A signature of estimate-stromal-immune score-based genes associated with the prognosis of lung adenocarcinoma
Introduction
Lung cancer is the primary cause of cancer death and the most frequently diagnosed malignancy globally. Worldwide lung cancer incidence and mortality were estimated 2.1 million new lung cancer cases and 1.8 million related deaths in 2018 (1). In the past 15 years, lung adenocarcinoma (LUAD) has become the most common subtype of lung cancer (2). For patients with LUAD, outcomes are variable and difficult to predict (3,4). Novel technologies and strategies for detection of LUAD and stratification of prognosis are being developed, yet standardized predictive and surrogate biomarkers still lack for personalized prognostication.
For many years, the prognostication of lung cancer was based on the tumor-node-metastasis (TNM) staging system, which allowed major clustering for management and prognostication (5). However, the static measurement method of TNM system does not fully reflect the dynamic process of the disease, such as the time to occurrence or death, and lacks relevant prognostic information: clinical outcomes can vary 8–47% among stage I–II lung cancer (6,7). The TNM staging assumes homogeneous growth, which is not reflected by actual neoplastic processes where also the host immune response plays a key role (8). Thus, novel biomarkers and risk evaluation models are warranted to improve prognostic prediction and precision treatment in addition to the TNM staging system.
LUAD tissue comprise tumor cells, infiltrating immune cells, tumor-related stromal cells, airway epithelial cells, and other normal endothelial cells. Recent studies have found that stromal cells play an essential role in the progression and treatment of cancer (9). Along with the tumor growth, immune cells also play important roles (10). Stromal and immune cells constitute the major components of the tumor microenvironment of LUAD (11,12). Therefore, the prognostic stratification of LUAD patients can be improved by comprehensive evaluation that includes metrics for stromal and immune cells to support personalize prognostication over the TNM staging system. The Estimation of STromal and Immune cells in MAlignant Tumor tissues using Expression data (ESTIMATE) algorithm was recently developed. It integrates the transcriptional profiles of cancer tissues and various infiltrating normal cells (13). Using this algorithm, the levels of infiltrating stromal and immune cells are predicted by calculating the scores based on gene signatures. Previously, researchers have used this method to predict the prognosis of patients with cancers of the digestive system cancer (14). However, data on stromal and immune scores in lung cancer are lacking.
In this study, we aimed to test immune and stromal scores in association with prognosis in LUAD. In particular, we aimed to investigate the interaction between the tumor and tumor immune microenvironment (TIME) during lung tumorigenesis and progression, and to introduce a new potential gene signature risk stratification model based on the estimate-stromal-immune scores that could be clinically used to predict the survival outcomes of LUAD patients. We present the following article in accordance with the TRIPOD reporting checklist (available at http://dx.doi.org/10.21037/tlcr-21-223).
Methods
Population
This retrospective study included derivation and validation cohorts from publicly available database, as follows:
- Derivation cohort: 514 LUAD samples from The Cancer Genome Atlas (TCGA);
- Validation cohorts: external validation was operated in two independent cohorts from the Gene Expression Omnibus (GEO) database:
- 96 LUAD samples from GSE68571 database;
- 442 LUAD samples from GSE72094 database.
Clinicopathological data for the corresponding patients were obtained including age, sex, pathologic stage, and survival outcome. The inclusion criteria for this study were available survival information and available expression data.
In accordance with the database policy, access to the dataset was obtained from TCGA and GEO. The study was conducted in accordance with the Declaration of Helsinki (as revised in 2013).
Estimation of stromal and immune scores
The gene expression data of LUAD tissues in derivation population were downloaded from the Genomic Data Commons (GDC, available at: http://potal.gdc.cancer.gov/) Data Portal on November 6, 2019. The FPKM (fragments per kilobase of exon per million reads mapped) method was used to quantify gene expression.
The expression matrix for estimating the stromal and immune scores was normalized by the ESTIMATE algorithm. Stromal and immune scores were calculated by performing single-sample gene set enrichment analysis. These scores formed the basis for the ESTIMATE score (15).
Statistical analysis
The association of the Estimate, stromal, and immune scores with the clinicopathological characteristics of LUAD patients was analyzed by comparing the score distributions among sex, pathologic T, pathologic M, and TNM stage subgroups.
Relationship of estimate-stromal-immune scores and prognosis
The Kaplan–Meier estimator was used to estimate the patients’ overall survival. The derivation cohort was divided into 2 groups based on their ESTIMATE, stromal, and immune scores: maximally selected rank statistics were employed to identify the optimal score cutoff for classifying patients by each score. The R package “maxstat” was used during identification (16). Log-rank tests were used to compare the survival outcomes of the 2 groups.
Identification of differentially expressed genes (DEGs)
The high and low ESTIMATE score groups (stromal or immune) were compared for gene expression: the DEGs were identified using the “limma” package in R (17). For the identification of DEGs, the threshold was a simultaneously absolute value of log2 (fold change) >1 and false discovery rate (FDR) adjusted P value <0.05. The low-risk group was used as reference: genes that were up-regulated in the high-risk group were considered “up-regulated DEGs” and those that were comparatively down-regulated were considered “down-regulated DEGs”. A heatmap was created to show the expression patterns of significant DEGs. Complete linkage was used to measure distances between unsupervised hierarchical clusters.
Analyses of Gene Ontology and Kyoto Encyclopedia of Genes and Genomes Pathway Enrichment
Gene Ontology (GO) biological process (BP), cellular component (CC), and molecular function (MF) enrichment analyses were performed. The Kyoto Encyclopedia of Genes and Genomes (KEGG) pathway analysis was performed for all DEGs. The criterion for statistical significance was an FDR adjusted P value of <0.05.
Gene signature and risk evaluation model
Prognostic genes were defined as those with a log-rank P value of <0.01 for survival comparison at the optimal expression cutoff. All DEGs performed the prognostic identification. A robust partial likelihood-based Cox proportional hazards regression survival model was used to select prognostic signature genes from all prognostic DEGs (18). A series of gene signatures were generated by forward selection. The Akaike information criterion (AIC) and negative log-likelihood methods were used to identify the optimal gene signature.
A risk stratification model was developed as follows:
- positive prognostic genes earned a score of 0 when up-regulated and 1 when down-regulated;
- negative prognostic genes earned a score of 1 when up-regulated and 0 when down-regulated.
This model was applied in the external validation cohorts by summing the scores of all signature genes. Then, the patients were stratified into risk groups according to their risk score level. The clinicopathological characteristics of patients in the risk score =0, risk score =1, risk score =2, and risk score =3 groups are list in Table 1.
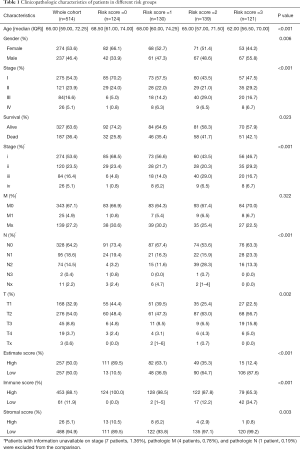
Full table
R version 3.5.2 (http://www.R-project.org) was used for the statistical analyses. The “estimate” package with default parameters was used to calculate the ESTIMATE, stromal, and immune scores.
The “rbsurv” package with 3-fold cross-validation and 1,000 iterations was used to construct the robust likelihood-based survival model. GO and KEGG enrichment analyses were performed using the “clusterProfiler” package. Heatmaps and Venn diagrams were constructed with the “pheatmap” and “VennDiagram” packages, respectively. The FDR method was used to adjust for multiple testing. Multivariate Cox regression analysis was performed to identify the independent risk factors for overall survival. And the protein-protein interaction (PPI) network was constructed. After the integration of clinicopathological risk factors and risk group (based on the Estimate, stromal, and immune scores), a nomogram was built for precise prediction of overall survival.
Results
Estimation of estimate-stromal-immune scores
The expression data and clinical information from 514 LUAD patients in the TCGA database were analyzed. The clinicopathological characteristics of patients in the risk score =0, risk score =1, risk score =2, and risk score =3 groups are listed in Table 1. Patients in the group with the highest risk (risk score =3) were more likely to be younger and male (P<0.001, and P=0.006 respectively). Furthermore, patients in the highest risk group had a larger tumor size and lymph node metastases (P=0.002, and P<0.001 respectively). The lower risk groups included more early-stage patients (P<0.001).
In the validaiton cohort, the estimate scores ranged from −2,963.63 to 4,485.76; the stromal scores ranged from −1,959.31 to 2,098.77; and the immune scores ranged from −1,355.85 to 3,286.67. Patients in higher risk groups yielded lower Estimate scores (P<0.001), lower immune scores (P<0.001), and lower stromal scores (P=0.003).
Association of estimate, stromal, and immune scores with the clinicopathological characteristics and prognoses of LUAD patients
The association between the scores (Estimate, stromal, or immune) and patients data is summarized in Figure 1A. The estimate, stromal, and immune scores of female patients were increased compared to those of males (P=0.002, P=0.0058, and P=0.0033, respectively). Significant correlations were found between the estimate, stromal, immune scores and pathologic T factor. Larger tumors (T3 and T4) yielded lower estimate and immune scores than those of smaller sizes (T1 and T2) (Kruskal Wallis test, P=0.04 for Estimate score, P=0.22 for stromal score, and P=0.012 for immune score). Significant correlations were also observed between the estimate and stromal scores and pathologic M factor. Patients with metastases (M1) yielded lower estimate and stromal scores than those without metastases or with unknown metastatic status (M0 and Mx) (Kruskal Wallis test, P=0.025 and P=0.016 respectively). Both the estimate and immune scores were variously distributed among TNM stage. Tumors with an advanced stage (stage III and stage IV) yielded lower estimate and immune scores than early-stage tumors (stage I and stage II) (Kruskal Wallis test, P=0.028 and P=0.027 for estimate score and immune score, respectively).
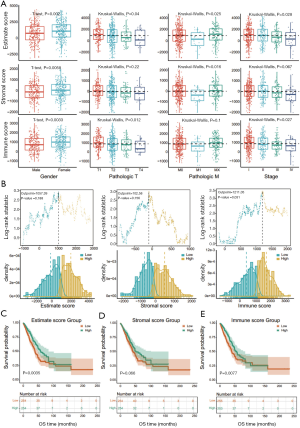
Illustrations of optimal cutoff identification for estimate, stromal, and immune score are displayed in Figure 1B. Patients with high estimate scores had better overall survival than those with low estimate scores (log-rank test, P=0.0035) (Figure 1C). Patients with high stromal scores showed a trend of better overall survival than lower group (log-rank test, P=0.066), and this trend seemed more obvious after 5 years of follow-up (Figure 1D). Patients with high immune scores yielded better overall survival than those with low immune scores (log-rank test, P=0.0077), and this survival advantage was more remarkable after 5 years of follow-up (Figure 1E).
Comparison of gene expression profiles by estimate, stromal, and immune scores in LUAD
The expression profiles of LUAD patients with high estimate (high stromal and high immune) scores were compared with those of patients with low estimate (low stromal and low immune) scores to identify DEGs related to estimate-stromal-immune score.
The DEGs related to Estimate, stromal, and immune score are visualized in volcano plots (Figure 2A). There were 86 shared up-regulated DEGs (Figure 2B) and 3 down-regulated DEGs (Figure 2C) in the estimate, stromal, and immune score groups. A heatmap was created to visualize the expression profiles of the selected 89 DEGs.
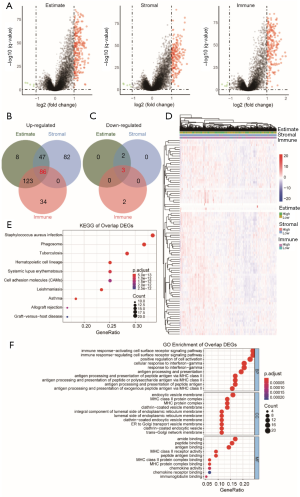
Identified DEGs had distinct expression profiles from the unsupervised hierarchical clustering analyses. Patients with high and low Estimate (stromal or immune) scores could be distinguished effectively according to these DEGs (Figure 2D).
KEGG pathways enriched by the DEGs were related to staphylococcus aureus infection, phagosome, tuberculosis, and cell adhesion molecules (Figure 2E). The stromal-related DEGs were enriched in protein digestion and absorption, focal adhesion, and extracellular matrix receptor interaction (Figure S1A). Meanwhile, the immune score-related DEGs were enriched in antigen processing and presentation, the chemokine signaling pathway, and Th1 and Th2 cell differentiation (Figure S1B). GO analysis showed that the DEGs were enriched in the immune response-activating and -regulating cell surface receptor signaling pathway, MHC class II protein complex antigen processing, and presentation of peptide antigen via MHC class II (Figure 2F). Separate GO enrichment analyses were carried out and showed that the stromal scores were enriched in extracellular matrix organization, connective tissue development, collagen trimer, and extracellular matrix structural constituent (Figure S1C). The immune scores were enriched in T-cell activation, positive regulation of innate immune response, T-cell receptor complex, and C-C chemokine receptor activity (Figure S1D).
Identification of prognostic DEGs in LUAD
These prognostic genes were defined from the above 89 estimate-stromal-immune score-related DEGs and comprised 36 up-regulated DEGs and 1 down-regulated DEG. A forest plot showing the prognostic effects of these 37 DEGs is displayed in Figure 3.
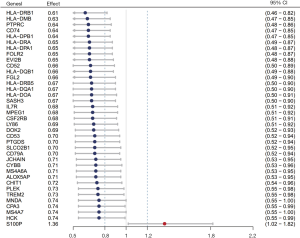
As recently illustrated in clinical trials boosting T-cell responses, 7 genes were summarized as follows: PDCD1, LAG3, HAVCR2, CTLA4, KIR2DL3, TNFRSF18, and TNFRSF9. These 7 genes and the 37 prognostic DEGs selected from TCGA were screened using the STRING (Search Tool for the Retrieval of Interacting Genes/Proteins) database, and the protein–protein interaction (PPI) network was constructed. Finally, IL-7R (interleukin 7 receptor), CD52, CD53, HCK (hemopoietic cell kinase), and CYBB (Cytochrome B-245 Beta Chain) were found to interact most closely with the immune checkpoint inhibitors (ICIs) based on the number of links (Figure S2).
Gene signature based on the estimate, stromal, and immune scores and risk stratification model for LUAD
Thirty-seven DEGs were selected to identify the gene signature with the most prognostic significance. Based on the AIC and the smallest values for the negative log-likelihood statistics, CD74, JCHAIN, and PTGDS were identified as the optimal signature genes (Table 2). These 3 genes were significantly associated with favorable survival outcomes (Figure 4A). For risk stratification, an individual-level score was developed based on the 3 signature genes (Figure 4B). A lower risk score was found to be significantly associated with a longer survival time, and patient living months increased as the risk score decreased (Figure 4C). Using this risk score stratification model, 4 groups were classified according to their survival outcomes (log-rank test, P=0.005) (Figure 4D). Further validation of the risk stratification model was carried out among patients with stage I, II, and III LUAD (Figure 4E,F,G).
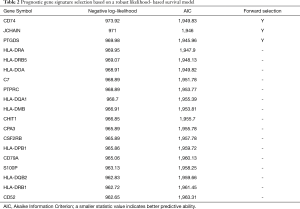
Full table
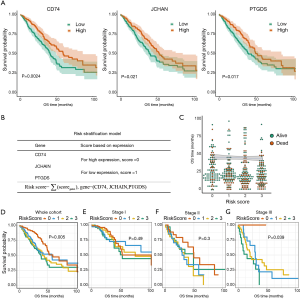
Relationship of estimate-stromal-immune score-based risk group with overall survival in patients with LUAD
The prognostic value of the estimate-stromal-immune score-based risk stratification model was evaluated through multivariate cox regression (Table 3). After adjusting the variables of age, sex, race, and pathologic stage, risk group was identified as a significant prognostic factor (P=0.009).
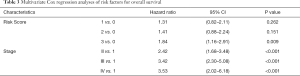
Full table
After the integration of clinicopathological risk factors and risk group (based on the estimate, stromal, and immune scores), a nomogram was built for precise prediction of OS (Figure 5A). A higher points total was associated with improved survival. However, the difference in points between pathologic stages III and IV was not obvious. This result was also observed in relation to sex, with the difference between females and males being less than 7 points. Compared against the ideal model, the calibration plot showed our nomogram to perform well (Figure 5B).
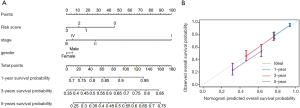
Validation of the risk stratification model in 2 external cohorts
GSE68571 cohort: among 96 patients, those with a high expression of JCHAIN and PTGDS had better OS (log-rank test, P=0.025, and P=0.038, respectively) (Figure 6A). A negative correlation was found between the risk scores calculated from these 3 signature genes and patient living months (Figure 6B). The patients in the validation cohort were classified into 4 groups using the 3-gene model, and the survival outcomes in the groups were found to be significantly different (log-rank test, P=0.027) (Figure 6C).
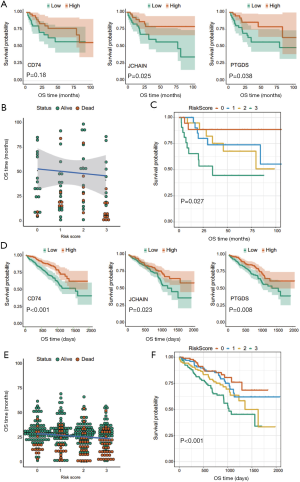
GSE72094 cohort: among 442 patients, high expression levels of CD74, JCHAIN, and PTGDS were significantly associated with favorable overall survival (log-rank test, P<0.001, P=0.023, and P=0.008, respectively) (Figure 6D). A negative correlation was also found between risk score and patient living months (Figure 6E). Significantly different survival outcomes were also observed in the 4 risk-score groups (log-rank test, P<0.001) (Figure 6F).
Discussion
We report a signature for prognostication of LUAD beyond TNM stage, notably to assist the use of adjuvant therapy after surgery for early stage LUAD. The signature based on the ESTIMATE-stromal-immune score could predict the prognosis of patients with LUAD. Eight to forty-seven percent of LUAD patients experience disease progression, indicating that occult metastases already exist at the time of “curative” surgery (7). Traditional TNM staging classification relies solely on the characteristics of tumor cells, regardless of the effect of the host immune response. In fact, the onsite and progression of tumors are affected both by the tumor cells and the TIME. Tumor progression and patient outcomes have been found to be related to the TIME. Tumor-infiltrating immune cells and stromal cells are 2 major components of the TIME. These cells have been shown to play important roles in recent studies. It is essential to consider immune parameters as prognostic factors and to improve the accuracy of cancer classification using the immunescore.
The immunescore is derived from the immune contexture, and is defined by immune cell infiltration based on single-sample gene set enrichment analysis. It was initially established to evaluate the prognosis of patients with stage I, II, and III colon cancer (19). For early-stage patients, the relapse and 5-year survival rates for the high-immunescore group were 4.8% and 86.2%, respectively. However, the relapse and 5-year survival rates for the low-immunescore group were 72% and 27.5%, respectively (20). Therefore, patients in the low-immunescore group could potentially have benefited from adjuvant therapy. Recent studies have proved that the immunescore can also play a role in prognostic prediction in other human malignancies such as hepatocellular carcinoma, pancreatic cancer, melanoma, lung cancer, and even brain metastasis (21,22). Two advantages of the immunescore are as follows: firstly, it appears to be the strongest prognostic factor for survival outcome; and secondly, it provides a tool or target for novel therapeutic approaches (23,24).
In 2006, the relationship between the immunescore and clinicopathological features of patients with non-small cell lung cancer (NSCLC) was studied (25). To improve the prognostic, and most possible a predictive tool for NSCLC, Roy M. recommended that the immunescore should be added to the TNM classification (TNM-I) (26). A study showed that the former displayed T-cells, Interferon, and M1 macrophage signatures and was associated with a better prognosis, while the latter presented with M2 macrophage signatures and immunosuppressive factors such as WNT/transforming growth factor-β (27).
In LUAD, T cells may cooperate to suppress the tumor. Many subtypes of T cells have been discovered due to the development of immunohistochemical technology. CD3+ is expressed in all T lymphocytes and is thus considered to be a biomarker. CD4+ T helper lymphocytes (Th), CD8+ cytotoxic T lymphocytes (CTLs), CD45RO+ memory T cells, and FoxP3+ (forkhead box P3 lymphocytes, regulatory T cells) regulatory cells (Tregs) are other subtypes of T-lymphocyte cell surface markers (28). High CD3+, CD8+, and CD4+ T-cell infiltration is an independent favorable prognostic factor (29). CD45RO+ has been proposed as a risk factor for disease-free survival (HR =1.79, P=0.001), disease-specific survival (HR =1.80, P=0.001), and OS (HR =1.61, P=0.001) (30). FoxP3 contributes to more aggressive behavior of LUAD (such as solid-predominant subtype), and is associated with poor OS (31). Our result revealed that the stromal component in pulmonary tissue might act as a barrier in tumorigenesis by constraining tumor cell proliferation. Detailed molecular analysis of lung stroma might be important for long-term health management of LUAD patients. Patients with high immune scores yielded better overall survival than those with low immune scores, and this survival advantage was more remarkable after 5 years of follow-up. This finding suggested that, from the beginning of the tumor formation, there was a stronger adaptive immune response in LUAD patients with higher immune scores than in patients with lower immune scores.
Apart from the subtypes of TILs, spatial organization of tumor cells, stromal cells, and lymphocytes also plays an important role in tumor progression and metastasis. The immunescore comprises 2 parts, namely the tumor core and its invasive margin. Compared with those in the tumor nest, the numbers of infiltrating CD8+ or CD4+ T cells in the tumor stroma are much higher. Furthermore, the CD8+ T cell stromal score also seems to be superior to the tumor score. More specifically, CD8+ T-cell stromal scoring at the invasive margin is a strong prognostic factor (32).
It is unclear how tumor-infiltrating B cells (TIBs) and antibodies interact with each other in the microenvironment. For Kirsten RAt Sarcoma (KRAS) mutation-type LUAD, a low proportion of IgA isotype and a high proportion of IgG1 among all intratumorally produced immunoglobulins were specifically associated with improved overall survival. In STK11 mutation-type LUAD, a high level of IgG4-producing TIBs was associated with better overall survival. The specificity of protective B-cell populations might provide basic information for the development of efficient targeted immunotherapies (33).
MHC-II is an essential component of the adaptive immune system and is critical for antigen presentation to CD4+ T lymphocytes. Accumulating evidence has demonstrated that tumor-specific MHC-II is associated with favorable outcomes (34). Furthermore, positive MHC-II expression in tumor cells was found to be associated with improved disease-free survival in triple-negative breast cancer patients with lymph node metastasis (35). Park IA also found that aberrant expression of MHC II in triple-negative breast cancer might trigger an antitumor immune response that reduces the rate of relapse and enhances progression-free survival (36). In anti-PD-1-treated melanoma patients, MHC-II positivity of tumor cells was associated with the therapeutic response (37). For Hodgkin lymphoma, Roemer MGM. reported that genetically driven PD-L1 expression and MHC-II positivity were potential predictors of favorable outcome after PD-1 blockade (38).
In this study, a TIME-related prognostic model was constructed to predict the outcomes of LUAD patients based on differentially expressed stromal and immune signature genes. Yue found that a 3-gene signature (ADAM12, BTK, and ERG) could be an independent prognostic factor (39). A total of 37 DEGs related to the estimate, stromal, and immune scores were identified in our study. A gene signature containing CD74, JCHAIN, and PTGDS was utilized to develop a risk stratification model.
CD74, also known as the invariant chain (Ii), is the molecular chaperone of MHC-II. It is a high-affinity receptor of the macrophage migration inhibitory factor (MIF), a proinflammatory cytokine. Lee found that CD74-ROS1 fusion represented a genomic signature in early oncogenesis (40). Secretory polymeric immunoglobulins (IgA dimers and IgM pentamers) are unique because, as well as light and heavy chains, they contain joining chains (J-chains) responsible for their oligomerization. These antibodies constitute parts of the local adaptive immune system, acting on the mucosa membranes of the respiratory and digestive systems as the first barrier of protection against potential infectious agents (41). JCHAIN is the coding gene of immunoglobulin J-chains. It has been reported to be related to the clinical outcomes of acute lymphoblastic leukemia patients (42). Along with CHAC2, CLEC9A, and 8 other genes, JCHAIN was also found to be associated with survival in head and neck squamous cell carcinoma (43). Moreover, You reported that JCHAIN might exert a critical influence on resistance and tumorigenesis in Marek’s disease (44).
Prostaglandin is well known to be essential for tumor angiogenesis and growth. Mast cell-derived prostaglandin D2 governs the tumor microenvironment by limiting excessive responses to TNF-α production and vascular permeability (45). When added exogenously, PTGDS reportedly suppressed the hyperproliferation and migration of A549 cells (46). Furthermore, Shyu found that overexpression of PTGDS could inhibit the invasion of H-rev107-mediated testis tumor cells through the PGD2-cAMP-SOX9 signaling pathway (47).
Immunotherapy has emerged as a key pillar of cancer treatment. Seven 7 genes (PDCD1, LAG3, HAVCR2, CTLA4, KIR2DL3, TNFRSF18, and TNFRSF9) and the 37 prognostic DEGs selected from TCGA were screened using the STRING (Search Tool for the Retrieval of Interacting Genes/Proteins) database, and the protein–protein interaction (PPI) network was constructed. In the current study, PTPRC, FGL2, CD74, HLA-DRB1, CD52, CSF2RB, LY86, PLEK, HLA-DPA1, and HLA-DQB1 were the top 10 immunotherapy target genes in the PPI network. PTPRC, also known as CD45, encodes some members from the protein tyrosine phosphatase (PTP) family. Proteins from this family are commonly activated in tumors. PTPRC has been shown to be an essential regulator of T- and B-cell antigen receptor signaling (48). Fibrinogen-like protein 2 (FGL2) is essential for both innate and adaptive immunity. FGL2 might promote tumor progression by activating cancer-associated fibroblasts in the tumor microenvironment (49). HLA-DRB1 is strongly associated with the risk of lung cancer and seemed to affect antigen presentation. Qin provided evidence for the substantial contributions of HLA II molecules to lung cancer susceptibility (50). CD52 is present on the surface of mature lymphocytes. It inhibits T-cell activation by impairing phosphorylation of the T-cell receptor-associated kinases Lck and Zap70 (51). Taken together, most prognostic DEGs from this study play essential roles in the tumor immune response and interact with immune checkpoint genes that have been verified in recent clinical trials. These newly discovered genes may be the potential targets for future immunotherapy. A nomogram was built after integration of clinicopathological risk factors and estimate, stromal, and immune scores. A higher points total was associated with improved survival. This objective evaluation method was consistent with the risk score model established in the current study.
In conclusion, tumor progression and survival outcomes in LUAD depend on the microenvironmental characteristics in the tumor tissue. Gene signatures based on estimate, stromal, and immune scores can provide more prognostic information for the TNM staging system. This risk score model for stratifying prognosis is essential for individualized treatment and follow-up.
Acknowledgments
The authors appreciate the academic support from AME Lung Cancer Collaborative Group and Jianyan Wen.
Funding: This study is supported by the funding from the research and demonstration application of clinical diagnosis and treatment technology in Beijing capital city (Z191100006619008).
Footnote
Reporting Checklist: The authors have completed the TRIPOD reporting checklist. Available at http://dx.doi.org/10.21037/tlcr-21-223
Conflicts of Interest: All authors have completed the ICMJE uniform disclosure form (available at http://dx.doi.org/10.21037/tlcr-21-223). The authors have no conflicts of interest to declare.
Ethical Statement: The authors are accountable for all aspects of the work in ensuring that questions related to the accuracy or integrity of any part of the work are appropriately investigated and resolved. The study was conducted in accordance with the Declaration of Helsinki (as revised in 2013).
Open Access Statement: This is an Open Access article distributed in accordance with the Creative Commons Attribution-NonCommercial-NoDerivs 4.0 International License (CC BY-NC-ND 4.0), which permits the non-commercial replication and distribution of the article with the strict proviso that no changes or edits are made and the original work is properly cited (including links to both the formal publication through the relevant DOI and the license). See: https://creativecommons.org/licenses/by-nc-nd/4.0/.
References
- Bray F, Ferlay J, Soerjomataram I, et al. Global cancer statistics 2018: GLOBOCAN estimates of incidence and mortality worldwide for 36 cancers in 185 countries. CA Cancer J Clin 2018;68:394-424. [Crossref] [PubMed]
- Travis WD. Pathology of Lung cancer. Clin Chest Med 2011;32:669-92. [Crossref] [PubMed]
- Li Z, Yamada S, Wu Y, et al. Polypeptide N-acetylgalactosaminytransferse-6 expression independently predicts poor overall survival in patients with lung adenocarcinoma after curative resection. Oncotarget 2016;54463-73. [Crossref] [PubMed]
- Greenhalgh J, Dwan K, Boland A, et al. First-line treatment of advancedepidermal growth factor receptor (EGFR) mutation positive non-squamousnon-small cell lung cancer. Cochrane Database Syst Rev 2013;5:CD010383
- Sobin L, Wittekind C. TNM Classification of Malignant Tumors. Wiley-Liss: New York, 2002.
- Nagtegaal ID, Quirke P, Schmoll HJ. Has the new TNM classification for colorectal cancer improved care? Nat Rev Clin Oncol 2011;9:119-23. [Crossref] [PubMed]
- Goldstraw P, Chansky K, Crowley J, et al. The IASLC Lung Cancer Staging Project: Proposals for Revision ofthe TNM Stage Groupings inthe Forthcoming (Eighth) Edition of the TNM Classification for Lung Cancer. J Thorac Oncol 2016;11:39-51. [Crossref] [PubMed]
- Bindea G, Mlecnik B, Fridman WH, et al. Natural immunity to cancer in humans. Curr Opin Immunol 2010;22:215-22. [Crossref] [PubMed]
- Hanahan D, Weinberg RA. Hallmarks of cancer: the next generation. Cell 2011;144:646-74. [Crossref] [PubMed]
- Fridman WH, Pages F, Sautes-Fridman C, et al. The immune contexture in human tumours: impact on clinical outcome. Nat Rev Cancer 2012;12:298-306. [Crossref] [PubMed]
- Wang L, Sebra RP, Sfakianos JP, et al. A reference profile-free deconvolution method to infer cancer cell-intrinsic subtypes and tumor-type-specific stromal profiles. Genome Med 2020;12:24. [Crossref] [PubMed]
- Watabe S, Kikuchi Y, Morita S, et al. Clinicopathological significance of microRNA-21 in extracellular vesicles of pleural lavage fluid of lung adenocarcinoma and its functions inducing the mesothelial to mesenchymal transition. Cancer Med 2020;9:2879-90. [Crossref] [PubMed]
- Yoshihara K, Shahmoradgoli M, Martinez E, et al. Inferring tumour purity and stromal and immune cell admixture from expression data. Nat Commun 2013;4:2612. [Crossref] [PubMed]
- Wang H, Wu XS, Chen Yiming, et al. Stromal-Immune Score-Based Gene Signature: A Prognosis Stratification Tool in Gastric Cancer. Front Oncol 2019;9:1212. [Crossref] [PubMed]
- Verhaak RG, Hoadley KA, Purdom E, et al. Integrated genomic analysis identifies clinically relevant subtypes of glioblastoma characterized by abnormalities in PDGFRA, IDH1, EGFR, and NF1. Cancer Cell 2010;17:98-110. [Crossref] [PubMed]
- Hothorn T, Zeileis A. Generalized maximally selected statistics. Biometrics 2008;64:1263-9. [Crossref] [PubMed]
- Ritchie ME, Phipson B, Wu D, et al. limma powers differential expression analyses for RNA-sequencing and microarray studies. Nucleic Acids Res 2015;43:e47 [Crossref] [PubMed]
- Cho H, Yu A, Kim S, et al. Robust likelihood-based survival modeling with microarray data. J Stat Softw 2009;29:16. [Crossref]
- Galon J, Pagès F, Marincola F M, et al. Cancer classification using the Immunoscore: a worldwide task force. J Transl Med 2012;10:205. [Crossref] [PubMed]
- Pagès F, Kirilovsky A, Mlecnik B, et al. In situ cytotoxic and memory T cells predict outcome in patients with early-stage colorectal cancer. J Clin Oncol 2009;27:5944-51. [Crossref] [PubMed]
- Ros-Martínez S, Navas-Carrillo D, Alonso-Romero JL, et al. Immunoscore: a novel prognostic tool. Association with clinical outcome, response to treatment and survival in several malignancies. Crit Rev Clin Lab Sci 2020;57:432-43. [Crossref] [PubMed]
- Mazzaschi G, Milanese G, Madeddu PPD, et al. Integrated CT imaging and tissue immune features disclose a radio-immune signature with high prognostic impact on surgically resected NSCLC. Lung Cancer 2020;144:30-9. [Crossref] [PubMed]
- Hodi FS, O’Day SJ, McDermott DF, et al. Improved survival with ipilimumab in patients with metastatic melanoma. N Engl J Med 2010;363:711-23. [Crossref] [PubMed]
- Topalian SL, Hodi FS, Brahmer JR, et al. Safety, activity, and immunecorrelates of anti-PD-1 antibody in cancer. N Engl J Med 2012;366:2443-54. [Crossref] [PubMed]
- Hiraoka K, Miyamoto M, Cho Y, et al. Concurrent infiltration by CD8+ T cells and CD4+ T cells is a favourable prognostic factor in non-small-cell lung carcinoma. Br J Cancer 2006;94:275-80. [Crossref] [PubMed]
- Bremnes RM, Busund LT, Kilvær TL, et al. The Role of Tumor-Infiltrating Lymphocytes in Development, Progression, and Prognosis of Non-Small Cell Lung Cancer. J Thorac Oncol 2016;11:789-800. [Crossref] [PubMed]
- Tan Q, Huang Y, Deng K, et al. Identification immunophenotyping of lung adenocarcinomas based on the tumor microenvironment. J Cell Biochem 2020;121:4569-79. [Crossref] [PubMed]
- Geng Y, Shao Y, He W, et al. Prognostic role of tumorinfiltrating lymphocytes in lung cancer: a meta-analysis. Cell Physiol Biochem 2015;37:1560-71. [Crossref] [PubMed]
- Kim R, Keam B, Kim S, et al. Differences in tumor microenvironments between primary lung tumors and brain metastases in lung cancer patients: therapeutic implications for immune checkpoint inhibitors. BMC Cancer 2019;19:19. [Crossref] [PubMed]
- Paulsen EE, Kilvaer T, Khanehkenari MR, et al. CD45RO(+) Memory T lymphocytes-a candidate marker for TNM-Immunoscore in squamous non-small cell lung cancer. Neoplasia 2015;17:839-48. [Crossref] [PubMed]
- Saruwatari K, Ikemura S, Sekihara K, et al. Aggressive tumor microenvironment of solid predominant lung adenocarcinoma subtype harboring with epidermal growth factor receptor mutations. Lung Cancer 2016;91:7-14. [Crossref] [PubMed]
- Donnem T, Hald SM, Paulsen EE, et al. Stromal CD8+ T-cell density-A promising supplement to TNM staging in non-small cell lung cancer. Clin Cancer Res 2015;21:2635-43. [Crossref] [PubMed]
- Isaeva OI, Sharonov GV, Serebrovskaya EO, et al. Intratumoral immunoglobulin isotypes predict survival in lung adenocarcinoma subtypes. J Immunother Cancer 2019;7:279. [Crossref] [PubMed]
- Axelrod ML, Cook RS, Johnson DB, et al. Biological Consequences of MHC-II Expression by Tumor Cells in Cancer. Clin Cancer Res 2019;25:2392-402. [Crossref] [PubMed]
- Forero A, Li Y, Chen D, et al. Expression of the MHC Class II Pathway in Triple-Negative Breast Cancer Tumor Cells Is Associated with a Good Prognosis and Infiltrating Lymphocytes. Cancer Immunol Res 2016;4:390-9. [Crossref] [PubMed]
- Park IA, Hwang SH, Song IH, et al. Expression of the MHC class II in triple-negative breast cancer is associated with tumor-infiltrating lymphocytes and interferon signaling. PLoS One 2017;12:e0182786 [Crossref] [PubMed]
- Johnson DB, Estrada M V, Salgado R, et al. Melanoma-specific MHC-II expression represents a tumour-autonomous phenotype and predicts response to anti-PD-1/PD-L1 therapy. Nat Commun 2016;7:10582. [Crossref] [PubMed]
- Roemer MGM, Redd RA, Cader FZ, et al. Major Histocompatibility Complex Class II and Programmed Death Ligand 1 Expression Predict Outcome After Programmed Death 1 Blockade in Classic Hodgkin Lymphoma. J Clin Oncol 2018;36:942-50. [Crossref] [PubMed]
- Yue C, Ma H, Zhou Y. Identification of prognostic gene signature associated with microenvironment of lung adenocarcinoma. Peer J 2019;7:e8128 [Crossref] [PubMed]
- Lee JJ, Park S, Park H, et al. Tracing Oncogene Rearrangements in the Mutational History of Lung Adenocarcinoma. Cell 2019;177:1842-57. [Crossref] [PubMed]
- Slizhikova DK, Zinov'eva MV, Kuz'min DV, et al. Decrease in Expression of Human J-chain in Lung Squamous Cell Cancer and Adenocarcinoma. Mol Biol (Mosk) 2007;41:659-65. [PubMed]
- Tomar AK, Agarwal R, Kundu B. Most Variable Genes and Transcription Factors in Acute Lymphoblastic Leukemia Patients. Interdiscip Sci 2019;11:668-78. [Crossref] [PubMed]
- Liu G, Zeng X, Wu B, et al. RNA-Seq analysis of peripheral blood mononuclear cells reveals unique transcriptional signatures associated with radiotherapy response of nasopharyngeal carcinoma and prognosis of head and neck cancer. Cancer Biol Ther 2020;21:139-46. [Crossref] [PubMed]
- You Z, Zhang Q, Liu C, et al. Integrated analysis of lncRNA and mRNA repertoires in Marek’s disease infected spleens identifies genes relevant to resistance. BMC Genomics 2019;20:245. [Crossref] [PubMed]
- Murata T, Aritake K, Matsumoto S, et al. Prestagladin D-2 is a mast cell-derived antiangiogenic factor in lung carcinoma. Proc Natl Acad Sci U S A 2011;108:19802-7. [Crossref] [PubMed]
- Ragolia L, Palala T, Hall CE, et al. Diminished lipoealintype prostaglandin D-2 synthase expression in human lung tumor. Lung Cancer 2010;70:103-9. [Crossref] [PubMed]
- Shyu RY, Wu CC, Wang CH, et al. H-revl07 regulates ptostaglandin D2 synthase-mediated suppression of cellular invasion in testicular cancer cells. J Biomed Sci 2013;20:30. [Crossref] [PubMed]
- Hendriks WJ, Pulido R. Protein tyrosine phosphatase variants in human hereditary disorders and disease susceptibilities. Biochim Biophys Acta 2013;1832:1673-96. [Crossref] [PubMed]
- Yuan K, Feng Y, Wang H, et al. FGL2 is positively correlated with enhanced antitumor responses mediated by T cells in lung adenocarcinoma. PeerJ 2020;8:e8654 [Crossref] [PubMed]
- Qin N, Wang C, Zhu M, et al. Fine-mapping the MHC region in Asian populations identified novel variants modifying susceptibility to lung cancer. Lung Cancer 2017;112:169-75. [Crossref] [PubMed]
- Toh BH, Kyaw T, Tipping P, et al. Immune regulation by CD52-expressing CD4 T cells. Cell Mol Immunol 2013;10:379-82. [Crossref] [PubMed]