Performance of various risk prediction models in a large lung cancer screening cohort in Gdańsk, Poland—a comparative study
Introduction
With accumulating evidence on both benefits and harms of lung cancer screening, unresolved issues such as optimal selection criteria remain a matter of debate (1,2). Current inclusion criteria based on age and categorized smoking history are derived from the National Lung Screening Trial (NLST) (3). Recent results of the NELSON trial proved their modification may alter results of the screening programme (4). Development of more complex epidemiologic and molecular models for estimating an individualised lung cancer risk progressed in recent years, and it should inevitably reduce the screening false positive rate (5-8). These mathematical models are based on the regression analysis and aid in predicting lung cancer probability (6). Such risk prediction models include Tammemagi’s modified Prostate, Colorectal, Lung and Ovarian Cancer Screening Trial 2012 (PLCOm2012), Liverpool Lung Project (LLP) and Bach’s risk prediction model. Additionally, one of the abovementioned models—Tammemagi’s—is recommended by the National Comprehensive Cancer Network (NCCN) Lung Cancer Screening Clinical Practice Guidelines to assist in the risk stratification of a lung cancer in selected individuals (9). Data from a large Polish lung cancer screening cohort of 6,631 individuals from a single European centre was re-analysed applying (I) Tammemagi’s PLCOm2012, (II) LLP, and (III) Bach’s lung cancer risk models. We present the following article in accordance with the TRIPOD reporting checklist (available at http://dx.doi.org/10.21037/tlcr-20-753).
Methods
MOLTEST BIS programme
Six thousand six hundred and thirty one volunteers (median age 63.0 years) were enrolled in the MOLTEST BIS programme between January 2016 and December 2018. Inclusion criteria in the programme (age 50–79 and ≥30 pack-years smoking history) were based on the Lung Cancer Screening NCCN Clinical Practice Guidelines (9). A comparative analysis was performed on the whole screening cohort. Each participant underwent 1–2 low-dose computed chest tomography (LDCT) screening rounds and a selected group underwent a further diagnostic work-up. The screening protocol was based on the modified United Kingdom Lung Screen protocol with a 1-year follow-up including two screening rounds (10). Detailed methodology of the screening management in the MOLTEST BIS programme was the subject of the previous publication (11). Primarily, the MOLTEST BIS programme was a validation study for the early lung cancer biomarkers, which were discovered in our previous lung cancer screening programme (12). Currently, independent validation is performed, and the results will be published. The trial was conducted in accordance with the Declaration of Helsinki and the Harmonized Tripartite Guideline for Good Clinical Practice from the International Conference on Harmonization. The study was approved by the independent ethics committee of the Medical University of Gdańsk (NKEBN/376/2014), and all participants provided written informed consent.
Recruited cohort’s re-analysis according to the lung cancer risk prediction models
A comparative study of (I) Tammemagi’s PLCOm2012, (II) LLP, and (III) Bach’s lung cancer risk models was performed. Data required for risk estimation by different models were collected by the core research team and two independent groups of dieticians and cardiologists. The variables of less than 5% of missing data (e.g., body mass index) were subjected to missing data imputation; the k-nearest neighbour algorithm was used for that purpose. With regard to the Tammemagi’s risk prediction model, authors used a conversion algorithm to make the model’s education levels applicable to the Polish education system and the data available. Details are provided in the Supplementary file. In Tammemagi’s PLCOm2012 model (13) the following factors are taken into regression equation: (I) ethnicity; (II) age; (III) education; (IV) body mass index; (V) family history of lung cancer; (VI) personal history of cancer; (VII) COPD diagnosis; (VIII) smoking status; (IX) smoking duration; (X) number of cigarettes per day; and (XI) time elapsed since quitting.
The construction of the LLP model required personal data on (I) presence of pneumonia, (II) asbestos exposure, (III) personal history of cancer, (IV) family history of cancer, (V) smoking duration, and (VI) age (14).
The Bach’s model’s predictors include: (I) age; (II) gender; (III) asbestos exposure; (IV) smoking intensity (cigarettes per day); (V) smoking duration; and (VI) quit time in former smokers.
Formulas of the models, along with their mathematical and statistical aspects are presented in the Supplementary file.
The detection rate was defined as the number of detected lung cancers divided by the number of screened participants given in percent. True positive cases were considered as diagnosed lung cancer cases, while false positive were cases classified as high risk according to the MOLTEST BIS inclusion criteria without a diagnosis of lung cancer.
Statistical analysis
The descriptive statistics and their 95% confidence intervals were calculated per each factor as well as for prediction model quality indices (15). Missing data at the level of less than 5% of the cases were imputed with the use of k-nearest neighbours’ algorithm (16). The hypothesis on equality of the areas under the receiver operator curve (AUC) among all prediction models was verified by Hanley-McNeil test (17). Contingency tables were analysed with the use of the chi-square test of independence. Formulas of the models, along with its mathematical and statistical aspects are presented in the Supplementary file.
Results
MOLTEST BIS programme—general characteristics
From January 2016 to December 2018, a total of 6,631 patients were screened. Interim results of the diagnostic work-ups and surgeries were previously published (11). Characteristics of the cohort are presented in Table 1. Lung cancer was diagnosed in 154 individuals. The overall lung cancer detection rate was 2.3%.
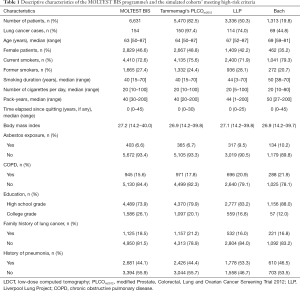
Full table
LDCT, low-dose computed tomography; PLCOm2012, modified Prostate, Colorectal, Lung and Ovarian Cancer Screening Trial 2012; LLP, Liverpool Lung Project; COPD, chronic obstructive pulmonary disease.
Data re-analysis according to the lung cancer risk prediction models
There were 6,631 screenees, who entered the Tammemagi’s, LLP, and Bach’s risk prediction models. Out of 6,631 MOLTEST BIS volunteers: (I) 5,470 (82.4%) met the 1.3% threshold criterion of 6-year lung cancer probability by Tammemagi’s PLCOm2012; (II) 3,336 (50.3%) met the 5-year lung cancer probability ≥5.0% based on the LLP risk estimates; and (III) 1,313 (19.8%) met the 5-year lung cancer probability ≥2.0% obtained with the use of Bach’s formula. Figure 1 presents a flowchart regarding the abovementioned participants’ assignment process. Characteristics of the cohorts are presented in Table 1. Considerably fewer individuals would have been eligible for enrollment to the lung cancer screening programme in the Polish setting, i.e., 17.6%, 49.7%, and 80.2% based on Tammemagi’s PLCOm2012, LLP and Bach’s risk prediction models, respectively (P<0.0001). In the MOLTEST BIS programme154 participants were diagnosed with lung cancer. Among (I) 5,470 high-risk individuals in Tammemagi’s PLCOm2012, (II) 3,336 in LLP, and (III) 1,313 in Bach there would be accordingly 150 (97.4%), 114 (74.0%) and 69 (44.8%) detected lung cancer cases. The potential overall detection rate, i.e., the number of detected lung cancer cases among the high-risk population, would be 2.7% in Tammemagi’s PLCOm2012, 3.4% in LLP and 5.3% in Bach risk prediction model. The rate of screen-detected lung cancer cases applying Tammemagi’s PLCOm2012, LLP, and Bach cohorts to the MOLTEST BIS cohort would be 97.4%, 74.0%, and 44.8%, respectively. The areas under the receiver operating characteristic curve (AUC ROC) for the Tammemagi’s PLCOm2012, LLP and Bach’s models did not differ significantly (P>0.05) (15), and were 0.717 [95% confidence interval (CI): 0.670–0.763], 0.667 (95% CI: 0.619–0.714) and 0.701 (95% CI: 0.655–0.748), respectively. ROC curves are presented in Figure 2.
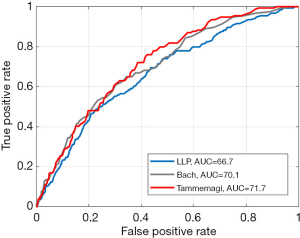
In addition, thresholds for the three models that select the same number of individuals for screening were considered. Thresholds that screen 2/3 of the study population were chosen and compared. Sensitivities were 93.51%, 89.61% and 83.12% for the Tammemagi’s PLCOm2012, LLP and Bach’s models, respectively.
Discussion
NCCN recommendations suggest the implementation of a lung cancer risk model (PLCOm2012) while discussing with an individual at-risk enrollment into the screening programme (shared decision-making). Additionally, utilising Lung Imaging and Reporting Data System (LungRADS) has resulted in the re-definition of positive results. Both tools, in a different manner, are aimed at more accurate targeting the population at need of screening in terms of gaining beneficial effect, i.e., early diagnosis of lung cancer followed by radical surgical treatment, which, in turn, leads to many-year survival.
Therefore, risk prediction models have gained much attention in recent years (1,13,18,19). The collected evidence indicates that use of high-quality risk stratification models to define the eligibility for lung cancer screening enrollment can—among other benefits—improve the screening efficiency (6). Ten Haaf and colleagues have recently reported a validation study, which showed that selected risk prediction models outperform the NLST eligibility criteria (18). Such models include Tammemagi’s modified Prostate, Colorectal, Lung and Ovarian Cancer Screening Trial 2012 (PLCOm2012), LLP and Bach’s risk prediction model. This study assessed the performance of these three risk prediction models in a large lung cancer screening cohort from Gdańsk, Poland. Throughout last ten years over 15,250 individuals have been screened in two lung cancer screening programmes in one screening centre (11,12). This retrospective study was based on full data collected during the latest screening programme conducted in Gdańsk—the MOLTEST BIS programme. We decided to follow the NCCN Guidelines and perform a comparative analysis of risk estimates and enrollment thresholds of the only one risk prediction model recommended in the document, along with two other, well-documented—LLP and Bach’s (7,9,20).
Yet, we are aware that there are more models, e.g., Two-Stage Clonal Expansion (21). It is difficult to answer the question what makes a risk prediction model superior to others. We did not directly compare the validated models to the NCCN criteria. However, NCCN criteria make obvious epidemiological context for our study. Taking the AUC (true positive vs. false positive) under the consideration—the three tested models do not significantly differ. There is other, less obvious criterion which may help to assess the value of the model namely the desired reduction of screened population with a minimal loss to the number of detected lung cancer cases. It is difficult to describe this approach with mathematical tools. We believe that this is the most important criterion. The range of screenees’ reduction varies from 17.6% to 80.2%, yet it comes along with a substantial loss of detected lung cancers of up to 55.2%. In authors’ opinion, out of the three analysed models the Tammemagi’s PLCOm2012 offers an optimal balance between clinical and statistical features. If Tammemagi’s PLCOm2012 risk prediction model would have been applied—(I) the number of individuals included to the screening programmes would have been reduced by 17.6%; (II) only 2.6% of the detected lung cancer cases would have been missed. However, the other two models’ power is similar with AUC of 0.667 and 0.701. In specific settings such models, which (I) screen fewer individuals, (II) reach higher detection rates, yet (III) quantitatively fail to lose more cancer cases may be more appealing in terms of the cost-effectiveness. Based on ethnicity/race, our cohort was homogenous and all participants were Caucasian. However, Tammemagi’s PLCOm2012 risk prediction model includes an ethnicity/race factor, which must be specified. Thus, in authors’ opinion the results are relevant among the majority of the European settings. Implemented definitions of true positive (i.e., lung cancer cases) and false positive (i.e., high risk according to the MOLTEST BIS inclusion criteria without diagnosis of lung cancer cases) do not come along with the classic screening definitions of other programmes (4,22). They are associated with the direct analysis of prediction models and vastly alter from the screening ones.
To conclude, all three models perform better than a screening programme based on the NCCN selection criteria. In authors’ opinion, lung cancer risk prediction models are more accurate in targeting a population at-risk in comparison to currently widely used standard lung cancer screening eligibility criteria based on age and pack-years (9,23). However, further research is sought to harness the full advantage of the risk prediction model’s potential. Optimal thresholds require evaluation based on long-term benefits and harms, preferably in a prospective study.
There are a few study limitations with the majority of them concerning Tammemagi’s model. Firstly, the risk prediction models considered in this study were developed in a cohort originating from a different region than the Eastern European setting (7,13,20). For example, the educational system varies widely between North America and Europe. In our region of Europe, a typical educational path is structured as high school-university.
However, the level of education in Tammemagi’s model is not related to the character of labour which is being performed. It instead serves as an indicator of possessed knowledge, the level of self-consciousness and internal intention to specific sort of behaviour, self-care, and healthy living style, Therefore, it is likely that an individual who had accomplished a graduate degree, temporarily working in a profession below his/her professional qualifications, will be featured by the lower lung cancer incidence than a person with lower educational degree executing the same type of labour. The opposite situation is encountered much more seldom. Briefly, the decisive epidemiological factor here is not labour character by itself, but educational status ensuring the health-promoting behaviour. Keeping this in mind ad hoc small group interviewing for the purpose of confronting educational level with labour character (manual or mental) will not alter analysis outcomes. The dichotomised educational status variable, definitely not ideally, but sufficiently emulates Tammemagi’s model component.
Next, our data about the age of cancer diagnosis in personal or family history was incomplete. Along with the information regarding the presence of COPD and history of pneumonia, it was self-reported by the participants. However, we strived to reduce the risk of overestimation of positive cancer prediction, as the formula’s variable responding to the age below 60 years was additionally promoted in the estimation of the 6-year lung cancer probability.
Finally, we suggest that risk prediction models in their current state of standardisation are valuable selection criteria almost ready for the application in the screening programmes as selection criteria. Yet, results of our paper constitute a factual opinion based on the retrospective study of the large European screening cohort. In our opinion, Tammemagi’s PLCOm2012 is the most promising example due to the minimal loss of detected cases of lung cancer. Perhaps lung cancer prognostic models can be used as a method of deciding how often high-risk individuals should be screened—on an annual or biennial basis.
Prospective validation studies among various populations should be launched to confirm our findings and establish a firm statement about the superiority of the risk prediction models as primary selection criteria for the inclusion to the lung cancer screening programme.
Conclusions
Lung cancer screening enrollment based on the risk prediction models is superior to standard NCCN selection criteria and offers a clinically significant reduction of screenees with a comparable proportion of detected lung cancer cases. Tammemagi’s risk prediction model reduces the proportion of patients eligible for inclusion to a screening programme with a minimal loss of detected lung cancer cases.
Acknowledgments
Funding: MOLTEST BIS programme was financed by a grant funded by the National Centre for Research and Development (Narodowe Centrum Badań i Rozwoju) no PBS3/A7/29/2015/ID-247184. The work was partially financed by SUT grant no 02/010/BK_19/0143.
Footnote
Provenance and Peer Review: This article was commissioned by the Guest Editor (Witold Rzyman) for the series “Implementation of CT-based screening of lung cancer” published in Translational Lung Cancer Research. The article was reviewed by the editorial office.
Reporting Checklist: The authors have completed the TRIPOD reporting checklist. Available at http://dx.doi.org/10.21037/tlcr-20-753
Data Sharing Statement: Available at http://dx.doi.org/10.21037/tlcr-20-753
Conflicts of Interest: All authors have completed the ICMJE uniform disclosure form (available at http://dx.doi.org/10.21037/tlcr-20-753). The series “Implementation of CT-based screening of lung cancer” was commissioned by the editorial office without any funding or sponsorship. WR served as the unpaid Guest Editor of the series. TM reports personal fees from Roche/Genentech, outside the submitted work. The authors have no other conflicts of interest to declare.
Ethical Statement: The authors are accountable for all aspects of the work in ensuring that questions related to the accuracy or integrity of any part of the work are appropriately investigated and resolved. The study was conducted in accordance with the Declaration of Helsinki (as revised in 2013). The study was approved by the independent ethics committee of the Medical University of Gdańsk (NKEBN/376/2014), and all participants provided written informed consent.
Open Access Statement: This is an Open Access article distributed in accordance with the Creative Commons Attribution-NonCommercial-NoDerivs 4.0 International License (CC BY-NC-ND 4.0), which permits the non-commercial replication and distribution of the article with the strict proviso that no changes or edits are made and the original work is properly cited (including links to both the formal publication through the relevant DOI and the license). See:
References
- Kaaks R, Hüsing A, Fortner RT. Selecting high-risk individuals for lung cancer screening; the use of risk prediction models vs. simplified eligibility criteria. Ann Transl Med 2017;5:406. [Crossref] [PubMed]
- Adamek M, Wachuła E, Szabłowska-Siwik S, et al. Risk Factors assessment and risk prediction models in lung cancer screening candidates. Ann Transl Med 2016;4:151. [Crossref] [PubMed]
- National Lung Screening Trial Research Team, Aberle DR, Adams AM, et al. Reduced lung-cancer mortality with low-dose computed tomographic screening. N Engl J Med 2011;365:395-409. [Crossref] [PubMed]
- De Koning H. Effects of volume CT lung cancer screening: mortality results of the Nelson randomised-controlled population based trial. In: 19th World Conference on Lung Cancer, Toronto, Canada. 2018.
- National Lung Screening Trial Research Team, Church TR, Black WC, et al. Results of initial low-dose computed tomographic screening for lung cancer. N Engl J Med 2013;368:1980-91. [Crossref] [PubMed]
- Tammemägi MC. Application of risk prediction models to lung cancer screening. J Thorac Imaging 2015;30:88-100. [Crossref] [PubMed]
- Marcus MW, Chen Y, Raji OY, et al. LLPi: Liverpool Lung Project Risk Prediction Model for Lung Cancer Incidence. Cancer Prev Res (Phila) 2015;8:570-5. [Crossref] [PubMed]
- Widlak P, Pietrowska M, Polanska J, et al. Serum Mass Profile Signature as a Biomarker of Early Lung Cancer. Lung Cancer 2016;99:46-52. [Crossref] [PubMed]
- National Comprehensive Cancer Network. NCCN Clinical Practice Guidelines in Oncology (NCCN Guidelines): Lung Cancer Screening. 2018.
- Baldwin DR, Duffy SW, Wald NJ, et al. UK Lung Screen (UKLS) nodule management protocol: modelling of a single screen randomised controlled trial of low-dose CT screening for lung cancer. Thorax 2011;66:308-13. [Crossref] [PubMed]
- Ostrowski M, Marjański T, Dziedzic R, et al. Ten years of experience in lung cancer screening in Gdańsk, Poland: a comparative study of the evaluation and surgical treatment of 14 200 participants of 2 lung cancer screening programmes†. Interact Cardiovasc Thorac Surg 2019. [Epub ahead of print]. [Crossref] [PubMed]
- Rzyman W, Dziedzic R, Jelitto-Górska M, et al. Results of an open-access lung cancer screening program with low-dose computed tomography: the Gdańsk experience. Pol Arch Med Wewn 2015;125:232-9. [Crossref] [PubMed]
- Tammemägi MC, Katki HA, Hocking WG, et al. Selection Criteria for Lung-Cancer Screening. N Engl J Med 2013;368:728-36. [Crossref] [PubMed]
- Cassidy A, Myles JP, Van Tongeren M, et al. The LLP risk model: An individual risk prediction model for lung cancer. Br J Cancer 2008;98:270-6. [Crossref] [PubMed]
- Hanley JA, McNeil BJ. The meaning and use of the area under a receiver operating characteristic (ROC) curve. Radiology 1982;143:29-36. [Crossref] [PubMed]
- García-Laencina PJ, Sancho-Gómez JL, Figueiras-Vidal AR, et al. K nearest neighbours with mutual information for simultaneous classification and missing data imputation. Neurocomputing 2009;72:1483-93. [Crossref]
- Krzanowski W, Hand D. ROC Curves for Continuous Data. Chapman and Hall/CRC, 2009.
- Ten Haaf K, Jeon J, Tammemägi MC, et al. Risk prediction models for selection of lung cancer screening candidates: A retrospective validation study. PLoS Med 2017;14:e1002277. [Crossref] [PubMed]
- Sanchez-Salcedo P, Wilson DO, De-Torres JP, et al. Improving Selection Criteria for Lung Cancer Screening. The Potential Role of Emphysema. Am J Respir Crit Care Med 2015;191:924-31. [Crossref] [PubMed]
- Bach PB, Kattan MW, Thornquist MD, et al. Variations in Lung cancer risk among smokers. J Natl Cancer Inst 2003;95:470-8. [Crossref] [PubMed]
- Foy M, Spitz MR, Kimmel M, et al. A smoking-based carcinogenesis model for lung cancer risk prediction. Int J Cancer 2011;129:1907-13. [Crossref] [PubMed]
- National Lung Screening Trial Research Team, Church TR, Black WC, et al. Results of initial low-dose computed tomographic screening for lung cancer. N Engl J Med 2013;368:1980-91. [Crossref] [PubMed]
- US Preventive Services Task Fore. Final recommendation statement, screening for lung cancer. 2017. Available online: https://www.uspreventiveservicestaskforce.org/Page/Document/RecommendationStatementFinal/lung-cancer-screening