3D radiomics predicts EGFR mutation, exon-19 deletion and exon-21 L858R mutation in lung adenocarcinoma
Introduction
Lung cancer is the most common cause of mortality among malignant tumors. With the application of lung cancer CT screening and advancement of treatment methods, the 5-year survival rate has increased from 10–20% to 15–30% (1). Lung adenocarcinoma is the most common subtype of lung cancer, accounting for 40% (2). The core mechanism of carcinogenesis of lung adenocarcinoma is the somatic mutation of epidermal growth factor receptor (EGFR) that leads to persistent activation of EGFR tyrosine kinase domain in tumor tissue. Structural changes of the EGFR gene due to activating mutations are associated with the proliferation of adenocarcinoma cells. The therapeutic effect of tyrosine kinase inhibitors (TKIs) depends on the mutation type of the EGFR gene (3,4). Tumor response percentage of patients with activating mutant EGFR is 68% and the progression-free survival time in these patients is 12.1 months compared with only 11% and 3.4 months in patients with wild type EGFR (5,6). The guideline from the College of American Pathologists, the International Association for the Study of Lung Cancer, and the Association for Molecular Pathology recommends routine testing for EGFR mutations to guide molecular targeted therapy in adenocarcinoma patients (7).
EGFR mutation sites mostly locate in exons 18, 19, 20, and/or 21. The two most common activating mutations are exon-19 deletion (Del19) and exon-21 mutation (L858R) (8,9). Patients who have tumors harboring one of these two mutations exhibit a greater objective response rate to afatinib, erlotinib, and gefitinib (10). There is differential prognosis based on EGFR mutation: the overall survival time for patients with exon-19 and -21 mutations with targeted therapy was found to be 41 to 44 months, longer than those with exon-18 mutation (19 months) (11). Therefore, detection of specific EGFR mutations may be of interest in guiding the application of targeted therapy in lung adenocarcinoma patients.
The detection of EGFR mutation status relies on gene sequencing or amplification refractory mutation systems. These detection techniques require invasive sampling methods such as surgical excision or tissue biopsy (12), associated with costs and patient discomfort. More importantly, the intra-tumor heterogeneity leads to heterogenous biopsy sampling results. The implementation of these molecular tests is still moderate to poor. A non-invasive approach would be helpful to screen for EGFR mutation to guide further treatment before the clinical intervention. Because imaging phenotype seems to reflect the genotype of lung cancer (13,14), imaging morphology, features, and patterns, such as texture and intensity distribution are often used to derive information regarding diagnosis, tumor heterogeneity, and prognosis. In comparison with a traditional visual inspection, radiomics transforms medical images into minable data to develop models to guide clinical decision (15,16). Zhou et al. found no difference in CT morphology in 346 patients with and without EGFR mutation (17). Rios Velazquez et al. analyzed 763 adenocarcinoma cases and observed an AUC of 0.69 to distinguish mutant EGFR based on radiological features (18). However, there have been improved results on the association between CT radiomic features and EGFR mutation. Yip et al. revealed that radiomic features could classify the mutation status of EGFR (19). To our knowledge, no study has comprehensively investigated whether the different activating EGFR mutations can be estimated from imaging features. We performed a comprehensive CT-derived radiomics analysis on lung adenocarcinoma patients to classify the presence of the EGFR mutation status, and whether we are able to classify exon-19 deletion and exon-21 L858R mutation.
Methods
Study population
From July 2017 to December 2018, an exhaustive search in the electronic health records was performed for lung cancer patients who had molecular testing for EGFR mutation in our hospital. The inclusion criteria were: (I) tumor sample tissue obtained by surgery; (II) pathologically diagnosed adenocarcinoma based on hematoxylin-eosin and immunohistochemistry staining; (III) pre-surgery thin-slice (<1 mm) contrast-enhanced CT scan; (IV) time interval less than 2 months between CT scan and surgery. Patients were excluded if the tumor edge was indistinguishable with the naked eyes, and thus impossible to accurately segment on the CT images, caused by factors such as unclear demarcation between the tumor and surrounding consolidation or pleural effusion, etc. This retrospective study was approved by the Institutional Review Committee and waived the requirement for informed consent.
The collected data were patient characteristics, smoking status including non-smoker or smoker (former or current), histological subtype of adenocarcinoma and molecular outcome. The study flow diagram is shown in Figure 1.
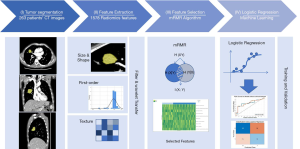
CT acquisition
CT examinations were performed on three modern CT systems (Somatom Force, Siemens Healthineers; Revolution and HD750, GE Healthcare). All included patients underwent contrast-enhanced chest CT scanning after injection of 60–80 mL contrast-media (Iopamiro 300, Bracco) into the antecubital vein at 3 mL/sec. The reconstructed slice thickness was 0.6 or 0.625 mm. The detailed acquisition protocol and reconstruction parameters are listed in Table S1.
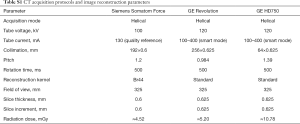
Full table
Histologic evaluation and molecular testing
The criteria for the histological definition of adenocarcinoma were according to the 2015 World Health Organization classification of lung cancer (20). Testing for EGFR mutations including exon expression of 18, 19, 20, and 21 was performed by using a human EGFR gene mutation detection kit (Aide Biomedical Technology).
Tumor segmentation and radiomic feature extraction
We used a radiomics analysis package (Radiomics 1.0.9a, Siemens Healthineers) on a research platform (SyngoVia VB10b, Research Frontier, Siemens Healthineers) to three-dimensionally segment the tumor on CT images as a volume of interest and extracted radiomic features (21). Two radiologists with experiences of at least five years in diagnostic chest CT semi-automatically segmented these tumors, by finding the lesion and click on it. Then the software automatically segmented the lesion.
A total of 1,672 features were extracted from each tumor (https://cdn.amegroups.cn/static/application/8373764accc56018fb4582edf66e4fe4/tlcr-20-122-1.pdf). The extracted computational features were classified into original features, filtered (9 types) and wavelet transformed (8 types). The composition and classification of features were listed in Figure S1. The algorithmic principles are explained in the Supplementary File.
Feature selection
Features with a consistent correlation coefficient (CCC) greater than 0.70 (22) were selected for further analysis. Subsequently, these candidate features were characterized by the minimum redundancy maximum relevance (mRMR) algorithm, which allows for further feature selection by maximizing the correlation between features and label while minimizing the interaction information among features (23).
All candidate radiomic features were ranked according to their explanatory power with regards to the presence of EGFR mutation. The most relevant features were additionally selected and clustered. The thresholds of significance and effect size were set to 0.05 and 0.1 for feature relevance, respectively. All features satisfying these relevance criteria were considered for subsequent decorrelation mRMR procedure. In this second part of the mRMR algorithm process, redundant features are removed. For decorrelation, classic mRMR was chosen in this study. The maximum number of features was defined as 10 and a fast mRMR algorithm was applied to select the proper number of features.
Model establishment and validation
The enrolled patients were randomly divided into training (80%) and test (20%) cohort for model establishment and validation, respectively. Five-fold cross-validation was performed for the data in the training cohort, to make sure that the stability of the established models was sufficient. A logistic regression algorithm was used as a machine learning-based classification model built with the features selected from the univariate statistics on the training cohort. Logistic regression, a traditional method in radiomic research, has the advantages of understandability and interpretability, and can incorporate both discrete and continuous variables (24,25).
Six logistic regression models were established by Radiomics software (Radiomics 1.0.9a, Siemens Healthineer). Three logistic regression models based on radiomic features were built to detect EGFR mutation, and to identify exon-19 deletion and exon-21 mutations, respectively (Model EGFR_A, Exon19A, and Exon21A). In addition, three multivariate logistic regression models were generated based on radiomic features combined with clinical factors (age, gender, and smoking history) (Model EGFR_B, Exon19B, and Exon21B). The whole training cohort was used to establish each model.
The most relevant model was determined by the best subset forward selection method. This method starts with an empty model and adds in first 10 features one by one. In each forward step, the one feature that gives the best improvement to the model is added. The most relevant model was finally selected by the model selection criterion. For each model, the most relevant feature subset was chosen by Akaike information criterion (AIC) and Bayesian information criterion (BIC). When fitting model, it is possible to lead to overfitting if increasing the likelihood by adding parameters, AIC and BIC are designed to handle this problem by introducing a penalty term for the number of parameters in the model. This penalty term is smaller in AIC than in BIC. 95% confidence level was reported when assessing the best subset’s model.
Statistics
The normality of data was assessed by the Kolmogorov-Smirnov test. An independent-sample t-test or Mann-Whitney U test was used for continuous variables and chi-square or Fisher’s exact test was applied for comparing the categorical variables. Two observers independently evaluated 50 randomly selected patients to access inter-observer agreement of feature extraction expressed as an intraclass correlation coefficient (ICC). High ICC indicates high inter-observer agreement. The created logistic regression models were used to evaluate the classification of EGFR mutation in the independent test cohort. The model performance was assessed by using receiver operating characteristic (ROC) curve and area under the ROC curve (AUC), sensitivity, specificity, and confusion matrix. Pairwise comparisons were performed among AUCs by DeLong’s test (26). The model validation and statistical analysis part were performed by two software packages (Matlab R2019a, Mathworks; MedCalc 19.0.4, MedCalc Software). A P value <0.05 was deemed to indicate statistical significance.
Results
Demographics
Among 472 Chinese candidate patients, 263 (mean age 62.5±9.4 years) were finally included (Table 1). The training and test cohort consisted of 210 and 53 patients, respectively. The inclusion flowchart is shown in Figure 2. The tumors of all patients were histologically confirmed lung adenocarcinoma, including 215 (81.7%) invasive adenocarcinoma, 30 (11.4%) microinvasive adenocarcinoma, and 18 (6.8%) other types. In terms of CT lesion density, 153 (58.2%) were solid, and 110 (41.8%) were sub-solid. With regards to molecular testing, 84 (31.9%) of cancers were wild type, and 179 (68.1%) showed EGFR mutation, including 73 (27.8%) exon-19 deletion, and 99 (37.6%) exon-21 L858R mutation. Seven (2.7%) had a mutation of either exon-18 G719X, exon-20 20-INS, exon-20 S768I, exon-20 T790M, exon-21 L859R, exon-21 L860R, or exon-21 L861R. Figure 3 shows the relationship between tumor location, age, and maximum tumor diameter. Figure 4 shows several representative cases.
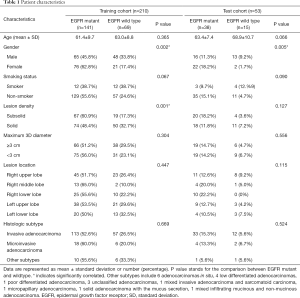
Full table
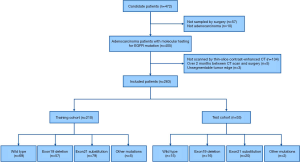
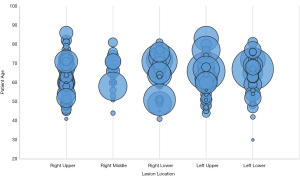
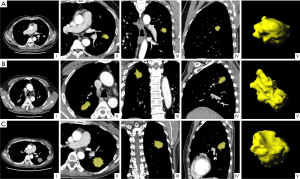
In univariate analysis, female gender and subsolid density showed a significant positive correlation with EGFR mutation (P<0.01). EGFR exon-19 deletion associated with higher age (P=0.014), but was not associated with other clinical factors (P>0.05). Exon-21 mutation was more common in men (P=0.016) and in solid lesions (P=0.005).
Classification of EGFR mutation status
A total of 1,672 radiomic features were extracted from each tumor. The ICC between two assessments for all extracted features was 0.937±0.063, indicating an optimal interobserver agreement. Summary of diagnostic metrics to detect EGFR mutation, exon-19 deletion, and exon-21 L858R mutation is shown in Table 2.
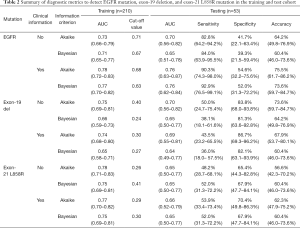
Full table
To establish a multivariate logistic regression model for only radiomic features (Model EGFR_A), all features with CCC >0.7 were first selected before proceeding feature processing. Then 172 features that were both statistically significantly correlated with EGFR mutation and non-redundant were finally selected by the mRMR algorithm. Subsequently, Model EGFR_A was established by selecting the top 10 most relevant features, which were all image texture features (Table S2). When clinical factors were integrated (Model EGFR_B), the resulting top 10 features changed to one clinical character (gender), three first-order, and six texture features (Table S3).
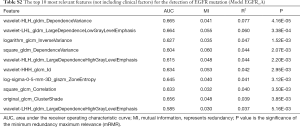
Full table
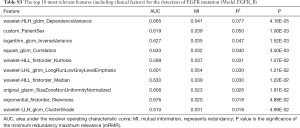
Full table
Adding clinical factors to the regression models improved the performance of the model. In the training cohort, the AUC increased from 0.73 (95% CI: 0.66–0.79) to 0.78 (0.72–0.83) (DeLong’s P=0.136) with AIC selection criteria, and from 0.71 (0.65–0.77) to 0.77 (0.70–0.82) (P=0.110) with BIC (Figure 5), respectively. In the test cohort, the AUC increased from 0.70 (0.56–0.82) to 0.76 (0.63–0.87) (P=0.114) and significantly increased from 0.65 (0.51–0.78) to 0.76 (0.62–0.84) (P=0.011), respectively. Subsequently, the accuracy in the test cohort increased to 75.5% (61.7–86.2%) and 73.6% (59.7–84.7%) with AIC and BIC selection criteria, respectively (Table S4). Among these methods, Model EGFR_B using BIC selection criteria exhibited a sensitivity of 92.9% (76.5–99.1%).
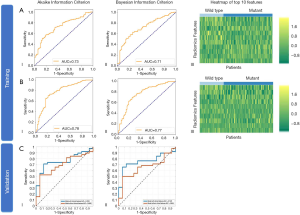
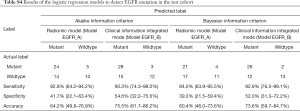
Full table
Classification of EGFR exon-19 deletion and exon-21 L858R mutation
For exon-19 deletion, 71 features were finally selected by mRMR and the top 10 most relevant features were all texture types (Table S5). Adding clinical factors changed the top 10 features to one clinical feature (age), three first-order, and six texture features (Table S6). When training Model Exon19, integration of clinical factors significantly improved AUC with AIC selection criteria (DeLong’s P=0.019) but not improved with BIC (P=0.835) (Figure S2). Additionally, in both training and test cohort of Model Exon19, the AUC of AIC selected models was larger than that of BIC selected models. The highest accuracy among the two models in the test cohort was 73.6% (59.7–84.7%) with AIC selection criteria without the integration of clinical factors (Table S7). Model Exon19B using AIC selection criteria exhibited a specificity of 86.7% (69.3–96.2%).
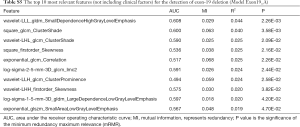
Full table
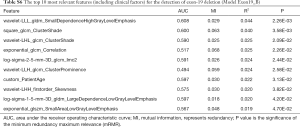
Full table
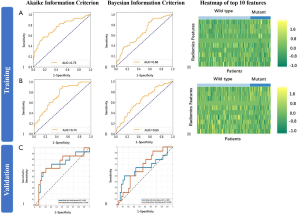
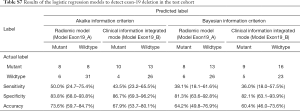
Full table
Regarding exon-21 L858R mutation, more features were selected. The top 10 most relevant features are shown in Tables S8 and S9. When training Model Exon21, integration of clinical factors did not improve AUC with AIC selection criteria (P=0.413), but significantly improved with BIC (P=0.043) (Figure S3). Using Model Exon21, the highest accuracy among the two models in the test cohort was 62.3% (47.9–75.2%) with AIC selection criteria with the integration of clinical factors (Table S10). Model Exon21B using AIC selection criteria exhibited a specificity of 70.4% (49.8–86.3%).
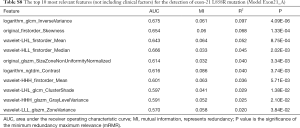
Full table
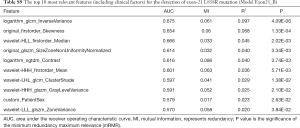
Full table
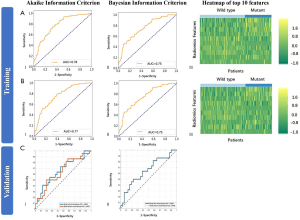
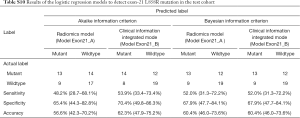
Full table
Discussion
In this study, we established six logistic regression models based on radiomic features and clinical factors to identify and differentiate EGFR mutations. The highest sensitivity among the two models to identify EGFR mutation was 92.9%, indicating a very low false-negative rate. This suggests that a radiomics based model can be helpful to select EGFR mutation patients for further invasive procedures. The highest specificities among two exon-19 deletion classifying models and among two exon-21 L858R mutation models were 86.7% and 70.4%, respectively, indicating a low false-positive rate. Our study reveals the possibility to screen for EGFR mutation by radiomic features on CT images.
In this study, a multivariate model combining radiomic features as well as patient characteristics improved the diagnostic performance to detect EGFR mutation in lung adenocarcinoma patients and reached an AUC of 0.78 and 0.76 in the training and test cohort, respectively. Liu et al. also showed that CT imaging features of adenocarcinoma combined with clinical variables could better classify EGFR mutation status than only using clinical variables (27). Yip et al. reported an AUC of 0.67 in predicting EGFR mutation status (19). Zhang et al. reported an AUC of 0.86 and 0.87 for the training and validation cohort, respectively, based on 140 patients, including 68 adenocarcinomas, 54 squamous cell carcinoma, and 18 others (28). Our results were based on 263 patients with histologically confirmed lung adenocarcinoma, which might be closer to a clinical setting, in view of the experience that the therapeutic effect of tyrosine kinase inhibitors is more significant in lung adenocarcinoma. Importantly, we found a sensitivity to detect EGFR mutation of 92.9%, which indicates a very low false-negative rate.
In addition, we found that lung cancer was located predominantly in the upper lobes, especially on the right side (Table 1), that is consistent with the prior study (29). One well-accepted explanation is that hazardous particles deposit more readily in the upper lobes because of the gravity and ventilation, and they may persist longer there due to less relative ventilation or less efficient lymphatic clearance (30). Another reason might be the higher tissue PO2 levels in the upper lobes that help with tumor initiation, and neovascularization.
The major strength of this study is a comprehensive analysis of the two most common activating EGFR mutations, exon-19 deletion, and exon-21 L858R mutation. Rosell et al. found that the probability of mutation sites of EGFR gene expression was higher in exon-19 than in exon-21 (31). Therapeutic effects of TKIs in EGFR mutation-positive lung cancer patients differ due to specific activating EGFR mutation (32-34). To the best of our knowledge, only a few studies investigated imaging factors associated with exon expression. Li et al. showed an AUC of approximately 0.79 for the detection of exon-19 deletion and exon-21 mutations using radiomics, but did not apply the model to an independent test cohort (35). Our results reveal that radiomic features have a high specificity to identify exon-19 deletion and exon-21 L858R mutation.
3D segmentation algorithm was applied to extract a large number of candidate radiomic features for establishing the predicting model. Most radiomics-related studies only extracted relatively few features for analysis (35-38). In our study, 1,672 features covering three different categories and various filters and translations were extracted in the feature extraction stage, which provides a broad base for features involving in the predicting models. These features can maximize the potential information hidden behind the images, thus improving the capacity of established models.
Radiomics has received extensive attention in recent years (21,22,24). The intrinsic association between image features and EGFR gene expression in lung adenocarcinoma could be explored further by data mining for diagnosis, prognosis, and clinical decision-making (36). Zhang et al. combined clinical data and 485 radiation features extracted from CT images to classify EGFR mutation status, finally reached an accuracy of 75.6% (28). There are some radiomics studies on lung adenocarcinoma that reported similar contributing features as our study. For the EGFR mutation detection models, the radiomic features entered the model all reflect texture-related information. Park et al. (39) and Jiang et al. (40) included glszm_ZoneEntropy feature, representing the tumor heterogeneity in the texture pattern, in the radiomic models to predict the subtype and spread through air space in lung adenocarcinoma patients. Hong et al. (41) and Sun et al. (42) showed that InverseVariance was associated with EGFR mutation and invasion in patients with advanced lung adenocarcinoma. In addition, skewness, mean and median that belong to the first-order feature type contributed to the radiomic models in predicting lesion invasion (42), ALK mutation (43), spread through air space (40) and EGFR mutation (41).
There are limitations to this study. First, this evaluation was a retrospective study performed in a single center. Ideally, a multicenter prospective study would enhance the conclusion of this study, as well as test the effect of the implementation of such a radiomics-based model in clinical practice. Second, although we included 263 patients, increasing the sample size would further strengthen the accuracy of the algorithm model in this study. Third, one single algorithm was used for feature selection and building regression model, more algorithms could increase the robustness by horizontal comparison.
Conclusions
CT radiomics can sensitively identify the presence of EGFR mutation with a low false-negative rate, and increase the certainty of identification of EGFR exon-19 deletion and exon-21 L858R mutation in lung adenocarcinoma patients but the discrimination between both DNA aberrations show less specificity. CT radiomics may become a non-invasive alternative biomarker to select EGFR mutation patients for invasive sampling.
Supplementary
Predictive features
In the six top-10 most relevant feature lists for the six models in the maintext classifying EGFR mutation and exon expression, glcm-Cluster Shade feature existed in all the six lists, glcm-Correlation in four models (Model EGFR_A, Model EGFR_B, Model Exon19A, Model Exon19B), and glcm-Inverse Variance in four models (Model EGFR_A, Model EGFR_B, Model Exon21A, Model Exon21B). Gray-level co-occurrence matrix (GLCM) is a statistical method of examining image texture that considers the spatial relationship of pixels. More details for radiomic features interpretation are shown as the following.
Radiomic features interpretation
1. gldm-Large Dependence High Gray Level Emphasis (LDHGLE) (Model EGFR_A):
[1]
The joint distribution of large dependence is measured with higher gray-level values.
2. glcm-Inverse Difference (ID) (Model EGFR_A):
[2]
ID (a. K. A. homogeneity 1) is another measure of image local homogeneity. If the gray level is more uniform, the denominator will remain at a lower level, thus produce a higher overall value.
3. glszm-Zone Entropy (ZE) (Model EGFR_A):
[3]
Here, ϵ is an arbitrarily small positive number (≈2.2×10−16).
Uncertainty/randomness of ZE measures area size and gray distribution. The larger the value, the stronger the heterogeneity in the texture pattern.
4. gldm-Dependence Variance (Model EGFR_B, Model EGFR_A):
[4]
Measures the variance in dependence size in the image.
5. first-order-Kurtosis (Model EGFR_B):
[5]
Where µ4 is the 4th central moment.
Kurtosis is a measure of the ‘peakedness’ of the distribution of values in the image ROI. A higher kurtosis implies that the mass of the distribution is concentrated towards the tail (s) rather than towards the mean. A lower kurtosis implies the reverse: that the mass of the distribution is concentrated towards a spike near the mean value.
Related links: https://en.wikipedia.org/wiki/Kurtosis
6. glrlm-Long Run Low Gray Level Emphasis (LRLGLE) (Model EGFR_B):
[6]
LRLGLRE measures the joint distribution of long run lengths with lower gray-level values.
7. gldm-Small Dependence High Gray Level Emphasis (SDHGLE) (Model Exon19_A, Model Exon19_B):
Measures the joint distribution of small dependence with higher gray-level values.
8. gldm-Large Dependence Low Gray Level Emphasis (LDLGLE) (Model Exon19_A, Model Exon19_B, Model EGFR_A):
[7]
Measures the joint distribution of large dependence with lower gray-level values.
9. glcm-Correlation (Model Exon19_A, Model Exon19_B, Model EGFR_A, Model EGFR_B):
[8]
The correlation is between 0 (uncorrelated) and 1 (perfectly correlated), which shows the linear dependence of gray level values on the voxels in GLCM.
10. glcm-Informational Measure of Correlation (IMC) 2 (Model Exon19_A, Model Exon19_B):
[9]
IMC2 also assesses the correlation between the probability distributions of i and j (the complexity of quantifying textures). It should be noted that HXY1=HXY2 and HXY2−HXY≥0 represent the mutual information of the two distributions.
Therefore, the range of IMC2 = [0, 1], where 0 represents the cases of 2 independent distributions (without mutual information), and the maximum represents the cases of 2 complete correlation and uniform distributions [maximal mutual information, equal to log2 (Ng)]. In this latter case, the maximum value is then equal to
11. Glcm-Cluster Prominence (Model Exon19_A, Model Exon19_B):
[10]
Cluster Prominence is a measure of skewness and asymmetry of GLCM. The larger the value is, the more asymmetric the average value is; the smaller the value is, the peak value appears near the average value, and the smaller the change of the average value is.
12. glszm-Small Area Low Gray Level Emphasis (SALGLE) (Model Exon19_A, Model Exon19_B):
[11]
SALGLE measures the proportion of the joint distribution of small size zones with lower gray-level values in the image.
13. glcm-Inverse Variance (Model EGFR_A, Model EGFR_B, Model Exon21_A, Model Exon21_B):
[12]
Note that skipping k = 0 causes division by 0.
14. glcm-Cluster Shade (Model EGFR A, Model EGFR B, Model Exon19_A, Model Exon19_B, Model Exon21_A, Model Exon21_B):
[13]
Cluster Shade is a measure of the skewness and uniformity of the GLCM. A higher cluster shade implies greater asymmetry about the mean.
15. firstorder-Skewness (Model EGFR B, Model Exon19_A, Model Exon21_A, Model Exon21_B):
[14]
Where µ3 is the 3rd central moment.
Skewness measures the asymmetry of mean distribution. According to the tail elongation and the location of the distribution mass concentration, the value can be positive or negative.
Related links: https://en.wikipedia.org/wiki/Skewness
16. firstorder-Mean (Model Exon21_A, Model Exon21_B):
[15]
The average gray level intensity within the ROI.
17. firstorder-Median (Model EGFR B, Model Exon21_A, Model Exon21_B):
The median gray level intensity within the ROI.
18. glszm-Size-Zone Non-Uniformity Normalized (SZNN) (Model EGFR B, Model Exon21_A, Model Exon21_B):
[16]
SZNN measures the variability of the volume of large and small areas in the whole image, and smaller values indicate higher homogeneity between the volume of large and small areas in the image. This is a standardized version of the SZN formula.
19. Glszm-Gray Level Variance (GLV) (Model Exon21_A, Model Exon21_B):
[17]
Here,
[18]
GLV measures the variance in gray level intensities for the zones.
20. Glszm-Zone Variance (ZV) (Model Exon21_A, Model Exon21_B):
[19]
Here,
[20]
ZV measures the variance in zone size volumes for the zones.
21. ngtdm-Contrast (Model Exon21_A, Model Exon21_B):
[21]
Contrast is an index to measure the change of spatial intensity, but it also depends on the whole gray level dynamic range. When the dynamic range and spatial change rate are very high, the contrast is very high, i.e., the gray scale range is large, and the image between a voxel and its neighborhood changes greatly.
N.B. For fully isomorphic images, Ng, p=1, which results in dividing by 0. In this case, the arbitration value is returned to 0.
Definition of the computational medical imaging vocabulary
Radiomics: Known as computional medical imaging, involving analyzing, translating and extracting medical images into quantitative data to establish models for clinical decision.
Features: Quantitative variables extracted from medical images.
Training cohort: Cohort used to train a machine learning model based on radiomic features, and a set of examples applied to fit the parameters of the model.
Test cohort: Cohort used to provide an unbiased assessment of the radiomic model fitting on the training cohort. The predicted value of the radiomic features is compared with the actual value for evaluation.
AIC: Akaike information criterion, an estimator of the relative quality of radiomic model built with training cohort. AIC assesses the goodness of model fitting for model selection.
BIC: Bayesian information criterion, also a criterion for model selection which is based on the likelihood function.
Acknowledgments
Funding: This study was sponsored by Ministry of Science and Technology of China (2016YFE0103000), National Natural Science Foundation of China (project no. 81971612), Shanghai Municipal Education Commission – Gaofeng Clinical Medicine Grant Support (20181814), Shanghai Jiao Tong University (ZH2018ZDB10), and Clinical Research Innovation Plan of Shanghai General Hospital (CTCCR-2018B04, CTCCR-2019D05). The funders played no role in the study design, data collection, and analysis, decision to publish, or preparation of the manuscript.
Footnote
Data Sharing Statement: Available at http://dx.doi.org/10.21037/tlcr-20-122
Conflicts of Interest: All authors have completed the ICMJE uniform disclosure form (available at http://dx.doi.org/10.21037/tlcr-20-122). Dr. Liu reports non-financial support from Siemens Healthineers during the conduct of the study. Dr. Xu is an employee of Siemens Healthineers, who provided technical supports, but played no role for image data assessment. Dr. Ge is an employee of Siemens Healthineers, who provided technical supports, but played no role for image data assessment. Dr. Xie reports non-financial support from Siemens Healthineers during the conduct of the study. The other authors have no conflicts of interest to declare.
Ethical Statement: The authors are accountable for all aspects of the work in ensuring that questions related to the accuracy or integrity of any part of the work are appropriately investigated and resolved. The study was conducted in accordance with the Declaration of Helsinki (as revised in 2013). This retrospective study was approved by the Institutional Review Committee and waived the requirement for informed consent (SGH [2018]56).
Open Access Statement: This is an Open Access article distributed in accordance with the Creative Commons Attribution-NonCommercial-NoDerivs 4.0 International License (CC BY-NC-ND 4.0), which permits the non-commercial replication and distribution of the article with the strict proviso that no changes or edits are made and the original work is properly cited (including links to both the formal publication through the relevant DOI and the license). See: https://creativecommons.org/licenses/by-nc-nd/4.0/.
References
- Allemani C, Matsuda T, Di Carlo V, et al. Global surveillance of trends in cancer survival 2000–14 (CONCORD-3): analysis of individual records for 37,513,025 patients diagnosed with one of 18 cancers from 322 population-based registries in 71 countries. Lancet 2018;391:1023-75. [Crossref] [PubMed]
- Cheng T, Zhang Z, Cheng Y, et al. ETV4 promotes proliferation and invasion of lung adenocarcinoma by transcriptionally upregulating MSI2. Biochem Biophys Res Commun 2019;516:278-84. [Crossref] [PubMed]
- Yang JC, Wu YL, Schuler M, et al. Afatinib versus cisplatin-based chemotherapy for EGFR mutation-positive lung adenocarcinoma (LUX-Lung 3 and LUX-Lung 6): analysis of overall survival data from two randomised, phase 3 trials. Lancet Oncol 2015;16:141-51. [Crossref] [PubMed]
- Liang SK, Ko JC, Yang JC, et al. Afatinib is effective in the treatment of lung adenocarcinoma with uncommon EGFR p.L747P and p.L747S mutations. Lung Cancer 2019;133:103-9. [Crossref] [PubMed]
- Nishino M, Cardarella S, Dahlberg SE, et al. Radiographic assessment and therapeutic decisions at RECIST progression in EGFR-mutant NSCLC treated with EGFR tyrosine kinase inhibitors. Lung Cancer 2013;79:283-8. [Crossref] [PubMed]
- Thatcher N, Chang A, Parikh P, et al. Gefitinib plus best supportive care in previously treated patients with refractory advanced non-small-cell lung cancer: results from a randomised, placebo-controlled, multicentre study (Iressa Survival Evaluation in Lung Cancer). Lancet 2005;366:1527-37. [Crossref] [PubMed]
- Lindeman NI, Cagle PT, Aisner DL, et al. Updated Molecular Testing Guideline for the Selection of Lung Cancer Patients for Treatment With Targeted Tyrosine Kinase Inhibitors: Guideline From the College of American Pathologists, the International Association for the Study of Lung Cancer, and the Association for Molecular Pathology. J Mol Diagn 2018;20:129-59. [Crossref] [PubMed]
- Yano M, Sasaki H, Kobayashi Y, et al. Epidermal Growth Factor Receptor Gene Mutation and Computed Tomographic Findings in Peripheral Pulmonary Adenocarcinoma. J Thorac Oncol 2006;1:413-6. [Crossref] [PubMed]
- Locatelli-Sanchez M, Couraud S, Arpin D, et al. Routine EGFR Molecular Analysis in Non-Small-Cell Lung Cancer Patients is Feasible: Exons 18–21 Sequencing Results of 753 Patients and Subsequent Clinical Outcomes. Lung 2013;191:491-9. [Crossref] [PubMed]
- Carey KD, Garton AJ, Romero MS, et al. Kinetic analysis of epidermal growth factor receptor somatic mutant proteins shows increased sensitivity to the epidermal growth factor receptor tyrosine kinase inhibitor, erlotinib. Cancer Res 2006;66:8163-71. [Crossref] [PubMed]
- Zheng H, Zhang Y, Zhan Y, et al. Prognostic analysis of patients with mutant and wild-type EGFR gene lung adenocarcinoma. Cancer Manag Res 2019;11:6139-50. [Crossref] [PubMed]
- Wu W, Parmar C, Grossmann P, et al. Exploratory Study to Identify Radiomics Classifiers for Lung Cancer Histology. Front Oncol 2016;6:71. [Crossref] [PubMed]
- Yang Y, Yin W, He W, et al. Phenotype-genotype correlation in multiple primary lung cancer patients in China. Sci Rep 2016;6:36177. [Crossref] [PubMed]
- Plodkowski AJ, Drilon A, Halpenny DF, et al. From genotype to phenotype: Are there imaging characteristics associated with lung adenocarcinomas harboring RET and ROS1 rearrangements? Lung Cancer 2015;90:321-5. [Crossref] [PubMed]
- Wilson R, Devaraj A. Radiomics of pulmonary nodules and lung cancer. Translational Lung Cancer Research 2017;6:86-91. [Crossref] [PubMed]
- Gillies RJ, Kinahan PE. H H. Radiomics Images Are More than Pictures, They Are Data. Radiology 2016;278:563-77. [Crossref] [PubMed]
- Zhou JY, Zheng J, Yu ZF, et al. Comparative analysis of clinicoradiologic characteristics of lung adenocarcinomas with ALK rearrangements or EGFR mutations. Eur Radiol 2015;25:1257-66. [Crossref] [PubMed]
- Rios Velazquez E, Parmar C, Liu Y, et al. Somatic Mutations Drive Distinct Imaging Phenotypes in Lung Cancer. Cancer Res 2017;77:3922-30. [Crossref] [PubMed]
- Yip SS, Kim J, Coroller TP, et al. Associations Between Somatic Mutations and Metabolic Imaging Phenotypes in Non-Small Cell Lung Cancer. J Nucl Med 2017;58:569-76. [Crossref] [PubMed]
- Travis WD, Brambilla E, Burke AP, et al. WHO Classification of Tumours of the Lung, Pleura, Thymus and Heart. 4 edition. Lyon: International Agency for Research on Cancer, 2015:996.
- Wels MG, Lades F, Muehlberg A, et al. General purpose radiomics for multi-modal clinical research. SPIE Medical Imaging, 2019, San Diego, California, United States.
- Park JE, Park SY, Kim HJ, et al. Reproducibility and Generalizability in Radiomics Modeling: Possible Strategies in Radiologic and Statistical Perspectives. Korean J Radiol 2019;20:1124-37. [Crossref] [PubMed]
- Peng H, Long F, Ding C. Feature Selection Based on Mutual Information Criteria of Max-Dependency, Max-Relevance,and Min-Redundancy. IEEE Trans Pattern Anal Mach Intell 2005;27:1226-38. [Crossref] [PubMed]
- Tu W, Sun G, Fan L, et al. Radiomics signature: A potential and incremental predictor for EGFR mutation status in NSCLC patients, comparison with CT morphology. Lung Cancer 2019;132:28-35. [Crossref] [PubMed]
- Ji GW, Zhang YD, Zhang H, et al. Biliary Tract Cancer at CT: A Radiomics-based Model to Predict Lymph Node Metastasis and Survival Outcomes. Radiology 2019;290:90-8. [Crossref] [PubMed]
- DeLong ER, DeLong DM. DL C-P. Comparing the areas under two or more correlated receiver operating characteristic curves: a nonparametric approach. Biometrics 1988;44:837-45. [Crossref] [PubMed]
- Liu Y, Kim J, Qu F, et al. CT Features Associated with Epidermal Growth Factor Receptor Mutation Status in Patients with Lung Adenocarcinoma. Radiology 2016;280:271-80. [Crossref] [PubMed]
- Zhang L, Chen B, Liu X, et al. Quantitative Biomarkers for Prediction of Epidermal Growth Factor Receptor Mutation in Non-Small Cell Lung Cancer. Transl Oncol 2018;11:94-101. [Crossref] [PubMed]
- Byers TE, Vena JE. TF R. Predilection of lung cancer for the upper lobes: an epidemiologic inquiry. J Natl Cancer Inst 1984;72:1271-5. [PubMed]
- Tseng CH, Chen KC, Hsu KH, et al. EGFR mutation and lobar location of lung adenocarcinoma. Carcinogenesis 2016;37:157-62. [Crossref] [PubMed]
- Rosell R, Moran T, Queralt C, et al. Screening for Epidermal Growth Factor Receptor Mutations in Lung Cancer. N Engl J Med 2009;361:958-67. [Crossref] [PubMed]
- Goto K, Nishio M, Yamamoto N, et al. A prospective, phase II, open-label study (JO22903) of first-line erlotinib in Japanese patients with epidermal growth factor receptor (EGFR) mutation-positive advanced non-small-cell lung cancer (NSCLC). Lung Cancer 2013;82:109-14. [Crossref] [PubMed]
- Jackman DM, Yeap BY, Sequist LV, et al. Exon 19 deletion mutations of epidermal growth factor receptor are associated with prolonged survival in non-small cell lung cancer patients treated with gefitinib or erlotinib. Clin Cancer Res 2006;12:3908-14. [Crossref] [PubMed]
- Riely GJ, Pao W, Pham D, et al. Clinical Course of Patients with Non-Small Cell Lung Cancer and Epidermal Growth Factor Receptor Exon19 and Exon 21 Mutations Treated with Gefitinib or Erlotinib. Clin Cancer Res 2006;12:839-44. [Crossref] [PubMed]
- Li S, Ding C, Zhang H, et al. Radiomics for the prediction of EGFR mutation subtypes in non-small cell lung cancer. Med Phys 2019;46:4545-52. [Crossref] [PubMed]
- Liu Y, Kim J, Balagurunathan Y, et al. Radiomic features are associated with EGFR mutation status in lung adenocarcinomas. Clin Lung Cancer 2016;17:441-448.e6. [Crossref] [PubMed]
- Mei D, Luo Y, Wang Y, et al. CT texture analysis of lung adenocarcinoma: can Radiomic features be surrogate biomarkers for EGFR mutation statuses. Cancer Imaging 2018;18:52. [Crossref] [PubMed]
- Jiang M, Zhang Y, Xu J, et al. Assessing EGFR gene mutation status in non-small cell lung cancer with imaging features from PET/CT. Nucl Med Commun 2019;40:842-9. [Crossref] [PubMed]
- Park S, Lee SM, Noh HN, et al. Differentiation of predominant subtypes of lung adenocarcinoma using a quantitative radiomics approach on CT. Eur Radiol 2020. [Epub ahead of print]. [Crossref] [PubMed]
- Jiang C, Luo Y, Yuan J, et al. CT-based radiomics and machine learning to predict spread through air space in lung adenocarcinoma. Eur Radiol 2020. [Epub ahead of print]. [Crossref] [PubMed]
- Hong D, Xu K, Zhang L, et al. Radiomics Signature as a Predictive Factor for EGFR Mutations in Advanced Lung Adenocarcinoma. Front Oncol 2020;10:28. [Crossref] [PubMed]
- Sun Y, Li C, Jin L, et al. Radiomics for lung adenocarcinoma manifesting as pure ground-glass nodules: invasive prediction. Eur Radiol 2020. [Epub ahead of print]. [Crossref] [PubMed]
- Song L, Zhu Z, Mao L, et al. Clinical, Conventional CT and Radiomic Feature-Based Machine Learning Models for Predicting ALK Rearrangement Status in Lung Adenocarcinoma Patients. Front Oncol 2020;10:369. [Crossref] [PubMed]