Risk-based prediction model for selecting eligible population for lung cancer screening among ever smokers in Korea
Introduction
Lung cancer is the most common incident cancer and the most common cause of cancer-related death worldwide, with 2.1 million incident cases and 1.8 million deaths in 2018 (1). To reduce the morbidity and mortality from lung cancer, annual lung cancer screening using low-dose computed tomography (LDCT) is recommended for high-risk smokers (2-5), largely based on the results from the National Lung Screening Trial (NLST) (6) and simulation studies (7).
Current recommendations for lung cancer screening are based on cumulative smoking exposure using pack-years, quit year, and age. However, previous studies have continuously suggested that selecting a target population for lung cancer screening according to individual risk models, which are based not only on age and smoking history but also on other risk factors, could be more effective (8-10). A recent guideline for clinical practice suggests the use of risk model-based recommendation for smokers with younger age or less cumulative smoking exposure. Further, the performance of selecting eligible population for lung cancer screening based on individual risk by prediction models compared to the NLST or USPSTF criteria within a specific population has also been evaluated (11-13).
In Korea, lung cancer is the third most common cancer with 28,628 new cases and is the most common cause of cancer-related deaths in 2018. The crude incidence and mortality rate of lung cancer was 55.8 and 34.8 per 100,000 person-years, respectively (14). After assessing the feasibility (15), the national lung cancer screening program has been provided to current or past smokers aged 50–74 years who quit smoking within 15 years with ≥30 pack-years screened using biannual LDCT, which has similar eligibility criteria to that of the NLST since 2019 in Korea. However, risk model-based eligible populations for lung cancer screening have rarely been evaluated in Asia. Park et al. developed a lung cancer risk model in Korean men including non-smokers (16). A Japanese study showed that family history of lung cancer and a sex-based risk model identified more lung cancer risk groups than the NLST criteria (17). However, considering that lung cancer screening has no beneficial effect on never-smokers (18,19), selecting the eligible population for LDCT screening based on individual risk should be considered mainly for smokers.
This study evaluated the performance of available lung cancer risk models and developed a new model including both smoking information and other demographic factors for smokers in the Korean population. Additionally, we compared the efficiency of model-based selection with the eligibility criteria of the NLST or Korean national screening program. We present the following article in accordance with the STROBE reporting checklist (available at https://dx.doi.org/10.21037/tlcr-21-566).
Methods
Study population and follow-up
The Korean National Health Insurance Service (NHIS) provides biennial general health screening for individuals aged ≥40 years. All individuals aged 40–79 years who underwent NHIS health screening in 2007 and 2008 were included. During the health screening, participants were instructed to fill out a set of questionnaires about tobacco smoking, alcohol consumption, physical activity, medical history, and family history of cancer. Body mass index (BMI) was calculated using the measured height and weight by trained nurses. Medical history of chronic obstructive pulmonary disease, emphysema, pneumoconiosis, and interstitial pulmonary disease was obtained from health insurance claims data in the NHIS. Based on the questionnaires, ever-smokers were identified, and information on the number of cigarettes smoked per day, timing of smoking, total years of smoking in lifetime, and years since quitting smoking (for former smokers) was obtained.
Lung cancer incidence was identified based on the participants’ records with the Korea Central Cancer Registry (KCCR) database until 2014. The KCCR covers approximately ≥96% of all newly diagnosed cancers annually in Korea (20). Lung cancer cases were classified using the International Classification of Diseases (ICD) 10th revision, codes C33 and C34. Individuals’ vital status was identified from the death certification from the Korean Statistics Office.
Among the 6,811,893 people who received health examinations from the NHIS between 2007 and 2008, those with missing information on smoking history (N=69,427), those with lung cancer before the date of health examination (N=45,952), and those aged ≥80 years (N=54,091) were excluded. Based on information on smoking history, never-smokers were excluded and participants who had missing information on any of the variables considered possible predictors were excluded. The proportion of missing information was highest for BMI (31.8% for all smokers), alcohol drinking (3.2%), and exercise (0.5%) (duplicates possible). The other variables did not contain missing information. Finally, 969,351 ever-smokers with all available predictors were included in the analysis (Figure 1).
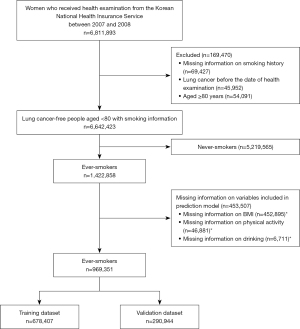
The study was conducted in accordance with the Declaration of Helsinki (as revised in 2013). The study was approved by the Institutional Review Board (IRB) of the National Cancer Center, Korea (IRB No. NCC20160278). Deidentified linkage data of the NHIS and KCCR were obtained, and the requirement for informed consent was waived for this study with permission from the IRB of the National Cancer Center.
Application of previous lung cancer risk models
Risk models for lung cancer incidence that have been developed and applied in the previous studies were considered. Among the nine models previously validated in the US population (11), models for lung cancer death (8,21) were not considered because in the data, information on the cause of death was not available. Subsequently, five models, including Bach (22), lung cancer risk models for screening (LCRAT) (8), the Prostate, Lung, Colorectal, and Ovarian Cancer Screening Trial Model 2012 (PLCOM2012), Pittsburgh, and Liverpool Lung Project models (LLPi) (10,23,24), were applied to the participants. Data on family history of lung cancer, asbestos exposure, and educational level were not available in the NHIS database because only family history of stomach, liver, colorectal, breast, cervix cancer, and other cancers was asked in the questionnaire. Therefore, we assumed that none of the participants had family history of lung cancer and all the participants had ‘some college’ level which was a reference for PLCOM2012 (10) despite the biased estimates (25), similar to a previous study (12). For asbestos exposure, all participants were assumed to be non-exposed. Considering that a previous study has observed that the potential number of individuals with family history of lung cancer was low and differences in educational level between lung cancer cases and controls were insignificant in Korean population (26), the bias could be minimal. Based on a sensitivity analysis, we considered participants with a family history of cancer as having family history of lung cancer for PLCOM2012 and as late-onset family history of lung cancer for the LLPi model. The description of three models has been previously reported (11,12). Race information included in PLCOM2012 was set to Asians. The performance of each model in the whole dataset was presented as discrimination [receiver operating characteristic curve and area under the curve (AUC)] and calibration [expected/observed (E/O) ratio].
Statistical analysis
To construct and validate the lung cancer risk model, we divided the participants (N=969,351) into the training dataset (70%, N=678,407) and the validation dataset (30%, N=290,992) by random sampling stratified by 5-year age group and sex. The lung cancer prediction model was constructed based on the Cox proportional hazards model. The follow-up time (person-years) was calculated from the date of the health examination to December 31, 2014, date of death, or date of lung cancer diagnosis, whichever came first.
We considered age, sex, cumulative smoking exposure (pack-years), smoking status in combination with years since cessation for past smokers, physical activity, alcohol consumption, BMI, family history of cancer, and medical history of chronic pulmonary obstructive disease, emphysema, pneumoconiosis, interstitial pulmonary disease, and cancer based on known risk factors of lung cancer (27) and available information in NHIS health examinations. First, we performed a univariate Cox proportional hazards model regression analysis and variables that showed a P value of <0.05 were selected. The proportional hazards assumption for each variable was assessed using a log-log survival plot. At this stage, family history of cancer and medical history of cancer were excluded. For selected variables, various cut-offs were applied and cut-offs with the lowest Akaike information criterion (AIC) were selected. Continuous variables, including age, units of pack-years, and years since cessation, raw, log-transformed, squared, square root, and various categories were applied, and those with the lowest AIC were included in the model. Then, with selected variables, multiple Cox proportional hazards model regression analysis was performed. Based on the beta coefficient from multiple Cox proportional hazards model and individual risk factors, the 6.6-year cumulative risk model of lung cancer incidence was estimated using the following equation, where P, s (t), and f (x) denote 6.6-year cumulative risk of lung cancer incidence, survival probability at the time t (6.6 years) if one had all risk factors at a mean value of 0.99622, and individual risks based on the beta coefficient of the Cox proportional hazards model as , respectively:
To assess the discrimination of the model regarding lung cancer development, Harrell’s concordance was quantified in both the training and validation datasets. The calibration of the model was evaluated in terms of E/O ratio in both datasets. Additionally, the discrimination and calibration were evaluated in subgroups of population by smoking status (current and past smokers) and cumulative amount of smoking (<10, ≥10, ≥20, and ≥30 pack-years).
The eligibility criteria of the NLST or Korean national screening program (age 50–74, ≥30 pack-years of smoking and <15 years since cessation) was applied to the training and validation datasets. We identified the number of people who met or did not meet the criterion. Based on the number of lung cancer incidence in each group, sensitivity, specificity, positive predictive value (PPV), and negative predictive value (NPV) were calculated. We selected a model-based lung cancer risk threshold at which the equal number of people screened using the NLST criteria was selected. Sensitivity, specificity, PPV, and NPV were calculated and compared with those of the NLST eligibility criteria. All analyses were performed using SAS version 9.4 (SAS Institute Inc., Cary, NC) and R statistics (R Foundation for Statistical Computing, Vienna, Austria).
Results
Characteristics of the study population
Table 1 summarizes the baseline characteristics of the study population, stratified by training and validation datasets. A total of 7,767 (1.14%) people developed lung cancer among the 678,407 people in the training dataset. In the validation dataset with 290,994 people, 3,368 (1.16%) people developed lung cancer. The mean and interquartile range of the follow-up times were 6.6 years and 6.2–7.1 years, respectively.
Table 1
Characteristics | Total (N=969,351) | Training dataset (N=678,407) | Validation dataset (N=290,994) |
---|---|---|---|
Age, years | |||
Mean (standard deviation) | 54.9 (9.3) | 54.9 (9.3) | 54.9 (9.3) |
40–49 | 323,722 (33.4%) | 226,492 (33.4%) | 97,230 (33.4%) |
50–59 | 350,747 (36.2%) | 245,545 (36.2%) | 105,202 (36.2%) |
60–69 | 195,808 (20.2%) | 137,092 (20.2%) | 58,716 (20.2%) |
70–79 | 99,074 (10.2%) | 69,278 (10.2%) | 29,796 (10.2%) |
Sex | |||
Male | 893,906 (92.2%) | 625,650 (92.2%) | 268,256 (92.2%) |
Female | 75,445 (7.8%) | 52,757 (7.8%) | 22,688 (7.8%) |
Smoking status | |||
Past | 364,768 (37.6%) | 255,331 (37.6%) | 109,487 (37.6%) |
Current | 604,583 (62.4%) | 423,076 (62.4%) | 181,507 (62.4%) |
Pack-year | |||
Mean (standard deviation) | 23.1 (15.2) | 23.1 (15.2) | 23.1 (15.2) |
<10 pack-years | 157,453 (16.2%) | 110,149 (16.2%) | 47,304 (16.3%) |
10–19.9 pack-years | 265,981 (27.4%) | 186,561 (27.5%) | 79,420 (27.3%) |
20–29.9 pack-years | 245,179 (25.3%) | 171,334 (25.3%) | 73,845 (25.4%) |
≥30 pack-years | 300,738 (31.0%) | 210,363 (31.0%) | 90,375 (31.1%) |
Years since cessation | |||
Current | 604,583 (62.4%) | 423,076 (62.4%) | 18,1507 (62.4%) |
<5 years | 111,446 (11.5%) | 77,983 (11.5%) | 33,463 (11.5%) |
<15 years | 163,007 (16.8%) | 114,229 (16.8%) | 48,778 (16.8%) |
≥15 years | 90,315 (9.3%) | 63,119 (9.3%) | 27,196 (9.4%) |
Number of days of alcohol consumption | |||
<5/week | 855,843 (88.3%) | 599,042 (88.3%) | 256,801 (88.3%) |
≥5/week | 113,508 (11.7%) | 79,365 (11.7%) | 34,143 (11.7%) |
Number of days of sweating exercise | |||
<3/week | 675,201 (69.7%) | 472,545 (69.7%) | 202,656 (69.7%) |
≥3/week | 294,150 (30.4%) | 205,862 (30.3%) | 88,288 (30.4%) |
Body mass index, kg/m2 | |||
Mean (standard deviation) | 24.0 (3.0) | 24.0 (2.9) | 24.0 (3.1) |
<20.0 | 23,317 (2.4%) | 16,258 (2.4%) | 7,059 (2.4%) |
20.0–24.9 | 595,045 (61.4%) | 416,797 (61.4%) | 178,248 (61.3%) |
≥25 | 350,989 (36.2%) | 245,352 (36.2%) | 105,637 (36.3%) |
Family history of cancer | |||
No | 746,127 (77.0%) | 522,416 (77.0%) | 223,711 (76.9%) |
Yes | 223,224 (23.0%) | 155,991 (23.0%) | 67,233 (23.1%) |
History of chronic pulmonary obstructive disease | |||
No | 948,895 (97.9%) | 664,079 (97.9%) | 284,816 (97.9%) |
Yes | 20,456 (2.1%) | 14,328 (2.1%) | 6,128 (2.1%) |
History of emphysema | |||
No | 966,894 (99.8%) | 676,738 (99.8%) | 290,156 (99.7%) |
Yes | 2,457 (0.3%) | 1,669 (0.3%) | 788 (0.3%) |
History of pneumoconiosis | |||
No | 968,848 (100%) | 678,045 (100%) | 290,803 (100%) |
Yes | 503 (0.1%) | 362 (0.1%) | 141 (0.1%) |
History of interstitial pulmonary disease | |||
No | 968,454 (99.9%) | 677,769 (99.9%) | 290,685 (99.9%) |
Yes | 897 (0.1%) | 638 (0.1%) | 259 (0.1%) |
History of cancer | |||
No | 961,814 (99.2%) | 673,163 (99.2%) | 288,651 (99.2%) |
Yes | 7,537 (0.8%) | 5,244 (0.8%) | 2,293 (0.8%) |
N, number.
Comparison of the performance of lung cancer risk models in the Korean population
Table 2 summarizes the discrimination and calibration of Bach, LCRAT, PLCOM2012, Pittsburgh, and LLPi models in Korean smokers. When these five models were applied to Korean population, the range of AUCs was 0.661–0.811, and Bach showed lowest discrimination and LCRAT showed better discrimination than other models. Regardless of imputation of family history of lung cancer as none (none of the study participants had a family history of lung cancer) or family history of any cancer (those with a family history of any cancer was considered having a family history of lung cancer) for the PLCOM2012 or LLPi models, the AUC was comparable. Even for the PLCOM2012 model, when the family history of any cancer was treated as none, the increment of AUC compared with the imputation with family history of any cancer was observed. Regarding calibration, all models overestimated the risk in Koreans, with an E/O ratio of 1.10–4.73. Specifically, the LCRAT and LLPi models overestimated the lung cancer risk.
Table 2
Model | Area under the curve | Expected/observed ratio |
---|---|---|
Bach | 0.661 (0.598–0.665) | 2.23 (2.07–2.40) |
LCRAT | 0.811 (0.807–0.814) | 4.73 (4.50–4.96) |
PLCOM2012 2013† | 0.772 (0.768–0.777) | 1.24 (1.22–1.26) |
Simplified PLCOM2012 2013‡ | 0.781 (0.776–0.785) | 1.10 (1.08–1.16) |
Pittsburgh 2015 | 0.781 (0.778–0.784) | 1.21 (1.19–1.23) |
LLPi 2015§ | 0.803 (0.800–0.806) | 3.25 (3.20–3.31) |
Simplified LLPi 2015¶ | 0.803 (0.800–0.806) | 3.21 (3.16–3.26) |
Korean model | 0.816 (0.810–0.822) | 0.995 (0.973–1.017) |
†, the race was assumed to be Asian. The educational level was assumed to be some college. A family history of lung cancer was imputed as a family history of any cancer. ‡, the race was assumed to be Asian. The educational level was assumed to be some college. A family history of lung cancer was imputed as none. §, family history of lung cancer was imputed as a family history of cancer. All participants with a family history of any cancer were assumed to have a late onset. ¶, family history of lung cancer was imputed as none. LCRAT, Lung Cancer Risk Models for Screening; PLCOM2012, the Prostate, Lung, Colorectal, and Ovarian Cancer Screening Trial Model 2012; LLPi, Liverpool Lung Project models.
Lung cancer risk prediction model in the Korean population
In univariate and multivariate analyses, age, sex, pack-years of smoking, smoking status and years since cessation, physical activity, alcohol consumption, BMI, and medical history of chronic pulmonary obstructive disease, emphysema, pneumoconiosis, and interstitial pulmonary disease were associated with lung cancer. Considering model fitting based on the AIC in univariate analysis for continuous variables, age-mean of age, square of age-mean of age, and square root of pack-years smoking were applied in the multivariate analysis. Information on smoking status and years since cessation were categorized as current smokers, <5 years, 5–14.9 years, and ≥15 years since cessation.
Table 3 presents the variables, their hazard ratios (HRs), 95% confidence intervals (CIs), and beta values included in the final model for lung cancer risk in the Korean population. History of interstitial pulmonary disease (HR 4.424, 95% CI: 3.475–5.633) was the most significant predictor of lung cancer, followed by smoking status (HR of current smokers 2.824, 95% CI: 2.543–3.137) and history of pneumoconiosis (HR 2.05, 95% CI: 1.335–3.149). The HR of the square root of pack-years was 1.191 (95% CI: 1.174–1.208). The equation of lung cancer risk estimation for individuals in terms of HR and the 6.6-year risk is described concisely in Appendix 1.
Table 3
Variables | Beta | HR (95% CI) |
---|---|---|
Age | ||
Age-mean | 0.14618 | 1.157 (1.150–1.164) |
(Age-mean)2 | −0.00242 | 0.998 (0.997–0.998) |
Sex | ||
Male | 0 | 1 (ref) |
Female | −0.38713 | 0.679 (0.611–0.754) |
Pack-year | ||
Square root | 0.17456 | 1.191 (1.174–1.208) |
Smoking status and years since cessation in past smokers | ||
Current smokers | 1.03818 | 2.824 (2.543–3.137) |
<5 years | 0.62246 | 1.864 (1.644–2.112) |
5–14.9 years | 0.34404 | 1.411 (1.248–1.595) |
≥15 years | 0 | 1 (ref) |
Physical activity | ||
<3/week | 0 | 1 (ref) |
≥3/week | −0.06768 | 0.935 (0.889–0.983) |
Number of days of alcohol consumption | ||
<5/week | 0 | 1 (ref) |
≥5/week | 0.05952 | 1.061 (1.001–1.125) |
Body mass index | ||
<18.5 kg/m2 | 0.26841 | 1.308 (1.189–1.439) |
18.5–24.9 kg/m2 | 0 | 1 (ref) |
≥25 kg/m2 | −0.24695 | 0.781 (0.741–0.824) |
History of chronic pulmonary obstructive disease | ||
No | 0 | 1 (ref) |
Yes | 0.36037 | 1.434 (1.316–1.563) |
History of emphysema | ||
No | 0 | 1 (ref) |
Yes | 0.25687 | 1.293 (1.029–1.624) |
History of pneumoconiosis | ||
No | 0 | 1 (ref) |
Yes | 0.7179 | 2.05 (1.335–3.149) |
History of interstitial pulmonary disease | ||
No | 0 | 1 (ref) |
Yes | 1.48709 | 4.424 (3.475–5.633) |
Prediction performance of lung cancer risk prediction model in the Korean population
The discrimination and calibration of the estimated individual risks and 6.6-year lung cancer risk based on the model were evaluated in the training and validation datasets, stratified by smoking status and cumulative smoking exposure (Table 4). The Harrell’s C-index was 0.816 in both training and validation datasets, and the E/O ratios were 1.002 (95% CI: 0.979–1.024) and 0.989 (0.956–1.023), respectively. When divided by smoking status or total pack-years of smoking, the Harrell’s C-index was 0.753–0.816 according to the subgroups. The discrimination was better in past smokers [0.803 (95% CI: 0.783–0.823)] than current smokers [0.816 (95% CI: 0.804–0.828)] in the validation and training datasets. Regarding calibration, the lung cancer risk model overestimated the risk in past smokers [E/O ratio, 1.510 (95% CI: 1.444–1.580) and 1.504 (95% CI: 1.404–1.611) in the training and validation datasets, respectively] and underestimated the risk in current smokers [E/O ratio, 0.881 (95% CI: 0.858–0.904) and 0.824 (95% CI: 0.793–0.857) in the training and validation datasets, respectively]. As the pack-years of smoking increased, the prediction model showed relatively lower discrimination and tendency to underestimate the risk in both the training and validation datasets.
Table 4
Statistic | Value (95% CI) |
---|---|
Harrell’s C-index in the training dataset | |
Ever-smokers | 0.816 (0.810–0.822) |
Current smokers | 0.816 (0.808–0.824) |
Past smokers | 0.804 (0.790–0.818) |
Smokers with <10 pack-years | 0.787 (0.760–0.814) |
Smokers with 10–19.9 pack-years | 0.812 (0.800–0.818) |
Smokers with 20–29.9 pack-years | 0.826 (0.820–0.829) |
Smokers with ≥30 pack-years | 0.754 (0.746–0.762) |
E/O ratio in the training dataset | |
Ever-smokers | 1.002 (0.979–1.024) |
Current smokers | 0.881 (0.858–0.904) |
Past smokers | 1.510 (1.444–1.580) |
Smokers with <10 pack-years | 1.143 (1.043–1.252) |
Smokers with 10–19.9 pack-years | 0.919 (0.915-0.924) |
Smokers with 20–29.9 pack-years | 0.984 (0.979-0.989) |
Smokers with ≥30 pack-years | 0.958 (0.930–0.988) |
Harrell’s C-index in the validation dataset | |
Ever-smokers | 0.816 (0.806–0.826) |
Current smokers | 0.816 (0.804–0.828) |
Past smokers | 0.803 (0.783–0.823) |
Smokers with <10 pack-years | 0.797 (0.758–0.836) |
Smokers with 10–19.9 pack-years | 0.819 (0.801-0.829) |
Smokers with 20–29.9 pack-years | 0.823 (0.809-0.830) |
Smokers with ≥30 pack-years | 0.753 (0.739–0.767) |
E/O ratio in the validation dataset | |
Ever-smokers | 0.989 (0.956–1.023) |
Current smokers | 0.824 (0.793–0.857) |
Past smokers | 1.504 (1.404–1.611) |
Smokers with <10 pack-years | 1.072 (0.936–1.227) |
Smokers with 10–19.9 pack-years | 0.913 (0.906-0.919) |
Smokers with 20–29.9 pack-years | 1.036 (1.029-1.044) |
Smokers with ≥30 pack-years | 0.962 (0.919–1.007) |
E/O, expected/observed.
Comparison of eligible population of the NLST with lung cancer risk model-based populations
When the NLST criteria were applied to the study participants, approximately 17.5% smokers were eligible. For a comparable number of population based on lung cancer risk model, participants with a 6.6-year lung cancer risk >2.1% were eligible. When we applied the NLST criteria or 6.6-year lung cancer risk cut-off of >2.1% based on the model to the study population, the sensitivity, specificity, and PPV were 44.4% versus 60.6, 82.8% versus 83.1%, and 2.9% versus 4.0% in the training dataset and 44.3% versus 60.1%, 82.8% versus 83.0%, and 2.9% versus 4.0% in the validation dataset, respectively (Table 5). When we applied the model-based cut-off risk of >2.1% instead of the NLST criteria, 73.5% of the participants remained ineligible, 8.4% remained eligible, and 18.1% changed eligibility statuses. For individuals who changed from ineligible using the NLST criteria to eligible using the model-based cut-off, 3.7% developed lung cancer within 6.6 years. For individuals who changed from eligible to ineligible, only 1.6% developed lung cancer. Individuals who changed from ineligible to eligible were older, predominantly women, and had more underlying pulmonary diseases (Table 5). The median ages of selected people through the NLST criteria or 6.6-year lung cancer risk cut-off of >2.1% were 63.1 and 69.6 in the training and validation dataset, respectively. The relative risk of developing lung cancer in participants who met the criteria compared to those who did not meet the criteria was 3.77 based on the NLST, which increased to 8.53 based on the model-based risk group.
Table 5
Criteria | Lung cancer development | Without lung cancer development | Predictive value |
---|---|---|---|
Lung cancer screening criteria in the training dataset | |||
Positive | 3,448 | 115,197 | PPV: 2.9 (2.8–3.0) |
Negative | 4,319 | 555,443 | NPV: 99.2 (99.2–99.3) |
Sensitivity | 44.4 (95% CI: 43.2–45.5) | ||
Specificity | 82.8 (95% CI: 82.7–82.9) | ||
Lung cancer prediction model in the training dataset | |||
Positive | 4,708 | 113,402 | PPV: 4.0 (3.9–4.1) |
Negative | 3,059 | 557,238 | NPV: 99.5 (99.4–99.5) |
Sensitivity | 60.6 (95% CI: 59.5–61.7) | ||
Specificity | 83.1 (95% CI: 83.0–83.2) | ||
Lung cancer screening criteria in the validation dataset | |||
Positive | 1,491 | 49,328 | PPV: 2.9 (2.8–3.1) |
Negative | 1,877 | 238,248 | NPV: 99.2 (99.2–99.3) |
Sensitivity | 44.3 (95% CI: 42.6–46.0) | ||
Specificity | 82.8 (95% CI: 82.7–83.0) | ||
Lung cancer prediction model in the validation dataset | |||
Positive | 2,053 | 48,695 | PPV: 4.0 (3.9–4.2) |
Negative | 1,315 | 238,881 | NPV: 99.5 (99.4–99.5) |
Sensitivity | 60.1 (95% CI: 59.2–62.6) | ||
Specificity | 83.0 (95% CI: 82.9–83.2) |
PPV, positive predictive value; NPV, negative predictive value; CI, confidence interval.
Based on the lung cancer risk model to identify 90% of people who would develop lung cancer within 6.6-years, 47.0% smokers would have to be screened (cut-off, 0.054%), and the corresponding specificity and PPV were 53.4% and 2.2%, respectively.
Discussion
To assess the efficiency of lung cancer risk model-based selection of eligible population for LDCT screening, we compared the performance of a new lung cancer risk model for the Korean population based on a large-scale health screening cohort with three risk models developed for European and US populations. Additionally, a new lung cancer risk model for the Korean population was validated and compared with the current eligibility criteria of the NLST. The lung cancer risk model-based selection criteria possibly include more high-risk ever-smokers than the eligibility criteria of the NLST.
When models developed for ever-smokers in the Western population were applied to the Korean population, they moderately discriminated people who would develop and those who would not develop lung cancer (AUC, 0.66–0.81). Regarding the calibration of models developed for the Western population in the Korean population, the E/O ratio was >1.00. The higher lung cancer incidence rate (1), higher average smoking amount, earlier starting age of smoking, and significantly greater effect of smoking on lung cancer in the Western population than in the Asian population (28) would be the cause of overestimation and moderate discrimination. Therefore, developing models for the Asian population that reflect the effects of smoking, other relevant risk factors, and lung cancer incidence in Asian countries is necessary. The new risk model developed for the Korean population in this study showed better calibration and discrimination than models developed for the Western population. However, despite the strong association between the family history of lung cancer, age at onset of lung cancer in family members, and individual lung cancer risk (29) and its prediction power (8,10,23,30), it could not be included because of insufficient information.
In this study, occupational exposures were not considered risk factors for the Korean lung cancer model despite their strong causal association with lung cancer (31) considering the insufficient information in the questionnaire. However, measurement of occupational exposure was complex, based on detailed job information and the duration of employment (22,30). A simple measurement based on the questionnaire about experience of occupational exposure did not show significant association in the PLCOM2012 (9). Thus, individual risk assessment based on the occupational exposure may be less applicable when selecting high-risk group for ever-smokers in the general population.
A few lung cancer risk models have been developed in the Asian population (16,32-35). This model is different from the previously developed models in Asian countries. First, previous Asian models targeted both ever-smokers and non-smokers (16,32-35), but this model targeted only ever-smokers, considering the significantly low lung cancer risk among never-smokers to require LDCT screening (18,19). When the PLCOM2012 model was applied to never-smokers, none of them reached a threshold risk (≥0.0151), concluding that LDCT screening should not be offered to never-smokers (18). Second, previous Asian models incorporated various biomarkers (16,32,33,35). Considering the cost of the biomarker measurement, these models would be useful for high-risk non-smoker group such as those who were exposed to occupational exposure or those with family history of lung cancer.
The risk model showed good discrimination and calibration in the training and validation dataset overall. When divided by smoking history, the discrimination of the model was relatively lower in smokers with higher cumulative smoking exposure, which underestimated the risk. Otherwise, overestimation of risk was observed in past smokers or smokers with lower cumulative smoking. If this was caused by model fitting, there were underestimated risk prediction in low-risk populations and overestimated risk prediction in high-risk groups (1,4,36,37). However, based on the direction of over- and underestimation of the model, it was caused by the characteristics of the sub-population (current smokers and past smokers). These results are consistent with those of a previous model validation study (3).
The key observation of this study is that Korean lung cancer model-based selection of high-risk population would identify more lung cancers than the eligibility criteria of the NLST. With comparable number of screened population, improvements in sensitivity, specificity, PPV, and NPV were achieved. Thus, model-based selection could identify more population of 10–29.9 pack-years of smoking with underlying pulmonary diseases associated with increased lung cancer risk. The NLST eligibility criteria use dichotomized criteria in combination with age (55–74 years or not), cumulative pack-years (≥30 or <30 pack-years), and quit year (<15 or ≥15 years) (38). When the USPSTF criteria were applied to other study populations, approximately 20–38.0% participants (6,11) were eligible for LDCT screening. In this study population, 17.5% and 18.3% of ever-smokers met the NLST and USPSTF criteria, respectively. In a previous study, approximately 6.9% Korean representative population aged ≥40 years met the NLST criteria (39). In another study, approximately 20% Korean representative population aged 55–74 years met the NLST criteria (40). However, these studies included both never-smokers and smokers (39,40). Considering that 43.9% people aged ≥40 years were ever-smokers (39), the proportion of smokers who met the NLST criteria in Korean representative population would be approximately 15–16%. A study conducted in Korea including both never-smokers and smokers demonstrated that the NLST criteria is useful to identify high-risk population for lung cancer screening based on the comparison of cancer incidence ratio of 5.78 between individuals who met and those who did not meet the criteria (39). In our study population, the ratio was 8.53 when model-based selection was applied. If one-third of the population was selected similarly as a previous study (10), 83.4% lung cancer among ever-smokers could be identified, suggesting the clinical utility of LDCT screening based on lung cancer risk model.
To the best of our knowledge, this is the first study to evaluate lung cancer risk models for the Western population in the Asian population. Further, we developed a new model including the most representative and largest study population was developed for Asian ever-smokers. However, this study has some limitations. First, the study population comprised health examinees in 2007–2008, and their characteristics may not be comparable with non-examinees or recent examinees. In addition, we used the complete dataset to develop and validate the model. A large proportion of data (around 34%) mostly due to missing values in BMI (31.8%) were not included in the analysis. Although we tried multiple imputation using the MICE package of R statistics, the results suggested an over-fitted model (1,4) and the assumption of multiple imputation could not be identified (7,41). Therefore, we only showed the training and validation results based on the complete dataset. Second, the dataset for model construction and validation were extracted from the same data source. Validation of the model in a different population, such as people who did not undergo NHIS health examination, would increase the external validity of the model. However, when the model was applied to select high-risk populations based on questionnaires and measurements during health examinations, training and validation among people who received health examinations was more appropriate for application in the real world. Despite NHIS health examination being free, people who received health examination had higher socioeconomic status and were more predominantly non-smokers. Additionally, the participants could not represent smoking exposure in the current Korean population because the current study included the target health screening participants from 2006 to 2007 and the smoking rate had been decreased in Korea since that time. Third, we evaluated the previously developed models, but due to the unavailable information included in the previously developed models, only five models could be evaluated and models developed in Asian countries could not be evaluated. In addition, for the unavailable variables in our data, we considered them as a single value. It could cause biased estimates (28) and overall over- or under-estimation of the risk.
Conclusions
Selecting an eligible population for lung cancer screening could be improved with the application of risk models. Hence, more cancers can be detected than NLST or the USPSTF criteria. When implemented in the population level, consensus on the risk threshold considering cost-effectiveness, balanced information including benefits and harms of risk-based screening, shared decision-making is required. Korea started the national lung cancer screening program based on the eligibility criteria of NLST in 2019. It could be expected that a combination of lung cancer prediction models tailored for the Korean population and a national lung cancer screening program would provide a more efficient nationwide screening program. Further research on the cost-effectiveness of the model-based and current criteria of the national lung cancer screening program in the Korean population is needed.
Acknowledgments
Funding: This study was supported by grants (No. 1631000) from the National R&D Program for Cancer Control, Ministry of Health and Welfare, Republic of Korea and national cancer center grant (No. 1610430), National Cancer Center, Republic of Korea.
Footnote
Reporting Checklist: The authors have completed the STROBE reporting checklist. Available at https://dx.doi.org/10.21037/tlcr-21-566
Data Sharing Statement: Available at https://dx.doi.org/10.21037/tlcr-21-566
Peer Review File: Available at https://dx.doi.org/10.21037/tlcr-21-566
Conflicts of Interest: All authors have completed the ICMJE uniform disclosure form (available at https://dx.doi.org/10.21037/tlcr-21-566). The authors have no conflicts of interest to declare.
Ethical Statement: The authors are accountable for all aspects of the work in ensuring that questions related to the accuracy or integrity of any part of the work are appropriately investigated and resolved. The study was conducted in accordance with the Declaration of Helsinki (as revised in 2013). The study was approved by the Institutional Review Board (IRB) of the National Cancer Center, Korea (IRB No. NCC20160278). Deidentified linkage data of the NHIS and KCCR were obtained, and the requirement for informed consent was waived for this study with permission from the IRB of the National Cancer Center.
Open Access Statement: This is an Open Access article distributed in accordance with the Creative Commons Attribution-NonCommercial-NoDerivs 4.0 International License (CC BY-NC-ND 4.0), which permits the non-commercial replication and distribution of the article with the strict proviso that no changes or edits are made and the original work is properly cited (including links to both the formal publication through the relevant DOI and the license). See: https://creativecommons.org/licenses/by-nc-nd/4.0/.
References
- Bray F, Ferlay J, Soerjomataram I, et al. Global cancer statistics 2018: GLOBOCAN estimates of incidence and mortality worldwide for 36 cancers in 185 countries. CA Cancer J Clin 2018;68:394-424. [Crossref] [PubMed]
- Moyer VAU.S. Preventive Services Task Force. Screening for lung cancer: U.S. Preventive Services Task Force recommendation statement. Ann Intern Med 2014;160:330-8. [Crossref] [PubMed]
- Wender R, Fontham ET, Barrera E Jr, et al. American Cancer Society lung cancer screening guidelines. CA Cancer J Clin 2013;63:107-17. [Crossref] [PubMed]
- Wood DE, Kazerooni E, Baum SL, et al. Lung cancer screening, version 1.2015: featured updates to the NCCN guidelines. J Natl Compr Canc Netw 2015;13:23-34; quiz 34. [Crossref] [PubMed]
- Detterbeck FC, Mazzone PJ, Naidich DP, et al. Screening for lung cancer: Diagnosis and management of lung cancer, 3rd ed: American College of Chest Physicians evidence-based clinical practice guidelines. Chest 2013;143:e78S-92S.
- National Lung Screening Trial Research Team. Reduced lung-cancer mortality with low-dose computed tomographic screening. N Engl J Med 2011;365:395-409. [Crossref] [PubMed]
- de Koning HJ, Meza R, Plevritis SK, et al. Benefits and harms of computed tomography lung cancer screening strategies: a comparative modeling study for the U.S. Preventive Services Task Force. Ann Intern Med 2014;160:311-20. [Crossref] [PubMed]
- Katki HA, Kovalchik SA, Berg CD, et al. Development and Validation of Risk Models to Select Ever-Smokers for CT Lung Cancer Screening. JAMA 2016;315:2300-11. [Crossref] [PubMed]
- Tammemägi MC. Application of risk prediction models to lung cancer screening: a review. J Thorac Imaging 2015;30:88-100. [Crossref] [PubMed]
- Tammemägi MC, Katki HA, Hocking WG, et al. Selection criteria for lung-cancer screening. N Engl J Med 2013;368:728-36. [Crossref] [PubMed]
- Katki HA, Kovalchik SA, Petito LC, et al. Implications of Nine Risk Prediction Models for Selecting Ever-Smokers for Computed Tomography Lung Cancer Screening. Ann Intern Med 2018;169:10-9. [Crossref] [PubMed]
- Ten Haaf K, Jeon J, Tammemägi MC, et al. Risk prediction models for selection of lung cancer screening candidates: A retrospective validation study. PLoS Med 2017;14:e1002277. [Crossref] [PubMed]
- Li K, Hüsing A, Sookthai D, et al. Selecting High-Risk Individuals for Lung Cancer Screening: A Prospective Evaluation of Existing Risk Models and Eligibility Criteria in the German EPIC Cohort. Cancer Prev Res (Phila) 2015;8:777-85. [Crossref] [PubMed]
- Hong S, Won YJ, Lee JJ, et al. Cancer Statistics in Korea: Incidence, Mortality, Survival, and Prevalence in 2018. Cancer Res Treat 2021;53:301-15. [Crossref] [PubMed]
- Lee J, Lim J, Kim Y, et al. Development of Protocol for Korean Lung Cancer Screening Project (K-LUCAS) to Evaluate Effectiveness and Feasibility to Implement National Cancer Screening Program. Cancer Res Treat 2019;51:1285-94. [Crossref] [PubMed]
- Park S, Nam BH, Yang HR, et al. Individualized risk prediction model for lung cancer in Korean men. PLoS One 2013;8:e54823. [Crossref] [PubMed]
- Wu FZ, Huang YL, Wu CC, et al. Assessment of Selection Criteria for Low-Dose Lung Screening CT Among Asian Ethnic Groups in Taiwan: From Mass Screening to Specific Risk-Based Screening for Non-Smoker Lung Cancer. Clin Lung Cancer 2016;17:e45-56. [Crossref] [PubMed]
- Tammemägi MC, Church TR, Hocking WG, et al. Evaluation of the lung cancer risks at which to screen ever- and never-smokers: screening rules applied to the PLCO and NLST cohorts. PLoS Med 2014;11:e1001764. [Crossref] [PubMed]
- Ten Haaf K, de Koning HJ. Should Never-Smokers at Increased Risk for Lung Cancer Be Screened? J Thorac Oncol 2015;10:1285-91. [Crossref] [PubMed]
- McCormack VA, dos Santos Silva I. Breast density and parenchymal patterns as markers of breast cancer risk: a meta-analysis. Cancer Epidemiol Biomarkers Prev 2006;15:1159-69. [Crossref] [PubMed]
- Kovalchik SA, Tammemagi M, Berg CD, et al. Targeting of low-dose CT screening according to the risk of lung-cancer death. N Engl J Med 2013;369:245-54. [Crossref] [PubMed]
- Bach PB, Kattan MW, Thornquist MD, et al. Variations in lung cancer risk among smokers. J Natl Cancer Inst 2003;95:470-8. [Crossref] [PubMed]
- Marcus MW, Chen Y, Raji OY, et al. LLPi: Liverpool Lung Project Risk Prediction Model for Lung Cancer Incidence. Cancer Prev Res (Phila) 2015;8:570-5. [Crossref] [PubMed]
- Wilson DO, Weissfeld J. A simple model for predicting lung cancer occurrence in a lung cancer screening program: The Pittsburgh Predictor. Lung Cancer 2015;89:31-7. [Crossref] [PubMed]
- Gorelick MH. Bias arising from missing data in predictive models. J Clin Epidemiol 2006;59:1115-23. [Crossref] [PubMed]
- Lamichhane DK, Kim HC, Choi CM, et al. Lung Cancer Risk and Residential Exposure to Air Pollution: A Korean Population-Based Case-Control Study. Yonsei Med J 2017;58:1111-8. [Crossref] [PubMed]
- Malhotra J, Malvezzi M, Negri E, et al. Risk factors for lung cancer worldwide. Eur Respir J 2016;48:889-902. [Crossref] [PubMed]
- Jung KJ, Jeon C, Jee SH. The effect of smoking on lung cancer: ethnic differences and the smoking paradox. Epidemiol Health 2016;38:e2016060. [Crossref] [PubMed]
- Coté ML, Liu M, Bonassi S, et al. Increased risk of lung cancer in individuals with a family history of the disease: a pooled analysis from the International Lung Cancer Consortium. Eur J Cancer 2012;48:1957-68. [Crossref] [PubMed]
- Cassidy A, Myles JP, van Tongeren M, et al. The LLP risk model: an individual risk prediction model for lung cancer. Br J Cancer 2008;98:270-6. [Crossref] [PubMed]
- Spyratos D, Zarogoulidis P, Porpodis K, et al. Occupational exposure and lung cancer. J Thorac Dis 2013;5:S440-5. [PubMed]
- Wu X, Wen CP, Ye Y, et al. Personalized Risk Assessment in Never, Light, and Heavy Smokers in a prospective cohort in Taiwan. Sci Rep 2016;6:36482. [Crossref] [PubMed]
- Lyu Z, Li N, Chen S, et al. Risk prediction model for lung cancer incorporating metabolic markers: Development and internal validation in a Chinese population. Cancer Med 2020;9:3983-94. [Crossref] [PubMed]
- Charvat H, Sasazuki S, Shimazu T, et al. Development of a risk prediction model for lung cancer: The Japan Public Health Center-based Prospective Study. Cancer Sci 2018;109:854-62. [Crossref] [PubMed]
- Wang X, Ma K, Cui J, et al. An individual risk prediction model for lung cancer based on a study in a Chinese population. Tumori 2015;101:16-23. [Crossref] [PubMed]
- Van Calster B, McLernon DJ, van Smeden M, et al. Calibration: the Achilles heel of predictive analytics. BMC Med 2019;17:230. [Crossref] [PubMed]
- Van Calster B, Vickers AJ. Calibration of risk prediction models: impact on decision-analytic performance. Med Decis Making 2015;35:162-9. [Crossref] [PubMed]
- Royston P, Altman DG, Sauerbrei W. Dichotomizing continuous predictors in multiple regression: a bad idea. Stat Med 2006;25:127-41. [Crossref] [PubMed]
- Lee YJ, Choi SM, Lee J, et al. Utility of the National Lung Screening Trial Criteria for Estimation of Lung Cancer in the Korean Population. Cancer Res Treat 2018;50:950-5. [Crossref] [PubMed]
- Kim EY, Shim YS, Kim YS, et al. Adherence to general medical checkup and cancer screening guidelines according to self-reported smoking status: Korea National Health and Nutrition Examination Survey (KNHANES) 2010-2012. PLoS One 2019;14:e0224224. [Crossref] [PubMed]
- Jakobsen JC, Gluud C, Wetterslev J, et al. When and how should multiple imputation be used for handling missing data in randomised clinical trials - a practical guide with flowcharts. BMC Med Res Methodol 2017;17:162. [Crossref] [PubMed]