Looking through the eyes of the multidisciplinary team: the design and clinical evaluation of a decision support system for lung cancer care
Introduction
Clinical decision-making in lung cancer is complex, fueled by a large and rapidly growing amount of patient data. Besides radiology data, pathology data and patient contextual data, such as the patient’s medical history, comorbidities, overall health status, pulmonary function, signs and symptoms, patient needs and preferences, this includes an increasing amount of molecular data (1-3). Complexity in lung cancer care mandates collaboration and coordination between clinicians across medical disciplines within the hospital and in the larger hospital network (1,4-7). Multidisciplinary tumor board meetings or MDT meetings can be seen as a coordinating mechanism to synthesize information from multiple disciplines into a single personalized diagnosis and care plan. Benefits that are attributed to high-performing MDT meetings include improvement of diagnosis and TNM staging, timely care, higher treatment rates and better adherence to clinical guidelines (8).
The efficacy of MDT meetings and the quality of the decision-process is mediated by a variety of technical and non-technical factors, manifesting in significant variation in quality of diagnosis and treatment (9,10). Team science studies have shown that high-functioning lung cancer MDTs are structured and supported such that team members develop comprehensive shared mental models of the case at hand, and critically evaluate multidisciplinary evidence (4). Important technical facilitators of this process are clinical decision support systems (CDSSs) that provide diagnostic and prognostic information to the multidisciplinary team (MDT) as input for decision-making (11). Proper delivery of the relevant information by the CDSS in an understandable format into the clinical workflow has shown to be problematic, thereby hampering the impact of CDSS on clinical quality (12,13).
The aim of this study was twofold:
- The design and case-based evaluation of a CDSS that aims to support lung cancer MDTs in constructing such shared mental models of the patient case. This allows the team to assess a patient case holistically and supports multidisciplinary quality control as a basis for definitive diagnosis and personalized therapy selection.
- Development of methods for evaluating CDSS to improve seamless integration of CDSS in the clinical workflow.
Shared mental models
In lung cancer care, essential shared mental models are the teams’ collective understanding of (I) the disease: the TNM classification and the reliability of the underlying diagnostic data, including the need for histologic confirmation by biopsy of stage- and treatment-determining lesions, and (II) effective stratification for the right personalized therapy driven by clinical stage, other prognostic factors and patient preferences (4).
Shared mental models help MDTs to adapt their care strategy accordingly based on a mutual understanding of the situation at hand and can thereby improve quality and outcomes of lung cancer care (4), especially under time pressure and high workload conditions (14) which are essential characteristics of tumor board meetings (9). The ability to adapt strategy is an important skill in high-performance teams (15-17). In fact, high levels of inaccurate clinical staging and underuse and misuse of invasive staging in lung cancer care have been reported (18-22), which is partly explained by a lack of an integrated multidisciplinary perspective of the patients disease and state (4).
Unfortunately, CDSS are often not developed based on a proper understanding of oncological mental models, thereby creating a misfit with existing clinical workflows. Poor usability and difficulties in presenting summarized patient-level information in CDSSs are key barriers to their adoption and routine use (12,13).
In this study the CDSS presents information in a format that is congruent with the user’s existing shared mental model structure. This allows the team to quickly obtain a comprehensive mental model of the patient case and increase the teams’ capacity to adapt strategy (i.e., diagnosis, stage and care plan proposal), through a transparent and verifiable insight in diagnostic information on three levels:
❖ Completeness and strength of diagnosis and staging information.
❖ Discordance between results of diagnostic tests. For example, a lymph node may be radiologically suspicious for malignancy, but with a negative endobronchial ultrasound (EBUS) result and therefore a potential necessity for more invasive staging.
❖ Stage- and fitness-appropriate treatment selection. For a full list of parameters, see Table S1.

Full table
Accordingly, we pose the following hypotheses (Figure 1):
❖ Hypothesis 1 (H1). A CDSS presenting information congruent with the MDTs’ shared mental model structure enables the MDT to discuss the patient case with the complete set of relevant multidisciplinary information in mind.
❖ Hypothesis 2a (H2a). The MDT shared mental model of a case will have a positive effect on objective decision outcome, manifested in spotting relevant—possibly discordant or incomplete—diagnostic findings and contra-indications for treatment and appropriately adapting diagnosis and treatment strategy (vs. baseline).
❖ Hypothesis 2b (H2b). The MDT shared mental model of a case will have a positive effect on self-perceived decision outcome, manifested in higher perceived decision quality and confidence.
Case mix and team composition are other important factors influencing the team decision-making and therefore potential confounders to our study (9). Case mix and team composition were included as control factors in the study design as defined in the study methods section.
Methods
The study was designed to closely mimic actual MDT meetings. This level of realism is reflected in all aspects of the study design, including the selection of participants and team composition, room setup, case mix and protocol.
CDSS prototype
A CDSS prototype was developed to present case-relevant multidisciplinary evidence for lung cancer diagnosis and staging, stage-appropriate treatment selection and treatment-influencing factors such as patient fitness and comorbidities. Parameters were selected in line with the clinical guidelines at the time of study (National Comprehensive Cancer Network, NCCN) and included findings from radiology, biopsy procedures, pathology and molecular diagnostics as well as patient fitness indicators including lab tests, functional tests, and clinical data such as information on cancer history and comorbidities, patient preferences and living status. A full set of parameters is available in Table S1.
The User Interface (UI) of the CDSS is shown in Figure 2A and Figure S1. The UI was optimized to present the diagnostic findings in line with oncological mental models: starting with diagnosis and staging, followed by treatment selection as two consecutive screens. Within the diagnosis screen, diagnostic findings were organized according to TNM classification structure: primary tumor (left side of screen), regional lymph nodes (middle) and distant metastasis (right) congruent with oncology shared mental model structures (4).
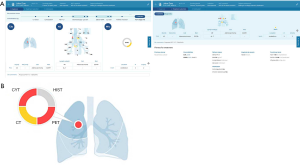
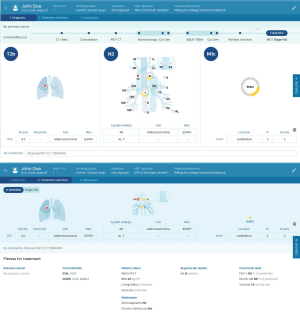
Co-visualization of this data was optimized to assess these findings in conjunction rather than as individual findings, thereby allowing spotting data completeness and discordance in diagnostic findings. Figure 2B shows an illustrative example that shows at a glance the diagnostic test results of a lesion in the right upper lobe (RUL) where the CT was inconclusive (yellow), PET suspicious (red), cytology suspicious (i.e., adenocarcinoma: red) and histology not available (grey). Benign findings are shown in green. A similar structure is used to represent findings on regional lymph node metastases and distant metastases.
A fully functioning software prototype of the CDSS was installed locally on an encrypted laptop. Retrospective de-identified data of n=8 primary lung cancer patients were loaded locally into the prototype. For the purpose of this study, this data was entered manually into the prototype from the EMR. Actual deployment into clinical practice will require further integration with IT systems such as the EMR, as will be elaborated on in the Discussion section.
Participants
Seven medical specialists and two senior pulmonology residents participated as one MDT in the study, representative of the typical team composition in a tumor board according to local hospital practice as well as national Dutch guidelines (23). The MDT members participated on a voluntary basis and their time was compensated in accordance with the Sunshine act.
The team members were senior in their medical specialism and experienced in working together as an MDT in a lung cancer tumor board meeting. This allowed isolation of the effect of the CDSS on the team’s decision-making by controlling for the potential impact of an unfamiliar team. Members had at least twelve years of experience in their medical profession, except the pathologist (six years) and the residents. All members participated in more than a hundred tumor boards in general, as well as with the current team in particular. Only the pathologist (50+ tumor boards) and the residents participated in less tumor boards with the current team. Detailed demographics are available in Table S2.
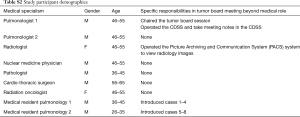
Full table
Case mix
A sample of n=8 primary lung cancer patient cases was selected for discussion in the simulated tumor board in this study. These were retrospective cases that the MDT discussed more than one year prior to the tumor board simulation and their data were de-identified. The case mix covered a diverse set of early and late stage patients requiring different decisions and considerations in the tumor board. Cases mainly differed in terms of extent of the disease and patient fitness, influencing eligibility for various treatments (e.g., based on comorbidities and functional tests). The historical tumor board decisions for these cases with respect to TNM and care plan served as baseline decisions in this study against which the teams adaptation of strategy could be assessed.
Ethics procedure and informed consent
This study was approved by the Internal Committee for Biomedical Experiments (ICBE) of Philips (No. 2017-0075) and conducted in accordance with the Declaration of Helsinki (as revised in 2013). Written informed consent was obtained from the participating clinicians.
The study protocol
The study consisted of four phases (Figure 3). The first phase took place one week prior to the simulated tumor board. In this introduction phase, participants received a 1-hour training to provide a high-level understanding of the CDSS according to a standardized training protocol. Participants also filled out an informed consent form and pre-test questionnaire measuring their experience as individual specialists and team. They received a list of patient cases for the simulated tumor board meeting. In phase two, participants were requested to prepare the cases “as usual”, hence individually and using the Picture Archiving and Communication System (PACS) and Electronic Medical Record (EMR) system as needed.
The third phase was the main focus of the study. The MDT handled eight de-identified cases in a simulated tumor board. These were the cases that they had prepared in phase two. Their decision making process was objectively captured in various ways as outlined in the measurement section. Finally, all participants filled out a questionnaire to measure perceived added value of the CDSS to the decision-making process and outcome.
Room setup
The MDT sat at a U-shaped table in a seat of their preference. They all had a view on the two large 2K resolution monitors mounted to the wall. The monitors showed the PACS with radiology images (left monitor) and the CDSS prototype (right monitor).
The chairman operated the CDSS prototype from a monitor in front of him. The eye-tracker was mounted to the monitor to register gaze patterns of the chairman on the screen during the tumor board discussion.
In addition, several clinicians had access to other systems on demand. The radiologist and nuclear medicine specialist sat behind their regular 4K PACS viewer presenting the radiology images to the team while also having a direct view on the radiology reports. The pathologist had access to the Pathology Information System in case detailed pathological information was required.
Measures and measurement instruments
Triangulation of various measurement instruments was used in this study to capture perception, cognition and behavioral components of decision making driven by the CDSS.
Eye-tracker measures perception and attention focus: the gaze pattern of the chairman pulmonologist was recorded using a Tobii Pro X3-120 eye-tracker and analyzed using Tobii Studio 3.4.8 software (Tobii Technology: Danderyd, Sweden). The eye-tracker recorded the eye movements of the chairman on the user interface of the CDSS, including the focus points, duration and sequence. This allowed the researchers to understand which pieces of (discordant) information in the CDSS (recorded by the eye-tracker) triggered the team to change its strategy (analyzed using the audio-visual recording).
Audio-visual recording of cognition and reasoning: the team’s clinical decision-making process was verbalized naturally as part of their simulated tumor board discussion (think aloud method) and recorded using an audio-visual recording system. Video analysis of the team’s decision-making was performed by two trained observers to identify the points in the tumor board discussion where strategy adaptation from baseline occurred.
Objective decision outcome: case decisions such as TNM and clinical stage, as well as the proposed care plan were captured by the CDSS. These were compared against the baseline decisions that were made more than 1 year prior to the simulation to identify cases involving care strategy adaptation.
Self-perception: a pre-test questionnaire was administered to measure control factors, specifically the team members’ level of experience in their profession and experience working together as a team. A post-test questionnaire was administered to measure the team members’ level of shared mental model of the patient case and perceived decision quality, as well as relevance, readability and understandability of the clinical data presented by the CDSS. A list of measurement items is presented in Table S3.
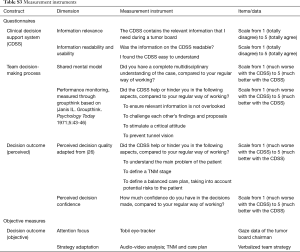
Full table
Results
Clinically correct and usable CDSS
Whether or not the CDSS is clinically correct and usable are important preconditions for the CDSS to have a positive effect on the shared mental model and decision-making of the team. The MDT members generally found that the CDSS contained the relevant information to assess the case (median =4; min =4; max =5 on a 5-point scale). Recommendations were made to include patient complaints and additional granularity in level of suspicion for malignancy. Participants also found the information easily readable (median =4; min =4; max =5 on a 5-point scale) and understandable (median =4; min =4; max =5 on a 5-point scale).
Team decision-making process
Participants generally found that they had a better multidisciplinary perspective of the case using the CDSS, compared to their regular way of working without the CDSS (median =4; min =3; max =4 on a 5-point scale, 2 missing data points). This multidisciplinary understanding represents their shared mental model of the case. The nuclear medicine physician further emphasized the importance of information structure representing shared mental models by indicating “particularly the lymph node stations (.) were discussed more systematically and thus better”.
Participants also reported that the CDSS increases the self-corrective capacity of the team (median =3.88; min =3; max =4 on a 5-point scale, 1 missing data point). Particularly, participants indicated that the CDSS aided them in not overlooking relevant information (6 better or much better with the CDSS than without the CDSS; 2 neutral; 1 missing), challenging each other’s findings and proposals (6 better; 2 neutral; 1 missing), stimulating a critical attitude (5 better; 2 neutral; 1 worse; 1 missing) and preventing tunnel vision (6 better; 2 neutral; 1 missing). This facilitates countering “groupthink” which is one of the biggest potential treats in group decision-making; it is “the mode of thinking that persons engage in when concurrence-seeking becomes so dominant in a cohesive in-group that it tends to override realistic appraisal of alternative courses of action” (24).
In sum, this evidence supports Hypothesis 1 by demonstrating the positive impact of well-designed CDSS in creating shared mental models of the case (Hypothesis 1).
Case-based analysis of team decision-making outcome
Discussion outcomes (diagnosis and care plan) of the eight cases that were discussed by the MDT were compared against the baseline decisions to isolate cases that involved care strategy adaptation. Three out of eight cases showed strategy adaptation from baseline.
The combined results of the eye-tracker analysis (perception), decision making process (cognition) and objective decision outcome (behavior) are depicted in decision diagrams in line with Klein and Sullivan (25). Figure 4 and Figure S2 show the three cases where strategy adaptation was observed. The top of the diagrams shows the MDTs decision-making process, analyzed based on the audio-video recordings. The diagram shows in light grey the baseline considerations from the original case reports in light grey, as a benchmark for the decision process as it was observed in this study in dark grey. From left to right it shows the considerations that were made by the MDT (yellow circles) along with the teams decisions to these considerations (lines coming out of the circles). The final decision of TNM stage and care plan proposal are depicted on the right.
The lower half of the diagrams show the results of the eye-tracking analysis of the chairman’s gaze data in the corresponding episodes. The UI screenshots contain a heat map overlay, where red spots represent areas of the screen where most attention was paid to. The set of red rectangles represent the accumulation of insights gained and verbalized by the MDT, triggered by the patient data on the CDSS.
Case A
The decision course analysis of case A is shown in Figure 4. In the baseline situation, i.e., the tumor board one year prior to the current study, the patient was diagnosed with cT1bN0M0 disease. The corresponding treatment options according to NCCN guidelines were surgery or radiotherapy. It was decided that the eligibility for these therapies was dependent on the fitness of the patients, which needed to be assessed further by the geriatrist.
In the current study, the patient was also diagnosed with cT1bN0M0 disease. The CDSS further revealed that the patient was sufficiently fit with respect to pre-operative pulmonary function, whereas the fact that he also had a cerebrovascular accident (CVA) and was an elderly patient triggered the MDTs preference for SABR radiotherapy over surgery.
This difference in decision outcome reveals that the CDSS supports the MDT to take the holistic patient understanding into account—including patient fitness—to define personalized therapy.
Case E
The decision course analysis of case E is shown in Figure S2. In the baseline situation, the working hypothesis for this patient was cT3N0M0 disease. Also, it was not decided during the tumor board meeting to request a brain MRI scan.
In the current study, the MDT decided that N1 disease could not be excluded based on the available evidence, and hence lymph node station 12R should be included in the treatment. More specifically, the CDSS revealed that both CT and PET scans concordantly showed a suspicious finding in the 12R lymph node region. The CDSS further revealed that subsequently, an EBUS was performed, but that it was unclear from the EBUS report whether 12R was assessed in that study. This led the team to conclude that N1 disease could not be ruled out (working hypothesis N1) and hence 12R should be included in treatment. Also, an MRI of the brain was requested, in line with clinical guidelines, triggered by the stage information in the CDSS.
This case reveals two interesting differences from baseline. First, it shows that simultaneous presentation of diagnostic test results of the lymph nodes enabled the MDT to consider them in conjunction. The concordant suspicion on imaging combined with the cue that EBUS did not conclusively cover the 12R station was driving the N1 decision, versus N0 working hypothesis in the baseline situation. Considering that the individual test results were equal in both scenarios, the suspicious radiology test results apparently had less decisive impact on the baseline decision, even though the coverage of the EBUS was not conclusive.
Secondly, cT3N0 stage in baseline and cT3N1 stage working hypothesis are both indications for requesting a brain MRI scan according to clinical guidelines. Only in this study, it was actually requested during the tumor board meeting, triggered by the CDSS as indicated above.
Case G
In case G a similar reasoning process was observed (see Figure S2 for the visual representation). In the baseline situation, the working hypothesis was cT3N1M0 disease. Also, it was not decided during the tumor board meeting to request a brain MRI scan.
In the current study, the MDT team decided that N2 disease could not be excluded based on the available evidence, and hence lymph node station 4R should be included in the radiation field. More specifically, the CDSS revealed that both CT and PET scans concordantly showed suspicion in the 4R lymph node station. The CDSS further revealed that an EBUS-TBNA was performed, and in the cytology report 4R was described to be a reactive lymph node. However, the discordance between this finding and the strong suspicion on both CT and PET led the team to conclude that N2 disease could not be conclusively ruled out (working hypothesis N2) and hence 4R should be included in radiation field. Also, an MRI of the brain was requested, in line with clinical guidelines.
This case reinforces the added value of the CDSS that was also observed in case E. Again, it shows that simultaneous presentation of diagnostic test results of the lymph nodes enabled the MDT to consider them in conjunction. The nuclear medicine specialist indicated that the PET scan showed considerable FDG-uptake in enlarged lymph node 4R. The MDT discussed the discordance of this finding with the negative EBUS-TBNA cytology result. The CDSS reinforced this insight of discordance to the team throughout the decision making process.
Secondly, cT3N1 stage in baseline and cT3N2 stage working hypothesis are both indications for requesting a brain MRI scan according to clinical guidelines. Only in this study, it was actually requested during the tumor board meeting.
In sum, these results support Hypothesis 2a, since the CDSS allowed the MDT to spot relevant -possibly discordant or incomplete- diagnostic findings and contra-indications for treatment leading to appropriate adaptation of diagnosis and treatment strategy compared to baseline.
Perceived decision quality and confidence
The positive impact of CDSS on the team’s decision-making demonstrated above on case-level was recognized by the individual team members. Participants generally indicated that the CDSS enables them to get to higher quality decisions in the tumor board (median =3.75 out of 5; min =3 and max =4) compared to their normal way of working, particularly on the component tasks that lead to a high quality tumor board (26). These are: to understand the main problem of the patient (4 better with the CDSS than without the CDSS; 4 neutral; 1 missing), to define a TNM stage (5 better or much better with the CDSS than without the CDSS; 2 neutral; 1 missing), and to define a balanced care plan, taking into account potential risks to the patient (3 better with the CDSS than without the CDSS; 5 neutral; 1 missing).
These finding partially support Hypothesis 2b: the MDT shared mental model of a case had a positive effect on self-perceived decision outcome, manifested in higher perceived decision quality.
Interestingly, the team’s confidence in their decisions was equal compared to their regular way of working (median =3, min =2.5 and max =4). This might indicate that the insight provided by the CDSS in concordance/discordance across disciplines is something that the individual MDT members are not necessarily aware of. In other words, it seems to reflect a latent need at the team level rather than at the level of individual team members.
Discussion
Best decisions and self-correcting teams
Lung cancer decision-making is inherently complex and uncertain. Diagnostic findings always carry a certain probability of being false-negative or false-positive, and eligibility for therapy is a careful consideration that depends on a variety of factors. Hence, differences in decision outcome can to some extent be a result of natural variation.
Nonetheless, this study presents initial evidence for the potential of CDSSs that are explicitly designed for multidisciplinary interpretation of diagnostic evidence in conjunction with each other, and surface discordance across multiple medical disciplines and diagnostic findings at the patient level. The CDSS in this study was demonstrated to be capable of triggering multidisciplinary discussion, directing focus of the MDT particularly when findings were inconclusive or discordant. It enabled the team to (re)discuss those findings explicitly in the context of other relevant findings, deliberately consider the consequences, and make a final decision with the relevant objective data in mind. This enabled the team to be adaptive and self-corrective, based on a shared mental model of the case: the combined findings from various individual diagnostic tests creating an additional “safety layer” (27) in the process of the tumor board meeting. Such a self-correcting safety layer can make the tumor board meeting outcome less susceptible to negative performance factors inherent to tumor boards such as team decision-making under time pressure and negative team dynamics (9,10,28). Besides, discrepancies between diagnostic findings or incomplete diagnostic workup can potentially be identified and resolved before the tumor board meeting, to prevent unnecessary delays in care delivery.
Implementation into clinical practice
From a practical point of view, implementing a decision support system is not just “switching on” a CDSS that will instantly enable cross-validation of diagnostic findings, surface discordance between diagnostic tests, and spot contra-indications for personalized treatment selection. The MDT team and the Information Technology (IT) department will initially need to invest time and be open to change(s) in the care path in order to let the CDSS “work for them” and to display quality improvement in decision making and the care path.
Optimizing data exchange and system interoperability is an essential element. For the purpose of this study the method of data input was manual data entry into a research prototype. However, the final product aims to pull data using various extraction techniques from various underlying systems. This includes transfer of structured data (e.g., lab values from LIS) as well as parsing of unstructured radiology and pathology reports using techniques such as Natural Language Processing (NLP). Also, decisions captured in the CDSS tool would be exportable (e.g., through PDF format) back into the EMR or for report-out to the General Practitioner (GP), and subsets of structured data could be made available for exporting to cancer registries.
Once the data is in the system, it can be deployed at different points in the clinical workflow. The best way to integrate a CDSS into the clinical workflow is to set strategic aims in terms of expected efficiency gains and outcome improvements. For example, the CDSS could be used already before the MDT meeting to triage which cases need to be discussed by the MDT. If all the data for a specific patient is there and no data points towards deviation from guidelines (i.e., “straight forward case”) perhaps such cases can be handled by individual physicians. Of course, it is essential that clinical protocols are established to define what makes a case “straight forward” to not require discussion in an MDT. During the MDT meeting such tools can drive efficiency by allowing the MDT to focus their discussion time on cases that are complete. For instance, hospitals could aim to discuss only patients of which the essential data is available in the decision support system.
Once the CDSS has been integrated into the clinical workflow, the generated data can be used to improve quality of care and reduce workload. The CDSS will not only streamline the decision making process of the MDT but also provide insight into quality and efficiency of care.
Conclusions
This study shows the potential of CDSS on clinical decision making, when these systems are properly designed in line with clinical workflow. The CDSS supported the team in their self-correcting capacity for under- and over-staging. It enabled cross-validation of diagnostic findings, surfaced discordance between diagnostic tests and facilitated defining a TNM based on the collected evidence. Finally, the system enabled spotting contra-indications for personalized treatment selection. Additional research is needed to further test these hypotheses quantitatively in a larger sample of cases and MDTs.
This study also proposed and tested a triangulation methodology for assessment of CDSS solutions, through a combined set of measurement instruments that capture perception, cognition and behavioral components of decision making driven by the CDSS. The developed test setup in this study, using a triangulation of decision-analysis, eye-tracking and self-reported data enables assessment of the impact of CDSS design on clinical decision making and optimization of CDSSs to maximize their effect on decision quality and confidence.
Acknowledgments
We would like to thank Prof. Dr. De Ruysscher (Maastro Clinic) for his valuable feedback on the manuscript. We thank the Philips Research integrated oncology software engineering team for building the software prototype and Jeroen Raijmakers from Philips Design for making it possible to use eye-tracking in this study. We are grateful to the medical specialists in the lung cancer tumor board of the participating hospital. We also would like to thank the radiology room manager and the audio-visual service for hosting and facilitating the test. Finally, we would like to acknowledge SeYoung Kim, Niels Laute, Jurriën Gosselink, and Nils Rotgans for their contribution to previous iterations of the CDSS design.
Funding: The study was funded by Philips Research Europe & Philips Experience Design.
Footnote
Conflicts of Interest: All authors have completed the ICMJE uniform disclosure form (available at http://dx.doi.org/10.21037/tlcr-19-441). Dr. JRP, Dr. SL, and Dr. DC have a patent Multidisciplinary Decision Support WO/2018/215603 pending. The other authors have no conflicts of interest to declare.
Ethical Statement: The authors are all accountable for all aspects of the work in ensuring that questions related to the accuracy or integrity of any part of the work are appropriately investigated and resolved. This study was approved by the Internal Committee for Biomedical Experiments (ICBE) of Philips (No. 2017-0075) and conducted in accordance with the Declaration of Helsinki (as revised in 2013). Written informed consent was obtained from the participating clinicians.
Open Access Statement: This is an Open Access article distributed in accordance with the Creative Commons Attribution-NonCommercial-NoDerivs 4.0 International License (CC BY-NC-ND 4.0), which permits the non-commercial replication and distribution of the article with the strict proviso that no changes or edits are made and the original work is properly cited (including links to both the formal publication through the relevant DOI and the license). See: https://creativecommons.org/licenses/by-nc-nd/4.0/.
References
- Detterbeck FC, Lewis SZ, Diekemper R, et al. Diagnosis and Management of Lung Cancer, 3rd ed: American College of Chest Physicians Evidence-Based Clinical Practice Guidelines. Executive Summary. Chest 2013;143:7S-37S.
- Silvestri GA, Gonzalez AV, Jantz MA, et al. Methods for staging non-small cell lung cancer: Diagnosis and management of lung cancer, 3rd ed: American college of chest physicians evidence-based clinical practice guidelines. Chest 2013;143:e211S-e250S.
- Remon J, Ahn MJ, Girard N, et al. Advanced-Stage Non-Small Cell Lung Cancer: Advances in Thoracic Oncology 2018. J Thorac Oncol 2019;14:1134-55. [Crossref] [PubMed]
- Osarogiagbon RU, Rodriguez HP, Hicks D, et al. Deploying Team Science Principles to Optimize Interdisciplinary Lung Cancer Care Delivery: Avoiding the Long and Winding Road to Optimal Care. J Oncol Pract 2016;12:983-91. [Crossref] [PubMed]
- Osarogiagbon RU. Making the Evidentiary Case for Universal Multidisciplinary Thoracic Oncologic Care. Clin Lung Cancer 2018;19:294-300. [Crossref] [PubMed]
- Fasola G, Rizzato S, Merlo V, et al. Adopting integrated care pathways in non-small-cell lung cancer: From theory to practice. J Thorac Oncol 2012;7:1283-90. [Crossref] [PubMed]
- Osarogiagbon RU, Freeman RK, Krasna MJ. Implementing effective and sustainable multidisciplinary clinical thoracic oncology programs. Transl Lung Cancer Res 2015;4:448-55. [PubMed]
- Pillay B, Wootten AC, Crowe H, et al. The impact of multidisciplinary team meetings on patient assessment, management and outcomes in oncology settings: A systematic review of the literature. Cancer Treat Rev 2016;42:56-72. [Crossref] [PubMed]
- Soukup T, Lamb BW, Arora S, et al. Successful strategies in implementing a multidisciplinary team working in the care of patients with cancer: An overview and synthesis of the available literature. J Multidiscip Healthc 2018;11:49-61. [Crossref] [PubMed]
- Soukup T, Petrides KV, Lamb BW, et al. The anatomy of clinical decision-making in multidisciplinary cancer meetings: A cross-sectional observational study of teams in a natural context. Medicine (Baltimore) 2016;95:e3885. [Crossref] [PubMed]
- Janssen A, Robinson T, Brunner M, et al. Multidisciplinary teams and ICT: A qualitative study exploring the use of technology and its impact on multidisciplinary team meetings. BMC Health Serv Res 2018;18:444. [Crossref] [PubMed]
- Horsky J, Schiff GD, Johnston D, et al. Interface design principles for usable decision support: A targeted review of best practices for clinical prescribing interventions. J Biomed Inform 2012;45:1202-16. [Crossref] [PubMed]
- Sittig DF, Wright A, Osheroff JA, et al. Grand challenges in clinical decision support. J Biomed Inform 2008;41:387-92. [Crossref] [PubMed]
- Stout RJ, Salas E, Kraiger K. The role of trainee knowledge structures in aviation team environments. Int J Aviat Psychol 1997;7:235-50. [Crossref] [PubMed]
- Cannon-Bowers JA, Salas E, Converse S. Shared mental models in expert team decision making. In: Castellan NJ Jr. editor. Individual and Group Decision Making. Hillsdale, NJ, US.: Lawrence Erlbaum Associates, Inc.; 1993:221-46.
- Cannon-Bowers JA, Tannenbaum SI, Salas E, et al. Defining team competencies and establishing team training requirements. In: Guzzo R, Salas E. editors. Team effectiveness and decision making in organizations. San Francisco, CA: Jossey-Bass; 1995:333-80.
- McIntyre RM, Salas E. Measuring and managing for team performance: Emerging principles from complex environments. In: Guzzo R, Salas E. editors. Team Effectiveness and Decision Making in Organizations. San Francisco, CA: Jossey-Bass; 1995:9-45.
- Navani N, Fisher DJ, Tierney JF, et al. The Accuracy of Clinical Staging of Stage I-IIIa Non-Small Cell Lung Cancer: An Analysis Based on Individual Participant Data. Chest 2019;155:502-9. [Crossref] [PubMed]
- Heineman DJ, ten Berge MG, Daniels JM, et al. The Quality of Staging Non-Small Cell Lung Cancer in the Netherlands: Data From the Dutch Lung Surgery Audit. Ann Thorac Surg 2016;102:1622-9. [Crossref] [PubMed]
- Detterbeck F. Editorial: What is quality and does it matter? J Thorac Oncol 2009;4:279-80. [Crossref] [PubMed]
- Farjah F, Flum DR, Ramsey SD, et al. Multi-modality mediastinal staging for lung cancer among medicare beneficiaries. J Thorac Oncol 2009;4:355-63. [Crossref] [PubMed]
- Osarogiagbon RU, Phelps G, McFarlane J, et al. Causes and consequences of deviation from multidisciplinary care in thoracic oncology. J Thorac Oncol 2011;6:510-6. [Crossref] [PubMed]
- Westerhuis W. Kwaliteitscriteria multidisciplinair overleg (mdo) [Internet]. Integraal Kankercentrum Nederland 2016. p 21. Available online: https://www.iknl.nl/getmedia/4dea4687-6c96-42cb-8860-72d1adb0e9f7/Kwaliteitscriteria_multidisciplinair_overleg_2016_IKNL.pdf
- Kaba A, Wishart I, Fraser K, et al. Are we at risk of groupthink in our approach to teamwork interventions in health care? Med Educ 2016;50:400-8. [Crossref] [PubMed]
- Klein GA, Sullivan J. Sources of power: How people make decisions. Leadersh Manag Eng 2001;1:21. [Crossref]
- Ten Have E. Mdo gebaat bij heldere afspraken [Internet]. Vol. 36, Medisch Contact 2016. Available online: https://www.medischcontact.nl/nieuws/laatste-nieuws/artikel/mdo-gebaat-bij-heldere-afspraken.htm
- Reason JT. Human Error. Cambridge: Cambridge University Press; 1990.
- Soukup T, Lamb BW, Weigl M, et al. An Integrated Literature Review of Time-on-Task Effects With a Pragmatic Framework for Understanding and Improving Decision-Making in Multidisciplinary Oncology Team Meetings. Front Psychol 2019;10:1245. [Crossref] [PubMed]