Development and validation of a preoperative noninvasive predictive model based on circular tumor DNA for lymph node metastasis in resectable non-small cell lung cancer
Introduction
Lung cancer is one of the most prevalent and deadly cancers in the world (1), with non-small cell lung cancer (NSCLC) constituting approximately 80% of the diagnosed cases. The eighth edition TNM staging system is currently the most widely used prognosis tool and primary treatment determinant (2). Regional lymph node involvement is a core aspect of TNM staging. The 5-year survival rates are in descending order for clinical N0, N1 and N2 patients as 37%, 23% and 9% respectively. Moreover, primary treatment strategy differs depending on clinical N stage. For resectable NSCLC without mediastinal lymph node involvement, surgical resection remains as the primary option. However, for NSCLC patients with mediastinal lymph node involvement, the National Comprehensive Cancer Network (NCCN) guideline recommends definitive concurrent chemoradiation or induction chemotherapy as the primary treatment (3).
Chest computed tomography and positron emission tomography/computed tomography are usually the first steps of preoperative lymph node staging. For resectable NSCLC patients with negative mediastinal lymph node results, surgical resection may proceed. Meanwhile for positive results, further pathological confirmation by invasive techniques is required (3,4). These include endoscopy needle biopsy, mediastinoscopic lymph node evaluation, and sometimes even video-assisted thoracic surgery for lymph node evaluation. Unfortunately, certain number of patients are wrongfully proceeded to surgical resection due to the occasional false negative results in radiological examination. Furthermore, these invasive techniques, though with proven sensitivity and specificity, are accompanied with the risk of potentially severe complications and patients’ discomfort. Therefore, the demand of noninvasive tool for preoperative lymph node metastasis prediction remains significant.
Circular tumor DNA (ctDNA), referring to the tumoral DNA fragment detected in patients’ plasma, has been widely used for the diagnosis of driver mutations in advanced stage NSCLC (5). Several pioneering studies have demonstrated the feasibility and potential application of ctDNA in the screening and detection of postoperative minimal residual disease in early stage NSCLC (6-9). A comprehensive review and data analysis of these studies has revealed variant allele frequency (VAF) to be closely correlated with the stage of NSCLC, as more advanced stage patients have higher level VAF (10). Moreover, preoperative ctDNA analysis of TRACERx cohort [Tracking Non-Small-Cell Lung Cancer Evolution through Therapy (Rx)] has demonstrated that larger tumor size is associated with higher VAF level (6,11). Therefore, VAF may be a tumor burden indicator and potentially useful in preoperative lymph node metastasis prediction in NSCLC patients.
To the best of our knowledge, no previous study has applied preoperative ctDNA in NSCLC lymph node metastasis prediction, and the relationship between VAF and lymph node metastasis remains unclear. In this study, we utilized the publicly available data of the TRACERx study (6,11), and we aimed to build a preoperative noninvasive predictive model for the lymph node metastasis of resectable NSCLC based on ctDNA. Furthermore, we used a separate cohort from our hospital to externally validate the prediction model. We present the following article in accordance with the STROBE reporting checklist (available at http://dx.doi.org/10.21037/tlcr-20-593).
Methods
Study design
Related clinicopathological factors and single nucleotide variation data were extracted from the publicly available baseline preoperative cohort of the TRACERx study (6,11). The primary outcome in this study was pathological lymph node metastasis, which was evaluated with systemic lymph node sampling or dissection during surgery. We aimed to develop a prediction model based on ctDNA and preoperative noninvasively accessible clinical factors. The discrimination and calibration of the model were evaluated and the model underwent external validation by a similar cohort from our hospital.
Study population
The selection criteria of the TRACERx group was described previously (6,11) and summarized as follows: (I) resectable stage I–IIIA NSCLC adult patients; (II) patients received preoperative ctDNA test and at least one single-nucleotide variants (SNV) was detected; (III) patients underwent primary tumor resection and intraoperative systemic lymph node sampling or dissection; (IV) patients received any anti-cancer neoadjuvant therapy were excluded; (V) patients with any other current malignancy or previous malignancy diagnosed or relapsed within the recent 5 years were excluded. A similar cohort was retrospectively identified in our hospital and used as the external validation group. Overall, 58 patients from TRACERx cohort were selected into the training group, and 37 patients from our hospital were selected into the external validation group from 2018.7.1 to 2019.8.31 (Figure 1). This study was conducted in accordance with the Declaration of Helsinki and was approved by our hospital institutional review board, and informed consents were acquired from all participants. All data in this study was deposited in our hospital research data management committee website (RDDA2020001480) and could be accessed upon request.
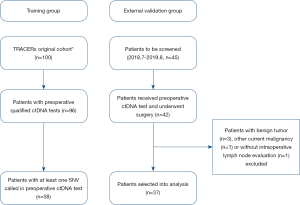
ctDNA test
The preoperative ctDNA test method of the TRACERx study was described previously (6), and could be briefly reviewed as the following procedures: (I) cfDNA extraction from plasma; (II) cfDNA library construction with the Natera Library Prep kit; (III) SNV assay optimization and target enrichment; (IV) Sequencing with Illumina HiSeq 2500. On the other hand, the ctDNA test of the external validation group was performed with the 150 genes panel acquired from 3D Medicines, Inc. (Shanghai, China, Table S1). The testing procedure was summarized as follows: (I) collect 8–10 mL venous blood into EDTA tube and centrifuge to isolate the plasma within 4 hours; (II) cell free DNA (cfDNA) was extracted from the plasma with QIAamp Circulating Nucleic Acid kit (Qiagen) following the standard procedure, and the cfDNA was quantified by Qubit dsDNA HS Assay Kit (Life Technologies); (III) cfDNA libraries was established by Accel-NGS 2S Plus DNA Library Kit (SWIFT) with barcoding to tag individual cfDNA fragment following the manufacture manual; (IV) target enrichment was performed with 3DMed 150-Gen Lockdown Probe Kit following the standard procedure; (V) the captured libraries were sequenced with NextSeq500 (Illumina) following the manufacture manual. Called mutations with high VAF (>0.2) were excluded as they are likely to be germline mutations.
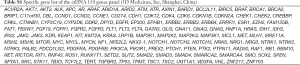
Full table
Statistical analysis
Discrete variables were presented with count and percentage, their difference between groups was compared with Pearson chi-square test (all expected values no less than 5) or Fisher’s exact test (any expected values less than 5). Continuous variables were described with median and interquartile range and their difference between groups was compared with Mann-Whitney-Wilcoxon test. The mean of VAF (meanVAF) was calculated as the sum of all variant allele frequency (VAF) divided by the number of mutations within the same patient. Continuous variables were further transformed into binary variables with the optimal cutoff to facilitate model development and clinical appliance.
Preoperative noninvasively accessible factors were entered simultaneously in multivariate logistic regression analysis, and potential statistical significant factors (P<0.15) from the multivariate analysis were further included in the development of the nomogram. The nomogram was developed with “rms” package, which contained many useful functions of model development and visualization (12,13). The discriminative power was evaluated with area under the receiver operating characteristic curve (AUC). The range of AUC was 0.5–1.0, with 0.5 indicating random prediction and 1.0 indicating perfect prediction. The optimal sensitivity and specificity were determined by Youden Index. The model was calibrated and evaluated with Hosmer-Lemeshow test. The model was internally validated with Bootstrap resampling and externally validated with a similar cohort from our hospital. A two-sided P value <0.05 was considered statistically significant. All statistical analysis was conducted by IBM SPSS statistics version 25 and R version 3.6.1.
Results
Study population and related clinicopathological factors
The training group comprised 58 patients from TRACERx cohort, and 37 patients from our hospital were selected into external validation group as shown by the selection flow (Figure 1). Lymph node metastasis occurred in 17 (29.3%) patients in the training group and 7 (18.9%) patients in the external validation group. All related clinicopathological factors and meanVAF of the both groups were listed in Table 1.
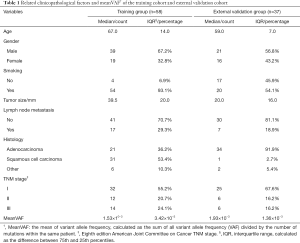
Full table
VAF comparison between patients with and without lymph node metastasis
The overall VAF distribution of the training group was shown in the boxplot and histogram (Figure 2A,B). The meanVAF was calculated within each patient, and when grouped by the status of the lymph node metastasis, there was a clear tendency that lymph node metastasis was associated with higher meanVAF (Figure 2C,D).
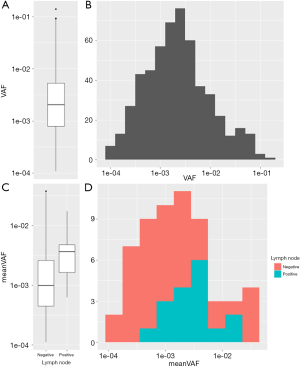
Development of the predictive model for lymph node metastasis
In the univariate and multivariate analysis of preoperative noninvasively accessible variables, higher meanVAF level was significantly associated with lymph node metastasis (OR: 4.89, 95% CI: 1.22–19.54, P=0.03, Table 2). In addition, younger age at the time of diagnosis and larger tumor size were potentially associated with lymph node metastasis (P<0.15, Table 2). These 3 variables were further included to develop the predictive nomogram (Figure 3). In the nomogram, each subcategory within the variable was assigned a point by the point scale at top. Locate the sum of these points on the total point scale and the predicted lymph node metastasis probability can be found perpendicularly below. The AUC of the nomogram was 0.77 (95% CI: 0.65–0.90, P=0.001, Figure 4A) with the optimal sensitivity and specificity as 70.6% and 73.1% respectively, indicating satisfactory discriminative power. The nomogram was internally validated by bootstrap resampling and shown to be well calibrated, demonstrating fine agreement between predicted probability and actual probablity (Figure 4B, Hosmer-Lemeshow test: P=0.88).
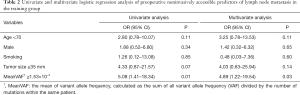
Full table
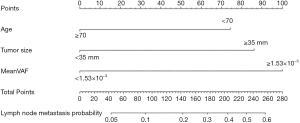
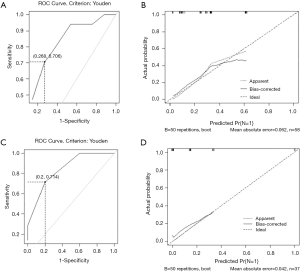
External validation of the predictive model
In the external validation group, the prediction model also demonstrated satisfactory discriminative power with an AUC of 0.84 (95% CI: 0.70–0.99, P=0.005, Figure 4C). And the optimal sensitivity and specificity were 71.4% and 80.0% respectively. Moreover, the calibration plot in the external validation group demonstrated fine agreement between the predicted probability and actual probability (Figure 4D, Hosmer-Lemeshow test: P=0.99).
Discussion
In this study, VAF level was discovered to be significantly associated with lymph node metastasis in resectable NSCLC patients. Previous studies had found a close association between VAF level and tumor burden in NSCLC patients, as higher level VAF was correlated with larger tumor size and more advanced stage (6,10). However, to the best of our knowledge, the specific relationship between VAF level and lymph node metastasis had never been clarified. We believe our finding is consistent with the previous perspective that higher VAF level is associated with higher tumor burden. And compared to NSCLC patients without lymph node metastasis, patients with lymph node metastasis can certainly have higher tumor burden. Therefore, VAF level can be a useful indicator for the lymph node metastasis and provide valuable information for preoperative clinical N staging.
Moreover, we built a lymph node metastasis predictive model based on this finding from ctDNA testing and other preoperative noninvasively accessible clinical factors (namely, age and tumor size). Previous studies had found that younger age at NSCLC diagnosis was more likely to be associated with lymph node metastasis, as their tumors were more aggressive and their diagnosis were often delayed (14,15). In addition, larger tumor size was well documented to have an association with lymph node metastasis (16-18). Thus, the final predictive model incorporated age, tumor size and VAF level.
The model demonstrated good discriminative power and calibration in both training group and extenal validation group. Actually, multiple lymph node metastasis prediction models with similar performance had been built previously (17-19). However, it should be noted that these models were based on postoperative pathological or genomic characteristics, therefore were limited in preoperative lymph node metastasis prediction. On the contrary, all predictors in our models can be acquired noninvasively before surgery, and we believe this predictive model can help improve the accuracy of preoperative clinical N staging.
Our study further validates the application prospect of ctDNA as a useful technique for preoperative lymph node metastasis prediction. And it should be noted that the full potential of ctDNA has yet to be unlocked in clinical practice. Early cancer detection via a simple blood draw has always been the final prize of ctDNA, which specifically includes early cancer screening and early postoperative minimal residual disease detection. This is no longer a fantasy as several pioneering studies have made great progress in advancing the ctDNA techniques (6-9). We believe the full potential of ctDNA as a noninvasive technique will soon be realized in the near future.
Several limitations are worth mentioning in this study. First, our predictive model lacked the capacity to further differentiate clinical N1 and N2 stage. We believe this limitation was caused by not only the limited discriminative power of included predictors, but also the limited sample size, especially the number of positive N2 patients. Second, the radiological characteristics of the lymph node, which were likely to be predictive, were not available in either training group or external validation group. Third, the concordance between ctDNA and primary tumor tissues whole-exome sequencing was less than ideal (20), as the tumor burden in resectable NSCLC was relatively small, and the trace amount of tumor DNA released into the plasma was technically challenging to be all correctly detected without missing. Fourth, this study was subjected to potential bias due to its retrospective nature. Therefore, future efforts should be directed toward prospective trial with larger sample size, improved technique and comprehensive evaluation.
In conclusion, the VAF level in ctDNA was demonstrated to be a useful indicator for the NSCLC lymph node metastasis. And a preoperative noninvasive predictive model based on ctDNA for the lymph node metastasis in resectable NSCLC patients was developed and externally validated with satisfactory discriminatory ability and calibration.
Acknowledgments
The authors sincerely thank the researchers of the TRACERx study for their pioneering work and making their data publicly available.
Funding: This work was funded by grant from Major R&D projects of State Ministry of Science and Technology (2016YFC0905402).
Footnote
Reporting Checklist: The authors have completed the STROBE reporting checklist. Available at http://dx.doi.org/10.21037/tlcr-20-593
Data Sharing Statement: Available at http://dx.doi.org/10.21037/tlcr-20-593
Conflicts of Interest: All authors have completed the ICMJE uniform disclosure form (available at http://dx.doi.org/10.21037/tlcr-20-593). The authors have no conflicts of interest to declare.
Ethical Statement: The authors are accountable for all aspects of the work in ensuring that questions related to the accuracy or integrity of any part of the work are appropriately investigated and resolved. This study was conducted in accordance with the Declaration of Helsinki and was approved by our hospital institutional review board, and informed consents were acquired from all participants.
Open Access Statement: This is an Open Access article distributed in accordance with the Creative Commons Attribution-NonCommercial-NoDerivs 4.0 International License (CC BY-NC-ND 4.0), which permits the non-commercial replication and distribution of the article with the strict proviso that no changes or edits are made and the original work is properly cited (including links to both the formal publication through the relevant DOI and the license). See: https://creativecommons.org/licenses/by-nc-nd/4.0/.
References
- Bray F, Ferlay J, Soerjomataram I, et al. Global cancer statistics 2018: GLOBOCAN estimates of incidence and mortality worldwide for 36 cancers in 185 countries. CA Cancer J Clin 2018;68:394-424. [Crossref] [PubMed]
- Yun JK, Lee GD, Kim HR, et al. Validation of the 8th edition of the TNM staging system in 3,950 patients with surgically resected non-small cell lung cancer. J Thorac Dis 2019;11:2955-64.
- National Comprehensive Cancer Network. Non-small Cell Lung Cancer (Version 3 2020). Available online: https://www.nccn.org/professionals/physician_gls/pdf/nscl.pdf. Accessed March 3, 2020.
- De Leyn P, Dooms C, Kuzdzal J, et al. Revised ESTS guidelines for preoperative mediastinal lymph node staging for non-small-cell lung cancer. Eur J Cardiothorac Surg 2014;45:787-98. [Crossref] [PubMed]
- Xia S, Ye J, Chen Y, et al. Parallel serial assessment of somatic mutation and methylation profile from circulating tumor DNA predicts treatment response and impending disease progression in osimertinib-treated lung adenocarcinoma patients. Transl Lung Cancer Res 2019;8:1016-28. [Crossref] [PubMed]
- Abbosh C, Birkbak NJ, Wilson GA, et al. Phylogenetic ctDNA analysis depicts early-stage lung cancer evolution. Nature 2017;545:446-51. [Crossref] [PubMed]
- Chaudhuri AA, Chabon JJ, Lovejoy AF, et al. Early Detection of Molecular Residual Disease in Localized Lung Cancer by Circulating Tumor DNA Profiling. Cancer Discov 2017;7:1394-403. [Crossref] [PubMed]
- Phallen J, Sausen M, Adleff V, et al. Direct detection of early-stage cancers using circulating tumor DNA. Sci Transl Med 2017;9:eaan 2415.
- Cohen JD, Li L, Wang Y, et al. Detection and localization of surgically resectable cancers with a multi-analyte blood test. Science 2018;359:926-30. [Crossref] [PubMed]
- Abbosh C, Birkbak NJ, Swanton C. Early stage NSCLC - challenges to implementing ctDNA-based screening and MRD detection. Nat Rev Clin Oncol 2018;15:577-86. [Crossref] [PubMed]
- Jamal-Hanjani M, Wilson GA, McGranahan N, et al. Tracking the Evolution of Non–Small-Cell Lung Cancer. N Engl J Med 2017;376:2109-21. [Crossref] [PubMed]
- Zhang Z, Kattan MW. Drawing Nomograms with R: applications to categorical outcome and survival data. Ann Transl Med 2017;5:211. [Crossref] [PubMed]
- Harrell FE Jr. 2019. rms: Regression Modeling Strategies. R package version 5.1-4. Available online: https://CRAN.R-project.org/package=rms
- Shafazand S, Gould MK. A clinical prediction rule to estimate the probability of mediastinal metastasis in patients with non-small cell lung cancer. J Thorac Oncol 2006;1:953-9. [Crossref] [PubMed]
- DeCaro L, Benfield JR. Lung cancer in young persons. J Thorac Cardiovasc Surg 1982;83:372-6. [Crossref] [PubMed]
- Seok Y, Yang HC, Kim TJ, et al. Frequency of lymph node metastasis according to the size of tumors in resected pulmonary adenocarcinoma with a size of 30 mm or smaller. J Thorac Oncol 2014;9:818-24. [Crossref] [PubMed]
- Chen B, Xia W, Wang Z, et al. Risk analyses of N2 lymph-node metastases in patients with T1 non-small cell lung cancer: a multi-center real-world observational study in China. J Cancer Res Clin Oncol 2019;145:2771-7. [Crossref] [PubMed]
- Zhang Y, Sun Y, Xiang J, et al. A prediction model for N2 disease in T1 non-small cell lung cancer. J Thorac Cardiovasc Surg 2012;144:1360-4. [Crossref] [PubMed]
- Moriya Y, Iyoda A, Kasai Y, et al. Prediction of lymph node metastasis by gene expression profiling in patients with primary resected lung cancer. Lung Cancer 2009;64:86-91. [Crossref] [PubMed]
- Jamal-Hanjani M, Wilson GA, Horswell S, et al. Detection of ubiquitous and heterogeneous mutations in cell-free DNA from patients with early-stage non-small-cell lung cancer. Ann Oncol 2016;27:862-7. [Crossref] [PubMed]