Predicting brain metastasis in early stage non-small cell lung cancer patients by gene expression profiling
Introduction
Lung cancer is the most common cause of cancer-death worldwide (1). Non-small cell lung cancer (NSCLC) comprised around 80% of the cases. Among NSCLC, adenocarcinoma is the most prevalent subtype, followed by squamous cell carcinoma. Lung cancer is notorious for dissemination to brain, occurring in up to 44% of non-resectable lung adenocarcinoma patients (2), or in up to 55% in patients with locally advanced NSCLC (3,4). A higher frequency is seen as patients’ survival increases (2). Among patients with brain metastases (BM), the most common origin of disease is the lungs (5,6). Adenocarcinoma histology and young age are correlated with higher risk of brain spread (4,7). Metastatic NSCLC patients with driver mutations commonly develop BM, possibly simply a reflection of the long-term control of systemic spread of disease accomplished by novel targeted agents. For example, more than 40% of patients harboring a driver rearrangement in the ALK gene develop BM within a year when treated with crizotinib, an ALK tyrosine kinase inhibitor (8). However, this rate drops to less than 10% if treated by alectinib, an inhibitor with better brain penetration. Identification of patients with a high risk of BM could allow closer monitoring and conceivably earlier detection of BM and more effective treatments.
The metastatic process is not a simple random spread of cells in the circulation with stochastic results. Rather, multiple complex molecular process are involved and can determine the pattern of spread to specific organs (9). Expression of relevant genes or activation of specific signaling pathways may endow cancer cells with properties that will facilitate metastatic seeding and growth in specific microenvironments. The concept of pre-metastatic niche has evolved to describe signaling originating from the primary tumor and impacting distant organs. Such signaling modifies the microenvironment at the distant site and promotes future attachment and growth of metastasis spread at that site (10). Identification of genes or molecular signaling correlated with early BM would provide insight into involved mechanisms and point to potential therapeutic targets.
Several genes or signaling pathways have been implicated in the risk of metastatic spread of lung cancer to the brain. The mRNA expression of N-cadherin (CDH2), kinesin family member C1 (KIFC1) and Fetal Alzheimer Antigen (FALZ1) in primary lung cancer tissues were reported to be associated with lung cancer BM (11). Another study demonstrated that the mTOR pathway, known to have a role in cancer progression, was correlated with brain metastasis in NSCLC patients; specifically, protein expression of p-mTOR, p-S6 and Rictor were more common in brain metastases compared to the primary NSCLC, and more common in NSCLC cases that developed brain metastasis (12). The PI3K/AKT pathway as well as JAK/STAT and CHK1 signaling have been implicated in brain metastasis of adenocarcinoma NSCLC based on a next-generation sequencing study (13). Focusing on PIK3CA, PTEN, AKT1, AKT2, and FRAP1, an association between specific single nucleotide polymorphisms and BM of NSCLC patients was reported (14). A mouse-human model of brain metastasis of lung cancer identified TWIST2 and SPOKE1 as regulators of this process (15). A study focused on the expression of a metastasis related long non-coding RNA, Metastasis associated lung adenocarcinoma transcript 1 (MALAT1), identified it as related to BM in NSCLC patients, possibly through epithelial-to-mesenchymal transition (16). Whole genome sequencing of a small set of primary NSCLC and their corresponding BM as well as circulating tumor cells identified mutations in the Keap1-Nrf2-ARE survival pathway as related to BM (17). We report here our results regarding identification of genes whose expression in primary resected NSCLC is associated with enhanced risk of early spread to the brain.
Methods
Specimens
Inclusion criteria were the surgical resection of NSCLC and the availability of a fresh-frozen specimen from the surgical specimen. We focused on patients that either developed brain metastasis after a potentially curative surgery or those with brain-metastasis-free follow up after such surgery. Exclusion criteria were neo-adjuvant chemotherapy or radiotherapy prior to surgery, sub-lobar surgical resection or positive surgical margins. Consecutive patients and their samples were identified and their specimens collected, initially from our local institutional medical bio-repository bank of Sheba Medical Center, Israel. Aiming to analyze a set of 90–100 appropriate samples, tumor specimens were also provided by the Ontario Tumor Bank (OTB, which is supported by the Ontario Institute for Cancer Research through funding provided by the Government of Ontario, Canada), and the Alberta Cancer Research Biobank, Canada. A consort diagram of the source of the samples included in this study is depicted in Figure S1. Tumor banks’ standard operating procedures (SOP) in all cases included securing informed consent from participating patients prior to surgery, and rapid procurement and freezing of resected specimen at the time of surgery. Specimen handling and clinical data collection performed was done according to tumor banks’ SOP.
Clinical data was collected from patients’ charts regarding Sheba tumor bank, or reported by the other tumor banks based on their clinical data collection procedures. Sites of metastasis data relates to sites known at presentation of metastatic disease or sites found to be involved at initial diagnostic evaluation. Patients with brain metastasis could have also metastasis to other sites. Ontario tumor bank recorded age at surgery within 4-year ranges, the middle figure of each range was entered into the study database. Staging was recorded according to the American Joint Committee on Cancer (AJCC) scales. Alberta tumor bank data was based on the 5th version, the rest of the tumor banks used the AJCC 7th version. Histology was categorized as adenocarcinoma, squamous cell carcinoma, and all other types [poorly differentiated, large cell neuroendocrine, non-otherwise specified (NOS)] were defined as NSCLC. Grading was based on the highest grade reported on the pathology. Undifferentiated carcinoma designation or the presence of necrosis were regarded as indicating the highest pathologic grade.
RNA extraction
Resected tumor specimens were snap-frozen and stored at −80 °C or liquid nitrogen. RNA was extracted using electric homogenizer and TRIzol reagent (Invitrogen, Life Technologies, Carlsbad, CA, USA) according to the manufacturer’s instructions. Total RNA quality and quantity were evaluated by Qubit fluorometric quantitation (Thermo Scientific, Wilmington, DE, USA) and by TapeStation 2200 (Agilent Technologies, Palo Alto, CA, USA).
RNA-sequencing (RNA-seq)
Oligo-dT enrichment was utilized prior to library preparation. Library preparation was done using TruSeq RNA v2 sample prep kit (Illumina) according to manufacturer’s instructions using random hexamer reverse transcription. RNA-sequencing was performed by Illumina HiSeq 2500, by 50 base pair single read runs, with a raw data Q-score threshold of 33. Reads were aligned and mapped to the human reference genome build GRCh37 (equivalent to hg19) using TopHat software, allowing for up to 3 mismatches per read. Gene counts were obtained by HTSeq-count package. Raw counts were normalized by DESeq2 package. RNA-seq and initial data analysis was performed by the Technion Genome Center (Haifa, Israel).
Data statistical analysis
Clinical parameters of the study participants were compared among the BM and Control groups, by t-test, Chi square and Fisher’s exact test as appropriate. Corrections were performed for multiple comparisons in part of the analysis as described below. Regarding parameters missing for some of the cohort, statistical tests were calculated for the available data.
Transcriptional differences between BM and Control groups were evaluated via one-way-anova. Selected genes were then used to discern between BM and Control patients, utilizing a Multivariate regularized logistic regression model. Sex, age, histology classification, stage and grade were used as covariates in all statistical tests. All computational analysis was conducted in R (version 3.4.0, www.r-project.org).
For visualization purposes, the transcriptional profiles of the patients were reduced to two dimensions using either Principal Component Analysis (PCA) or the t-SNE algorithm (18). Genes related to the genes incorporated in the principal components (PC) were tested for pathway enrichment by Ingenuity Knowledge Base Pathway Analysis tool.
Ethics
Patients from Sheba Medical Center whose specimens were included in the study provided informed consent for donating samples and data to the Sheba medical bio-repository bank (approval #2019-SMC). The study was approved by the local ethics committee at Sheba Medical Center (approval #9815-12-SMC). Similar procedures took place at the Ontario Tumor Bank and at the Alberta Cancer Research Biobank (i.e., informed consent from patients for donation of tissues and local ethics committee approval of the study).
Results
Study participants and samples
Samples were collected from 91 patients diagnosed with NSCLC, including BM patients (n=32) and control (n=59). RNA integrity for samples was very variable and ranged between 2 to 9. Only samples with RNA integrity number equivalent (RINe) values higher than 4.6 were used in the study. The average RINe for the cohort included in this study was 6.6. A mean of 18,940,269 sequences were obtained per sample (SD of 4,135,246), of those a mean of 87.3% were uniquely mapped per sample. A mean of 13,657,357 (SD of 3,023,498) uniquely mapped reads were assigned each to a feature exon in the human genome from each sample (after exclusion of a single sample with less than 1,800,000 reads).
The clinical characteristics of the included patients can be seen in Table 1. Median follow up was 40.4 months for the entire cohort, and median time to BM for the entire cohort was 92.5 months. For the control group, median follow up was 33.7 months (range, 6.4–149.0 months); 37 patients had 2 years or longer follow up, 7 with 1.5–2 years follow up and 6 with less than one year of follow up. For the BM group, median time to BM was 12.2 months (range, 0–73 months). Four of the included cases were diagnosed with BM at or earlier than the time of surgery. Of the study patients, 29 (32%) had metastatic disease at non-brain sites; this proportion and the distribution of metastatic sites did not differ significantly between the BM and the control group (Table 1).
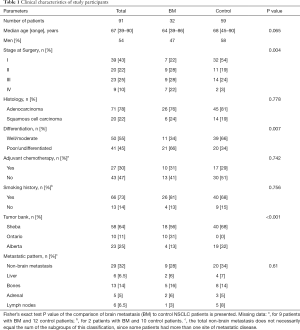
Full table
Clinical parameters as predictors of BM
Initially we were interested in examining the difference in each of the clinical parameters in patients with and without BM (Table 1). The stage at surgery distribution was significantly different between BM and control group (Fisher’s exact test P=0.004), where samples in the control group tend to have an earlier stage at surgery. Further, the grade of the tumor was significantly higher (P=0.007) in the BM group where most of the patients had poorly differentiated or undifferentiated tumors. By study design, all tumor samples received from the Ontario tumor bank were from patients with BM, comprising unbalanced distribution between the three included tumor banks.
Since both the stage and the grade were found to be significant in discerning between the control and BM patients, we used these parameters as well as sex, age and histological classification as covariates in a multivariate logistic regression model, in order to obtain the probability of developing BM for each individual. To explore the performance of our model, we performed Leave-One-Out-Cross-Validation (LOOCV) such that our model was fitted to the full cohort, excluding a single sample in each run. The coefficients of the resulting model were then applied to predict the score of the excluded sample. Based on this list of scores, we were able to distinguish BM from Control patients with 64.8% accuracy (95% CI: 54.1–74.6%), 81.4% specificity and 34.4% sensitivity.
Global RNA-seq signature is dominated by primary tumor characteristics
Broad transcriptional profile can reveal clinically relevant information and may enhance our ability to differentiate control from tumors that evolved BM. To that end, gene expression from primary tumor samples was analyzed. We then applied T-distributed stochastic neighbor embedding (t-SNE) to project the tumors on a two-dimensional map (Figure 1). By coloring the tumors BM vs. control, we could not observe a clear distinction (Figure 1A) but coloring based on histology revealed a clear-cut separation between adenocarcinoma and Squamous cell Carcinoma tumors (Figure 1B). Thus, the global gene expression profile predominantly represents the histological subtype of the primary tumor.
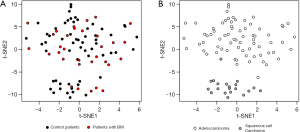
Gene selection for BM prediction analysis
We next focused on identifying a specific set of genes that will distinguish between BM and control patients. To establish such a gene set, in which differences in gene expression measurements among the BM and control groups are significant, we performed a one-way-anova test. The test was applied on each gene separately, utilizing the clinical parameters found significant as covariates. The null model of the F-test contained the clinical parameters age, sex, histology classification, grade and stage, whereas the alternative model also included information regarding the metastasis. A total of 101 genes were selected based on the F-test P values (P value threshold chosen to include about a 100-gene to prevent overfitting of the data). A multivariate regularized logistic regression model was then applied with the set of 101 genes and clinical parameters. We tested our performance as described above using LOOCV and were able to differentiate BM from control patients with 89.0% accuracy (95% CI: 80.7–94.6%), 94.9% specificity and 78.1% sensitivity. For comparison, using the same model with a random selection of 101 genes would provide poor results (Figure 2A).
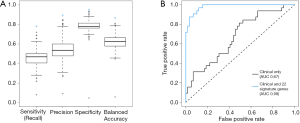
We next aimed to reduce the number of genes employed in our model to provide a robust set that could be used in the future for clinical diagnostics. As described above, we fitted the multivariate regularized logistic regression model 91 times, where each time one sample was omitted. Thus, for each of these models we obtained a vector of coefficients (one for each gene) that can be used as indications for the importance of the genes in correctly classifying the sample. Since a regularized model was applied, this vector was sparse, thus providing a more interpretable model and minimizing the risk of overfitting the model.
To minimize our set of genes, we explored the matrix of coefficients and observed that only 32 of the genes had a non-zero coefficient in at least one of the 91 models. Moreover, only 22 genes had a non-zero coefficient in 80% of the patients (Table 2). We evaluated the performance of using this set of 22 genes along with the clinical parameters in the same manner, and achieved 93.4% accuracy (95% CI: 86.2–97.5), 96.6% specificity and 87.5% sensitivity. Figure 2B demonstrates the superiority of a model with these 22 genes compared to a model which contained only clinical parameters.
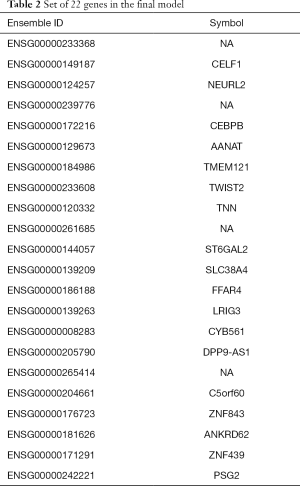
Full table
Gene signature specificity to BM prediction
We next asked whether the signature is specific for developing BM rather than metastasis in other sites. To that end, we compared the probabilities of individuals to develop brain metastasis, based on the expression of our set of 22 genes, between patients without metastasis, patients with metastasis in other sites, and patients who developed BM. No difference was found between the group of patients without metastasis and the patients who developed metastasis in other sites (Wilcoxon rank-sum test, P=0.97, Figure 3), while a significant difference in the distribution was found between patients that developed brain metastasis and the rest of the patients (Wilcoxon rank-sum test, P=4E-14, Figure 3). Similar results were achieved when considering the signature specificity for developing BM as oppose to recurrence of the disease (data not shown). Together, this indicates the specificity of the signature for the development of BM.
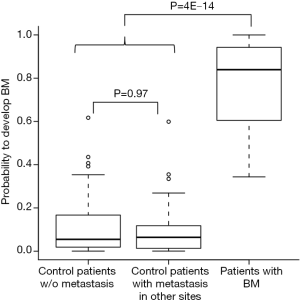
The oxidative phosphorylation pathway is associated with metastatic spread to the brain
Finally, to gain more insight into the signaling pathways involved in the metastatic spread to the brain, we wished to identify the canonical pathways most enriched for genes that were highly correlated to the signature of 22 genes we have found. In order to reduce dimensionality and visualize the similarity between the patients’ samples based on these genes we performed PCA using our signature gene list (Figure 4A). As expected, this PCA plot can be used to discern control from BM patients. Then, we investigated the enrichment of top 0.5% genes that are correlated to each of these PCs via the Ingenuity Knowledge Base Pathway Analysis tool (QIAGEN).
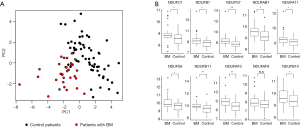
Interestingly, the oxidative phosphorylation pathway, and more specifically, the respiratory chain complex I, was highly significant (Hyper-Geometric test, P=8.74E-7) showing upregulation in primary NSCLC tumors that spread to the brain (Figure 4B).
Discussion
In this study, we have introduced a bioinformatics approach which utilizes a gene expression signature found in primary tumors, in combination with clinical parameters, to predict a development of BM. This signature was linked specifically to BM, and was not associated with the development of metastasis to other sites or simply to recurrence of disease. Leave-one-out validation procedure demonstrated a promising predictive value. If validated further, identifying patients at a higher risk for BM development may allow personalized follow-up focused on the brain and better treatment options for these patients. Importantly, from the genes in this signature, we found the oxidative phosphorylation pathway as strongly associated with the risk of BM. This result has a potential to direct novel approaches to understanding, and in the future possibly preventing, the molecular events at the basis of BM development. Interestingly, oxidative phosphorylation has recently been reported as a driver for various cancers (19,20), including lung cancer (21), and novel inhibitors of this pathway are currently being evaluated in early phase clinical studies (22-24).
Variations in metabolic patterns have been reported in the past to associate with brain tumors or brain metastasis. Primary brain malignancies as well as brain metastases of various cancers (including lung cancer) were found to utilize common metabolic pathways (25,26), possibly a reflection of the common unique microenvironment of the brain. In these tumors, glucose was found to be mostly oxidized in the citric acid cycle/oxidative phosphorylation, the more efficient metabolic pathway in comparison to glycolysis (25). Labeled glucose studies in mouse models of primary and metastatic brain tumors also indicate utilization of the citric acid cycle by such tumors, probably alongside the glycolytic pathway (27). The glycolytic pathway is known to be in general preferentially activated in tumors compared to oxidative phosphorylation, a phenomenon known as the Warburg effect. Glycolysis is an inefficient way to produce energy from glucose. Its use by cancer cells is presumed to reflect a requirement of these fast growing cells for precursors of nucleotides, amino acids and lipids genesis, available from glycolysis intermediates (28). Enhanced glycolysis and reduced oxidative phosphorylation in tumors was correlated with worse outcome for brain cancer patients (29). However, the overarching scope of the Warburg effect in cancer has been questioned by more recent observations.
Lung cancer cells grown in culture demonstrate the Warburg effect of reduced oxidative phosphorylation; however, in mice models of lung cancer, glucose is utilized more in the citric acid cycle (30). Human NSCLC was demonstrated to harbor enhanced levels of oxidative phosphorylation relative to neighboring normal lungs (31). A higher fraction of non-proliferating cells in real tumors compared to culture studies might be one explanation for these differences, stressing the potential importance of oxidative phosphorylation in cancer. In addition, different utilization of oxidative phosphorylation vs. the glycolytic pathway might exist in different cell types within brain tumors and metastases. Insulin like growth factor 2 mRNA binding protein (IGF2BP2, also called IMP2) was found to enhance oxidative phosphorylation and be essential for the survival of brain tumor stem cell population (32). Correlation of the activation of oxidative phosphorylation with stem cell-like self-renewal properties (32) further strength the link of this signaling pathway to metastatic spread. However, insight into the correlation between oxidative phosphorylation and metastatic spread specifically to the brain requires further studies.
Oxidative phosphorylation has been found as signaling pathway common to breast cancer metastasis to the brain and to primary brain tumors based on RNA expression array studies (33). Proteomic analysis of a mouse model for breast cancer metastasis to brain identified several components of the respiratory chain as correlated with brain metastasis, in addition to enhanced glycolytic pathway (34). Alterations in mitochondrial genes coding for oxidative phosphorylation proteins were found to correlate with brain metastasis of breast cancers (35). A more recent study identified oxidative phosphorylation as the most active metabolic pathway in brain metastasis of melanoma in several mice models, as well as in samples of human melanoma brain metastasis vs. patient-matched extra-cranial metastases (36). Importantly, an inhibitor of mitochondrial complex I reduced BM in a melanoma mouse model. Thus, the correlation we have found between enhanced oxidative phosphorylation and brain metastasis is in accordance with some of the previous studies despite not being in accordance with the Warburg hypothesis. Other, unknown as of yet genomic or microenvironmental factors may determine the metabolic pathway required for the development of BM from specific organs and cancers.
A limitation of this study is the lack of a separate validation cohort. However, interval validation by a leave-one-out procedure provides initial support of the potential value of our findings. Another limitation regarding any potential future clinical implementation of a gene signature as the one we have reported, is the requirement to overcome technical issues of quantifying these genes using clinical routine tumor samples. Recent advances in the field of mRNA quantifying from formalin-fixed paraffin-embedded samples are expected to facilitate clinical utilization of such gene expression signatures (37,38). Immunohistochemistry quantification of relevant proteins can constitute another approach for validation of our results, although entails additional technical challenges and has not been performed in this study.
Conclusions
The size of the cohort included in our study may be regarded as small, but is the largest set to our knowledge of RNA-sequencing data associated with detailed metastatic site and timing data. Further validation studies and mechanistic evaluation of the role of oxidative phosphorylation in the process of metastatic spread to the brain are required.
Acknowledgments
Funding: This work was supported by the Chief Scientist Office of the Ministry of Industry and Commerce of Israel (‘Nofar’ grant #48339), by the Center of Excellence grant from Flight Attendant Medical Research Institute (FAMRI), by the Israel Cancer Association through ICA-USA Board of Directors and by Yemini Family Foundation. The work of IG-V was supported by the Israel Science Foundation Grant 288/16 (YS and IG-V). Partial fellowships were from the European Research Council (637885), the Edmond J. Safra Center for Bioinformatics at Tel Aviv University (YS), and a Shulamit Aloni Scholarship, Ministry of Science and Technology, Israeli government (YS).
Footnote
Conflicts of Interest: All authors have completed the ICMJE uniform disclosure form (available at http://dx.doi.org/10.21037/tlcr-19-477). IK has a patent Gene signature prognostic of brain metastasis in NSCLC. 62/879,716 pending. ID has a patent Gene signature prognostic of brain metastasis in NSCLC. 62/879,716 pending. AO reports and honoraria from BI, MSD, Roche, AstraZeneca in Israel. JB has a patent Gene signature prognostic of brain metastasis in NSCLC. 62/879,716 pending and consultant fees from Roche, Boehringer Ingelheim, Novartis, BMS, Pfizer, AstraZeneca, Takada, MSD, VBL, Abbvie, Bayer, Lilly, grant support (to the institute) from MSD, Boehringer Ingelheim, AstraZeneca, Pfizer, Roche, Abbvie, BMS, Takeda. The other authors have no conflicts of interest to declare.
Ethical Statement: The authors are accountable for all aspects of the work in ensuring that questions related to the accuracy or integrity of any part of the work are appropriately investigated and resolved. The study was conducted in accordance with the Declaration of Helsinki (as revised in 2013). Patients from Sheba Medical Center whose specimens were included in the study provided informed consent for donating samples and data to the Sheba medical bio-repository bank (approval #2019-SMC). The study was approved by the local ethics committee at Sheba Medical Center (approval #9815-12-SMC). Similar procedures took place at the Ontario Tumor Bank and at the Alberta Cancer Research Biobank (i.e., informed consent from patients for donation of tissues and local ethics committee approval of the study).
Open Access Statement: This is an Open Access article distributed in accordance with the Creative Commons Attribution-NonCommercial-NoDerivs 4.0 International License (CC BY-NC-ND 4.0), which permits the non-commercial replication and distribution of the article with the strict proviso that no changes or edits are made and the original work is properly cited (including links to both the formal publication through the relevant DOI and the license). See: https://creativecommons.org/licenses/by-nc-nd/4.0/.
References
- Jemal A, Bray F, Center MM, et al. Global Cancer Statistics: 2011. CA Cancer J Clin 2011;61:69-90. [Crossref] [PubMed]
- Sørensen JB, Hansen HH, Hansen M, et al. Brain metastases in adenocarcinoma of the lung: frequency, risk groups, and prognosis. J Clin Oncol 1988;6:1474-80. [Crossref] [PubMed]
- Gore EM, Bae K, Wong SJ, et al. Phase III comparison of prophylactic cranial irradiation versus observation in patients with locally advanced non-small-cell lung cancer: primary analysis of radiation therapy oncology group study RTOG 0214. J Clin Oncol 2011;29:272-8. [Crossref] [PubMed]
- Chen AM, Jahan TM, Jablons DM, et al. Risk of cerebral metastases and neurological death after pathological complete response to neoadjuvant therapy for locally advanced nonsmall-cell lung cancer. Cancer 2007;109:1668-75. [Crossref] [PubMed]
- Sperduto PW, Chao ST, Sneed PK, et al. Diagnosis-specific prognostic factors, indexes, and treatment outcomes for patients with newly diagnosed brain metastases: a multi-institutional analysis of 4,259 patients. Int J Radiat Oncol Biol Phys 2010;77:655-61. [Crossref] [PubMed]
- Villano JL, Durbin EB, Normandeau C, et al. Incidence of brain metastasis at initial presentation of lung cancer. Neuro Oncol 2015;17:122-8. [Crossref] [PubMed]
- Bajard A, Westeel V, Dubiez A, et al. Multivariate analysis of factors predictive of brain metastases in localised non-small cell lung carcinoma. Lung Cancer 2004;45:317-23. [Crossref] [PubMed]
- Peters S, Camidge DR, Shaw AT, et al. Alectinib versus crizotinib in untreated ALK-positive non-small-cell lung cancer. N Engl J Med 2017;377:829-38. [Crossref] [PubMed]
- Obenauf AC, Massagué J. Surviving at a Distance: Organ-Specific Metastasis. Trends in Cancer 2015;1:76-91. [Crossref] [PubMed]
- Peinado H, Zhang H, Matei IR, et al. Pre-metastatic niches: Organ-specific homes for metastases. Nature Reviews Cancer 2017;17:302-17. [Crossref] [PubMed]
- Grinberg-Rashi H, Ofek E, Perelman M, et al. The Expression of Three Genes in Primary Non-Small Cell Lung Cancer Is Associated with Metastatic Spread to the Brain. Clin Cancer Res 2009;15:1755-61. [Crossref] [PubMed]
- Krencz I, Sebestyén A, Fábián K, et al. Expression of mTORC1/2-related proteins in primary and brain metastatic lung adenocarcinoma. Hum Pathol 2017;62:66-73. [Crossref] [PubMed]
- Hauck CR, Thibodeau BJ, Ahmed S, et al. Targeted DNA Sequencing of Non-small Cell Lung Cancer Identifies Mutations Associated With Brain Metastases. Int J Radiat Oncol 2017;99:s199-200. [Crossref]
- Li Q, Yang J, Yu Q, et al. Associations between single-nucleotide polymorphisms in the PI3K-PTEN-AKT-mTOR pathway and increased risk of brain metastasis in patients with non-small cell lung cancer. Clin Cancer Res 2013;19:6252-60. [Crossref] [PubMed]
- Singh M, Venugopal C, Tokar T, et al. RNAi screen identifies essential regulators of human brain metastasis-initiating cells. Acta Neuropathol 2017;134:923-40. [Crossref] [PubMed]
- Shen L, Chen L, Wang Y, et al. Long noncoding RNA MALAT1 promotes brain metastasis by inducing epithelial-mesenchymal transition in lung cancer. J Neurooncol 2015;121:101-8. [Crossref] [PubMed]
- Aljohani HM, Aittaleb M, Furgason JM, et al. Genetic mutations associated with lung cancer metastasis to the brain. Mutagenesis 2018;33:137-45. [Crossref] [PubMed]
- Laurens van der Maaten. Geoffrey Hinton. Visualizing Data using t-SNE. J Mach Learn Res 2008;9:2579-605.
- Jonsson M, Fjeldbo CS, Holm R, et al. Mitochondrial Function of CKS2 Oncoprotein Links Oxidative Phosphorylation with Cell Division in Chemoradioresistant Cervical Cancer. Neoplasia 2019;21:353-62. [Crossref] [PubMed]
- Schöckel L, Glasauer A, Basit F, et al. Targeting mitochondrial complex I using BAY 87-2243 reduces melanoma tumor growth. Cancer Metab 2015;3:11. [Crossref] [PubMed]
- Rao S, Mondragón L, Pranjic B, et al. AIF-regulated oxidative phosphorylation supports lung cancer development. Cell Res 2019;29:579-91. [Crossref] [PubMed]
- Echeverria GV, Ge Z, Seth S, et al. Resistance to neoadjuvant chemotherapy in triple-negative breast cancer mediated by a reversible drug-tolerant state. Sci Transl Med 2019;11:eaav0936.
- Shi Y, Lim SK, Liang Q, et al. Gboxin is an oxidative phosphorylation inhibitor that targets glioblastoma. Nature 2019;567:341-6. [Crossref] [PubMed]
- Molina JR, Sun Y, Protopopova M, et al. An inhibitor of oxidative phosphorylation exploits cancer vulnerability. Nat Med 2018;24:1036-46. [Crossref] [PubMed]
- Mashimo T, Pichumani K, Vemireddy V, et al. Acetate is a bioenergetic substrate for human glioblastoma and brain metastases. Cell 2014;159:1603-14. [Crossref] [PubMed]
- Maher EA, Marin-Valencia I, Bachoo RM, et al. Metabolism of [U-13C]glucose in human brain tumors in vivo. NMR Biomed 2012;25:1234-44. [Crossref] [PubMed]
- Marin-Valencia I, Cho SK, Rakheja D, et al. Glucose metabolism via the pentose phosphate pathway, glycolysis and Krebs cycle in an orthotopic mouse model of human brain tumors. NMR Biomed 2012;25:1177-86. [Crossref] [PubMed]
- Vander Heiden MG, Cantley LC, Thompson CB. Understanding the warburg effect: The metabolic requirements of cell proliferation. Science 2009;324:1029-33. [Crossref] [PubMed]
- Li X, Jiang Y, Meisenhelder J, et al. Mitochondria-Translocated PGK1 Functions as a Protein Kinase to Coordinate Glycolysis and the TCA Cycle in Tumorigenesis. Mol Cell 2016;61:705-19. [Crossref] [PubMed]
- Davidson SM, Papagiannakopoulos T, Olenchock BA, et al. Environment impacts the metabolic dependencies of ras-driven non-small cell lung cancer. Cell Metab 2016;23:517-28. [Crossref] [PubMed]
- Hensley CT, Faubert B, Yuan Q, et al. Metabolic Heterogeneity in Human Lung Tumors. Cell 2016;164:681-94. [Crossref] [PubMed]
- Janiszewska M, Suvà ML, Riggi N, et al. Imp2 controls oxidative phosphorylation and is crucial for preservin glioblastoma cancer stem cells. Genes Dev 2012;26:1926-44. [Crossref] [PubMed]
- Schulten HJ, Bangash M, Karim S, et al. Comprehensive molecular biomarker identification in breast cancer brain metastases. J Transl Med 2017;15:269. [Crossref] [PubMed]
- Chen EI, Hewel J, Krueger JS, et al. Adaptation of energy metabolism in breast cancer brain metastases. Cancer Res 2007;67:1472-86. [Crossref] [PubMed]
- McGeehan RE, Cockram LA, Littlewood DTJ, et al. Deep sequencing reveals the mitochondrial DNA variation landscapes of breast-to-brain metastasis blood samples. Mitochondrial DNA A DNA Mapp Seq Anal 2018;29:703-13. [Crossref] [PubMed]
- Fischer GM, Jalali A, Kircher DA, et al. Molecular profiling reveals unique immune and metabolic features of melanoma brain metastases. Cancer Discov 2019;9:628-45. [Crossref] [PubMed]
- Koshkin VS, Garcia JA, Reynolds J, et al. Transcriptomic and protein analysis of small-cell bladder cancer (SCBC) Identifies prognostic biomarkers and DLL3 as a relevant therapeutic target. Clin Cancer Res 2019;25:210-21. [Crossref] [PubMed]
- Wang L, Saci A, Szabo PM, et al. EMT- and stroma-related gene expression and resistance to PD-1 blockade in urothelial cancer. Nat Commun 2018;9:3503. [Crossref] [PubMed]