Radiomic features of primary tumor by lung cancer stage: analysis in BRAF mutated non-small cell lung cancer
Introduction
Lung malignancy is the most frequent cause of cancer-related mortality in the world, accounting for approximately 24% of all cancer-related deaths in the United States (1). Tumor size, a readily assessed feature on medical imaging, is a key determinant in the staging of lung cancer, with many studies correlating increasing tumor size with decreasing overall survival (2,3). Unsurprisingly, most changes in the recently revised tumor-node-metastasis (TNM) classification for lung cancer are based on the largest tumor dimension (4,5).
Converging evidence from the biologic and genetic sciences suggests that size alone is insufficient to explain the progression of lung cancer. Small primary tumors (<5 mm) can demonstrate a remarkable ability to metastasize to local lymph nodes and distant organs (6), thus supporting the hypothesis that lung cancers are not uniform in their biologic or genetic make-up. Indeed, genetic analyses of lung cancers have revealed different genetic and morphologic phenotypes both within a tumor and across different tumors (7,8). Co-existence of lung cancers with non-invasive tumors cells, metastatic tumor cells, or a polyclonal population of both offers a compelling explanation for the co-existence of an early T stage and an overall stage IV tumor (9).
CT helps guide clinical decision-making related to diagnosis, staging, and treatment-response in lung cancer (10,11). Traditional semantic imaging characteristics help establish the TNM stage but cannot differentiate between different clonal populations of lung cancer. Several studies suggest that this limitation can be addressed with radiomic features (12). Extraction of sub-visual and quantifiable data from imaging modalities such as CT and MRI with radiomic features can help create cancer predictive and prognostic correlations. Recent studies report that radiomic features can distinguish between well- and poorly-differentiated lung cancers, categorize benign and malignant subsolid nodules, assess tumor prognosis and treatment response, and predict underlying lung cancer histology (12-17). However, the utility of radiomic features is unclear in differentiating or predicting lung cancer stage based on differences in underlying tumor clonal populations.
Patients with BRAF-mutated non-small cell lung cancer (NSCLC) were included in the study in order to have a homogeneous study population and minimize any confounders related to different driver mutations of lung cancer and different histologic subtypes. BRAF mutations are a rare, but potentially targetable mutation in the treatment of NSCLC (18). BRAF is a protein kinase in the RAS/MAPK pathway, which can lead to cell proliferation and oncogenesis when constitutively activated (19). The clinical features and traditional semantic imaging characteristics of BRAF-mutated NSCLC have been previously reported (19,20). To our knowledge, however, the radiomic features of BRAF-mutated NSCLC and the role of these radiomic features in predicting cancer stage have yet to be investigated. To address these knowledge gaps, we assessed the role of radiomic features to differentiate between different TNM stages (I-IV) of BRAF-mutated NSCLC.
We present the following article in accordance with the MDAR and STROBE reporting Checklist (available at http://dx.doi.org/10.21037/tlcr-20-347).
Methods
The study was conducted in accordance with the Declaration of Helsinki (as revised in 2013). This study was reviewed and approved by the Partners Human Research Committee (Institutional review board protocol numbers 2019P000198, 2016P000767). Informed consent was waived by the IRB due to retrospective nature of the study.
Patient characteristics
Our hospital thoracic oncology database between 2005–2017 was searched to identify BRAF-mutant NSCLC. The presence of BRAF mutation was confirmed with either SNaPshot, a technique that uses next generation sequencing to interrogate BRAF exons 11 and 15, or polymerase chain reaction technique for those diagnosed before 2014. This search yielded 105 patients who had pre-treatment scans available for review. After excluding 43 patients who had only attenuation correction CT performed as part of CTPET imaging and those with CT imaging without IV contrast, 62 patients with diagnostic contrast enhanced CT scans were selected for the study. The included scans were without motion or beam hardening artifacts that could limit the assessment of lung cancer. The specific BRAF mutations were V600 in 27 and non-V600 in 35 patients. The functional classes (21) of BRAF mutant NSCLC were: class 1 in 27/62, class 2 in 22/62 and class 3 in 13/62 patients (Figure 1). The demographics and clinical features such as gender, age, race, histology, smoking history and duration of smoking were extracted from patient electronic medical records.
CT scan parameters
The CT examinations were performed on multidetector-row CT scanners (MDCT) from various vendors using automatic exposure control or fixed mA at a tube potential of 100–120 kV. Images were acquired after administration of intravenous iodinated contrast with standard oncology imaging protocol. Image reconstruction was performed at slice thickness of 1–3 mm for chest using standard soft tissue reconstruction kernels and at a minimum of 5 mm for abdomen and pelvis with images also reconstructed at sagittal and coronal planes. When available brain MRI, head CT, and FDG-PET images were reviewed to determine the stage of lung cancer.
Image analysis
The imaging studies were reviewed by two thoracic radiologists (DPM and SRD) with 1 and 17 years of experience. The images were assessed concurrently and in consensus. The primary tumor assessment included size, location, density (solid, mixed, ground-glass), cavitation, air bronchogram and calcification. The stage of the tumor was determined based on the primary tumor, nodal disease and other sites of disease with in the thorax and outside the thorax based on TNM 8th edition. The histological confirmation was considered gold standard for nodes and metastases when available. Otherwise FDG avidity on PET scan and standard CT imaging features such as nodes greater than 1 cm in short axis and interval growth were taken as proof of disease. The measurements on standard imaging features included maximum and minimum tumor dimensions and lesion density (mean Hounsfield unit, HU).
Radiomic features
The transverse CT images [de-identified transverse CT images (DICOM)] were de-identified and exported offline from the Picture Archiving and Communication System archives and were processed using 3D slicer (Version 4.7), an open source software package. Manual segmentation of area for each lesion was performed using the paint function on chest CT images in lung window (Figure 2). The images were contoured with 3D slicer and we applied the resampling to isotropic voxel size and we used the default discretization of voxel bins. The original radiomic features (n=113) were extracted using radiomics applet. The first order features include mean, median, standard deviation, maximum, minimum, entropy (randomness), and skewness and kurtosis. The second-order features include Gray-Level Co-Occurrence Matrix (GLCM), Gray-Level Run-Length Matrix (GLRLM), Gray Tone Difference Matrix (GTDM) and Grey Level Size Zone Matrix (GLSZM). Data were exported to Microsoft EXCEL (Microsoft Inc., Redmond, Washington). A study co-investigator (AP) made all measurements in consultation with a fellowship-trained thoracic radiologist (SRD, 17 years of experience). The detailed description of the radiomic features is available on https://pyradiomics.readthedocs.io/en/latest/features.html.
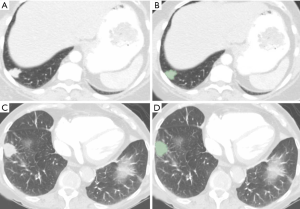
Statistical analysis
Data were analyzed using SPSS 21 statistical software (IBM, Armonk, NY). Pearson correlation coefficient was calculated for clinical, imaging and radiomic features. Univariate and multivariate logistic regression was performed to determine the most significant features. To avoid the multiple collinearity, the variance inflation factor (VIF) was calculated with linear regression analysis. Only, predictors with VIF of less than 5 were selected to determine the significance of radiomic features. Receiver operating characteristics (ROC) area under the curve was generated for clinical, imaging, and radiomic features. For comparing the radiomic features, two-tailed Student’s t-test was used. A P value of less than 0.05 was considered statistically significant.
Results
The patient demographics are described in Table 1.
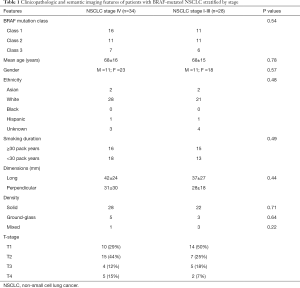
Full table
Comparison of stage IV vs. stages I–III combined (Table 2)
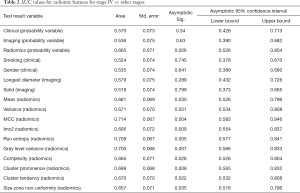
Full table
Between stage IV and combined stages I-III, there was significant difference in forty (40/113) radiomic features by Pearson correlation (P=0.04–<0.0001) and in 26 (26/113) radiomic features by ROC (P=0.04–<0.009) [AUC 0.71 (95% CI: 0.59–0.85)]. The common radiomic features that were different included, mean, variance, 10percentile, energy, run entropy, interquartile range, cluster prominence and tendency, complexity contrast, correlation, imc1/2, MCC, gray level variance, non-uniformity and small area emphasis. Complexity was the strongest predictor of differentiating stage IV versus other stages (P=0.009, Nagelkerke R2=0.18). None of the clinical (0/6) or imaging (0/3) features were significantly different on Pearson correlation or ROC (AUC 0.54, P=0.3–0.9: AUC 0.61, P=0.1–0.9).
Multivariate logistic regression analysis (Table 3) was performed by including selective radiomic features (including complexity, run entropy, variance, cluster tendency, grey level variance B, MCC, busyness). The backward conditional model based only on radiomic features correctly differentiated 73% of stage IV from other stages (sensitivity 88%, specificity 54%), slightly increasing the sensitivity compared to the univariate model. After adding clinical, imaging features to the radiomic features to multivariate logistic regression models there was differentiation 82% of stage IV from other stages (sensitivity 88%, specificity 76%). The logistic regression models were statistically significant (backward: conditional P=0.022, Nagelkerke R2=0.26).
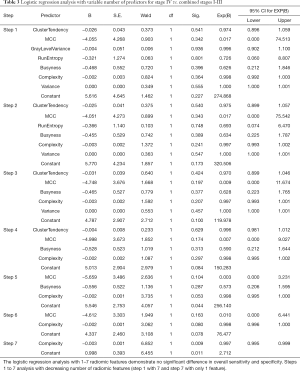
Full table
For probability variables (radiomics, clinical, imaging), ROC analysis showed higher AUC value for radiomic (AUC 0.67, P=0.026) compared to clinical (AUC 0.57, P=0.3) and standard imaging features (AUC 0.54, P=0.6) in differentiating for stage IV from other stages.
Comparison of stage IV vs. stage I–III separately (Table 4)
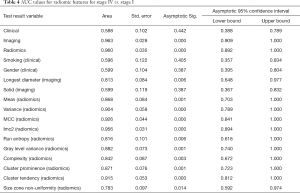
Full table
There was progressive increase in differences in radiomic features of the primary tumor with increase in stage of cancer. The highest difference was between stage IV and stage I and least between stage IV and stage III. Based on Pearson correlation, there were differences in 72/113 radiomic features in primary tumor between stage I and IV, compared to 33/113 between stage 2 and 4 and only in 1/113 between stage III and stage IV. Whereas based on ROC, there were differences in 84/113 of primary tumor radiomic features in stage I, 5/113 in stage II and 4/113 in stage III when compared to primary tumor in stage IV.
None of the clinical (best AUC 0.60; 0.62) or imaging (best AUC 0.60; 0.57) features of primary tumor were significantly different for differentiating stage IV from stage II or III respectively (P=0.3–0.9). Whereas between stage I and stage IV none of the clinical features (AUC 0.60, P=0.2–0.7) were different, and 1/3 imaging features (AUC 0.82, P=0.006–0.9) was significantly different.
Discussion
Our study demonstrates that CT radiomic features of primary tumor in BRAF-mutated lung cancers are significantly different between TMN stages I, II, III, and IV. In comparison, commonly assessed tumor imaging features such as size, location, or density as well as common clinical characteristics related to age, sex, and smoking history could not differentiate between different stages of lung cancer. Similarly, we found that the highest accuracy in differentiation was between stage I and stage IV lung cancers (AUC 0.94 with 72 differentiating radiomic features), followed by between stage II and stage IV lung cancers (AUC 0.77 with 33 features), and finally between a stage III and stage IV lung cancer (AUC 0.71 with just 1 feature). The most important differentiating radiomic features included variance, energy, run entropy, interquartile range, cluster prominence and tendency, complexity contrast, correlation, gray level variance, non-uniformity and small area emphasis.
In our study, only 15% of stage IV BRAF-mutated lung cancers were T4 while most stage IV lung cancers (73%) were T1 or T2. This apparent discordance may be explained by differences in underlying tumor biology, wherein clonal mutations within a tumor population increases the propensity for metastasis. A recent study on breast cancer metastasis have shown similar findings, with a non-linear relationship between cancer size and metastasis potential (22). The authors postulate a parallel model for explaining metastases whereby at the time of original tumor presentation, a parallel process is occurring in underlying cancer stem cell populations, which confers aggressivity in the cancer cells. Under this model, there is not necessarily a stepwise progression of metastatic disease from primary cancer, to local lymph nodes, and then to distant organs. On the contrary, the potential for metastasis is an intrinsic property of the tumor stem cell population, and clonal mutations conferring aggressivity may occur early in the course of disease irrespective of local progression (6). Lending support to this model are several recent molecular studies in breast (23), pancreatic (24), melanoma (25), and colon cancer (26). Our results suggest that radiomic features of the primary lung tumors may helpful in capturing these biological differences, whether due to subtle differences in density or vascularity, which were previously beyond the scope of visual interpretation.
Advances in molecular research have established that tumors are more genetically and phenotypically unstable when compared to their normal counterparts (26,27). Furthermore, it has been established that the majority of secondary or metastatic tumors are clonal in origin (28,29). It is therefore perhaps unsurprising that we see more radiomic differences between early stage tumors and advanced stage tumors, providing further support to the notion of increased tumor heterogeneity as cancers progress along their life cycles. As tumor cells invade the stroma, multiple additional microscopic changes need to occur in the background, including angiogenesis and organization of vascular supply (30). Our results demonstrate that radiomic features can potentially tap into these features, which cannot be semantically captured during conventional image interpretation. Radiomic features can play an important role in noninvasively characterizing primary lung cancers and predict biologic aggressivity and metastatic potential.
Current cancer treatment relies on the resection of primary tumor and the prevention of metastasis, with much emphasis on distant metastasis given its direct association with mortality in patients (30). Current TNM and AJCC staging systems rely on cancer dimensions, local invasiveness, and the presence of distant metastases as an estimate of prognosis. However, since metastatic potential is likely an intrinsic characteristic of the tumor stem cells, there is a potential for radiomic features to be included in staging of tumors and prognosis.
There is a paucity of studies on the utility of radiomic features in differentiating or predicting the TMN stage of primary lung cancers. Radiomic survival analyses of NSCLC patients have previously demonstrated that entropy, skewness, mean density, roundness, and gray-level nonuniformity were most significant predictive features of progression-free survival (31,32). Other studies have found that entropy, kurtosis, range, and skewness, were significant features that can differentiate or are associated with EGFR mutation status in primary lung adenocarcinoma (15,30). More work is needed in this field to validate our findings and establish a standardized set of radiomic features to be assessed in the routine staging of lung cancers.
To our knowledge, this is the first systematic analysis of the utility of radiomic features in the assessment of different TMN stages in primary lung cancer. Only contrast-enhanced CT exams were included to assess for differential enhancement in our texture analysis, especially given the importance of angiogenesis and vascular organization in the growth and invasion of lung cancer (30). Excluding any post-treatment lung cancer patients in this study also eliminated confounders related to treatment.
Our study has several limitations, including a relatively small sample size involving a relatively rare driver mutation, BRAF. There is a question of applicability of our results to other lung cancers with different driver mutations such as EGFR and ALK. Absence of a readily available cohort of patients with other mutations precluded us from performing a comparison. We also do not have data related to treatment response and long term outcomes. Further work in a multi-institutional study with other driver mutations and correlations with treatment response and outcomes will be needed next to assess the generalizability and robustness of our results. Another limitation of our study applies to the variable CT section thickness (1–3 mm), which could affect the reproducibility of radiomic features although the distribution of different section thicknesses was even across different cancer stages. Another potential limitation is use of scanners from different vendors, that can impact the reproducibility of radiomic features, particularly of higher statistical order (33). Given the time and effort involved in lesion identification, segmentation, radiomic features extraction and analyses, it is not clear how and if radiomic features can be applied in routine clinical practice.
In conclusion, radiomic features of primary tumor on contrast-enhanced CT scans are different between early and advanced tumor stages in BRAF-mutated lung cancer. There is increased heterogeneity in terms of texture features between early and advanced tumors, a finding that is in line with current molecular conception of parallel clonal expansion of tumor cells and intrinsic metastatic potential. Radiomic features can serve as a non-invasive biomarker in the initial staging and surveillance of lung cancer, although more work is needed to confirm the validity and generalizability of these findings.
Acknowledgments
Funding: This research did not receive any specific grant from funding agencies in the public, commercial, or not-for-profit sectors.
Footnote
Reporting Checklist: The authors have completed the MDAR and STROBE reporting Checklist. Available at http://dx.doi.org/10.21037/tlcr-20-347
Data Sharing Statement: Available at http://dx.doi.org/10.21037/tlcr-20-347
Conflicts of Interest: All authors have completed the ICMJE uniform disclosure form (available at http://dx.doi.org/10.21037/tlcr-20-347). Dr. IDJ reports personal fees from Boehringer Ingelheim, and AstraZeneca, personal fees from Foundation Medicine, personal fees from Array and Pfizer, grants from Array, Genentech, Pfizer, and Guardant Health, outside the submitted work; Dr. MKK reports grants from Riverain technologies, grants from Siemens Healthineers, outside the submitted work. Dr. SRD reports Merck, Pfizer, Bristol Mayer Squibb, Novartis, Roche, Polaris, Cascadian, Abbvie, Gradalis, Clinical Bay, Zai laboratories, Siemens Medical Solutions, Lunit INC, outside the submitted work. The other authors have no conflicts of interest to declare.
Ethical Statement: The authors are accountable for all aspects of the work in ensuring that questions related to the accuracy or integrity of any part of the work are appropriately investigated and resolved. The study was conducted in accordance with the Declaration of Helsinki (as revised in 2013). This study was reviewed and approved by the Partners Human Research Committee (Institutional review board protocol numbers 2019P000198, 2016P000767). Informed consent was waived by the IRB due to retrospective nature of the study.
Open Access Statement: This is an Open Access article distributed in accordance with the Creative Commons Attribution-NonCommercial-NoDerivs 4.0 International License (CC BY-NC-ND 4.0), which permits the non-commercial replication and distribution of the article with the strict proviso that no changes or edits are made and the original work is properly cited (including links to both the formal publication through the relevant DOI and the license). See: https://creativecommons.org/licenses/by-nc-nd/4.0/.
References
- Siegel RL, Miller KD, Jemal A. Cancer statistics, 2019. CA Cancer J Clin 2019;69:7-34. [Crossref] [PubMed]
- Rami-Porta R, Bolejack V, Crowley J, et al. The IASLC Lung Cancer Staging Project: Proposals for the Revisions of the T Descriptors in the Forthcoming Eighth Edition of the TNM Classification for Lung Cancer. J Thorac Oncol 2015;10:990-1003.
- Zhang J, Gold KA, Lin HY, et al. Relationship between tumor size and survival in non-small-cell lung cancer (NSCLC): an analysis of the surveillance, epidemiology, and end results (SEER) registry. J Thorac Oncol 2015;10:682-90. [Crossref] [PubMed]
- Goldstraw P, Chansky K, Crowley J, et al. The IASLC Lung Cancer Staging Project: Proposals for Revision of the TNM Stage Groupings in the Forthcoming (Eighth) Edition of the TNM Classification for Lung Cancer. J Thorac Oncol 2016;11:39-51. [Crossref] [PubMed]
- Rami-Porta R, Asamura H, Travis WD, et al. Lung cancer - major changes in the American Joint Committee on Cancer eighth edition cancer staging manual. CA Cancer J Clin 2017;67:138-55.
- Friberg S, Nyström A. Cancer Metastases: Early Dissemination and Late Recurrences. Cancer Growth Metastasis 2015;8:43-9. [Crossref] [PubMed]
- Almendro V, Marusyk A, Polyak K. Cellular heterogeneity and molecular evolution in cancer. Annu Rev Pathol 2013;8:277-302. [Crossref] [PubMed]
- de Bruin EC, McGranahan N, Mitter R, et al. Spatial and temporal diversity in genomic instability processes defines lung cancer evolution. Science 2014;346:251-6. [Crossref] [PubMed]
- Yokota J. Tumor progression and metastasis. Carcinogenesis 2000;21:497-503. [Crossref] [PubMed]
- Silvestri GA, Gonzalez AV, Jantz MA, et al. Methods for staging non-small cell lung cancer: Diagnosis and management of lung cancer, 3rd ed: American College of Chest Physicians evidence-based clinical practice guidelines. Chest 2013;143:e211S-e250S.
- Nishino M, Hatabu H, Johnson BE, et al. State of the art: Response assessment in lung cancer in the era of genomic medicine. Radiology 2014;271:6-27. [Crossref] [PubMed]
- Thawani R, McLane M, Beig N, et al. Radiomics and radiogenomics in lung cancer: A review for the clinician. Lung Cancer 2018;115:34-41. [Crossref] [PubMed]
- Digumarthy SR, Padole AM, Lo Gullo R, et al. CT texture analysis of histologically proven benign and malignant lung lesions. Medicine (Baltimore) 2018;97:e11172. [Crossref] [PubMed]
- Digumarthy SR, Padole AM, Rastogi S, et al. Predicting malignant potential of subsolid nodules: can radiomics preempt longitudinal follow up CT? Cancer Imaging 2019;19:36. [Crossref] [PubMed]
- Digumarthy SR, Padole AM, Gullo RL, et al. Can CT radiomic analysis in NSCLC predict histology and EGFR mutation status? Medicine (Baltimore) 2019;98:e13963. [Crossref] [PubMed]
- Shi L, He Y, Yuan Z, et al. Radiomics for Response and Outcome Assessment for Non-Small Cell Lung Cancer. Technol Cancer Res Treat 2018;17:1533033818782788. [Crossref] [PubMed]
- Avanzo M, Stancanello J, Pirrone G, et al. Radiomics and deep learning in lung cancer. Strahlenther Onkol 2020. [Crossref] [PubMed]
- Planchard D, Smit EF, Groen HJM, et al. Dabrafenib plus trametinib in patients with previously untreated BRAFV600E-mutant metastatic non-small-cell lung cancer: an open-label, phase 2 trial. Lancet Oncol 2017;18:1307-16. [Crossref] [PubMed]
- Paik PK, Arcila ME, Fara M, et al. Clinical characteristics of patients with lung adenocarcinomas harboring BRAF mutations. J Clin Oncol 2011;29:2046-51. [Crossref] [PubMed]
- Mendoza DP, Dagogo-Jack I, Chen T, et al. Imaging characteristics of BRAF-mutant non-small cell lung cancer by functional class. Lung Cancer 2019;129:80-4. [Crossref] [PubMed]
- Dagogo-Jack I, Martinez P, Yeap BY, et al. Impact of BRAF Mutation Class on Disease Characteristics and Clinical Outcomes in BRAF-Mutant Lung Cancer. Clin Cancer Res 2019;25:158-65. [Crossref] [PubMed]
- Sopik V, Narod SA. The relationship between tumour size, nodal status and distant metastases: on the origins of breast cancer. Breast Cancer Res Treat 2018;170:647-56. [Crossref] [PubMed]
- Hosseini H, Obradović MMS, Hoffmann M, et al. Early dissemination seeds metastasis in breast cancer. Nature 2016;540:552-8. [Crossref] [PubMed]
- Rhim AD, Mirek ET, Aiello NM, et al. EMT and dissemination precede pancreatic tumor formation. Cell 2012;148:349-61. [Crossref] [PubMed]
- Werner-Klein M, Scheitler S, Hoffmann M, et al. Genetic alterations driving metastatic colony formation are acquired outside of the primary tumour in melanoma. Nat Commun 2018;9:595. [Crossref] [PubMed]
- Heppner GH, Miller FR. The cellular basis of tumor progression. Int Rev Cytol 1998;177:1-56. [PubMed]
- Strauss BS. Hypermutability and silent mutations in human carcinogenesis. Semin Cancer Biol 1998;8:431-8. [Crossref] [PubMed]
- Popper HH. Progression and metastasis of lung cancer. Cancer Metastasis Rev 2016;35:75-91. [Crossref] [PubMed]
- Yamamoto N, Yang M, Jiang P, et al. Determination of clonality of metastasis by cell-specific color-coded fluorescent-protein imaging. Cancer Res 2003;63:7785-90. [PubMed]
- Ganeshan B, Miles KA, Young RCD, et al. In search of biologic correlates for liver texture on portal-phase CT. Acad Radiol 2007;14:1058-68. [Crossref] [PubMed]
- Ganeshan B, Abaleke S, Young RCD, et al. Texture analysis of non-small cell lung cancer on unenhanced computed tomography: initial evidence for a relationship with tumour glucose metabolism and stage. Cancer Imaging 2010;10:137-43. [Crossref] [PubMed]
- Shen TX, Liu L, Li WH, et al. CT imaging-based histogram features for prediction of EGFR mutation status of bone metastases in patients with primary lung adenocarcinoma. Cancer Imaging 2019;19:34. [Crossref] [PubMed]
- Traverso A, Wee L, Dekker A, et al. Repeatability and reproducibility of radiomic features. Int J Radiat Oncol Biol Phys 2018;102:1143-58. [Crossref] [PubMed]