Identification of serum biomarkers to predict pemetrexed/platinum chemotherapy efficacy for advanced lung adenocarcinoma patients by data-independent acquisition (DIA) mass spectrometry analysis with parallel reaction monitoring (PRM) verification
Introduction
Lung carcinoma is the most commonly diagnosed malignancy worldwide, and is the leading cause of cancer-related mortality with still increasing incidence (1). Approximately 85% of lung carcinomas are non-small cell lung carcinoma (NSCLC) (2). Although targeted therapy such as epidermal growth factor receptor-tyrosine kinase inhibitor (EGFR-TKI) and immunotherapy have developed rapidly in recent years, a great number of NSCLC patients, who are not eligible for these therapies, still need to take chemotherapy. Pemetrexed-based chemotherapy uses a chemical analogous to folic acid which inhibits the folate-dependent metabolic processes essential for cell replication (3). It has been the most effective chemotherapy regimen for advanced non-squamous NSCLC with EGFR wild type, and pemetrexed maintenance therapy was preferred to given until disease progression or intolerable toxicity (4). However, the efficacy of pemetrexed plus platinum doublet chemotherapy varies considerably (5-7), indicating that many patients do not benefit from it. Drug resistance of targeted therapy, immunotherapy and chemotherapy were all inevitable problems, But EGFR mutation status and programmed cell death ligand 1 (PD-L1) protein expression were all effective predictive biomarkers for targeted therapy and immunotherapy. There is still no biomarker to predict the efficacy of chemotherapy.
Intense research effort has been invested in identifying prognostic biomarkers for predicting the efficacy of pemetrexed/platinum chemotherapy regimens in lung cancer and malignant pleural mesothelioma. In recent years, most of the studies on the prediction of chemotherapy efficacy in advanced lung adenocarcinoma have focused on the genomic level of tumor tissue. Among them, the nucleotide excision repair (NER) system including the excision repair cross complementing (ERCC) family and other gene mutations were the research hotspots. For example, in malignant pleural mesothelioma patients undergoing pemetrexed/platinum chemotherapy, RRM1 and ERCC1 expression were identified as independent prognosticators (8,9). But some mutations, such as ERCC1 C118T/C8092A and ERCC5 rs1047768 mutations showed the opposite results. Some retrospective studies showed that advanced lung adenocarcinoma patients with human epidermal growth factor receptor 2 (HER2) mutation who received first-line chemotherapy were associated with better efficacy than patients with HER2 wild type. However the relationship between HER2 gene status and the efficacy of first-line chemotherapy in the treatment of advanced lung adenocarcinoma is still controversial. Therefore it is still unclear to determine the influence of genetic variation on the prognosis of patients with lung adenocarcinoma who received chemotherapy and new researches beyond the genomic level is urgently needed. In addition to the characteristics of tumor genetic mutations, tumor environment is also one of the important reasons for the chemotherapy efficacy difference, which is a frontier field that has not been covered. For instance, an observational phase II study reported by Sun et al. showed that low thymidylate synthase (TS) protein levels in tumors evaluated by immunohistochemistry are a predictive marker of better response rate and longer progression-free survival (PFS) in patients with non-squamous NSCLC treated with pemetrexed/cisplatin (10,11). However, due to the relatively low sensitivity and specificity, these biomarkers have not been used in clinical practice. Mass spectrometry techniques are widely used in the investigation of biomarkers. Using matrix-assisted laser desorption ionization time-of-flight mass spectrometry (MALDI-TOF-MS)-based serum peptidome profiling, Wang et al. discovered 8 potential peptide biomarkers that can predict outcomes of pemetrexed plus platinum regimens for advanced lung adenocarcinoma patients (12). Furthermore, Tian et al. developed a 7-metabolite panel that was predictive of pemetrexed plus platinum doublet in NSCLC patients by serum metabolite profiling analysis (13).
With the development of more robust and quantitative proteomic analysis techniques, the progress in biomarker discovery and validation will be greatly accelerated (14-16). The recent emergence of data-independent acquisition (DIA) represents a major advance in protein quantification and is significant due to its capacity to conduct high-throughput quantitative proteomics. The parallel reaction monitoring (PRM) assay emerged as a targeted quantification mass spectrometer method with a high resolution and high mass accuracy mode. The newer combination methodologies of untargeted DIA and targeted PRM have demonstrated great potential in comprehensively revealing and validating predictive and prognostic candidate biomarkers for a variety of diseases such as cancer, liver failure, and hematological diseases. In this study, we aimed to identify potential protein/peptide biomarkers using this approach based on quantitative proteomics DIA and PRM to predict the efficacy of pemetrexed/platinum in advanced lung adenocarcinoma patients before treatment.
We present the following article in accordance with the MDAR reporting checklist (available at http://dx.doi.org/10.21037/tlcr-21-153).
Methods
Patients
Serum samples were collected from 20 stage IIIB or IV lung adenocarcinoma patients who received pemetrexed/platinum chemotherapy, as shown in Table 1. Chemotherapy regimens included cisplatin (75 mg/m2 q21d) or carboplatin [area under the curve (AUC) =5 q21d] in combination with pemetrexed (500 mg/m2 q21d). Patients who achieved partial response (PR) were placed into the “good response” group, and patients who had progressive disease (PD) were placed into the “poor response” group. Among the 20 patients, 12 were PR and 8 were PD patients. PFS was defined as the time from the first dose of chemotherapy to the date of disease progression or death from any cause. The study was approved by the Medical Ethics Committee of Peking University Cancer Hospital. All patients signed the “Informed consent of obtaining the patient sample to conduct a scientific study”, and none of the authors had access to the patients’ identities. All procedures performed in this study involving human participants were in accordance with the Declaration of Helsinki (as revised in 2013).
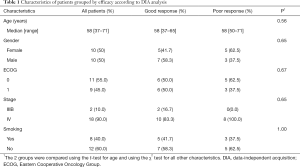
Full table
Serum sample collection
For sample collection, 7 mL of peripheral blood was drawn from the patients and collected with a serum separator tube 1 day before the chemotherapy treatment. Serum samples were separated by centrifugation at 1,000 g for 10 minutes at 4 °C and then stored in aliquots at –80 °C.
Sample preparation and DIA MS analysis
Protein extraction and peptide preparation
Protein extraction and peptide preparation were conducted by Novogene Co., Ltd. First, each sample was lysed with some DB lysis buffer (8 M Urea, 100 mM TEAB, pH 8.5) and centrifuged at 12,000 g for 15 min at 4 °C. Meanwhile, 20 µL serum from each sample was mixed as a pooled sample, and this pooled sample was used to construct the library for DIA protein identification. Then, proteins in the pooled sample were separated as high-abundance proteins and low-abundance proteins by the ProteoMinerTM protein enrichment kit (Bio-Rad). Each sample serum proteins and the low-abundance and high-abundance proteins from the pooled sample were then reduced with 2 mM of dithiothreitol (DTT) for 1 h at 56 °C and subsequently alkylated with sufficient iodoacetic acid for 1 h at room temperature in the dark. After acetone precipitation, the pellets were dissolved using 0.1 M triethylammonium bicarbonate (TEAB, pH 8.5) and 8 M urea buffer. Supernatant from each sample containing exactly 0.1 mg of protein was digested with Trypsin Gold (Promega) at 37 °C for 16 h, followed by a desalination procedure to remove high urea, and then dried by vacuum centrifugation.
Library construction
The low-abundance pooled sample peptides were fractionated using a C18 column (Waters BEH C18, 4.6×250 mm, 5 µm) on a Rigol L3000 HPLC operating at 1 mL/min, with the column oven being set to 50 °C. Mobile phases A [2% acetonitrile (ACN), adjusted pH 10.0 using ammonium hydroxide] and B (98% ACN, adjusted pH 10.0 using ammonium hydroxide) were used to develop a gradient elution. The solvent gradient was set as follows: 3% B, 5 min; 3–8% B, 0.1 min; 8–18% B, 11.9 min; 18–32% B, 11 min; 32–45% B, 7 min; 45–80% B, 3 min; 80% B, 5 min; 80–5% B, 0.1 min, 5% B, 6.9 min. The eluates were monitored at UV 214 nm, collected for a tube per minute, and finally merged into 4 fractions. The 4 fractions from the low-abundance peptides were dried in a vacuum. The high-abundance peptides and the 4 fractions of low-abundance peptides were reconstituted in 0.1% (v/v) formic acid (FA) in water. Then, 0.2 µL of standard peptides (iRT kit, Biognosys) were added into the peptide sample for subsequent analyses.
For transition library construction, shotgun proteomics analyses were performed using an EASY-nLCTM 1200 UHPLC system coupled with an Orbitrap Q Exactive HF-X mass spectrometer (Thermo Fisher Scientific) operating in the data-dependent acquisition (DDA) mode. The high-abundance peptides and 4 fractionated low-abundance peptides were injected into a home-made C18 Nano-Trap column (2 cm × 100 µm, 3 µm). Peptides were then separated onto a home-made analytical column (15 cm × 150 µm, 1.9 µm), using a 120 min linear gradient from 5% to 100% eluent B (0.1% FA in 80% ACN in eluent A (0.1% FA in H2O) at a flow rate of 600 nL/min. The detailed solvent gradient was as follows: 5–10% B, 2 min; 10–40% B, 105 min; 40–50% B, 5 min; 50–90% B, 3 min; 90–100% B, 5 min.
The Q-Exactive HF-X mass spectrometer was operated in positive polarity mode with a spray voltage of 2.3 kV and a capillary temperature of 320 °C. Full MS scans ranging from 350 to 1,500 m/z were acquired at a resolution of 60,000 (at 200 m/z) with automatic gain control (AGC) target value of 3×106 and a maximum ion injection time of 20 ms. The 40 most abundant precursor ions from the full MS scan were selected for fragmentation using higher-energy collisional dissociation (HCD) fragment analysis at a resolution of 15,000 (at 200 m/z) with an AGC target value of 5×104, a maximum ion injection time of 45 ms, normalized collision energy (NCE) of 27%, and intensity threshold of 2.2×104, and a dynamic exclusion parameter of 40 s.
MS analysis for each sample-DIA mode
Each serum sample peptide was reconstituted in 0.1% FA, mixed with 0.2 µL standard peptides (iRT kit, Biognosys), and injected into the EASY-nLCTM 1200 UHPLC system coupled with an Orbitrap Q Exactive HF-X mass spectrometer (Thermo Fisher Scientific) operating in DIA mode. The liquid conditions were the same as those of the DDA model for library construction. For DIA acquisition, the MS1 resolution was set to 60,000 (at 200 m/z), and the MS2 resolution was set to 30,000 (at 200 m/z). The m/z range covered from 350 to 1,500 m/z and was separated into 30 acquisition windows (Table S1). The full scan AGC target was set to 3×106, with an injection time of 50 ms. DIA settings included NCE of 27%, a target value of 1×106, and an automatic maximum injection time was set to automatic to allow the MS to continuously operate in the parallel ion filling and detection mode.
LC-MS/MS DIA data analysis
Data analysis and visualization of DIA data were conducted by Novogene Co., Ltd. using the Proteome Discoverer 2.2 (PD 2.2, Thermo Fisher Scientific) platform, Biognosys Spectronaut v. 9.0, and R statistical framework. MS2-based label-free quantification was carried out by analyzing DIA raw data using Biognosys Spectronaut v.9. Data analysis was carried out as described in Bruder et al. (17) with minor modifications.
Gene ontology (GO) and InterPro (IPR) analysis were conducted using the interproscan-5 program against the non-redundant protein database (including Pfam, PRINTS, ProDom, SMART, ProSiteProfiles, PANTHER) (18), and the Clusters of Orthologous Groups of proteins (COG) and the Kyoto Encyclopedia of Genes and Genomes (KEGG) databases were used to analyze the protein family and pathway. The enrichment pipeline (19) was used to perform the enrichment analysis of GO, IPR, and KEGG, respectively. The discriminative ability of candidate biomarkers was assessed by the AUC produced by receiver operating characteristic (ROC) curves.
Validation study by PRM
The equal amount peptides from each sample were mixed as QC sample for PRM method construction. PRM analysis was performed using the same UHPLC-MS/MS system as described in library construction except for the 3 combined fractions from QC; 1 µg of each fraction peptide was analyzed with the “label-free” method using the EASY-nLCTM 1200 UHPLC system and Q Exactive HF-X mass spectrometer (Thermo Fisher Scientific) for 60 min. The offline raw data were searched by Proteome Discoverer v.2.2 software. The “missed cleavage” was set as 0, and 1–3 unique peptides were selected for each protein.
After selecting the peptides, the information of the target peptide including m/z, charge number, and charge type, were input into the “inclusion list”. The mixed peptides described above were analyzed by a “full scan” followed by a “PRM” pattern. The chromatographic separation and full scan condition were the same as above. The PRM was set to a resolution of 30,000 (at 200 m/z) with an AGC target value of 5×104, a maximum ion injection time of 80 ms, and an NCE of 27%. The off-line data were analyzed by Skyline software to determine whether the selected peptides were usable based on reproducibility and stability.
Equal amounts of the trypsin-treated peptide of each sample were taken and spiked with an equal amount of the labeled peptide, DSPSAPVNVTVR, as an internal standard. Samples were analyzed by “full scan” followed by the “PRM” pattern as described above. The off-line data were analyzed by Skyline software, and the peak area was corrected using the internal standard peptide (20).
Results
Quantitative proteomics profiling of pretreatment serum specimens
We collected the data of 20 advanced lung adenocarcinoma patients who received pemetrexed/platinum chemotherapy. They were divided into 2 groups according to chemotherapy efficacy. Among them, 12 patients with PR were classified as the good response group, and 8 patients with PD were classified as the poor response group. Figure S1 shows the Kaplan-Meier survival analysis of the two groups, indicating a complete separation of patients with good and poor prognosis. Median PFS in the good response group and the poor response group were 15 and 1.5 months respectively (P<0.0001). DIA quantitative MS was used to investigate the changes in protein abundance.
Functional classification and pathway analysis of total proteins
Twenty serum samples were analyzed using a DIA-MS approach, and 6,725 peptides and 911 proteins were identified by Proteome Discoverer 2.2 software [false discovery rate (FDR) <5%]. A total of 5,816 peptides recognized in 447 proteins were detected by DIA-MS analysis. The molecular weight of the identified proteins ranged from 3.7 to 571.6 kDa, and the isoelectric points ranged from 4.09 to 11.52.
Then, the complete dataset was categorized according to the Protein ANalysis THrough Evolutionary Relationships (PANTHER) classification scheme. The proteins were categorized and displayed according to the percentage by GO in 3 domains: 128 biological processes, 125 molecular functions, and 408 cellular components (Figure 1A). The most common category was cellular components. To evaluate the effectiveness of the annotation process, all data were aligned to the COG database to predict and classify possible functions. As shown in Figure 1B, the top 3 most common categories are posttranslational modification, protein turnover, and chaperones; general function prediction only; and cell wall/membrane/envelope biogenesis. The KEGG pathway annotation of total proteins is shown in Figure 1C. It was interesting to note that many proteins were annotated in the infectious disease–related category. In Figure 1D, a great number of protein domains are classified into the immunoglobulin subsets.
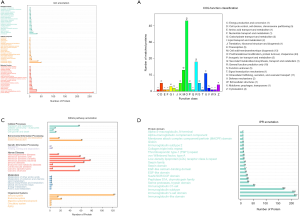
Functional categorization/classification and pathway analysis of differentially expressed proteins
We identified 23 significantly different expressed proteins between the groups of good and poor prognosis (Table 2). Figure 2 shows the volcano plots of these proteins. Proteins with statistically significant differential expression (≥1.2-fold, P<0.05) are located in the top right and left quadrants. Among them, 17 proteins were upregulated and 6 proteins were downregulated in the good prognosis group compared with the poor prognosis group.
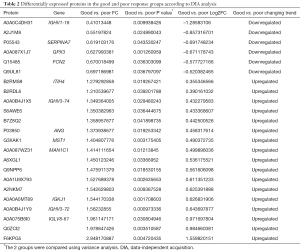
Full table
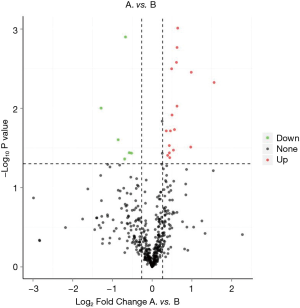
Figure 3A shows the proteins enriched in GO classified as biological processes, cellular components, and molecular functions. Figure 3B indicates that the most significantly enriched KEGG pathway is the thyroid hormone synthesis pathway, while Figure 3C shows the enriched Interpro (IPR) terms, including glutathione peroxidase (GPX), among others.
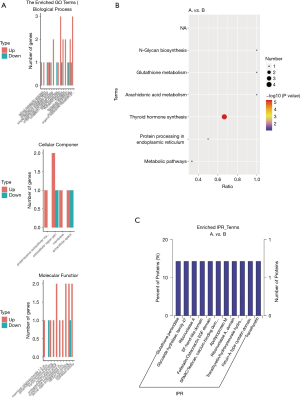
Assessment of the discriminative ability of candidate biomarkers by AUC
ROC analysis was performed, and the overall predictive accuracy of the 12 proteins that we proposed as potential biomarkers found in our study was assessed by AUC analysis (Figure 4). The AUC values for these proteins ranged from 0.885 to 0.698, with more than half (7 proteins) showing an AUC above 0.8. The protein with the highest AUC was GPX (A0A087X1J7, 0.885, coded by the GPX3 gene), followed by thyroxine-binding globulin (P05543, 0.833, coded by the SERPINA7 gene), interalpha (globulin) inhibitor H4 (plasma Kallikrein-sensitive glycoprotein) [B2RMS9, 0.823, coded by the Inter-Alpha-Trypsin Inhibitor Heavy Chain 4 (ITIH4) gene], albumin (F6KPG5, 0.823, coded by the albumin gene), ficolin-2 [Q15485, 0.814, coded by the ficolin-2 (FCN2) gene], alpha-1,2-mannosidase [A0A087WZ31, 0.812, coded by the Mannosyl-oligosaccharide alpha 1,2-mannosidase 1C (MAN1C1) gene], and hepatocyte growth factor-like protein (G3XAK1, 0.802, coded by the MST1 gene). In the good prognosis group, GPX, thyroxine-binding globulin, and ficolin-2 were downregulated compared with the poor response group, with the other 4 proteins being upregulated in the good response group.
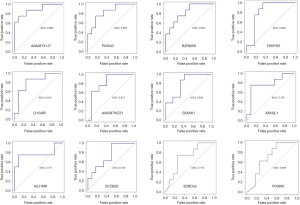
Biomarker validation by PRM
To further validate these results, we included the cohort of the above-mentioned 20 patients for DIA analysis and another cohort of 22 advanced lung adenocarcinoma patients who received pemetrexed/platinum chemotherapy regimens. Serum samples were collected before treatment. We used PRM, a targeted hypothesis substantiation proteomics method, to examine the candidate serum biomarkers (21). In total, 16 proteins were selected for quantification by PRM, including A0A0C4DH31, A2J1M8, P05543, A0A087X1J7, Q15485, B2RMS9, B2RDL6, A0A0B4J1X5, S6AWE6, B7Z8Q2, G3XAK1, A6XGL1, A0A1U9X793, A0A0A0MT89, A0A0B4J1Y9, and F6KPG5. Among the 42 patients, the tumors of 28 patients shrank, and these patients were classified as the good response group. The disease of 14 patients progressed, and these patients were considered the poor response group. In the validation process, we measured the quantity of 16 candidate proteins and calculated the expression diversity between the good response group and the poor response group. The quantification of 16 targeted proteins in 42 patients by PRM is shown in Figure 5. Quantitative validation by the PRM method correlated well with the previous DIA results. It was revealed that 10 promising proteins exhibited a similar up- or downregulation (Q15485, P05543, G3XAK1, B7Z8Q2, B2RMS9, A2J1M8, A0A1U9X793, A0A0B4J1X5, A0A0A0MT89, and A0A087X1J7) both in the DIA and PRM approaches (Table 3). Interestingly, GPX3 (A0A087X1J7) was significantly upregulated in the poor response group compared with the good response group, regardless of whether the DIA or PRM method was used (Figure 6).
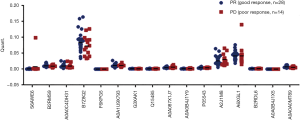
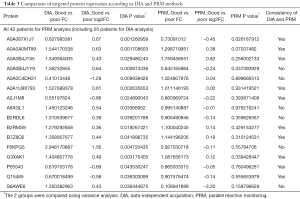
Full table
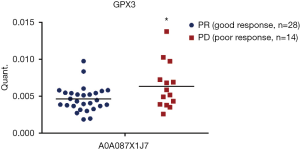
Discussion
In the present study, we used a DIA-based quantitative proteomics approach to investigate the potential prognostic biomarkers in serum. Twenty-three significantly different expressed proteins between the groups with a diverse response to chemotherapy were discovered, 7 of which had an AUC value over 0.8. The PRM method was used to validate the differences in protein levels of the biomarkers candidates we discovered, and 10 promising proteins exhibited similar up or down trends as observed with the DIA method.
Different protein expression levels in tumor and external environment might be closely related to the efficacy of chemotherapy, but no serum protein biomarkers have been successfully used in clinical practice. The recent emergence of DIA represents a major advance in protein quantification and is significant due to its capacity to conduct high-throughput quantitative proteomics. The newer combination methodologies of untargeted DIA and targeted PRM have demonstrated great potential in comprehensively revealing and validating predictive and prognostic candidate biomarkers for cancer patients (20).
GPX3, a member of the redox enzyme family of proteins, characterized by its vital antioxidant function, was found to be associated with tumorigenesis and chemotherapy response in various cancers (22). For instance, upregulation of GPX3 was found to predict poor prognosis in oral squamous cell carcinoma, hepatocellular carcinoma, and gastric carcinoma (23-25). Furthermore, GPX3 expression was reported to be related to the development of resistance to chemotherapy in breast cancer, ovarian cancer, and colorectal cancer (25,26). Both the DIA and PRM methods applied in our study indicated that GPX3 was significantly upregulated in the poor response group when compared with the good response group. However, the biological mechanism that causes chemotherapy resistance still needs to be clarified by further investigation.
FCN2 and MAN1C1 examined in our study have been shown to inhibit tumorigenesis or metastasis in different studies. FCN2 could inhibit epithelial-mesenchymal transition (EMT)-induced metastasis of hepatocellular carcinoma via TGF-β/Smad signaling (27), while MAN1C1 could suppress proliferation and invasion of clear cell renal cell carcinoma as a tumor inhibitor (28). These reports may explain the molecular mechanisms underlying the finding in our study in which MAN1C1 was upregulated in the good response group. On the other hand, FCN2 was downregulated in the good response group in our study which is inconsistent with previously reported results. Therefore whether FCN2 has the EMT inhibiting role in NSCLC patients still needs to be confirmed.
We also noticed that the candidate predictive biomarkers of the pemetrexed-based regimen discovered in this study contained several metabolic enzymes and endocrine-related proteins which are reflected in the surrounding tumor environment present in the individual patients. The interaction of a tumor with its surroundings might greatly affect the patient‘s response to chemotherapy. Meanwhile, the patient’s particular characteristics, such as metabolic and endocrine features, might have a significant influence on drug biotransformation and absorption, which in turn affect treatment efficacy. From this perspective, using a serum as a matrix for discovering prognostic biomarkers could have more benefits in revealing the global features of the tumor-host interaction, as opposed to focusing on the tumor samples themselves.
Our findings suggest that the DIA combined with the PRM MS approach was effective in identifying and validating the candidate serum predictive biomarkers for advanced lung adenocarcinoma patients who received pemetrexed/platinum chemotherapy. These potential biomarkers might be of great importance to predict pemetrexed/platinum chemotherapy efficacy. However, further studies are needed to explore the potential biological mechanisms of these biomarkers.
Acknowledgments
We acknowledge and thank all the medical staff who treated these patients. The authors also appreciate the academic support from the AME Lung Cancer Collaborative Group.
Funding: This research was funded by the Science Foundation of Peking University Cancer Hospital (18-02), Capital Clinical Characteristics and Application Research (no. Z181100001718104), and the Beijing Excellent Talent Cultivation Subsidy Young Backbone Individual Project (no. 2018000021469G264).
Footnote
Reporting Checklist: The authors have completed the MDAR reporting checklist. Available at http://dx.doi.org/10.21037/tlcr-21-153
Data Sharing Statement: Available at http://dx.doi.org/10.21037/tlcr-21-153
Conflicts of Interest: All authors have completed the ICMJE uniform disclosure form (available at http://dx.doi.org/10.21037/tlcr-21-153). The authors have no conflicts of interest to declare.
Ethical Statement: The authors are accountable for all aspects of the work in ensuring that questions related to the accuracy or integrity of any part of the work are appropriately investigated and resolved. The study was approved by the Medical Ethics Committee of Peking University Cancer Hospital. All patients signed “Informed consent of obtaining the patient sample to conduct a scientific study”, and none of authors had access to the patients’ identities. All procedures performed in this study involving human participants were in accordance with the Declaration of Helsinki (as revised in 2013).
Open Access Statement: This is an Open Access article distributed in accordance with the Creative Commons Attribution-NonCommercial-NoDerivs 4.0 International License (CC BY-NC-ND 4.0), which permits the non-commercial replication and distribution of the article with the strict proviso that no changes or edits are made and the original work is properly cited (including links to both the formal publication through the relevant DOI and the license). See: https://creativecommons.org/licenses/by-nc-nd/4.0/.
References
- Bray F, Ferlay J, Soerjomataram I, et al. Global cancer statistics 2018: GLOBOCAN estimates of incidence and mortality worldwide for 36 cancers in 185 countries. CA Cancer J Clin 2018;68:394-424. [Crossref] [PubMed]
- Molina JR, Yang PY, Cassivi SD, et al. Non-small cell lung cancer: epidemiology, risk factors, treatment, and survivorship. Mayo Clin Proc 2008;83:584-94. [Crossref] [PubMed]
- Joerger M, Omlin A, Cerny T, et al. The role of pemetrexed in advanced non small-cell lung cancer: special focus on pharmacology and mechanism of action. Curr Drug Targets 2010;11:37-47. [Crossref] [PubMed]
- Ettinger DS, Wood DE, Aggarwal C, et al. NCCN Guidelines Insights: Non-Small Cell Lung Cancer, Version 1.2020. J Natl Compr Canc Netw 2019;17:1464-72. [Crossref] [PubMed]
- Okamoto I, Nokihara H, Nomura S, et al. Comparison of Carboplatin Plus Pemetrexed Followed by Maintenance Pemetrexed With Docetaxel Monotherapy in Elderly Patients With Advanced Nonsquamous Non-Small Cell Lung Cancer: A Phase 3 Randomized Clinical Trial. JAMA Oncol 2020;6:e196828. [Crossref] [PubMed]
- Ramalingam SS, Dahlberg SE, Belani CP, et al. Pemetrexed, Bevacizumab, or the Combination As Maintenance Therapy for Advanced Nonsquamous Non-Small-Cell Lung Cancer: ECOG-ACRIN 5508. J Clin Oncol 2019;37:2360-7. [Crossref] [PubMed]
- Patel JD, Socinski MA, Garon EB, et al. PointBreak: a randomized phase III study of pemetrexed plus carboplatin and bevacizumab followed by maintenance pemetrexed and bevacizumab versus paclitaxel plus carboplatin and bevacizumab followed by maintenance bevacizumab in patients with stage IIIB or IV nonsquamous non-small-cell lung cancer. J Clin Oncol 2013;31:4349-57. [Crossref] [PubMed]
- Zucali PA, Giovannetti E, Destro A, et al. Thymidylate synthase and excision repair cross-complementing group-1 as predictors of responsiveness in mesothelioma patients treated with pemetrexed/carboplatin. Clin Cancer Res 2011;17:2581-90. [Crossref] [PubMed]
- Frischknecht L, Meerang M, Soltermann A, et al. Importance of excision repair cross-complementation group 1 and ribonucleotide reductase M1 as prognostic biomarkers in malignant pleural mesothelioma treated with platinum-based induction chemotherapy followed by surgery. J Thorac Cardiovasc Surg 2015;149:1539-46.e1. [Crossref] [PubMed]
- Sun JM, Ahn JS, Jung SH, et al. Pemetrexed Plus Cisplatin Versus Gemcitabine Plus Cisplatin According to Thymidylate Synthase Expression in Nonsquamous Non-Small-Cell Lung Cancer: A Biomarker-Stratified Randomized Phase II Trial. J Clin Oncol 2015;33:2450-6. [Crossref] [PubMed]
- Santarpia M, Rolfo C, Peters G, et al. On the pharmacogenetics of non-small cell lung cancer treatment. Expert Opin Drug Metab Toxicol 2016;12:307-17. [Crossref] [PubMed]
- Wang L, Tang C, Xu B, et al. Mass spectrometry-based serum peptidome profiling accurately and reliably predicts outcomes of pemetrexed plus platinum chemotherapy in patients with advanced lung adenocarcinoma. PloS One 2017;12:e0179000. [Crossref] [PubMed]
- Tian Y, Wang Z, Liu X, et al. Prediction of Chemotherapeutic Efficacy in Non-Small Cell Lung Cancer by Serum Metabolomic Profiling. Clin Cancer Res 2018;24:2100-9. [Crossref] [PubMed]
- Vidova V, Spacil Z. A review on mass spectrometry-based quantitative proteomics: Targeted and data independent acquisition. Anal Chim Acta 2017;964:7-23. [Crossref] [PubMed]
- Musa YR, Boller S, Puchalska M, et al. Comprehensive Proteomic Investigation of Ebf1 Heterozygosity in Pro-B Lymphocytes Utilizing Data Independent Acquisition. J Proteome Res 2018;17:76-85. [Crossref] [PubMed]
- Zheng X, Xu K, Zhou B, et al. A circulating extracellular vesicles-based novel screening tool for colorectal cancer revealed by shotgun and data-independent acquisition mass spectrometry. J Extracell Vesicles 2020;9:1750202. [Crossref] [PubMed]
- Bruderer R, Bernhardt O, Gandhi T, et al. Extending the limits of quantitative proteome profiling with data-independent acquisition and application to acetaminophen-treated three-dimensional liver microtissues. Mol Cell Proteomics 2015;14:1400-10. [Crossref] [PubMed]
- Jones P, Binns D, Chang HY, et al. InterProScan 5: genome-scale protein function classification. Bioinformatics 2014;30:1236-40. [Crossref] [PubMed]
- Huang da W. Sherman BT, Lempicki RA. Bioinformatics enrichment tools: paths toward the comprehensive functional analysis of large gene lists. Nucleic Acids Res 2009;37:1-13. [Crossref] [PubMed]
- Rauniyar N. Parallel Reaction Monitoring: A Targeted Experiment Performed Using High Resolution and High Mass Accuracy Mass Spectrometry. Int J Mol Sci 2015;16:28566-81. [Crossref] [PubMed]
- Bourmaud A, Gallien S, Domon B. Parallel reaction monitoring using quadrupole-Orbitrap mass spectrometer: Principle and applications. Proteomics 2016;16:2146-59. [Crossref] [PubMed]
- Chen B, Rao X, House M, et al. GPx3 promoter hypermethylation is a frequent event in human cancer and is associated with tumorigenesis and chemotherapy response. Cancer Lett 2011;309:37-45. [Crossref] [PubMed]
- Lee JR, Roh JL, Lee SM, et al. Overexpression of glutathione peroxidase 1 predicts poor prognosis in oral squamous cell carcinoma. J Cancer Res Clin Oncol 2017;143:2257-65. [Crossref] [PubMed]
- Liu T, Kan XF, Ma C, et al. GPX2 overexpression indicates poor prognosis in patients with hepatocellular carcinoma. Tumour Biol 2017;39:1010428317700410. [Crossref] [PubMed]
- Jardim BV, Moschetta MG, Leonel C, et al. Glutathione and glutathione peroxidase expression in breast cancer: an immunohistochemical and molecular study. Oncol Rep 2013;30:1119-28. [Crossref] [PubMed]
- Pelosof L, Yerram S, Armstrong T, et al. GPX3 promoter methylation predicts platinum sensitivity in colorectal cancer. Epigenetics 2017;12:540-50. [Crossref] [PubMed]
- Yang G, Liang Y, Zheng T, et al. FCN2 inhibits epithelial-mesenchymal transition-induced metastasis of hepatocellular carcinoma via TGF-β/Smad signaling. Cancer Lett 2016;378:80-6. [Crossref] [PubMed]
- Li H, Wang G, Yu Y, et al. α-1,2-Mannosidase MAN1C1 Inhibits Proliferation and Invasion of Clear Cell Renal Cell Carcinoma. J Cancer 2018;9:4618-26. [Crossref] [PubMed]
(English Language Editor: J. Gray)