.99, 95% CI: 1.71–2.31 and P<0.001; DSS: HR 1.94, 95% CI: 1.66–2.28 and P<0.001, Table S6). To visualize these effects, Kaplan-Meier curves for the associations between risk genotypes and NSCLC OS and DSS are shown in Figure 2 and Figure S4.
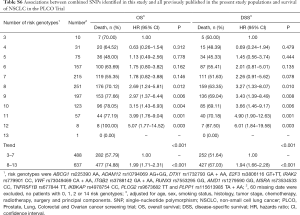
Full table
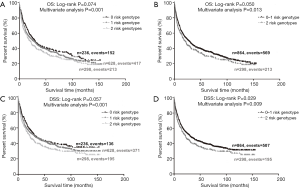
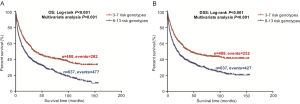
Stratified analysis was performed to investigate whether the joint effect of risk genotypes on NSCLC survival was modified by the covariates, including age, sex, smoking status, histology, tumor stage, chemotherapy, radiotherapy and surgery. Except for radiotherapy (OS: P=0.011; DSS: P=0.020), no significant interactions with these covariates were found (P>0.05 for both OS and DSS, Table S7). Therefore, we further reassessed the associations between the combined effect of the SNPs and NSCLC OS/DSS by subgroups of patients with or without radiotherapy (Table S8), and only in the subgroup of patients with radiotherapy, an increased genetic score was associated with a poorer survival (OS: Ptrend=0.001; HR 1.52, 95% CI: 1.19–1.96 and P=0.001 for 2 scores vs. 0–1 score; DSS: Ptrend<0.001; HR 1.55, 95% CI: 1.20–2.01 and P=0.001 for 2 scores vs. 0–1 score). To assess whether the SNP-associated outcomes in patients with NSCLC in early and advanced stages were also different, we calculated the survival for stage I–IIIA and IIIB–IV, separately. As a result, a significant association between an increased genetic score and a poorer survival was more obvious in patients with stage I–IIIA than in those with stage IIIB–IV [I–IIIA: Ptrend=0.030 and 0.017 for OS and DSS, respectively; scores 2 vs. 0–1: HR 1.27 (0.99–1.64) and 1.37 (1.03–1.81) for OS and DSS, respectively; IIIB–IV: Ptrend=0.012 and 0.017 for OS and DSS, respectively; HR 1.13 (0.92–1.39) and 1.14 (0.91–1.40) for OS and DSS, respectively, Table S9). These discrepancies in the survival between patients with stage I–IIIA and IIIB–IV were likely because the study population had more patients with stage I–IIIA than stage IIIN-IV, as no interaction was observed between the genetic scores and NSCLC stages.
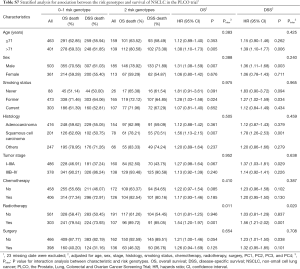
Full table
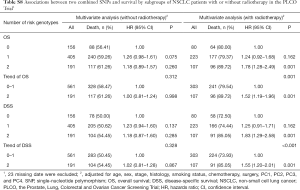
Full table
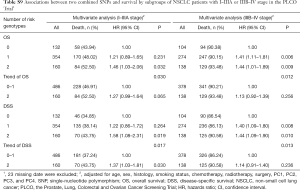
Full table
ROC and time-dependent area under the receiver operating characteristic curve (AUC) estimation for NSCLC survival prediction
To explore the ability of the combined model to predict survival of NSCLC, we estimated the predictive value of the combined risk genotypes with ROC and time-dependent AUC curves. We compared the AUC for clinical variables with or without the risk genotypes of the two SNPs. After adding the risk genotypes, the 5-year OS AUC increased non-significantly from 89.12% to 89.32% (P=0.347, Figure S5A) and the 5-year DSS AUC increased non-significantly from 88.92% to 89.11% (P=0.416, Figure S5B). However, the 5-year OS AUC significantly increased from 88.87% to 89.88% (P=0.040, Figure S5C) and the 5-year DSS AUC significantly increased from 88.57% to 89.76% (P=0.025, Figure S5D), when combining risk genotypes of these two SNPs and all other previously reported SNPs identified from the present study population, suggesting the importance of identifying additional risk genotypes in this study populations. Furthermore, time-dependent AUC curves of these two SNPs and all other previously reported SNPs identified in the present study populations were provided to assess the ability of model to predict NSCLC OS and DSS in this study populations through the entire follow-up period (Figure S5E,F,G,H).
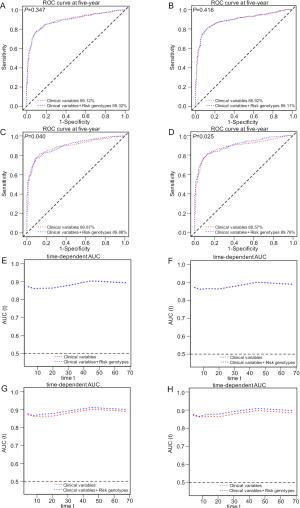
In silico functional validation
We further evaluated the correlations between SNPs and mRNA expression using RNA-seq of 373 transformed lymphoblastoid cell-lines in the 1,000 genomes project. Notably, the rs9673682 G allele was significantly correlated with increased mRNA expression levels of PLCG2 in both additive and dominant models (P=7.20×10−5 and 0.002, respectively), but not for PLPP1 rs115613985 (P=0.814 and 0.850, respectively) (Figure 3). Then, we extracted the eQTL analysis results from the database of the GTEx project and found that the rs9673682 G allele had a non-significant trend in correlation with mRNA expression levels of PLCG2 in the whole blood cells (n=369, P=0.645) and normal lung tissues (n=383, P=0.885); however, there were no PLPP1 expression data for further analysis (Table S10).
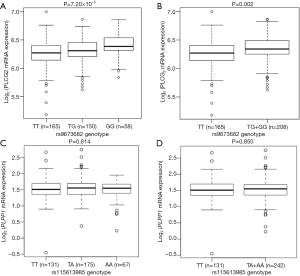

Full table
Additional differential expression analysis further revealed that both PLCG2 and PLPP1 had lower mRNA expression levels in 107 paired tumor tissues than in the adjacent normal tissues in the TCGA database (P≤0.001, Figure S6), and their lower expression levels were associated with a poorer NSCLC OS (26) (Figure S7).
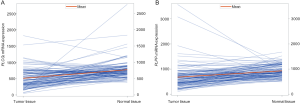
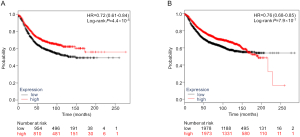
Discussion
In the present study, we re-analyzed the published GWAS datasets by a hypothesis-based gene-set approach in the post-GWAS era to explore the roles of genetic variants in clinical outcomes of NSCLC patients. We chose the FCGR-mediated phagocytosis pathway genes as the candidate gene-set. As a result, we identified two novel SNPs (i.e., PLCG2 rs9673682 T>G and PLPP1 rs115613985 T>A) as independent predictors of NSCLC survival after adjustment for available covariates as well as all other SNPs previously reported in the PLCO study population. We also assessed the effects of the combined risk genotypes of these two SNPs in the prediction model and found that they collectively predicted a poorer survival of NSCLC, which is consistent with the fact that both PLCG2 and PLPP1 harboring the SNPs are functioning in the same FCGR-mediated phagocytosis pathway. Further functional prediction analysis by assessing publicly available databases found that the PLCG2 rs9673682 G allele, which was associated with an improved survival, was also significantly associated with increased mRNA expression levels of PLCG2. Consistent with this, lower mRNA expression levels of PLCG2 were observed in tumor tissues than in adjacent normal tissues and were also associated with a poorer survival of lung cancer patients. Although we did not find evidence for a correlation between the PLPP1 rs115613985 A allele and its gene expression, the observed lower mRNA expression levels of PLPP1 in tumor tissues than in adjacent normal tissues were also associated with a poorer survival. In addition, a prediction model that included all identified risk genotypes of previously reported SNPs in the same study population had significantly improved 5-year survival prediction as indicated by the ROC curve. Taken together, our findings of associations between genetic variants in the FCGR-mediated phagocytosis pathway genes and NSCLC survival are biologically plausible.
PLCG2 is located on chromosome region 16q23.3 and encodes phosphoinositide specific phospholipase C family protein PLCG2, which is critical for the modulation of the calcium signals in response to the stimulation of immune receptors (27). The expression of PLCG2 is widely distributed on hematopoietic cells, including macrophages, natural killer cells, mast cells, and neutrophils (28). In macrophages, FCGR-mediated Ca2+ increase is dependent on PLCG2, and interestingly, this abrogation of Ca2+ increase changes the FCGR-mediated inflammatory reaction, but not the FCGR-mediated phagocytosis, suggesting other mechanisms may be involved in this process (29). Myeloid-derived suppressor cells (MDSCs) support tumor growth in many cancers by suppressing anti-tumor T cell responses. For example, one study demonstrated that down-regulation of PLCG2 signaling in MDSCs could result in their aberrant expansion during tumor progression, suggesting the possibility that PLCG2 may be the target of anti-cancer therapy (30). Moreover, mutations in PLCG2 are required for ibrutinib resistance in chronic lymphocytic leukemia, and patients with PLCG2 mutations are more likely to progress (31). In NSCLC, PLCG2 had a low somatic mutation rate according to the public available data from the database of the cBioPortal for Cancer Genomics (http://www.cbioportal.org). As shown in Figure S8, the mutation rate is low in both lung adenocarcinoma [2.19% (4/183) in the Broad study, 1.31% (12/915) in the MSK study, 1.23% (2/163) in the TSP study and 1.19% (7/586) in the TCGA study] and squamous cell carcinoma [0.78% (4/511) in the TCGA study], suggesting that germline genetic variants in PLCG2 may play a more important role in the regulation of mRNA expression of this gene. In the present study, we found that the rs9673682 minor G allele showed a significant association with a decreased risk of death in NSCLC patients and an increased PLCG2 expression level in normal blood cells. Additional experimental data from the ENCODE project suggest that PLCG2 rs9673682 T>G is located in a DNase I hypersensitive site with considerable levels of histone modification H3K4Me1, which may lead to an enhanced transcriptional activity (Figure S9A).
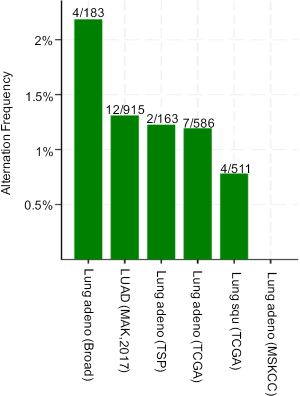
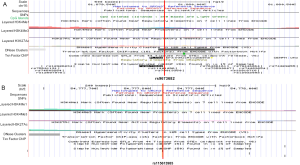
PLPP1, also known as PPAP2A or LPP1, is located on chromosome 5 and encodes a protein belonging to the phosphatidic acid phosphatase (PAP) family (32). One major function of PLPP1 is to dephosphorylate extracellular lysophosphatidic acid (LPA) and sphingosine-1-phosphate (S1P), which are important regulators of cell division, migration, and survival (33). It was shown that S1P might enhance the phagocytic activity of macrophages by up-regulating the expression of FCGR2A, suggesting PLPP1 might also contribute to the FCGR-mediated phagocytosis (34). Many cancer cells express low levels of PLPP1, which is an important contributor to the degradation of LPA, and high expression of LPA would produce resistance to chemotherapy and radiotherapy (35). Some in vivo studies showed that an increasing expression of PLPP1 in breast, ovarian and thyroid cancer cells would decrease tumor growth and metastasis in mouse models (36,37). In the present study, we observed that NSCLC patients with the PLPP1 rs115613985 A allele had a worse survival. However, the eQTL results showed that the rs115613985 A allele had a non-significant trend in a correlation with mRNA expression levels of PLPP1, indicating other mechanisms, such as somatic mutation or other epigenetic effects, might be involved in the transcription of the PLPP1 gene. Furthermore, PLPP1 rs115613985 T>A is located in an H3K4Me1 mark and a Tbp motif based on the ENCODE Project data and transcription factor CHIP-seq data (Figure S9B), suggesting the minor A allele might affect the transcription factors.
There are limitations in the present study. Although we identified associations of genetic variants in PLCG2 and PLPP1 with NSCLC survival, the results may be limited to Caucasian populations and thus not generalizable to other ethnic populations. The sample size of the PLCO was not large enough to pass the FDR cut-off value in the multiple test correction, for which we used the relatively less strident BFDP method as a result of the highly correlated SNPs from imputation. In the PLCO dataset, only a few clinical variables were available, and other needed information, especially the details of treatment and PFS, were not accessible. The HLCS dataset only provided the summary genotyping results and OS data but not DSS and PFS, and thus we used OS to identify independent SNPs as NSCLC survival predictors. In addition, although we found some functional evidence to support the observed associations, the potential molecular mechanisms (e.g., several molecular signature associated with cell survival, migration, and DNA damage response) between the SNPs and prognosis in NSCLC remain unclear, and further functional experiments are essential to explore these molecular mechanisms underlying the observed associations, which will eventually provide their clinical utility in future precision medicine.
Conclusions
In conclusion, we identified two independent, novel SNPs (i.e., PLCG2 rs9673682 T>G and PLPP1 rs115613985 T>A) that were significantly associated with NSCLC survival in both PLCO and HLCS GWAS datasets, of which PLCG2 rs9673682 T>G may have an effect on its mRNA expression. Our findings provided a rationale to further investigate the functions of survival-associated SNPs in the FCGR-mediated phagocytosis pathway genes in the development and progression of NSCLC.
Supplementary
Acknowledgments
We wish to thank all of the investigators and funding agencies that enabled the deposition of data in dbGaP and PLCO that we used in this study.
The datasets used for the analyses described in this manuscript were obtained from dbGaP at http://www.ncbi.nlm.nih.gov/gap through dbGaP accession number phs000336.v1.p1 and phs000093.v2.p2. Principal Investigators: Maria Teresa Landi. Genetic Epidemiology Branch, Division of Cancer Epidemiology and Genetics, National Cancer Institute, National Institutes of Health, Bethesda, MD, USA. Neil E. Caporaso. Genetic Epidemiology Branch, Division of Cancer Epidemiology and Genetics, National Cancer Institute, National Institutes of Health, Bethesda, MD, USA.
Funding support for the GWAS of Lung Cancer and Smoking was provided through the NIH Genes, Environment and Health Initiative (GEI) (Z01 CP 010200). The human subjects participating in the GWAS derive from The Environment and Genetics in Lung Cancer Etiology (EAGLE) case-control study and the Prostate, Lung Colon and Ovary Screening Trial and these studies are supported by intramural resources of the National Cancer Institute. Assistance with phenotype harmonization and genotype cleaning, as well as with general study coordination, was provided by the Gene Environment Association Studies, GENEVA Coordinating Center (U01HG004446). Assistance with data cleaning was provided by the National Center for Biotechnology Information. Funding support for genotyping, which was performed at the Johns Hopkins University Center for Inherited Disease Research, was provided by the NIH GEI (U01HG004438).
PLCO was also supported by the Intramural Research Program of the Division of Cancer Epidemiology and Genetics and by contracts from the Division of Cancer Prevention, National Cancer Institute, NIH, DHHS. The authors thank PLCO screening center investigators and staff, and the staff of Information Management Services Inc. and Westat Inc. Most importantly, we acknowledge trial participants for their contributions that made this study possible.
Acknowledgments
We thank all the participants of the PLCO Cancer Screening Trial. We also thank the National Cancer Institute for providing the access to the data collected by the PLCO trial. The statements contained herein are solely those of the authors and do not represent or imply concurrence or endorsement by National Cancer Institute. The authors would also like to acknowledge dbGaP repository for providing cancer genotyping datasets. The accession numbers for the datasets of lung cancer are phs000336.v1.p1 and phs000093.v2.p2. A list of contributing investigators and funding agencies for these studies can be found in the supplemental data.
Funding: QW was supported by the V Foundation for Cancer Research (D2017-19) and also partly supported by the Duke Cancer Institute as part of the P30 Cancer Center Support Grant (Grant ID: NIH/NCI CA014236). DQ was supported by the State Scholarship Fund, China Scholarship Council, the Ministry of Education of China through Fudan University Shanghai Cancer Center. The Harvard Lung Cancer Susceptibility Study was supported by NIH grants (CA092824, CA074386, CA090578) to DCC. SL was supported by National Institutes of Health (Grant ID: R01NS091307 and R56AG062302).
Footnote
Conflicts of Interest: All authors have completed the ICMJE uniform disclosure form (available at http://dx.doi.org/10.21037/tlcr-19-318). EFP reports personal fees from Grid Therapeutics, personal fees from OncoCyte, outside the submitted work. The other authors have no conflicts of interest to declare.
Ethical Statement: The authors are accountable for all aspects of the work in ensuring that questions related to the accuracy or integrity of any part of the work are appropriately investigated and resolved. The original studies were approved by the institutional review boards of the National Cancer Institute and Massachusetts General Hospital, respectively, with written informed consent obtained from each participant.
Open Access Statement: This is an Open Access article distributed in accordance with the Creative Commons Attribution-NonCommercial-NoDerivs 4.0 International License (CC BY-NC-ND 4.0), which permits the non-commercial replication and distribution of the article with the strict proviso that no changes or edits are made and the original work is properly cited (including links to both the formal publication through the relevant DOI and the license). See: https://creativecommons.org/licenses/by-nc-nd/4.0/.
References
- Bray F, Ferlay J, Soerjomataram I, et al. Global cancer statistics 2018: GLOBOCAN estimates of incidence and mortality worldwide for 36 cancers in 185 countries. CA Cancer J Clin 2018;68:394-424. [Crossref] [PubMed]
- Herbst RS, Morgensztern D, Boshoff C. The biology and management of non-small cell lung cancer. Nature 2018;553:446-54. [Crossref] [PubMed]
- Jia M, Zhu M, Zhou F, et al. Genetic variants of JNK and p38alpha pathways and risk of non-small cell lung cancer in an Eastern Chinese population. Int J Cancer 2017;140:807-17. [Crossref] [PubMed]
- Zienolddiny S, Skaug V. Single nucleotide polymorphisms as susceptibility, prognostic, and therapeutic markers of nonsmall cell lung cancer. Lung Cancer (Auckl) 2011;3:1-14. [PubMed]
- Stenzel-Bembenek A, Sagan D, Guz M, et al. Single nucleotide polymorphisms in lung cancer patients and cisplatin treatment. Postepy Hig Med Dosw (Online) 2014;68:1361-73. [Crossref] [PubMed]
- Thulin NK, Wang TT. The Role of Fc Gamma Receptors in Broad Protection against Influenza Viruses. Vaccines (Basel) 2018;6:36. [Crossref] [PubMed]
- Cassard L, Cohen-Solal J, Camilleri-Broet S, et al. Fc gamma receptors and cancer. Springer Semin Immunopathol 2006;28:321-8. [Crossref] [PubMed]
- Nimmerjahn F, Ravetch JV. Fcgamma receptors as regulators of immune responses. Nat Rev Immunol 2008;8:34-47. [Crossref] [PubMed]
- Pincetic A, Bournazos S, DiLillo DJ, et al. Type I and type II Fc receptors regulate innate and adaptive immunity. Nat Immunol 2014;15:707-16. [Crossref] [PubMed]
- Weiskopf K, Weissman IL. Macrophages are critical effectors of antibody therapies for cancer. MAbs 2015;7:303-10. [Crossref] [PubMed]
- Tamura K, Shimizu C, Hojo T, et al. FcgammaR2A and 3A polymorphisms predict clinical outcome of trastuzumab in both neoadjuvant and metastatic settings in patients with HER2-positive breast cancer. Ann Oncol 2011;22:1302-7. [Crossref] [PubMed]
- Wang DS, Wei XL, Wang ZQ, et al. FcgammaRIIA and IIIA polymorphisms predict clinical outcome of trastuzumab-treated metastatic gastric cancer. Onco Targets Ther 2017;10:5065-76. [Crossref] [PubMed]
- Shepshelovich D, Townsend AR, Espin-Garcia O, et al. Fc-gamma receptor polymorphisms, cetuximab therapy, and overall survival in the CCTG CO.20 trial of metastatic colorectal cancer. Cancer Med 2018;7:5478-87. [Crossref] [PubMed]
- Tryka KA, Hao L, Sturcke A, et al. NCBI's Database of Genotypes and Phenotypes: dbGaP. Nucleic Acids Res 2014;42:D975-9. [Crossref] [PubMed]
- Mailman MD, Feolo M, Jin Y, et al. The NCBI dbGaP database of genotypes and phenotypes. Nat Genet 2007;39:1181-6. [Crossref] [PubMed]
- Asomaning K, Miller DP, Liu G, et al. Second hand smoke, age of exposure and lung cancer risk. Lung Cancer 2008;61:13-20. [Crossref] [PubMed]
- Qian D, Liu H, Wang X, et al. Potentially functional genetic variants in the complement-related immunity gene-set are associated with non-small cell lung cancer survival. Int J Cancer 2019;144:1867-76. [Crossref] [PubMed]
- Lappalainen T, Sammeth M, Friedlander MR, et al. Transcriptome and genome sequencing uncovers functional variation in humans. Nature 2013;501:506-11. [Crossref] [PubMed]
- Consortium GT. Human genomics. The Genotype-Tissue Expression (GTEx) pilot analysis: multitissue gene regulation in humans. Science 2015;348:648-60. [Crossref] [PubMed]
- Cancer Genome Atlas Research N. Comprehensive molecular profiling of lung adenocarcinoma. Nature 2014;511:543-50. [Crossref] [PubMed]
- Aulchenko YS, Ripke S, Isaacs A, et al. GenABEL: an R library for genome-wide association analysis. Bioinformatics 2007;23:1294-6. [Crossref] [PubMed]
- Benjamini Y, Hochberg Y. Controlling the False Discovery Rate - a Practical and Powerful Approach to Multiple Testing. Journal of the Royal Statistical Society Series B-Statistical Methodology 1995;57:289-300.
- Wakefield J. A Bayesian measure of the probability of false discovery in genetic epidemiology studies. Am J Hum Genet 2007;81:208-27. [Crossref] [PubMed]
- Chambless LE, Diao G. Estimation of time-dependent area under the ROC curve for long-term risk prediction. Stat Med 2006;25:3474-86. [Crossref] [PubMed]
- Wang Y, Liu H, Ready NE, et al. Genetic variants in ABCG1 are associated with survival of nonsmall-cell lung cancer patients. Int J Cancer 2016;138:2592-601. [Crossref] [PubMed]
- Győrffy B, Surowiak P, Budczies J, et al. Online survival analysis software to assess the prognostic value of biomarkers using transcriptomic data in non-small-cell lung cancer. PLoS One 2013;8:e82241. [Crossref] [PubMed]
- Wilde JI, Watson SP. Regulation of phospholipase C gamma isoforms in haematopoietic cells: why one, not the other? Cell Signal 2001;13:691-701. [Crossref] [PubMed]
- Bae YS, Lee HY, Jung YS, et al. Phospholipase Cgamma in Toll-like receptor-mediated inflammation and innate immunity. Adv Biol Regul 2017;63:92-7. [Crossref] [PubMed]
- Wen R, Jou ST, Chen Y, et al. Phospholipase C gamma 2 is essential for specific functions of Fc epsilon R and Fc gamma R. J Immunol 2002;169:6743-52. [Crossref] [PubMed]
- Capietto AH, Kim S, Sanford DE, et al. Down-regulation of PLCgamma2-beta-catenin pathway promotes activation and expansion of myeloid-derived suppressor cells in cancer. J Exp Med 2013;210:2257-71. [Crossref] [PubMed]
- Ahn IE, Underbayev C, Albitar A, et al. Clonal evolution leading to ibrutinib resistance in chronic lymphocytic leukemia. Blood 2017;129:1469-79. [Crossref] [PubMed]
- Carman GM, Han GS. Phosphatidic acid phosphatase, a key enzyme in the regulation of lipid synthesis. J Biol Chem 2009;284:2593-7. [Crossref] [PubMed]
- Tang X, Benesch MG, Brindley DN. Lipid phosphate phosphatases and their roles in mammalian physiology and pathology. J Lipid Res 2015;56:2048-60. [Crossref] [PubMed]
- Duong CQ, Bared SM, Abu-Khader A, et al. Expression of the lysophospholipid receptor family and investigation of lysophospholipid-mediated responses in human macrophages. Biochim Biophys Acta 2004;1682:112-9. [Crossref] [PubMed]
- Brindley DN, Lin FT, Tigyi GJ. Role of the autotaxin-lysophosphatidate axis in cancer resistance to chemotherapy and radiotherapy. Biochim Biophys Acta 2013;1831:74-85. [Crossref] [PubMed]
- Tang X, Benesch MG, Dewald J, et al. Lipid phosphate phosphatase-1 expression in cancer cells attenuates tumor growth and metastasis in mice. J Lipid Res 2014;55:2389-400. [Crossref] [PubMed]
- Nakayama J, Raines TA, Lynch KR, et al. Decreased peritoneal ovarian cancer growth in mice lacking expression of lipid phosphate phosphohydrolase 1. PLoS One 2015;10:e0120071. [Crossref] [PubMed]