M5C regulator-mediated methylation modification patterns and tumor microenvironment infiltration characterization in lung adenocarcinoma
Introduction
According to the ribonucleic acid (RNA) modification database (MODOMICS), over 150 RNA modifications have been detected, including 5-methylcytosine (m5C), N6-methyladenosine (m6A), and N1-methyladenosine (m1A) (1,2). 5-methylcytosine (m5C) is one common methylation modification, and plays significant roles in various biological process. M5C modification is a kind of post-transcriptional modification regulated by “writers”, “erasers”, and “readers”, which are methyltransferases, demethylases, and binding proteins, respectively.
Methylation of the cytosine at the fifth carbon position (m5C) is mediated by methyltransferases consisting of NOL1/NOP2/Sun domain family, member 1-7 (NSUN1-7), DNA methyltransferase1 (DNMT1), DNMT2, DNMT3A, and DNMT3B, while the removal process is catalyzed by demethylases such as ten-eleven translocation 2 (TET2) . In addition, a group of specific RNA-binding proteins can read the m5C motif, thereby mediating its function. It has been found that m5C modification in messenger RNA (mRNA) is primarily enriched in the non-translated region (3'UTR and 5'UTR), guanine-cytosine (GC)-rich regions, and near the argonaute (AGO) protein binding site, which has a conserved sequence of AU(m5C)GANGU (3-6).
Immunotherapy has been an effective treatment against cancer, and is represented by immunological checkpoint blockades (ICBs). However, the overall response rates are still unsatisfying, especially for cancers with low mutational burdens (7). In recent years, with the development of immunotherapy, the therapeutic options for cancer treatment have undergone significant changes (8-11). Among the various immunotherapies, ICBs work by blocking the interaction between immunosuppressive receptors (immune checkpoints) expressed on the surface of immunocytes and their ligands. ICBs include a series of monoclonal antibody-based therapies. Cytotoxic T-lymphocyte-associated protein 4 (CTLA-4), programmed death 1 (PD-1) and programmed death-ligand 1 (PD-L1) are the main targets of immunotherapy (12,13). ICB has attracted widespread attention due to its persistence in reaction and its impact on the overall survival of patients. However, the challenge for clinicians is to determine who will respond to immunotherapy. The number of patients who actually benefit from immunotherapy remains small (14-16).
The complexity and strong interrelationship of the tumor microenvironment (TME), which comprises immune cells (such as macrophages, mast cells, polymorphonuclear cells, dendritic cells, natural killer (NK) cells, as well as T and B lymphocytes) and non-immune cells (including endothelial cells and stromal cells), play a key role in its development and progression (17,18). The immune cell components of the tumor are the basis for determining the fate of the tumor, as well as its invasion and metastasis. Interacting with other TME components either directly or indirectly can lead to a variety of biological behavioral changes in tumor cells, including proliferation and angiogenesis, apoptosis, hypoxia, and immune tolerance (19). An increasing number of studies have shown that the TME has a crucial impact on tumor progression, immunotherapy response, and immune escape (20,21). Recently, one research has also showed that under a scenario of balanced autophagy in the tumor microenvironment, the infiltrating immune cells control cytokine production and secretion (22). Sacco et al. indicated that tumor-infiltrating immune cells could affect the tumor immunosurveillance by regulating the iron metabolism (23). Therefore, the characteristics of tumor immune infiltration can provide new strategies for the prediction of immunotherapeutic effect, the improvement of immunotherapeutic response rate, and the development of novel immunotherapeutic targets.
Recently, several studies have shown a strong association between m5C modification and TME infiltrating immune cells. Schoeler et al. reported that TET enzymes control antibody production and shape the mutational landscape in germinal center B cells. They found that TET2 and TET3 guide the transition of germinal center B cells to antibody-secreting plasma cells (24). Also, Li et al. revealed that the TET family modulates the activation of dendritic cells. TET1-inhibited monocyte-derived dendritic cells were found to significantly decrease the percentage of CD45RA-FoxP3hi-activated regulatory T (Treg) cells in the allergic rhinitis group, which might be linked to immune activation (25). Yue et al. found that TET2/3-deficiency in Treg cells leads to T cell activation, TET2/3 double-knockout (DKO) Treg cells exhibited a dysregulated cell surface phenotype, and TET2/3 DKO CD4+ T cells induced disease in healthy mice (26). Moreover, some researches have focused on the intrinsic pathways of cancer cells, such as genomic variation and the disordered expression of m5C regulators. Chen et al. indicated that numerous oncogene RNAs with hypermethylated m5C sites were causally linked to their upregulation in urothelial carcinoma of the bladder, and demonstrated that Y-box binding protein 1 (Ybx1) is an m5C “reader” (27). Lastly, USUN5 expression has been verified to be related to shorter survival in glioblastoma and the high expression of USUN7 is correlated to the poor survival in low-grade gliomas (28,29).
However, the above studies only mentioned the role and mechanism of one or two regulatory factors of m5C in antitumor and immune processes, while the potential cross-talk between regulators remains uncharacterized in human cancers. Therefore, establishing a comprehensive understanding of the TME cell infiltration characterization mediated by m5C regulators will offer insight into TME immune regulation. In this study, we analyzed the gene mutation of The Cancer Genome Atlas (TCGA) lung adenocarcinoma (LUAD), the mRNA expression data, and the clinical information of patients. We also investigated the mechanisms through which m5C affected the prognosis of patients during the occurrence of LUAD, and further verified the results in an external dataset (GSE31210). We present the following article in accordance with the MDAR reporting checklist (available at http://dx.doi.org/10.21037/tlcr-21-351).
Methods
Dataset source and preprocessing
We conducted a systematic search of TCGA and the Gene-Expression Omnibus (GEO) databases for LUAD. Standardized matrix files of each cohort were downloaded for further analysis. The study was conducted in accordance with the Declaration of Helsinki (as revised in 2013). The procedure of data preprocessing lists was as follows: (I) we downloaded data of TCGA LUAD single nucleotide variation (SNV) (MuTect2 Annotation), which included 570 samples; (II) we downloaded data of TCGA LUAD copy number variation (CNV), which included 544 samples. We re-annotated the CNV of 13 genes using Bedtools software using hg38 as a reference (30); (III) we downloaded data of TCGA LUAD FPKM (Fragments Per Kilobase Million), which included 572 samples (513 tumor samples and 59 normal samples) and 513 follow-up data; and (IV) we downloaded 246 samples of the expression profile and follow-up data of GSE31210 from National Coalition Building Institute (NCBI) GEO, which included 226 tumor samples and 20 normal samples. The study roadmap is shown in Figure 1.
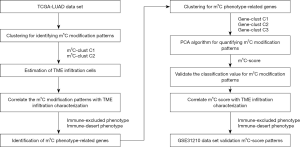
Unsupervised clustering for 11 m5C regulators
We extracted 11 regulators related to m5C that had expression in TCGA datasets for LUAD analysis using the prCOMP function in R language (13 genes related to m5C modification were detected, but only 11 had expression). These 13 regulators comprised 11 writers (NSUN2, NSUN3, NSUN4, NSUN5, NSUN6, NSUN7, DNMT1, DNMT2, DNMT3B, NSUN1, DNMT3A), one eraser (TET2), and one reader (Aly/REF export factor, ALYREF). In order to identify different m5C modification patterns and classify patients for further study, unsupervised clustering analysis was applied. The 11 m5C regulators were clustered with LUAD tumor samples by non-negative matrix factorization (NMF). The NMF method selected the standard “Brunet” and carried out 100 iterations. The number of clusters was set from 2 to 10, and we determined the average contour width of the common member matrix using the R package “NMF”, setting the minimum members of each subclass to 10. We selected the optimal clustering number as 2 based on the cophenetic, dispersion, and silhouette.
Gene set variation analysis (GSVA) and functional annotation
In order to explore the biological behavior between these different m5C modification patterns, GSVA enrichment analysis was carried out using the R language GSVA package (31), and the “c2.cp.kegg.v7.0.symbols.gmt” gene set was used as the background. GSVA, in a non-parametric and unsupervised method, is commonly employed for estimating the variations in pathway and biological process activity in the samples of an expression dataset. Differential pathways were screened by |t| >6 using the R package limma.
Estimation of TME cell infiltration
The cell type identification by estimating relative subsets of RNA transcripts (CIBERSORT) method was used to analyze the composition and relative abundance of m5C-modified immune cells of the two patterns. Since T cells.CD4.memory.activated was 0 in all samples, we removed the cells and calculated the correlation and significance of 11 m5C-related genes and TME infiltration types through the rcorr function of the R language Hmisc package. We also used the ESTIMATE algorithm to quantify the immune, matrix, and ESTIMATE scores between groups of high and low expression regulators.
Identification of differentially expressed genes (DEGs) between m5C distinct phenotypes
Previously, two m5C modification patterns were classified by clustering m5C-related genes. In the next step, we carried out principal component analysis (PCA) of these two subtypes, and the two patterns were separated from each other. Using the R package limma package for difference analysis, 226 differential genes were screened by |log2fold change| >1, false discovery rate (FDR) <0.05. The patients were divided into different gene clusters by unsupervised clustering of 226 m5C phenotype-related genes (the distance between samples was calculated by complete and Euclidean).
Generation of the m5C gene signature
Due to the heterogeneity and complexity of m5C modification, we constructed a scoring system to quantify the m5C modification pattern of individual LUAD patients based on these phenotypic genes, which was called the m5C score. We then performed a prognostic analysis on each gene in the signature using a univariate Cox regression model. We screened 124 genes related to prognosis with P<0.05 from 226 DEGs, and subsequently analyzed the 124 genes by PCA, scored PC1 and PC2, and calculated the m5C score of each sample. The formula was as follows:
m5C score = Σ (PC1i + PC2i)
where i is the expression of 125 m5C phenotype-related genes.
Statistical analysis
Spearman and distance correlation analyses were utilized to compute correlation coefficients between the expression of m5C regulators and TME infiltrating immune cells. To analyze difference between two groups, the Wilcoxon test was used, and in cases of three or more groups, difference comparisons were conducted using Kruskal-Wallis tests and one-way ANOVA (analysis of variance). For verification of the external dataset GSE31210, m5C score model samples were divided into high and low score subgroups according to the median. Using the survminer R package, survival curves were generated using log-rank tests and the Kaplan-Meier (KM) method. Statistical significance was set at P<0.05, and all statistical P values were two-sided. All data was processed using R 3.6.1 software.
Results
Genetic variation of m5C regulators in LUAD
Thirteen m5C regulators were identified in this study, including 11 writers, one eraser, and one reader. We first summarized the incidence of SNV and CNV in the 13 m5C regulators in LUAD. Figure S1 shows the dynamic and reversible regulation of m5C RNA methylation.
SNV analysis of m5C related genes
Of the 570 LUAD patients, gene mutations of the 13 m5C regulators appeared in 99 independent samples, with a frequency of 15.75%. The writer, DNMT3A, exhibited the highest incidence of mutation, followed by NSUN2, TET2, and DNMT3B, while “reader” genes had fewer mutations than “writer” and “eraser” genes. Figure 2A displays the mutations in the top 10 genes associated with m5C, including variant classification, type, and variants per sample.
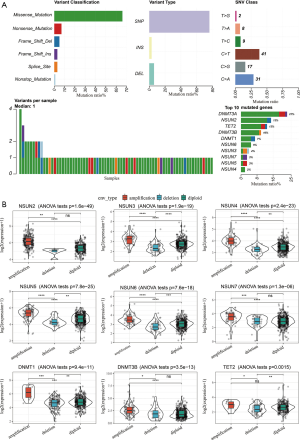
CNV analysis of m5C related genes
In addition to SNV, CNVs are also present as genetic variations, including amplification (Segment_Mean >0.2), diploid (−0.2< Segment_Mean <0.2), and deletion (Segment_Mean<−0.2). Table 1 shows the proportion of amplification and deletion of the 11 genes. We examined the incidence of CNV and the mRNA expression of these regulators to explore the relationship between gene variations and the expression levels of m5C regulators (Figure 2B), and found that CNV could be the key factor leading to the disordered expression of m5C regulators. The expression of m5C regulators in LUAD tissue was significantly higher than that in normal lung tissue (except NSUN3 and TET2) (Figure S2).

Full table
In total, nine CNV gene mutations had quantitative values in the gene expression profile. We observed that genes that experienced amplification showed higher expression, while those that experienced deletion exhibited lower expression. NSUN2, DNMT3B, ALYREF, and NSUN5 had a high frequency of CNV amplification, while DNMT1 and TET2 exhibited a high frequency of CNV deletion. These gene mutations may affect the transmission of the m5C signal in cells and result in cellular functional disorder. Among them, NSUN2, DNMT3b, NSUN5 and DNMT1 are writers, ALYREF is a reader, and TET2 is an eraser. Mutations of NSUN2, DNMT3b, ALYREF, NSUN5, DNMT1, and TET2 suggested that the function of m5C in tumor cells may be abnormal. The above analyses demonstrated the high heterogeneity of the genetic and expressional alteration landscape in m5C regulators between LUAD samples, indicating that the expression imbalance between m5C regulators plays a crucial role in the occurrence and progression of LUAD.
M5C methylation modification patterns mediated by 11 regulators
PCA analyses of m5C-related genes
We extracted 11 m5C-related genes from TCGA and performed PCA analyses using prCOMP (there were 13 genes related to m5C modification, but only 11 genes with a quantitative expression level). The first three principal components were shown by pca3d in Figure 3A. The 11 m5C-related samples could be completely distinguished between tumor samples and normal samples.
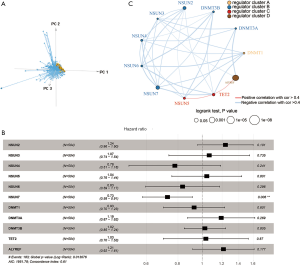
Network analyses of m5C-related genes
LUAD tumor samples from TCGA with available overall survival (OS) data and clinical information were enrolled into one meta-cohort. The prognostic values of the 11 m5C regulators were revealed via a univariate Cox regression model (Figure 3B). The 11 regulators were not related to the prognosis of LUAD patients, except for NSUN7, which also indicated that these 11 genes may indirectly interfere with the prognosis of LUAD patients. The m5C regulatory network described the integrated view of the mutual effect of m5C regulators, regulator connection, and their prognostic value for LUAD patients (Figure 3C and Table S1). The 11 genes were divided into four clusters. We found a correlation between expression and functional category of similar m5C regulators. ALYREF may be a key gene of m5C regulators, which affects the prognosis of LUAD through forward and reverse regulation of the other 10 genes.
TME cell infiltration characteristics in distinct m5C modification patterns
Identification of m5C modified subtypes (m5C clusters)
We used the NMF R package to classify patients into two distinct modification patterns via unsupervised clustering, according to the expression quantity of 11 m5C regulators (Figure 4A,B). A total of 504 samples were included, including 152 samples for cluster C1 and 352 samples for cluster C2. We termed these patterns: m5C cluster C1 and C2, respectively. Furthermore, prognostic analysis for the two main m5C modification subtypes was also performed, and the results showed significant differences in OS between cluster C1 and C2 (Figure 4C). The m5C cluster C2 modification pattern exhibited a significant survival advantage. Then, we analyzed the expression of 11 m5C regulators in the two main m5C modification subtypes. The expression of the 7 genes among 11 regulators were significantly different between cluster C1 and C2, and all 7 genes’ expression is higher in the cluster C1 (Figure 4D).
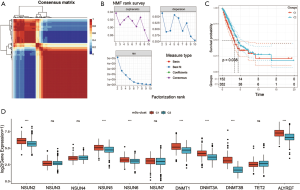
Functional enrichment of m5C modified subtypes
In order to explore the biological behavior of these different m5C modification patterns, enrichment analysis of GSVA was carried out using R language GSVA package, with the c2 cp.kegg.v7.0.symbols.gmt gene set as a background. A total of 187 pathways were enriched, and 39 differential pathways were screened by |t|>6. The m5C C1 subgroup was enriched in 14 pathways, mainly related to matrix pathways such as cell cycle and DNA (deoxyribonucleic acid) repair, while C2 was enriched in 25 pathways, mainly related to signal transduction and immune pathways (such as Fc epsilon RI signaling pathway and the mitogen-activated protein kinase (MAPK) signaling pathway) (Figure 5).
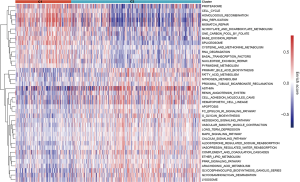
TME analyses of m5C modified subtypes
The CIBERSORT method was used to analyze the composition of immune cells of two m5C modification patterns (32). C1 was primarily composed of naïve B cells, activated CD4 memory T cells, follicular T helper cells, resting NK cells, M0 macrophages, and M1 macrophages, while C2 was mainly composed of memory B cells, resting CD4 memory T cells, monocytes, M2 macrophages, resting dendritic cells, resting mast cells, neutrophils, and eosinophils (Figure 6A).
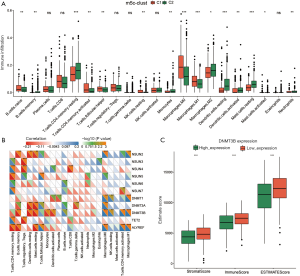
The correlation between m5C-related genes and TME infiltration type was calculated using the rcorr function of Hmisc package in R language. As shown in Figure 6B, the DNMT3B gene was significantly associated with 10 TME infiltrating immune cell groups, of which, six were composed of m5C modified C1 immune cells (naïve B cells, activated CD4 memory T cells, follicular T helper cells, resting NK cells, M0 macrophages and M1 macrophages). The remaining four were composed of immune cells of the C2 subgroup (memory B cells, resting CD4 memory T cells, resting dendritic cells and resting mast cells). We used the ESTIMATE algorithm to quantify DNMT3B (Figure 6C). DNMT3B expression was inversely correlated with the immune, matrix, and ESTIMATE scores. Furthermore, we analyzed the expression of DNMT3B in 21 immune cells, and found that the low expression of DNMT3B was significantly increased in the 21 immune cells (Figure 7A).
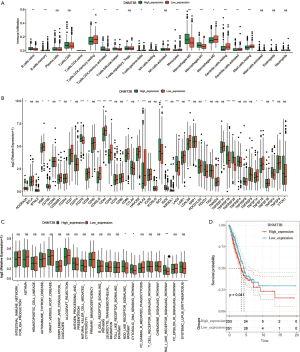
Next, we analyzed the relationship between the expression of DNMT3B and ICB inhibitors. Abnormal expression of DNMT3B was associated with immune function disorder (Figure 7B). Subsequent analyses of pathway enrichment revealed that tumors with high DNMT3B expression exhibited enrichment in the Nod-like receptor (NLR) signaling pathway, cytosolic DNA-sensing pathway, and RIG-I-like receptor (RLR) signaling pathway (Figure 7C). Furthermore, we analyzed the OS of high and low expression groups of DNMT3B. The results showed that low DNMT3b gene expression group was associated with immunity and had a better prognosis (Figure 7D).
Generation of m5C gene signatures and functional annotation
Using the limma package from R language, 226 DEGs were screened by |log2fold change| >1 and FDR <0.05, all of which were related to the m5C phenotype. The patients were divided into three different gene cluster subtypes through unsupervised clustering of 226 m5C phenotype-related genes (the cluster method was complete and Euclidean was used to calculate the distance between samples). PCA analysis demonstrated that they were separated from each other (Figure 8A). These three clusters were named m5C gene cluster C1–C3. We also observed the distribution of the 11 genes in the three m5C gene clusters (Figure 8B), and found that most samples of gene cluster C2 and C3 were included in m5C cluster C2, and most samples of gene cluster C1 coincided with m5C cluster C1. In order to further determine which biological processes these 226 genes were primarily involved in, R language WebGestaltR package was used for Kyoto Encyclopedia of Genes and Genomes (KEGG) enrichment analysis (31441146) (33). We screened a total of five pathways (by P<0.05): cell cycle, oocyte meiosis, progesterone mediated oocyte maturation, cellular sense, and the p53 signaling pathway. The 226 genes were associated with m5C modification and were significantly related to tumorigenesis (Figure 8C).
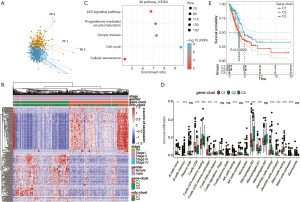
Subsequently, the distribution of 21 immune cells in the three subtypes of the m5C gene cluster was analyzed. As shown in Figure 8D, the three subtypes were statistically significant in 14 cells. Thus, it was clear that m5C modification had a critical role in TME, and the 226 genes modified by m5C also played an important role in the TME. We further analyzed the KM curve of gene clusters C1–C3, and found that these three subtypes were associated with prognosis (P<0.05, Figure 8E). Although the samples were divided into three subtypes, there were only nine cases of C3 samples. These results were consistent with the classification of m5C modification patterns. The prognosis of C2 was superior to that of C1.
Establishment of the m5C score model
Due to the individual heterogeneity and complexity of m5C modification, a scoring system was constructed to quantify the m5C modification pattern of individual LUAD patients, which was called the m5C score. Firstly, we screened 124 genes related to prognosis (P<0.05) from 226 isoform differential genes. Table S2 shows the results of the univariate COX analysis of 124 genes. PCA analysis was then performed on the 124 genes, PC1 and PC2 scores were taken, and the m5C score of each sample was calculated as follows: m5C-score=ΣPC1i+PC2i. The m5C score results of the 513 samples are displayed in Table S3.
We divided the high and low score groups according to the median of the m5C score and used the alluvial diagram to demonstrate the changes between m5C clusters, gene clusters, and m5C scores (Figure 9A). We found that most of the samples of the m5C cluster C2 subtype with good prognosis were identical with those of the gene cluster C2 subtype, and patients with good prognosis primarily exhibited a high m5C score.
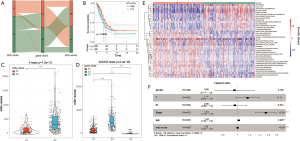
To further verify the relationship between our m5C score model and the prognosis of LUAD, we divided the high and low score groups according to the median m5C score. Survival analysis was then performed between these two groups. We observed that the high m5C score group had a better prognosis, which was consistent with the results of the previous analysis (Figure 9B).
As shown in Figure 9C, there was a significant difference in the m5C scores among the three gene cluster subtypes, with cluster C2 scoring the highest, and cluster C1 the lowest, which also verified that a high m5C score had a good prognosis. Additionally, m5C score difference was also statistically significant between the two m5C cluster subtypes (Figure 9D). The score of the C2 subtype was markedly higher than that of the C1 subtype, and the prognosis of C2 was better than that of C1, which further verified that a high m5C score had a better prognosis. Therefore, a high m5C score may predict a good prognosis for LUAD patients, while a low m5C score may predict a poor prognosis.
We also performed GSVA analysis to further explore the biological process involved in the m5C score difference. We found that the low m5C score group was mainly related to pathways of DNA repair, cell cycle, and stroma, while the high m5C score group was primarily associated with immune-related pathways and MAPK signaling pathways (Figure 9E). Furthermore, through multivariate Cox regression model analysis, we found that m5C score was an independent prognostic factor (sample with missing clinical information removed) (Figure 9F).
Moreover, we analyzed the expression of 11 m5C regulators in the high and low m5C score groups. The expression of seven regulators exhibited significant correlation with m5C score. As shown in Figure 10, in addition to TET2, a high m5C score also corresponded to low gene expression (NSUN2, NSUN5, DNMT1, DNMT3A, DNMT3B, and ALYREF).
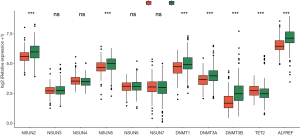
Validation of external datasets
Establishment of GSE31210 dataset m5C score
The PCA analysis results of 125 genes obtained from the previous analysis were used to establish a new m5C score model based on the GSE31210 dataset. In total, 116 genes were identified in the GSE31210 dataset, which were used to establish the m5C score model for 226 tumor samples in GSE31210. First, through PCA analysis, PC1 and PC2 of the 116 genes were calculated, and the m5C score was calculated for each sample. Figure 11A shows the distribution of the 11 genes in the high and low m5C scores. Figure 11B displays the prognosis of the high and low m5C score groups; a high m5C score may lead to a good prognosis, which was consistent with the results of TCGA.
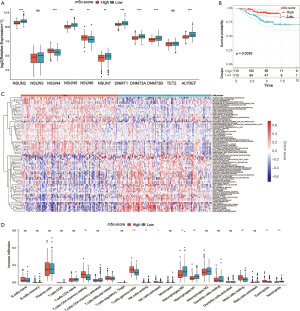
GSVA analysis of the high and low m5C score groups
In order to further investigate mechanisms through which the m5C score affected biological processes, we performed GSVA analysis using the R language. The results showed that the high m5C score group was associated with immune pathways, such as the complement and coagulation cascades, leukocyte transendothelial migration, and the intestinal immune network for immunoglobulin A (IgA) production. Meanwhile, the low score group was associated with the pathways related to the stroma, such as basal resection and repair, cell cycle, etc. (Figure 11C).
These results verified that high m5C scores were related to an immune desert type, which predicted a good prognosis, while low m5C scores indicated an immune exclusion phenotype, which suggested a poor prognosis. The table online (https://cdn.amegroups.cn/static/public/tlcr-21-351-1.xlsx) exhibits the enrichment scores of all samples in 186 pathways, and Table S4 shows the enrichment results of the high and low m5C score groups.
Composition of immune cells in the high and low levels of the m5C score model
To further verify the immunophenotype of the high and low m5C score groups of the dataset, we used CIBERSORT to analyze the composition of immune cells in the high and low m5C score groups (Figure 11D). The high m5C score exhibited more infiltration of resting CD4 memory T cells and resting mast cells, as well as less infiltration of M0 and M1 macrophages, which was similar to the immunocyte infiltration of gene cluster C2.
Discussion
With the development of deep sequencing and mass spectrometry (30), accumulating evidence has suggested that m5C modification is very important for maintaining the normal physiological function of cells and organisms (31-36), while its abnormal distribution and expression are closely related to tumor development. Studies have confirmed that m5C is involved in the progression of hepatocellular carcinoma (37,38). Also, there is increasing evidence that methylation regulatory factors can be used as prognostic and diagnostic markers of cancer (39-43). For example, the high expression of NSUN1 has been identified as a prognostic marker for non-small cell lung cancer (44-46). Recent studies have also confirmed that m5C may affect the behavior of immune cells, such as CD+ T cells (47). Since most studies have focused on the effect of single TME cell types or regulators on tumor development, there remains a lack of comprehensive recognition of TME infiltration mediated by multiple m5C regulators. Further understanding of the role of different m5C modification patterns in the infiltration of TME cell will help to improve our understanding of the TME antitumor immune response and provide novel immunotherapy strategies.
In this study, two m5C methylation modification patterns were revealed according to 11 m5C regulators, which had remarkably distinct TME immune cell infiltration characterization. Also, three genomic subtypes of the m5C gene were identified based on 226 m5C phenotype-related DEGs, which were also significantly related to tumor occurrence. This further revealed the important role of m5C modification in influencing the TME landscape. Identification of the m5C modification patterns of individual tumors was crucial due to the individual heterogeneity of m5C modification. Thus, a scoring system was constructed to assess the m5C modification pattern of LUAD patients. The m5C cluster C2 exhibited a higher m5C score, and patients in the m5C cluster C2 showed better prognosis. The high m5C score group had a better prognosis, while the low m5C score group had a poor prognosis. These results were further verified in the GSE31210 dataset, which indicated that the m5C score was a reliable method for the integrated evaluation of distinct tumor m5C modification patterns. Comprehensive analyses also proved that the m5C score was an independent prognostic marker in LUAD. Functional enrichment analyses in the groups with better prognosis tended to be associated with immunity; m5C cluster C2 exhibited enrichment pathways related to immunity, such as the Fc epsilon RI signaling pathway, and the high m5C score group in the GSE31210 dataset was correlated with immune pathways, such as the complement and coagulation cascades, leukocyte trans-endothelial migration, and the intestinal immune network for IgA production. NSUN2, NSUN5, DNMT1, DNMT3A, DNMT3B, and ALYREF were highly expressed in m5C cluster C2, as well as in TCGA and GSE31210 low m5C score groups, which had a poor prognosis. Above, we analyzed immune cell infiltration, immune checkpoint characteristics, and functional enrichment analysis among different expression levels of DNMT3B in LUAD.
Our study provides some insight for clinical application. Our m5C score system could serve as a reliable and independent biomarker for predicting the prognosis of patients with LUAD. Our findings may help to screen suitable patients who can benefit from immune checkpoint inhibitor therapy. Further research based on these m5C regulators, which regulate TME immune cell infiltration, may contribute to the discovery of novel immune drug combination treatment strategies or new immunotherapeutic agents, and promote the development of individual tumor immunotherapy.
The methylation modification patterns of gastric cancer, LUAD, and other cancers, which are mediated by the m6A modulator, and the invasion characteristics of the TME have been studied, and the m6A modulator is closely related to the tumor immunophenotype (48-53). Studies have also revealed that cross-talk between m6A and m5C regulators is associated with tumor immunogenicity and prognosis in 33 cancer types (54). In future studies, we will also aim to explore whether m5C and m6A have a synergistic effect on LUAD tumor microenvironmental characteristics and the patients’ response to immunotherapy. We will also further investigate how genes (NSUN2, NSUN5, DNMT1, DNMT3A, DNMT3B and ALYREF) that are highly expressed in groups with poor prognosis work. In addition, we cannot rule out the possibility that m5C regulatory factors affect the behavior of the matrix in the TME. Some researchers have found that m5C is related to PM2.5-induced pulmonary fibrosis in mice (55), thus the regulatory behavior of m5C on the TME may be complex.
Our study had limitations that should be noted. Firstly, we did not consider the correlation between immune infiltration location and TME heterogeneity. Secondly, due to the limited clinical annotation in public datasets, the clinicopathological parameters detected in this study are not comprehensive, which may contribute to potential bias in the predictive performance when the m5C score signature served as a prognosis biomarker. Thirdly, due to the time constraints and lack of enough budget, we haven’t carried out relevant experiments now. In future work, we will conduct further experiments to validate the results. Finally, due to the lack of overall clinical information in the datasets involved, we could not directly analyze the correlation between m5C score and the response of LUAD patients to immunotherapy.
Conclusions
In this study, we found that m5C modification played a significant role in formation of TME diversity and complexity. Based on the characteristics of m5C modification, a score model was constructed to predict the prognosis of LUAD patients, which was also verified in the external dataset. We believe that m5C modification will have some implications for tumor immunotherapy in the future.
Acknowledgments
This research was partly presented as an e-Poster at European Lung Cancer Virtual Congress during 25–27 March 2021.
Funding: This work was supported by the Chinese Society of Clinical Oncology/Beijing Xisike Clinical Oncology Research Foundation (Y-Young2020-0003) and the Shanghai Sailing Program (20YF1408300, 19YF1409200).
Footnote
Reporting Checklist: The authors have completed the MDAR reporting checklist. Available at http://dx.doi.org/10.21037/tlcr-21-351
Conflicts of Interest: All authors have completed the ICMJE uniform disclosure form (available at http://dx.doi.org/10.21037/tlcr-21-351). The authors have no conflicts of interest to declare.
Ethical Statement: The authors are accountable for all aspects of the work in ensuring that questions related to the accuracy or integrity of any part of the work are appropriately investigated and resolved. The study was conducted in accordance with the Declaration of Helsinki (as revised in 2013).
Open Access Statement: This is an Open Access article distributed in accordance with the Creative Commons Attribution-NonCommercial-NoDerivs 4.0 International License (CC BY-NC-ND 4.0), which permits the non-commercial replication and distribution of the article with the strict proviso that no changes or edits are made and the original work is properly cited (including links to both the formal publication through the relevant DOI and the license). See: https://creativecommons.org/licenses/by-nc-nd/4.0/.
References
- Boccaletto P, Machnicka MA, Purta E, et al. MODOMICS: a database of RNA modification pathways. 2017 update. Nucleic Acids Res 2018;46:D303-D307. [Crossref] [PubMed]
- Roundtree IA, Evans ME, Pan T, et al. Dynamic RNA Modifications in Gene Expression Regulation. Cell 2017;169:1187-200. [Crossref] [PubMed]
- Chen K, Zhao BS, He C. Nucleic Acid Modifications in Regulation of Gene Expression. Cell Chem Biol 2016;23:74-85. [Crossref] [PubMed]
- García-Vílchez R, Sevilla A, Blanco S. Post-transcriptional regulation by cytosine-5 methylation of RNA. Biochim Biophys Acta Gene Regul Mech 2019;1862:240-52. [Crossref] [PubMed]
- Squires JE, Patel HR, Nousch M, et al. Widespread occurrence of 5-methylcytosine in human coding and non-coding RNA. Nucleic Acids Res 2012;40:5023-33. [Crossref] [PubMed]
- Khoddami V, Cairns BR. Identification of direct targets and modified bases of RNA cytosine methyltransferases. Nat Biotechnol 2013;31:458-64. [Crossref] [PubMed]
- He X, Xu C. Immune checkpoint signaling and cancer immunotherapy. Cell Res 2020;30:660-9. [Crossref] [PubMed]
- Brahmer J, Reckamp KL, Baas P, et al. Nivolumab versus Docetaxel in Advanced Squamous-Cell Non-Small-Cell Lung Cancer. N Engl J Med 2015;373:123-35. [Crossref] [PubMed]
- Wu YL, Lu S, Cheng Y, et al. Nivolumab Versus Docetaxel in a Predominantly Chinese Patient Population With Previously Treated Advanced NSCLC: CheckMate 078 Randomized Phase III Clinical Trial. J Thorac Oncol 2019;14:867-75. [Crossref] [PubMed]
- Herbst RS, Baas P, Kim DW, et al. Pembrolizumab versus docetaxel for previously treated, PD-L1-positive, advanced non-small-cell lung cancer (KEYNOTE-010): a randomised controlled trial. Lancet 2016;387:1540-50. [Crossref] [PubMed]
- Borghaei H, Paz-Ares L, Horn L, et al. Nivolumab versus Docetaxel in Advanced Nonsquamous Non-Small-Cell Lung Cancer. N Engl J Med 2015;373:1627-39. [Crossref] [PubMed]
- Qureshi OS, Zheng Y, Nakamura K, et al. Trans-endocytosis of CD80 and CD86: a molecular basis for the cell-extrinsic function of CTLA-4. Science 2011;332:600-3. [Crossref] [PubMed]
- Sharpe AH, Pauken KE. The diverse functions of the PD1 inhibitory pathway. Nat Rev Immunol 2018;18:153-67. [Crossref] [PubMed]
- Champiat S, Dercle L, Ammari S, et al. Hyperprogressive Disease Is a New Pattern of Progression in Cancer Patients Treated by Anti-PD-1/PD-L1. Clin Cancer Res 2017;23:1920-8. [Crossref] [PubMed]
- van Kesteren MTR, Meeter M. How to optimize knowledge construction in the brain. NPJ Sci Learn 2020;5:5. [Crossref] [PubMed]
- Martini C, Marrucci W, Lucacchini A, et al. Specific inhibition of benzodiazepine receptor binding by some 1,2,3-triazole derivatives. J Pharm Sci 1988;77:977-80. [Crossref] [PubMed]
- Hanahan D, Weinberg RA. Hallmarks of cancer: the next generation. Cell 2011;144:646-74. [Crossref] [PubMed]
- Fridman WH, Pages F, Sautes-Fridman C, et al. The immune contexture in human tumours: impact on clinical outcome. Nat Rev Cancer 2012;12:298-306. [Crossref] [PubMed]
- Petitprez F, Meylan M, de Reynies A, et al. The Tumor Microenvironment in the Response to Immune Checkpoint Blockade Therapies. Front Immunol 2020;11:784. [Crossref] [PubMed]
- Fridman WH, Zitvogel L, Sautes-Fridman C, et al. The immune contexture in cancer prognosis and treatment. Nat Rev Clin Oncol 2017;14:717-34. [Crossref] [PubMed]
- Dunn GP, Old LJ, Schreiber RD. The three Es of cancer immunoediting. Annu Rev Immunol 2004;22:329-60. [Crossref] [PubMed]
- Gonçalves RC, Freire PP, Coletti D, et al. Tumor Microenvironment Autophagic Processes and Cachexia: The Missing Link? Front Oncol 2021;10:617109 [Crossref] [PubMed]
- Sacco A, Battaglia AM, Botta C, et al. Iron Metabolism in the Tumor Microenvironment-Implications for Anti-Cancer Immune Response. Cells 2021;10:303. [Crossref] [PubMed]
- Schoeler K, Aufschnaiter A, Messner S, et al. TET enzymes control antibody production and shape the mutational landscape in germinal centre B cells. FEBS J 2019;286:3566-81. [Crossref] [PubMed]
- Li H, Lu T, Sun W, et al. Ten-Eleven Translocation (TET) Enzymes Modulate the Activation of Dendritic Cells in Allergic Rhinitis. Front Immunol 2019;10:2271. [Crossref] [PubMed]
- Yue X, Lio CJ, Samaniego-Castruita D, et al. Loss of TET2 and TET3 in regulatory T cells unleashes effector function. Nat Commun 2019;10:2011. [Crossref] [PubMed]
- Chen X, Li A, Sun BF, et al. 5-methylcytosine promotes pathogenesis of bladder cancer through stabilizing mRNAs. Nat Cell Biol 2019;21:978-90. [Crossref] [PubMed]
- Janin M, Ortiz-Barahona V, de Moura MC, et al. Epigenetic loss of RNA-methyltransferase NSUN5 in glioma targets ribosomes to drive a stress adaptive translational program. Acta Neuropathol 2019;138:1053-74. [Crossref] [PubMed]
- Sato K, Tahata K, Akimoto K. Five Genes Associated With Survival in Patients With Lower-grade Gliomas Were Identified by Information-theoretical Analysis. Anticancer Res 2020;40:2777-85. [Crossref] [PubMed]
- Quinlan AR, Hall IM. BEDTools: a flexible suite of utilities for comparing genomic features. Bioinformatics 2010;26:841-2. [Crossref] [PubMed]
- Hänzelmann S, Castelo R, Guinney J. GSVA: gene set variation analysis for microarray and RNA-seq data. BMC Bioinformatics 2013;14:7. [Crossref] [PubMed]
- Newman AM, Liu CL, Green MR, et al. Robust enumeration of cell subsets from tissue expression profiles. Nat Methods 2015;12:453-7. [Crossref] [PubMed]
- Kanehisa M. Toward understanding the origin and evolution of cellular organisms. Protein Sci 2019;28:1947-51. [Crossref] [PubMed]
- Glasner H, Riml C, Micura R, et al. Label-free, direct localization and relative quantitation of the RNA nucleobase methylations m6A, m5C, m3U, and m5U by top-down mass spectrometry. Nucleic Acids Res 2017;45:8014-25. [Crossref] [PubMed]
- Chen H, Yang H, Zhu X, et al. m(5)C modification of mRNA serves a DNA damage code to promote homologous recombination. Nat Commun 2020;11:2834. [Crossref] [PubMed]
- Trixl L, Lusser A. The dynamic RNA modification 5-methylcytosine and its emerging role as an epitranscriptomic mark. Wiley Interdiscip Rev RNA 2019;10:e1510 [Crossref] [PubMed]
- Sun Z, Xue S, Zhang M, et al. Aberrant NSUN2-mediated m(5)C modification of H19 lncRNA is associated with poor differentiation of hepatocellular carcinoma. Oncogene 2020;39:6906-19. [Crossref] [PubMed]
- Fish GD, Stanley JH, Miller KS, et al. Postbiopsy pneumothorax: estimating the risk by chest radiography and pulmonary function tests. AJR Am J Roentgenol 1988;150:71-4. [Crossref] [PubMed]
- Sun L, Liu WK, Du XW, et al. Large-scale transcriptome analysis identified RNA methylation regulators as novel prognostic signatures for lung adenocarcinoma. Ann Transl Med 2020;8:751. [Crossref] [PubMed]
- He Y, Yu X, Li J, et al. Role of m(5)C-related regulatory genes in the diagnosis and prognosis of hepatocellular carcinoma. Am J Transl Res 2020;12:912-22. [PubMed]
- Wang P, Wu M, Tu Z, et al. Identification of RNA: 5-Methylcytosine Methyltransferases-Related Signature for Predicting Prognosis in Glioma. Front Oncol 2020;10:1119. [Crossref] [PubMed]
- McHam ML, Fulton A. Albinism. Int Ophthalmol Clin. 1992;32:185-200. [Crossref] [PubMed]
- Xue M, Shi Q, Zheng L, et al. Gene signatures of m5C regulators may predict prognoses of patients with head and neck squamous cell carcinoma. Am J Transl Res 2020;12:6841-52. [PubMed]
- Saijo Y, Sato G, Usui K, et al. Expression of nucleolar protein p120 predicts poor prognosis in patients with stage I lung adenocarcinoma. Ann Oncol 2001;12:1121-5. [Crossref] [PubMed]
- Sato G, Saijo Y, Uchiyama B, Kumano N, Sugawara S, Fujimura S, et al. Prognostic value of nucleolar protein p120 in patients with resected lung adenocarcinoma. J Clin Oncol 1999;17:2721-7. [Crossref] [PubMed]
- Uchiyama B, Saijo Y, Kumano N, et al. Expression of nucleolar protein p120 in human lung cancer: difference in histological types as a marker for proliferation. Clin Cancer Res 1997;3:1873-7. [PubMed]
- Guo G, Wang H, Shi X. Yet al. Disease Activity-Associated Alteration of mRNA m(5) C Methylation in CD4(+) T Cells of Systemic Lupus Erythematosus. Front Cell Dev Biol 2020;8:430. [Crossref] [PubMed]
- Zhang B, Wu Q, Li B, et al. m(6)A regulator-mediated methylation modification patterns and tumor microenvironment infiltration characterization in gastric cancer. Mol Cancer 2020;19:53. [Crossref] [PubMed]
- Li Y, Gu J, Xu F, et al. Molecular characterization, biological function, tumor microenvironment association and clinical significance of m6A regulators in lung adenocarcinoma. Brief Bioinform 2020; Epub ahead of print. [Crossref] [PubMed]
- Xu S, Tang L, Dai G, et al. Expression of m6A Regulators Correlated With Immune Microenvironment Predicts Therapeutic Efficacy and Prognosis in Gliomas. Front Cell Dev Biol 2020;8:594112 [Crossref] [PubMed]
- Xu F, Zhang Z, Yuan M, et al. M6A Regulatory Genes Play an Important Role in the Prognosis, Progression and Immune Microenvironment of Pancreatic Adenocarcinoma. Cancer Invest 2021;39:39-54. [Crossref] [PubMed]
- Lin S, Xu H, Zhang A, et al. Prognosis Analysis and Validation of m(6)A Signature and Tumor Immune Microenvironment in Glioma. Front Oncol 2020;10:541401 [Crossref] [PubMed]
- Fang J, Hu M, Sun Y, et al. Expression Profile Analysis of m6A RNA Methylation Regulators Indicates They Are Immune Signature Associated and Can Predict Survival in Kidney Renal Cell Carcinoma. DNA Cell Biol 2020; Epub ahead of print. [Crossref] [PubMed]
- Chen YT, Shen JY, Chen DP, et al. Identification of cross-talk between m(6)A and 5mC regulators associated with onco-immunogenic features and prognosis across 33 cancer types. J Hematol Oncol 2020;13:22. [Crossref] [PubMed]
- Han X, Liu H, Zhang Z, et al. Epitranscriptomic 5-Methylcytosine Profile in PM2.5-induced Mouse Pulmonary Fibrosis. Genomics Proteomics Bioinformatics 2020;18:41-51. [Crossref] [PubMed]