Characteristics of hypoxic tumor microenvironment in non-small cell lung cancer, involving molecular patterns and prognostic signature
Introduction
Lung cancer is one of the most malignant tumors that threatens the health, and its incidence has increased mainly owing to the rise in smokers, a known risk factor (1,2). Hypoxia related genes (HRGs) play an important role in maintaining energy metabolism, angiogenesis and metastasis of tumor cells, whose essence is mainly due to the vigorous metabolic capacity of tumor cells, resulting in an increase in oxygen content requirements beyond the normal range. Hypoxic tumor microenvironment (TME) contributes to the promotion of tumor cell invasion, thus contributing to the study of cancer mechanisms, progression, and recurrence (3). Some researches have suggested that certain HRGs and their mediators, hypoxic-inducible factors, may serve as prognostic and therapeutic targets for certain cancers, such as colorectal cancer, breast cancer, and hepatocellular carcinoma (4). Genomic analysis has been the main method used internationally to identify new biological targets for lung cancer. Although this method does not reveal a new mechanism, some studies have revealed the significance of tumor-related structures and the signaling pathways of HRGs in the tumor microenvironment. According to the report, there are differences in cell composition within TME, which are specifically reflected in cytotoxic T cells, dendritic cells, helper T cells, mesenchymal stem cells, tumor-related macrophages and related inflammatory pathways (5,6). Therefore, comprehensive identification of the characteristics of TME cell infiltration mediated by multiple HRGs will be good for our realization of the immune regulation of TME. In this research, we integrated genomic information from 1,121 non-small cell lung cancer (NSCLC) samples to comprehensively assess the modification patterns of HRGs and associate these modifications with TME immune infiltration. Three different modification patterns of HRGs were revealed to indicate that HRGs modification patterns play an indispensable role in shaping the individual tumor microenvironment. To this end, we specifically constructed a scoring system to build a scoring system to quantify the modification patterns of HRGs. We presented the following article in according with the MDAR checklist (available at http://dx.doi.org/10.21037/tlcr-20-1314).
Methods
Data sources and data processing for NSCLC
Complete clinical note and gene expression data were downloaded from the Gene Expression Omnibus (GEO) and The Cancer Genome Atlas (TCGA) database. Patients with missing clinical information were deleted. There were six data cohorts involved in this paper, including GSE30219, GSE42127, GSE41271, GSE50081, GSE37745, and TCGA cohort. For the GEO datasets, the normalized matrix files were downloaded, while the RNA sequencing data (FPKM value) came from the TCGA dataset. The “ComBat” algorithm in the sva package was used to correct for batch effects.
Unsupervised clustering for seventy-six HRGs
Seventy-six HRGs were originated from GSEA database (https://www.gsea-msigdb.org/gsea/index.jsp). Unsupervised cluster analysis was applicable for identifying the modification patterns of different HRGs according to the expression of HRGs, and the patients were classified for next research. Consensus algorithm was applied to determine the number of cluster and stability. The steps described above were conducted by the ConsensuclusterPlus package, and they were repeated 1,000 times to ensure the stability of the cluster.
Gene Set Variation Analysis (GSVA)
To further explore the differences of HRGs in biological processes, GSVA enrichment analysis was carried out using GSVA package (7). In a nonparametric and unsupervised approach, GSVA was commonly used to estimate changes in path and bioprocess activity in a sample of an expression dataset. The gene set of “c2.cp.kegg.v6.2.-symbols” originated from MSigDB database, aiming at performing GSVA analysis. P value <0.05 after correction [false discovery rate (FDR)] is generally considered to be statistically significant, which was also the basis for gene selection for GSVA analysis.
Evaluation of immune cell infiltration
The ssGSEA (single-sample gene set enrichment analysis) was used to quantify the relative abundance of 28 immune cell infiltrates. The set of genes used to label each TME infiltrating immune cell type that was derived from the Charoentong research, which contained a variety of human immune cell subtypes, consisting of macrophages, activated dendritic cells, activated CD8 T cells, regulatory T cells, naturally killing T cells, etc. The enrichment scores calculated by ssGSEA analysis was used to reflect the relative abundance of each TME infiltrated cell in each sample (8).
Analysis of the differentially expressed genes (DEG) among HRGs clusters
To verify the reliability and stability of the clustering, we screened out the DEG among the clusters, and the limma package was used to determine DEG. An empirical Bayesian approach was used to estimate the change of gene expression, and the involved method was the moderated t-test. The criterion for screening differential genes was P<0.001.
Construction and validation of HRGs signature
In an integrated GEO training cohort, a univariate Cox regression analysis was performed in the first step to assess the relationship between HRGs expression levels and patient survival. The least absolute shrinkage and selection operator (LASSO) method was used to screen out prognostic genes with P value <0.05. Subsequently, HRGs-based risk scores were constructed to predict the prognosis of NSCLC. Riskscore = Exp (Gene1) × β1 + Exp (Gene2) × β2 + … + Exp (Genen) × βn. Exp represented the expression level of the gene, and β represented the regression coefficient of each gene calculated by the LASSO Cox regression. Then the patients were then divided into low and high risk groups according to the optimal cut-off value of risk score (9). The Kaplan-Meier survival curve was used to analyze the survival differences between low and high risk groups. The time-dependent receiver operating characteristic (ROC) curve analysis was used to evaluate the predictive accuracy of the HRGs-based prognostic signature. What’s more, multivariate regression analysis was applied to determine whether the signature was an independent prognostic factor of NSCLC.
Correlation between HRGs signature and other related biological processes
A set of gene sets were constructed by Mariathasan which stored genes related to some biological process, such as (I) antigen processing machinery; (II) CD8 T-effector signature; (III) immune-checkpoint; (IV) pan-fibroblast TGFb response signature (Pan-F-TBRS); (V) angiogenesis signature; (VI) epithelial-mesenchymal transition (EMT) markers consisting of EMT1, EMT2 and EMT3; (VII) Nucleotide excision repair; (VIII) mismatch repair; (IX) DNA damage repair; (X) WNT target; (XI) antigen processing and presentation; (XII) DNA replication (10).
Statistical analysis
One-way ANOVA and Kruskal-Wallis test, non-parametric and parametric methods, which devoted to the comparison of two or more groups. Spearman and distance correlation analysis were used to calculate the correlation coefficient. The optimal cutoff value for each dataset was evaluated based on the overall patient survival and risk score value. To identify genes in the analysis of differential genes, the Benjamini-Hochberg method converted the P value to FDR. Kaplan-Meier method generated survival curves for the subgroups in each data set, and log rank (Mantel-Cox) test was applied to determine the statistical significance of the differences. All heat maps are generated by the pheatmap function. P values were two-side, and P value of less than 0.05 were regarded statistically significant. The study was in accordance with the Helsinki Declaration (as revised in 2013).
Results
Cluster analysis of five GEO data sets based on HRGs
To further explore the new molecular classification of HRGs expression patterns, 1,121 NSCLC patients which came from a combination of five data sets from the GEO database were subjected to unsupervised consensus clustering (GSE30219, GSE37745, GSE41271, GSE42127 and GSE50081). According to the relative change of the area under the cumulative distribution function (CDF) curve and the uniform heat map, the optimal number of cluster was determined to be three (k value =3), which suggested three molecular patterns, and there was no significant change in the area under the CDF curve (Figure 1A, Figure S1A,S1B). There were 353 cases in the first cluster (31.5%), 409 cases in the second cluster (36.5%), and 358 cases in the third cluster (32%). Kaplan-Meier survival analysis showed that patients in the third cluster had the best overall survival rate (Figure 1B), while patients in the first cluster had the worst overall survival rate. Principal component analysis displayed that there were significant differences among the three different clusters (Figure 1C). What’s more, the heat map showed that the expression of differential genes between different clusters was significantly different, which proved the reliability and stability of the three clusters (Figure 1D) (11).
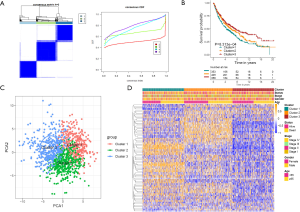
Analysis of immune infiltration among different clusters and GSVA enrichment
To explore the biological behavior among these different HRGs molecular patterns, immune infiltration and GSVA enrichment analysis were performed. The box plot displayed that the three molecular patterns were significantly different in twenty-eight kinds of immune cells (Figure 2A), while the heat map similarly showed the expression differences among molecular patterns (Figure 2B) (12). Compare cluster one and cluster two, cluster one was obviously enriched in carcinogenic activation pathways such as P53 pathway, PI3K AKT MTOR signaling and WNT beta catenin signaling, while cluster two showed multiple enrichment in the immune pathway such as IL2 STAT5 signaling, IL6 JAK STAT3 signaling and inflammatory response. Compare cluster one and cluster three, cluster one was significantly enriched in hypoxia and carcinogenic activation pathways. For instance, hypoxia, MTORC1 signaling and epithelial mesenchymal transition (Figure 2C) (13,14). Kruskal-Wallis test revealed that there were significant differences in HRG score between HRG clusters. In the stromal score, there were significant differences among the clusters. Meanwhile, cluster two showed higher stromal score than the other two clusters, indicating that cluster two was correlated with the matrix activation-related characteristics, which also helped explain that although cluster two was more active in immune infiltration, it did not survive as well as cluster three. Among the immune score, there was no significant difference between cluster one and cluster three. Although cluster two showed the highest immune score, the performance of immune-related characteristics is not obvious due to the characteristics of matrix activation. Furthermore, in the analysis of tumor purity, the differences between clusters were obvious, and cluster three showed higher tumor purity, which further verified that cluster three had the best survival (Figure 2D) (15).
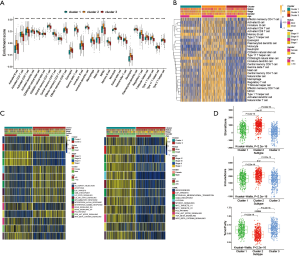
Analysis of cluster differences and GO enrichment
By evaluating the stromal microenvironment among the clusters, matrix expression was highest in cluster one and worst in cluster three, which also indicated that cluster one had the worst survival (Figure 3A). Although cluster two played a certain role in the immune pathway, it reduced the influence of the immune pathway due to the activation of the matrix pathway, so the survival status was worse than cluster three. Moreover, there were significant differences among costimulus molecules among different clusters (Figure 3B). By comparing the three clusters in pairs, we used the genes with a P value of less than 0.001 as the difference genes between the two, and screened out 1,694 common difference genes through the Venn diagram (Figure 3C). Through enrichment analysis of differential genes, it was found that these differential genes were mainly enriched in hypoxia pathway, extracellular matrix pathway and immune pathway, such as response to oxygen levels, response to hypoxia, regulation of adaptive immune response, activation of innate immune response, negative regulation of immune system process, extracellular matrix, extracellular matrix organization and so on (Figure 3D).
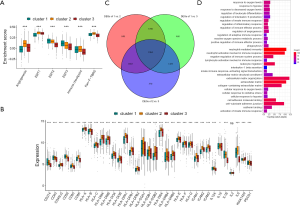
Construction of HRG prognostic signature
To further determine the value of HRGs in predicting the prognosis of patients with NSCLC, we constructed a prognosis signature of HRGs by a two-step approach. The first step was univariate Cox regression, and the second step was LASSO regression analysis (16). A total of 18 HRGs were selected to construct the signature. The HRG-based prognostic risk score model was established with the following formula: ExpADM × 0.053 + ExpBIK × 0.009 + ExpDDIT3 × (−0.003) + ExpENO1 × 0.17 + ExpEPAS1 × (−0.09) + ExpFGF3 × 0.051 + ExpGAPDH × 0.018 + ExpMIF × 0.044 + ExpNFKB1 × (−0.192) + ExpPFKP × 0.113 + ExpPGK1 × (−0.095) + ExpPLAUR × 0.0007 + ExpSPP1 × 0.078 + ExpSTC1 × 0.115 + ExpTEK × (−0.045) + ExpTFRC × (−0.02) + ExpTGFA × 0.035 + ExpXRCC6 × 0.0009 (Table 1). In the TCGA data set, there are significant differences in the expression of 18 genes in normal tissues and tumor tissues (Figure S1C). We have shown the correlation between genes and clinical factors, and we only list meaningful genes. Patients were divided into high and low risk groups by the optimal cutoff value (17). The survival analysis of signature genes showed that the HRGs of model construction had prognostic significance (Figure 4A). The area under the red curve in ROC curve was 0.69 (Figure 4B). Multivariate Cox regression signature analysis included patient age, sex, stage, and risk score, confirming that risk score was an independent prognostic factor for NSCLC patients (Figure 4C). To further validate the stability of the signature, we applied the HRGs signature constructed on the basis of the merged GEO dataset to the separate GEO database in order to verify its prognostic value (GSE30219, GSE37745, GSE41271, GSE42127, GSE50081) (Figure 4D,E,F,G,H). Prognostic analysis of HRGs showed that the genes in the signature could significantly predict the significance of patient survival (Figure 5A). In addition, we found significant differences in risk scores among the clusters through the box plot. Cluster one indicated the highest median score, while cluster three indicated the lowest median score (Figure 5B). The Sankey diagram fully demonstrated the association between prognostic signature, clusters, and clinical characteristics (Figure 5C). The network diagram of HRGs in signature was intended to visually show the combined appearance of the correlation between signature genes and their impact on the overall survival of NSCLC patients (Figure 5D). To better demonstrate the features of the HRGs signature, we also explored the link between the signature and the immune microenvironment (Figure 6A). In the three HRGs clusters, significant differences among signature genes were observed, which further verified the significance of clustering (Figure 6B). The signature presented significant differences in the matrix microenvironment (Figure 6C). The vast majority of costimulatory molecules showed significant differences between high and low risk groups (Figure 6D). Most immune cells are significantly different between the high and low risk groups (Figure 6E). Furthermore, the differences of somatic mutation distribution of lung adenocarcinoma (LUAD) and lung squamous carcinoma (LUSC) in TCGA were analyzed to further studying the characteristics of signature genes. The gene NFKB1 in the model had the highest mutation rate in LUSC, reaching 2%, while EPAS1 had the highest mutation rate in LUAD, reaching 2% (Figure 6F,G, Figure S1D).
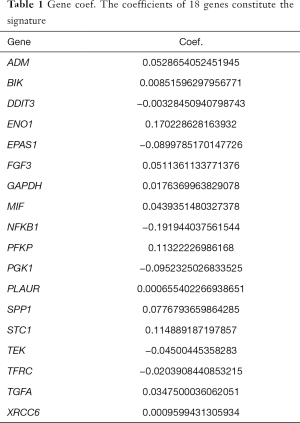
Full table
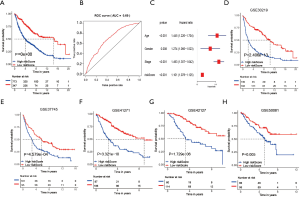
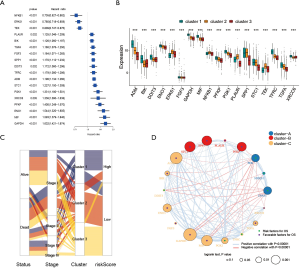
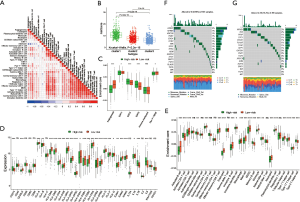
Verification of the prognostic signature in the TCGA cohort
To further explore the reliability and stability of the signature construction, we applied the risk score to the TCGA cohort, so that the feasibility of the hypoxia signature was confirmed (Figure 7A). It was noting that multivariate regression analysis suggested that the prognostic signature was indeed an independent prognostic factor for NSCLS (Figure 7B). The risk heat map showed that as the risk score increased, survival was worse and the expression of signature factors were different in the high and low risk groups (Figure 7C). We build a nomogram that could predict 1-, 3- and 5-year OS by signature and other clinical factors. The 1-, 3- and 5-year OS probability calibration curve showed that the OS predicted by nomogram was in good agreement with the actual OS of NSCLC patients. The ROC curve in the nomogram showed that the 1-, 3- and 5-year forecast values were 0.646, 0.652, and 0.615 (Figure 7D). The correlation between the 18 hypoxia-related genes in the constructed model and clinical factors is shown in box plots, and we only show meaningful genes (Figure S2).
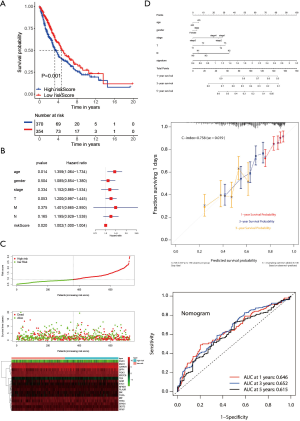
Efficacy of the signature in immunotherapy
The data on the smoking history of patients came from the cBioPortal database. It divided patients into four categories, including current reformed smoker for < or =15 years, current reformed smoker for >15 years, current smoker and lifelong non- smoker. Through analysis, it was shown that the six molecules in the signature had significant differences in these four categories (Figure 8A). The risk score and GAPDH displayed significance in follow up treatment (Figure 8B). And it was worth noting that EPAS1, TFRC, and XRCC6 had significant meanings in the primary therapy outcome (Figure 8C). In order to reflect the significance of prognostic signature in immunotherapy, it was verified in melanoma (Figure 8D) and renal carcinoma (Figure 8E) respectively, and the results showed that the HRGs-built signature had significant significance in immunotherapy (18,19).
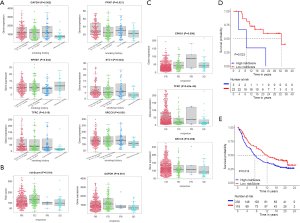
Discussion
Studies have found that HRGs play an indispensable role in inflammation, immunity and tumor growth and development. Moreover, it is worth noting that the overall TME infiltration characteristics mediated by the comprehensive action of multiple HRGs have not been fully explored, which is also the original intention of data mining in this study. Clarifying the role of different HRGs modification patterns in the infiltration of TME cells will help us deepen our understanding of the anti-tumor immune response of TME and guide more valid immunotherapy strategies. On the basis of 76 HRGs, we exposed the modification patterns of three different HRGs. These three patterns not only showed significant differences among the clusters, but also displayed significant TME cell infiltration characteristics. Cluster one was qualified by the activation of stromal and carcinogenic pathways, equivalent to immune-excluded phenotype. Cluster two was qualified by competing activation of immune and stromal pathways that was resemble to antagonistic effects, equivalent to immune-desert phenotype. Cluster three was qualified by the activation of immune-related pathways, equivalent to immune-activation phenotype, which presented as a mass infiltration of immune cells in TME. Though the immune-desert phenotype also showed the infiltration of a variety of immune cells, the activation of the matrix pathway lead to mutual antagonism, which weakened the anti-tumor effect of immune cells, so the survival of the immune-desert phenotype was slightly worse than that of immune-activation phenotype. Compatible with the above definition, we discovered that cluster two showed an important matrix activation state, for example, cluster two showed high expression of angiogenesis, EMT, immune checkpoint and Pan-F-TBRS. Interestingly, in this research, the mRNA transcriptome differences among different HRGs modification patterns have been shown to be markedly related to hypoxia and immune-related biological pathways. The DEG were regarded as HRGs, resemble to the clustering results of hypoxia-related gene modification phenotypes. Based on hypoxia characteristic genes, three sets of genome subtypes have been identified, and they were also significantly related to matrix and immune activation, which again proved that hypoxia modification patterns were of great importance for shaping different TME landscapes. Therefore, we constructed a prognostic model of hypoxia-related genes, and by analyzing the clustering and risk score, we could find that cluster one had a higher risk score and cluster three had a lower risk score. This was also consistent with our predicted results in IMvigor210 cohort. This suggested that the prognostic model could be applied to further determine the pattern of TME infiltration, namely the tumor immunophenotype.
In clinical practice, the prognostic signature can be applied to the HRG modification model and the corresponding TME cell immune infiltration model in a single patient, which is helpful to further determine the immunophenotype of the tumor and to fully realize the clinical practice. In addition, we further assessed the clinicopathologic features of the tumor, including tumor stage and mutation burden. More importantly, this research provides new directions for identifying different immunophenotypes and personalized immunotherapy for cancer. Reversing the characteristics of immune infiltration may help to reverse the progression of cancer, which may provide new ideas for the exploration of cancer treatment and mechanisms. Studies have found that the genes in the model play an important role in cancer. The original purpose of this study was to link these eighteen genes associated with hypoxia to predict NSCLC. Adrenomedullin (ADM) works by supplying and amplifying pathologic neovascularization and lymphatics to generate vital signals. Aryl hydrocarbon receptor (AHR) is part of the tobacco-induced carcinogenic effect, and ADM can greatly promote it (20,21). BCL2 interacting killer (BIK) reduces proliferative epithelial cells by releasing calcium from endoplasmic reticulum storage and causing apoptosis, and BIK peptides may have therapeutic potential in airway diseases related to chronic mucosal hypersecretion (22). DNA damage inducible transcript 3 (DDIT3) promotes stress-induced apoptosis and acts as a transcription factor in many diseases (23). As a glycolytic enzyme, ENO1 plays a key role in glucose metabolism and contributes to many cancer tumors. The reduction of phosphorylation of ENO1 leads to the reduction of phosphorylation of PI3K and AKT, which is further related to the decrease of cell proliferation and tumor progression (24,25). NSCLC may have a mechanism of EPAS1 negative feedback regulation (26). FGF3 is a gene belonging to the fibroblast growth factor gene family. It is related to the carcinogenesis of a variety of cancers, including esophageal squamous cell carcinoma, breast cancer and lung cancer (27). Respiratory inhibitor 3-bromopyruvate (3BP) can inhibit respiration by down-regulating the expression of HK-II and GAPDH, thereby significantly reducing the intracellular oxygen consumption rate and reducing the hypoxia of tumors. The high glycolysis rate in cancer cells is a recognized feature of many human tumors, which can provide metabolites that can be used as precursors of anabolic pathways for cancer cells whose metabolites proliferate rapidly. Maintaining a high glycolysis rate depends on the NADH produced by GAPDH on the regeneration of NAD catalyzed by lactate dehydrogenase, because an increase in the ratio of NADH:NAD will inhibit GAPDH (28,29). Macrophage migration inhibitory factor (MIF) is an inflammatory cytokine that plays many roles in inflammation and immunity. MIF is overexpressed in these malignancies in humans and leads to cell cycle disorders, angiogenesis and metastasis (30,31). An inflammatory cytokine that promotes EMT and invasiveness of cancer cells. SLC26A4-AS1 promotes the transcriptional activity of NPTX1 by recruiting NFKB1, thereby producing anti-angiogenic effects on glioma cells (32). The platelet subtype of phosphofructokinase (PFKP) is a rate-limiting enzyme involved in glycolysis. Overexpression of PFKP inhibits apoptosis and promotes the proliferation, migration, invasion and glycolysis of NSCLC cells (33,34). PFK enhanced the role of glycolysis through PPI3K activation and PI3K/AKT-dependent positive feedback regulation. Phosphoglycerate kinase (PGK1), which is both glycolytic enzyme and protein kinase, plays a crucial role in the development of cancer. Inhibition of glycolysis has been an attractive method to treat cancer since evidence suggests that tumor cells rely more on glycolysis than oxidative phosphorylation. Preliminary evidence suggests that inhibition of phosphoglycerate kinase 1 (PGK1) kinase activity will reverse the Warburg effect and cause tumors (35,36). PLAUR induces human LUAD cells to be resistant to gefitinib through the EGFR/P-AKT/survivin signaling pathway (37). SPP1 overexpression is related to the poor prognosis of multiple cancers, while silencing SPP1 inhibits the proliferation, migration and invasion of cancers (38). STC1 is a glycoprotein known to be involved in inflammation and tumor progression. Cancer cells overexpressing STC1 have an inhibitory effect on the migration/infiltration of macrophages. In addition, STC1 is related to calcium/phosphate homeostasis (39). TEK has been identified as a specific receptor for endothelial cells, which plays an important role in the regulation of angiogenesis and remodeling (40). TFRC promotes the proliferation and metastasis of cancer cells by up-regulating the expression of AXIN2 to accelerate the progress of epithelial ovarian cancer (41). Transforming growth factor (TGFA) stimulates cell growth and proliferation, and its overexpression is associated with the survival rate of patients with many tumors (42). PARP6 inhibits the expression of XRCC6 by inducing degradation, thereby affecting the Wnt/β-catenin signaling pathway, thereby helping to inhibit HCC (43). The DNA protein kinase subunit XRCC6 is necessary for the specific response of these outer membrane proteins in macrophages and epithelial cells (44).
Acknowledgments
Funding: This work was supported by the National Natural Science Foundation of China (81770266,81971761) and “Six-one” Project for High-level Health Talents (LGY2016037) in Jiangsu, China.
Footnote
Reporting Checklist: The authors have completed the MDAR checklist. Available at http://dx.doi.org/10.21037/tlcr-20-1314
Data Sharing Statement: Available at http://dx.doi.org/10.21037/tlcr-20-1314
Conflicts of Interest: All authors have completed the ICMJE uniform disclosure form (available at http://dx.doi.org/10.21037/tlcr-20-1314). The authors have no conflicts of interest to declare.
Ethical Statement: The authors are accountable for all aspects of the work in ensuring that questions related to the accuracy or integrity of any part of the work are appropriately investigated and resolved. The study was in accordance with the Helsinki Declaration (as revised in 2013).
Open Access Statement: This is an Open Access article distributed in accordance with the Creative Commons Attribution-NonCommercial-NoDerivs 4.0 International License (CC BY-NC-ND 4.0), which permits the non-commercial replication and distribution of the article with the strict proviso that no changes or edits are made and the original work is properly cited (including links to both the formal publication through the relevant DOI and the license). See: https://creativecommons.org/licenses/by-nc-nd/4.0/.
References
- Rocha V, Fraga S, Moreira C, et al. Life-course socioeconomic disadvantage and lung function: a multicohort study of 70 496 individuals. Eur Respir J 2021;57:2001600 [Crossref] [PubMed]
- Rivera MP, Katki HA, Tanner NT, et al. Addressing Disparities in Lung Cancer Screening Eligibility and Healthcare Access. An Official American Thoracic Society Statement. Am J Respir Crit Care Med 2020;202:e95-e112. [Crossref] [PubMed]
- Li L, Yang L, Fan Z, et al. Hypoxia-induced GBE1 expression promotes tumor progression through metabolic reprogramming in lung adenocarcinoma. Signal Transduct Target Ther 2020;5:54. [Crossref] [PubMed]
- Liang C, Dong Z, Cai X, et al. Hypoxia induces sorafenib resistance mediated by autophagy via activating FOXO3a in hepatocellular carcinoma. Cell Death Dis 2020;11:1017. [Crossref] [PubMed]
- Wu Q, Yin G, Lei J, et al. KLHL5 Is a Prognostic-Related Biomarker and Correlated With Immune Infiltrates in Gastric Cancer. Front Mol Biosci 2020;7:599110 [Crossref] [PubMed]
- Zhang M, Yang W, Wang P, et al. CCL7 recruits cDC1 to promote antitumor immunity and facilitate checkpoint immunotherapy to non-small cell lung cancer. Nat Commun 2020;11:6119. [Crossref] [PubMed]
- Ma C, Kang W, Yu L, et al. AUNIP Expression Is Correlated With Immune Infiltration and Is a Candidate Diagnostic and Prognostic Biomarker for Hepatocellular Carcinoma and Lung Adenocarcinoma. Front Oncol 2020;10:590006 [Crossref] [PubMed]
- Monkman J, Taheri T, Ebrahimi Warkiani M, et al. High-Plex and High-Throughput Digital Spatial Profiling of Non-Small-Cell Lung Cancer (NSCLC). Cancers (Basel) 2020;12:3551. [Crossref] [PubMed]
- Gong PJ, Shao YC, Huang SR, et al. Hypoxia-Associated Prognostic Markers and Competing Endogenous RNA Co-Expression Networks in Breast Cancer. Front Oncol 2020;10:579868 [Crossref] [PubMed]
- Zhang B, Li B, Zhou YL, et al. m 6 A regulator-mediated methylation modification patterns and tumor microenvironment infiltration characterization in gastric cancer. Mol Cancer 2020;19:53. [Crossref] [PubMed]
- Wang Z, Gao L, Guo X, et al. A novel hypoxic tumor microenvironment signature for predicting the survival, progression, immune responsiveness and chemoresistance of glioblastoma: a multi-omic study. Aging (Albany NY) 2020;12:17038-61. [Crossref] [PubMed]
- Zeng D, Li M, Zhou R, et al. Tumor Microenvironment Characterization in Gastric Cancer Identifies Prognostic and Immunotherapeutically Relevant Gene Signatures. Cancer Immunol Res 2019;7:737-50. [Crossref] [PubMed]
- Chang Y, Li G, Zhai Y, et al. Redox Regulator GLRX Is Associated With Tumor Immunity in Glioma. Front Immunol 2020;11:580934 [Crossref] [PubMed]
- Liu Q, Cheng R, Kong X, et al. Molecular and Clinical Characterization of PD-1 in Breast Cancer Using Large-Scale Transcriptome Data. Front Immunol 2020;11:558757 [Crossref] [PubMed]
- Horvath L, Thienpont B, Zhao L, et al. Overcoming immunotherapy resistance in non-small cell lung cancer (NSCLC) - novel approaches and future outlook. Mol Cancer 2020;19:141. [Crossref] [PubMed]
- Li M, Liang M, Lan T, et al. Four Immune-Related Long Non-coding RNAs for Prognosis Prediction in Patients With Hepatocellular Carcinoma. Front Mol Biosci 2020;7:566491 [Crossref] [PubMed]
- Qi L, Chen J, Yang Y, et al. Hypoxia Correlates With Poor Survival and M2 Macrophage Infiltration in Colorectal Cancer. Front Oncol 2020;10:566430 [Crossref] [PubMed]
- Chen G, Wu Q, Jiang H, et al. Impact of treatment delay due to the pandemic of COVID-19 on the efficacy of immunotherapy in head and neck cancer patients. J Hematol Oncol 2020;13:174. [Crossref] [PubMed]
- Takaya H, Namisaki T, Moriya K, et al. Association between ADAMTS13 activity-VWF antigen imbalance and the therapeutic effect of HAIC in patients with hepatocellular carcinoma. World J Gastroenterol 2020;26:7232-41. [Crossref] [PubMed]
- Greillier L, Tounsi A, Benyahia Z, et al. Functional Analysis of the Adrenomedullin Pathway in Malignant Pleural Mesothelioma. J Thorac Oncol 2016;11:94-107. [Crossref] [PubMed]
- Portal-Nuñez S, Shankavaram UT, Rao M, et al. Aryl hydrocarbon receptor-induced adrenomedullin mediates cigarette smoke carcinogenicity in humans and mice. Cancer Res 2012;72:5790-800. [Crossref] [PubMed]
- Mebratu YA, Leyva-Baca I, Wathelet MG, et al. Bik reduces hyperplastic cells by increasing Bak and activating DAPk1 to juxtapose ER and mitochondria. Nat Commun 2017;8:803. [Crossref] [PubMed]
- Tan W, Liao Y, Qiu Y, et al. miRNA 146a promotes chemotherapy resistance in lung cancer cells by targeting DNA damage inducible transcript 3 (CHOP). Cancer Lett 2018;428:55-68. [Crossref] [PubMed]
- Feng T, Feng N, Zhu T, et al. A SNP-mediated lncRNA (LOC146880) and microRNA (miR-539-5p) interaction and its potential impact on the NSCLC risk. J Exp Clin Cancer Res 2020;39:157. [Crossref] [PubMed]
- Zhou J, Zhang S, Chen Z, et al. CircRNA-ENO1 promoted glycolysis and tumor progression in lung adenocarcinoma through upregulating its host gene ENO1. Cell Death Dis 2019;10:885. [Crossref] [PubMed]
- Xu XH, Bao Y, Wang X, et al. Hypoxic-stabilized EPAS1 proteins transactivate DNMT1 and cause promoter hypermethylation and transcription inhibition of EPAS1 in non-small cell lung cancer. FASEB J 2018; [Crossref] [PubMed]
- Papanikolaou IS, Lazaris AC, Kavantzas N, et al. Minimal expression of the proto-oncogene int-2 encoded protein in a series of colorectal carcinomas. J Gastroenterol Hepatol 2002;17:1084-6. [Crossref] [PubMed]
- Deng Y, Song P, Chen X, et al. 3-Bromopyruvate-Conjugated Nanoplatform-Induced Pro-Death Autophagy for Enhanced Photodynamic Therapy against Hypoxic Tumor. ACS Nano 2020;14:9711-27. [Crossref] [PubMed]
- Birts CN, Banerjee A, Darley M, et al. p53 is regulated by aerobic glycolysis in cancer cells by the CtBP family of NADH-dependent transcriptional regulators. Sci Signal 2020;13:eaau9529 [Crossref] [PubMed]
- Liao CH, Yong CY, Lai GM, et al. Astragalus Polysaccharide (PG2) Suppresses Macrophage Migration Inhibitory Factor and Aggressiveness of Lung Adenocarcinoma Cells. Am J Chin Med 2020;48:1491-509. [Crossref] [PubMed]
- Guda MR, Rashid MA, Asuthkar S, et al. Pleiotropic role of macrophage migration inhibitory factor in cancer. Am J Cancer Res 2019;9:2760-73. [PubMed]
- Li H, Yan R, Chen W, et al. Long non coding RNA SLC26A4-AS1 exerts antiangiogenic effects in human glioma by upregulating NPTX1 via NFKB1 transcriptional factor. FEBS J 2021;288:212-28. [Crossref] [PubMed]
- Wang F, Li L, Zhang Z. Platelet isoform of phosphofructokinase promotes aerobic glycolysis and the progression of non-small cell lung cancer. Mol Med Rep 2021;23:1.
- Lee JH, Liu R, Li J, et al. EGFR-Phosphorylated Platelet Isoform of Phosphofructokinase 1 Promotes PI3K Activation. Mol Cell 2018;70:197-210.e7. [Crossref] [PubMed]
- Wang Y, Sun L, Yu G, et al. Identification of a novel non-ATP-competitive protein kinase inhibitor of PGK1 from marine nature products. Biochem Pharmacol 2021;183:114343 [Crossref] [PubMed]
- Wang WL, Jiang ZR, Hu C, et al. Pharmacologically inhibiting phosphoglycerate kinase 1 for glioma with NG52. Acta Pharmacol Sin 2021;42:633-40. [Crossref] [PubMed]
- Zhou J, Kwak KJ, Wu Z, et al. PLAUR Confers Resistance to Gefitinib Through EGFR/P-AKT/Survivin Signaling Pathway. Cell Physiol Biochem 2018;47:1909-24. [Crossref] [PubMed]
- Liu K, Hu H, Jiang H, et al. Upregulation of secreted phosphoprotein 1 affects malignant progression, prognosis, and resistance to cetuximab via the KRAS/MEK pathway in head and neck cancer. Mol Carcinog 2020;59:1147-58. [Crossref] [PubMed]
- Leung CCT, Wong CKC. Effects of stanniocalcin-1 overexpressing hepatocellular carcinoma cells on macrophage migration. PLoS One 2020;15:e0241932 [Crossref] [PubMed]
- Ha M, Son YR, Kim J, et al. TEK is a novel prognostic marker for clear cell renal cell carcinoma. Eur Rev Med Pharmacol Sci 2019;23:1451-8. [PubMed]
- Huang Y, Huang J, Huang Y, et al. TFRC promotes epithelial ovarian cancer cell proliferation and metastasis via up-regulation of AXIN2 expression. Am J Cancer Res 2020;10:131-47. [PubMed]
- Sauter ER, Coia LR, Eisenberg BL, et al. Transforming growth factor alpha expression as a potential survival prognosticator in patients with esophageal adenocarcinoma receiving high-dose radiation and chemotherapy. Int J Radiat Oncol Biol Phys 1995;31:567-9. [Crossref] [PubMed]
- Tang B, Zhang Y, Wang W, et al. PARP6 suppresses the proliferation and metastasis of hepatocellular carcinoma by degrading XRCC6 to regulate the Wnt/beta-catenin pathway. Am J Cancer Res 2020;10:2100-13. [PubMed]
- Chaudhary A, Kamischke C, Leite M, et al. β-Barrel outer membrane proteins suppress mTORC2 activation and induce autophagic responses. Sci Signal 2018;11:eaat7493 [Crossref] [PubMed]