An immune-related gene expression signature predicts brain metastasis in lung adenocarcinoma patients after surgery: gene expression profile and immunohistochemical analyses
Introduction
When cancer spreads to the brain, it results in a dangerous process. Approximately 20% of all brain metastases (BMs) are caused by non-small cell lung cancer (NSCLC) (1). In the past, the prognosis of NSCLC was particularly poor, but the survival and BM detection rates have slowly increased owing to the development of targeted treatments and immune checkpoint inhibitors. If NSCLC advances to BM, the average survival time is very poor, ranging from 4 to 6 months (2). In breast cancer, where BM is more frequent, biomarkers that predict BM have been studied extensively (3,4), however, little research has been conducted on NSCLC.
Immunological mediators are known to play an important role in the metastasis of primary tumors to specific organs. Primary cancer cells develop immunological mediators that can metastasize to specific organs, and tumor cells move to specific organs via these immunological mediators. In a breast cancer model, breast cancer cells expressing CXC chemokine receptor 4 (CXCR4) and CC motif receptor 7 (CCR7) were found to bind to CXC motif chemokine ligand 12 (CXCL12) of the lymph nodes or to chemokine ligand 21 (CCL21) of the lungs and metastasize to the lymph nodes or lungs (5,6). Breast cancer results in frequent bone metastasis by guiding breast cancer cells that secrete CXCR4 to the bones (7). NSCLC cells also produce interleukin (IL) 7, which promotes bone metastasis (8). Immunological mediators that promote BM have also been reported. Breast cancer cells promote BM by expressing the IL-6 receptor (4). In the breast cancer model, exosome integrin β3 promotes BM (3).
Previous studies have shown that the expression or mutation of some specific genes promotes BM in lung cancer (9-11). In a recent study by Tsakonas et al., a 12-gene immune signature was significantly downregulated in BM compared to matching primary tumors (12). Several previous studies, including Tsakonas et al., compared BM samples to matched primary lung tumor samples (12,13). When BM occurs, the prognosis is very poor; therefore, the most important goal of predicting BM is to do so before BM occurs. However, in previous studies (12-14), identifying the gene expression pattern before BM occurrence has proven difficult because brain samples that had already undergone BM were compared with matched primary lung tumor samples. Thus, to identify biomarkers that can predict BM before it occurs, it is necessary to compare primary lung tumor samples before the occurrence of BM and primary lung tumor samples for which BM has not been reported. Because the Tumor, Node, Metastasis (TNM) stage is an indicator that best reflects tumor progression, the higher the TNM stage, the more likely that BM will occur. Therefore, it is recommended to match TNM stages in patients with and without BM.
Here, we compared primary lung adenocarcinoma (LUAD) samples prior to BM with TNM staging-matched primary LUAD samples for which no BM was reported using gene expression profiling. The gene expression profiling results were verified by immunohistochemistry (IHC). Since brain samples were available from a small number of BM groups, the gene expression profiles between primary lung cancer samples and matched brain samples were also compared. Subgroup analysis was performed to determine any changes in gene expression profiles based on TNM stage. We present the following article in accordance with the TRIPOD reporting checklist (available at: http://dx.doi.org/10.21037/tlcr-20-1056).
Methods
The study was conducted in accordance with the Declaration of Helsinki (as revised in 2013). The study was approved by the Institutional Review Board of the Ajou University School of Medicine (AJIRB-BMR-KSP-17-357) and individual consent for this retrospective analysis was waived.
Study population
Figure 1 shows the strategy employed in the present study. Patient clinicopathologic information is summarized in Table S1. All patients were diagnosed with LUAD after undergoing pulmonary resection at Ajou University Hospital. The 36 patients from the discovery dataset had undergone surgery between 2005 and 2010, and the 36 patients from the validation dataset had undergone surgery between 2011 and 2015. All patients underwent a brain computerized tomography (CT) scan or magnetic resonance imaging (MRI) before surgery, but no BM was found. Of the patients in each dataset, half displayed BM during follow-up after surgery while the other half did not. All tumor samples were obtained from surgical specimens; therefore, they were taken before BM. Brain CT or MRI scans were used to detect BM. TNM stages can also contribute to BM because different tumor stages have different degrees of tumor aggression. Therefore, in each dataset, a sample with the same tumor stage as that of the group with BM was randomly selected for the group without BM. Patients with missing data relating to the TNM staging system were excluded. The median follow-up time was 34 months (range, 4–82 months).
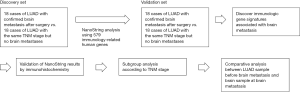
Gene expression analysis using NanoString Technologies
A total of 579 immunology-related human genes were used for NanoString analysis (nCounter GX Human Immunology V2 Assay Kit; NanoString Technologies, Seattle, WA, USA) (15). Total RNA (5 µL) was mixed with the reporter code and capture probe sets. When the hybridization reaction was complete, the sample was immediately transferred to the preparation station, and a high-sensitivity protocol was applied. Samples made in this way were scanned using the nCounter Digital Analyzer (NanoString). Data were normalized using the geometric mean of the positive control counts and a housekeeping gene. The 11-gene signature score was determined by applying a log10 transformation and calculating the average of the genes involved. The gene expression heatmap was plotted and analyzed using ClustVis software (16). The DAVID Bioinformatics Resources 6.8 tool was used for Kyoto Encyclopedia of Genes and Genomes (KEGG) pathway analysis (17).
IHC stain
Pathological tumor staging was determined based on the eighth edition of the TNM classification. Tissue microarrays were used for IHC staining. Two cores of 2 mm diameter were collected per patient. IHC was performed using a Benchmark XT automatic IHC staining device with an OptiView DAB IHC Detection Kit (Ventana Medical Systems, Tucson, AZ, USA). Information on the antibodies used is summarized in Table S2.
The intensity of cytoplasmic or membranous expression of tumor cells was determined on a four-point scale: 0 (no staining), 1 (faint = light yellow), 2 (moderate = yellow-brown), and 3 (strong = brown). The percentage (0–100%) of expression was also calculated. We used the H-score method for the determination of IHC staining (18). The definition of the overall H-score (0–300) is the intensity of positive cells multiplied by the percentage. IHC stainings were interpreted independently by two pathologists without prior knowledge of clinicopathological data. When discrepancies occurred, the mean value was used.
Statistical analysis
Gene expression normalization, fold-changes and p value between group with and without BM were obtained using the nanoString nSolver analysis software (nanoString technologies Inc.). The false discovery rate (FDR) was calculated using the ‘fdrtool’ package in R (The R Foundation, Vienna, Austria). Receiver operating characteristic (ROC) curves for the potential BM biomarkers were generated to determine the appropriate cutoff values. The Youden index was used as a method to select the optimal cutoff for gene expression levels to account for potential clinical benefits (19). IBM SPSS Statistics 25 software (IBM, Armonk, NY, USA) or R version 3.5.3 (The R Foundation) was used for the analyses, and a P value <0.05 was considered statistically significant.
Results
Identification of BM-associated genes and pathway enrichment analysis
To identify the immune-related gene expression signatures associated with BM, 36 samples from patients with LUAD were used for the discovery dataset. In the discovery dataset, the expression levels of 117 genes were elevated in the BM group compared to the non-BM group, and the P value was <0.05. Next, we evaluated the results of the discovery set again in the validation set. In the validation set, the gene signature was purified by removing genes that did not reach a P value <0.05 for a positive association with BM, which yielded 28 purified genes; 28 genes had an FDR <0.05 in all dataset. We added genes with significantly elevated expression in the brain metastasis (BM) group of the discovery, validation or both database (Appendix I—Supplementary data 1-3).
Previous studies have reported that BM is associated with cytokine and chemokine pathways (3,4). Therefore, a pathway enrichment analysis was performed using 28 genes to identify the pathways leading to BM. In the KEGG pathway analysis, 13 pathways were found with P values <0.05 (Table S3). Among the 13 KEGG pathways, cytokine-cytokine receptor interaction was identified as a pathway related to cytokines and chemokines. Five genes were related to the cytokine-cytokine receptor interaction KEGG pathway, including CCL18, CCL23, CSF2, IFNAR2, and IL6ST. Because it is believed that these five genes play an important role, the expression levels of the corresponding genes were verified using IHC. CCL23, CSF2, and IFNAR2 protein expression levels were not confirmed despite several trials. The protein expression of CCL18 in the BM group was higher in the discovery and validation sets than in the group without BM (P=0.075 and P=0.047, respectively). IL6ST protein expression in the BM group was also higher in the discovery and validation sets than in the group without BM (P=0.039 and P=0.046, respectively).
To select the genes most closely related to BM, genes with a P value <0.05 and a fold change >2 were selected. In the discovery set, 15 out of 28 genes had a fold change >2. In the validation set, 11 out of 15 genes had a fold change >2. Finally, we selected 11 genes that are very closely related to BM (DPP4, ICAM1, RARRES3, CD74, CSF2, HLA-DMB, ICAM5, MUC1, CCL18, RORC, and ICAM4). Figure 2 shows the heat maps of the unsupervised clustering analyses of the discovery and validation sets for the 11 genes. These genes are closely related to BM, as indicated by an increased frequency of BM at locations where each gene’s expression level is high. Figure S1 shows the expression levels of the 11 genes according to BM in the discovery set. The genes were significantly upregulated in the group with BM compared to the group without BM (P<0.05 for all 11 genes). Figure S2 shows the expression levels of the 11 genes according to BM in the validation set. Again, the genes were significantly elevated in the group with BM compared to the group without BM (P<0.05 for all 11 genes). The log10 transformed 11-gene signature was also significantly upregulated in the group with BM compared to the group without BM (P=0.002 for the discovery set and P=0.001 for the validation set; Figure 3A,B). ROC analysis for BM status over the range of gene expression levels demonstrated high discriminatory abilities of the genes. In the discovery set, the area under the curve (AUC) values ranged from 0.676 to 0.793 (Figure 3C). In the validation set, the AUC values ranged from 0.676 to 0.815 (Figure 3D). We performed ROC analysis using the log10 transformed 11-gene signature. The AUC values and their 95% confidence intervals (CIs) were 0.750 (0.579–0.921) for the discovery set and 0.787 (0.639–0.935) for the validation set (Figure 3E,F). To identify the potential clinical usefulness of the 11-gene signature in predicting BM, a Youden index-based cutoff value for the 11-gene signature was calculated (19) (cutoff 2.98, sensitivity 94%, and specificity 62% for the discovery set; cutoff 2.86, sensitivity 100%, and specificity 50% for the validation set). In the discovery set, the positive predictive value (PPV, BM rate above the cutoff) was 70.8%, and the negative predictive value (NPV, no BM rate below the cutoff) was 91.6%. In the validation set, the PPV was 66.6%, and the NPV was 100%.
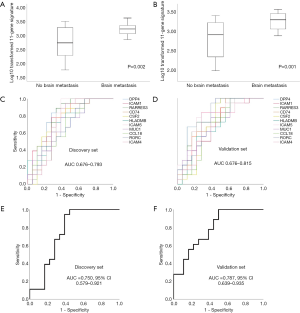
Validation of the 11-gene signature by IHC staining
We confirmed that the 11-gene signature can predict BM in advance by analyzing the mRNA expression levels of the genes. Next, we aimed to verify whether the protein expression levels of the 11 genes can predict BM using IHC. Of the 11 genes, CSF2 was not expressed in cancer tissues, and MUC1 and RORC were strongly expressed in tumor cells of all patients; therefore, it was confirmed that BM could not be predicted using CSF2, MUC1, and RORC. Because the amount of mRNA expression can affect protein expression, we calculated the average of the 11 gene mRNA expressions levels: DPP4 was 1,025, ICAM1 was 3,313, RARRES3 was 1,015, CD74 was 49,800, CSF2 was 30, HLA-DMB was 886, ICAM5 was 94, MUC1 was 8,156, CCL18 was 1,409, RORC was 239, and ICAM4 was 459. The mRNA expression level of MUC1 was high, and that of CSF2 was low. The level of mRNA expression in MUC1 and CSF2 may affect protein expression. Finally, eight genes were analyzed by IHC. The representative IHC expression levels of the eight genes in the groups with or without BM are summarized in Figure 4 and Figure S3. As shown in the representative figure, IHC expression of the eight genes was higher in the group with BM than in the group without BM. Figure S4 shows the protein expression levels of the genes according to BM in the discovery set. With the exception of CCL18, the genes were significantly upregulated in the group with BM compared to the group without BM (P<0.05 for seven genes and P=0.075 for CCL18). Figure S5 shows the expression levels of the eight genes according to BM in the validation set. With the exceptions of ICAM1 and RARRES3, the genes were significantly upregulated in the group with BM compared to the group without BM (P<0.05 for six genes, P=0.168 for ICAM1, and P=0.154 for RARRES3). The mean value of the IHC expression levels of the eight genes was also significantly higher in the group with BM than in the group without BM (P<0.001 for the discovery set and P=0.002 for the validation set; Figure 5A,B). Similar to the NanoString method, ROC analysis was performed to confirm the predictive power of each gene’s protein expression in the discovery and validation sets. In the discovery set, the AUC values ranged from 0.682 to 0.810 (Figure 5C). In the validation set, the AUC values ranged from 0.645 to 0.753 (Figure 5D). We performed ROC analysis using the average of the eight gene IHC expression levels. The AUCs and their 95% CIs were 0.844 (0.715–0.973) for the discovery set and 0.795 (0.639–0.950) for the validation set (Figure 5E,F). To identify the potential clinical usefulness of eight-gene IHC expression in predicting BM, a Youden index-based cutoff value was calculated (cutoff 127.5, sensitivity 83%, and specificity 72% for the discovery set; cutoff 146.2, sensitivity 72%, and specificity 84% for the validation set). In the discovery set, the PPV was 75%, and the NPV was 81.2%. In the validation set, the PPV was 81.2%, and the NPV was 75%.
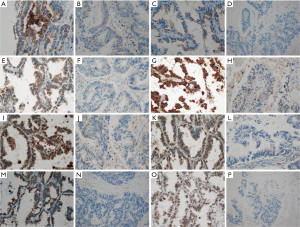
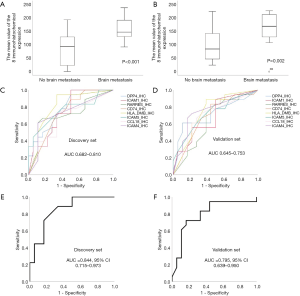
Relationship between the 11-gene signature and TNM stage
Because different tumor stages have different tumor aggressiveness, the expression of BM-associated genes or the prediction of BM may differ for each tumor stage. The subgroup analysis according to TNM stage was performed on a total of 72 patients. We compared the 11-gene signature profiles and IHC expression profiles for each TNM stage in 36 patients with BM. The 11-gene signature profile was not significantly different based on stage (stage I vs. II: P=0.978 and stage I vs. III: P=0.565; Figure 6A). The average IHC expression levels of the eight genes were also not significantly different based on stage (stage I vs. II: P=0.235 and stage I vs. III: P=0.501; Figure 6B). Next, we analyzed whether the expression levels of the BM-associated genes differ according to TNM stage. The 11-gene signature profile was significantly upregulated in the group with BM compared to the group without BM at all stages (P<0.05 for all stages; Figure 6C,D,E). The average of the eight-gene IHC expression was also significantly upregulated in the group with BM compared to the group without BM at all stages (P<0.05 for all stages; Figure 6F,G,H).
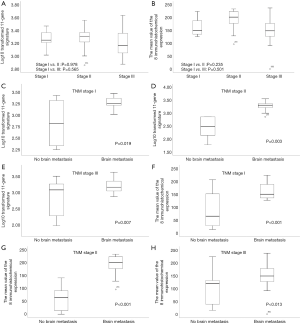
Relationship between the LUAD sample before BM and the brain sample upon BM
To confirm whether there is a change in the immune-related expression score when cancer cells metastasize to the brain, we evaluated the relationship between the LUAD sample before BM and the brain sample upon BM. Among the 36 BM cases, 9 brain samples were obtained. Figure 7A shows the change in the 11-gene signature profile in each patient’s BM. In the NanoString analysis, there was no significant difference between the LUAD sample before BM and the brain sample upon BM (P=0.218; Figure 7B). Next, we compared the brain samples of the group with BM and the lung samples of the group without BM after surgery. The 11-gene signature profile of the brain samples from the group with BM was statistically higher than that of the lung samples in the group without BM after surgery (P<0.001; Figure 7C).
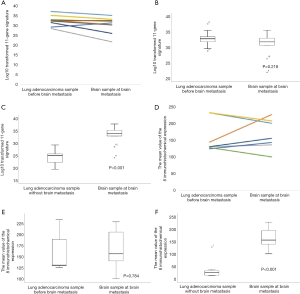
We then validated the NanoString results using IHC. Of the nine brain samples, only seven were analyzed because two patients had no remaining paraffin tissue. Figure 7D shows the change in the average of the eight-gene IHC expression levels in each patient’s BM. In IHC analysis, there was no significant difference between the LUAD sample before BM and the brain sample upon BM (P=0.784; Figure 7E). The average of the eight-gene IHC expression levels of the brain samples in the group with BM was statistically higher than that of the lung samples in the group without BM after surgery (P<0.001; Figure 7F).
The results suggest that there is no change in the immune-related gene expression profile during BM. However, upon BM, the immune-related gene expression profile of the brain samples was higher than that of the lung samples from the group without BM.
Discussion
Our research resulted in several important findings. First, we identified 11 immune-related gene expression signatures that could predict BM prior to its occurrence in LUAD. We verified the 11 immune-related gene expression signatures using IHC. Second, we found that BM is closely related to the cytokine-cytokine receptor interaction pathway through KEGG pathway enrichment analysis. Third, the 11 immune-related gene expression signatures did not change according to TNM stage, and BM could be predicted at all stages. Fourth, there were no differences in the 11 immune-related gene expression signatures between the primary LUAD samples and the matched brain samples.
Based on subgroup analysis, there was no significant difference in the 11 immune-related gene expression signatures according to TNM stage, and the gene expression profile at all TNM stages was able to predict BM. Because the 11 immune-related gene expression signatures are related to BM at all TNM stages, gene expression profiles can be utilized at any stage. Particularly in the early stage, if we identify patients with a higher risk of developing BM, personalized follow-up with a focus on BM and better treatments for these patients are possible.
Several previous studies have compared BM samples to matched primary lung tumor samples (12,13). Tsakonas et al. reported that a 12-gene immune signature was significantly reduced in BM samples compared to matched primary tumor samples (12). Wang et al. reported that alterations in genes encoding the CDK4/CCND1, CDKN2A/2B, and PI3K signaling pathways were found more frequently in BM samples than in paired primary lung tumor samples (13). However, in our study, there were no significant differences in the 11 immune-related gene expression signatures between the BM samples and the paired primary lung tumor samples. In addition, there was no difference in gene expression signature according to TNM stage. Two previous studies have hypothesized that primary tumors more often cause BM by acquiring changes in certain genes that were not initially present. However, our study suggests that certain genes are highly expressed in the early stages of cancer, which may lead to BM.
In our study, five genes (CCL18, CCL23, CSF2, IFNAR2, and IL6ST) involved in the cytokine-cytokine receptor interaction pathway were found to be closely related to BM. Su et al. also reported that cytokine-cytokine receptor interaction was the most significantly enriched signaling pathway in LUAD BM using microarray analysis of cDNA expression profiles (20). Among the genes associated with the cytokine-cytokine receptor interaction identified by Su et al., CCL18 was included in our results (20). The chemokine signaling pathway, an important immunological mediator pathway, was also elevated in BM samples compared to primary LUAD samples (20). In experiments using the Gene Expression Omnibus database, the cytokine-cytokine receptor interaction pathway was upregulated in HER2-positive breast cancer with BM (21). When breast cancer metastasizes to the dura and brain parenchyma, the gene expression profile is different (22). The most significantly different KEGG pathway was the cytokine-cytokine receptor interaction pathway (22). The reason why this pathway is important in BM is explained by the “seed-and-soil” theory. Primary cancer cells develop cytokines that can metastasize to a specific site, and tumor cells move to specific organs using these cytokines (23).
We verified the results of the NanoString analysis by IHC. In the clinical field, the NanoString method is expensive, requires many samples, and is difficult to set up. However, IHC is advantageous because it is installed in most hospitals and is not expensive. Of the 11 genes, CSF2 was not expressed in cancer tissues while MUC1 and RORC were strongly expressed in tumor cells from all patients. In our results, the mRNA expression level of MUC1 was relatively high, whereas that of CSF2 was low. In fact, MUC1 mRNA expression was too high to detect differences in protein expression, and CSF2 was too low to detect differences in protein expression. Thus, IHC analysis of the eight genes may be helpful in predicting BM in hospitals with IHC setups.
Our study had some limitations. First, we did not perform sequencing analysis on the 11 genes with increased expression. Second, in addition to tumor cells, tumor stroma/microenvironment is important for the expression of immune-related genes; however, NanoString analysis cannot differentiate the two areas. In IHC staining, protein expression was measured only in tumor cells, and results that were similar to those of the NanoString analysis were obtained. Therefore, it is expected that tumor cells have a greater influence on gene expression than tumor stroma/microenvironment, even in NanoString analysis. Third, because our findings could not be verified using the external validation set, we need to confirm the results on an external validation set with a larger sample size.
Conclusions
We identified a unique gene upregulation pattern in primary LUAD samples prior to BM compared to primary LUAD samples with no BM, especially for genes related to the cytokine-cytokine receptor interaction pathway. The unique gene pattern was verified by IHC and can be used at all TNM stages.
Acknowledgments
Funding: This research was supported by Basic Science Research Program through the National Research Foundation of Korea (NRF) funded by the Ministry of Science, ICT (NRF-2017R1C1B5076342 for Young Wha Koh) and the faculty research fund (Ajou translational research fund 2018) of Ajou University School of Medicine to Young Wha Koh and Seokjin Haam (M-2018-C0460-00035). The funding provider had no role in research design, data collection and analysis, publication decisions or manuscript preparation.
Footnote
Reporting Checklist: The authors have completed the TRIPOD reporting checklist. Available at: http://dx.doi.org/10.21037/tlcr-20-1056
Data Sharing Statement: Available at: http://dx.doi.org/10.21037/tlcr-20-1056
Conflicts of Interest: All authors have completed the ICMJE uniform disclosure form (available at: http://dx.doi.org/10.21037/tlcr-20-1056). The authors have no conflicts of interest to declare.
Ethical Statement: The authors are accountable for all aspects of the work in ensuring that questions related to the accuracy or integrity of any part of the work are appropriately investigated and resolved. The study was conducted in accordance with the Declaration of Helsinki (as revised in 2013). The study was approved by the Institutional Review Board of the Ajou University School of Medicine (AJIRB-BMR-KSP-17-357) and individual consent for this retrospective analysis was waived.
Open Access Statement: This is an Open Access article distributed in accordance with the Creative Commons Attribution-NonCommercial-NoDerivs 4.0 International License (CC BY-NC-ND 4.0), which permits the non-commercial replication and distribution of the article with the strict proviso that no changes or edits are made and the original work is properly cited (including links to both the formal publication through the relevant DOI and the license). See: https://creativecommons.org/licenses/by-nc-nd/4.0/.
References
- Barnholtz-Sloan JS, Sloan AE, Davis FG, et al. Incidence proportions of brain metastases in patients diagnosed (1973 to 2001) in the Metropolitan Detroit Cancer Surveillance System. J Clin Oncol 2004;22:2865-72. [Crossref] [PubMed]
- Mehta MP, Rodrigus P, Terhaard CH, et al. Survival and neurologic outcomes in a randomized trial of motexafin gadolinium and whole-brain radiation therapy in brain metastases. J Clin Oncol 2003;21:2529-36. [Crossref] [PubMed]
- Hoshino A, Costa-Silva B, Shen TL, et al. Tumour exosome integrins determine organotropic metastasis. Nature 2015;527:329-35. [Crossref] [PubMed]
- Sierra A, Price JE, Garcia-Ramirez M, et al. Astrocyte-derived cytokines contribute to the metastatic brain specificity of breast cancer cells. Lab Invest 1997;77:357-68. [PubMed]
- Müller A, Homey B, Soto H, et al. Involvement of chemokine receptors in breast cancer metastasis. Nature 2001;410:50-6. [Crossref] [PubMed]
- Weilbaecher KN, Guise TA, McCauley LK. Cancer to bone: a fatal attraction. Nat Rev Cancer 2011;11:411-25. [Crossref] [PubMed]
- Lu X, Kang Y. Organotropism of breast cancer metastasis. J Mammary Gland Biol Neoplasia 2007;12:153-62. [Crossref] [PubMed]
- Roato I, Caldo D, Godio L, et al. Bone invading NSCLC cells produce IL-7: mice model and human histologic data. BMC Cancer 2010;10:12. [Crossref] [PubMed]
- Grinberg-Rashi H, Ofek E, Perelman M, et al. The expression of three genes in primary non-small cell lung cancer is associated with metastatic spread to the brain. Clin Cancer Res 2009;15:1755-61. [Crossref] [PubMed]
- Krencz I, Sebestyén A, Fábián K, et al. Expression of mTORC1/2-related proteins in primary and brain metastatic lung adenocarcinoma. Hum Pathol 2017;62:66-73. [Crossref] [PubMed]
- Wilson GD, Johnson MD, Ahmed S, et al. Targeted DNA sequencing of non-small cell lung cancer identifies mutations associated with brain metastases. Oncotarget 2018;9:25957-70. [Crossref] [PubMed]
- Tsakonas G, Lewensohn R, Botling J, et al. An immune gene expression signature distinguishes central nervous system metastases from primary tumours in non-small-cell lung cancer. Eur J Cancer 2020;132:24-34. [Crossref] [PubMed]
- Wang H, Ou Q, Li D, et al. Genes associated with increased brain metastasis risk in non-small cell lung cancer: Comprehensive genomic profiling of 61 resected brain metastases versus primary non-small cell lung cancer (Guangdong Association Study of Thoracic Oncology 1036). Cancer 2019;125:3535-44. [Crossref] [PubMed]
- Iwamoto T, Niikura N, Ogiya R, et al. Distinct gene expression profiles between primary breast cancers and brain metastases from pair-matched samples. Sci Rep 2019;9:13343. [Crossref] [PubMed]
- Geiss GK, Bumgarner RE, Birditt B, et al. Direct multiplexed measurement of gene expression with color-coded probe pairs. Nat Biotechnol 2008;26:317-25. [Crossref] [PubMed]
- Metsalu T, Vilo J. ClustVis: a web tool for visualizing clustering of multivariate data using Principal Component Analysis and heatmap. Nucleic Acids Res 2015;43:W566-70. [Crossref] [PubMed]
- Huang W, Sherman BT, Lempicki RA. Systematic and integrative analysis of large gene lists using DAVID bioinformatics resources. Nat Protoc 2009;4:44-57. [Crossref] [PubMed]
- McCarty KS Jr, Szabo E, Flowers JL, et al. Use of a monoclonal anti-estrogen receptor antibody in the immunohistochemical evaluation of human tumors. Cancer Res 1986;46:4244s-8s. [PubMed]
- Youden WJ. Index for rating diagnostic tests. Cancer 1950;3:32-5. [Crossref] [PubMed]
- Su H, Lin Z, Peng W, et al. Identification of potential biomarkers of lung adenocarcinoma brain metastases via microarray analysis of cDNA expression profiles. Oncol Lett 2019;17:2228-36. [PubMed]
- Lu X, Gao C, Liu C, et al. Identification of the key pathways and genes involved in HER2-positive breast cancer with brain metastasis. Pathol Res Pract 2019;215:152475. [Crossref] [PubMed]
- Rippaus N, Taggart D, Williams J, et al. Metastatic site-specific polarization of macrophages in intracranial breast cancer metastases. Oncotarget 2016;7:41473-87. [Crossref] [PubMed]
- Langley RR, Fidler IJ. The seed and soil hypothesis revisited--the role of tumor-stroma interactions in metastasis to different organs. Int J Cancer 2011;128:2527-35. [Crossref] [PubMed]