Real-time digital polymerase chain reaction (PCR) as a novel technology improves limit of detection for rare allele assays
Introduction
Due to the prevalent issue of temporal and spatial tumor heterogeneity for tissue biopsy, liquid biopsy is an important tool for companion diagnostics (CDx) to avoid false negative test results, especially for patients with multiple tumors and large tumors. Currently, liquid biopsy tests for CDx are not as sensitive as tissue biopsy. A previously published paper by Xu et al. found that out of 13 samples that were all determined positive by tissue biopsy, 4 were determined positive by Roche Diagnostics’ Cobas-ARMS polymerase chain reaction (PCR) kit and 7 were determined positive by Thermo Fisher Scientific’s QuantStudioTM 3D Digital PCR System (1). While liquid biopsy assays are needed for CDx, there are still limitations regarding this technology. This mindset is reflected in the guidelines of the National Comprehensive Cancer Network (NCCN) for the use of liquid biopsy as the parameters around its use has changed twice within the past few years. The initial change in 2019 recommended the use of liquid biopsy for non-small cell lung cancer (NSCLC) patients for epidermal growth factor receptor (EGFR) mutation detection only when tissue biopsy is not available (2). The second change only a year later states that liquid biopsy can be ordered first—positive patients go directly to targeted therapy, negative patients will get another test from a tissue biopsy, if possible (3). While these changes reflect growing confidence in liquid biopsy, tissue biopsy is still needed on negative samples due to the lack of sensitivity of current liquid biopsy tests (4,5). Overcoming poor sensitivity is going to give liquid biopsy the strength it needs to be comparable with tissue biopsy as a CDx test.
Different forms of PCR are used for liquid biopsy tests. Quantitative PCR (qPCR) is the most commonly used with two assays currently approved for clinical use for liquid biopsy by the FDA (6-9). However, there are deficits in sensitivity as seen with the study discussed above. Compared to qPCR, digital PCR (dPCR) offers an absolute quantitation method with improved sensitivity, accuracy, and reproducibility although at a more limited dynamic range at lower copy numbers. dPCR improves the sensitivity by exploiting limiting dilutions and Poisson statistical analysis to provide an absolute quantification method without the need of an external reference standard (10). The analytical precision, as measured by variance coefficient, is significantly better for dPCR as compared to qPCR (11-13).
Commercial dPCR platforms have primarily been based on endpoint PCR signal detection, where identification of positive partitions is determined strictly by an endpoint measurement of amplification signal. Chip-based dPCR is one form of dPCR (Thermo Fisher Scientific, Waltham, MA, USA). This technology partitions PCR reaction mixture into ~20,000 wells on a silicon chip resulting in 0 or 1 (or more) molecules of DNA or RNA in each well (14). After thermocycling is complete, if the target or reference molecule is present, the well will have positive fluorescence; the individual molecules/wells can then be counted (15,16). For the purposes of this paper, when mutant [fluorescein amidite (FAM) labeled] positive wells are discussed, they are referring to the actual number of positive wells present. Another popular form of endpoint dPCR is droplet digital PCR (ddPCR). Bio-Rad’s ddPCRTM technology (Bio-Rad, Hercules, CA, USA) partitions PCR reaction mixture into ~20,000 droplets in a single step, then PCR amplification occurs in each droplet (17). The droplets, similar to chip-based dPCR, would contain 0 or 1 (or more) molecules of DNA or RNA and can be individually counted.
Some may consider Fluidigm’s BiomarkTM platform (Fluidigm, San Francisco, CA, USA), which is designed to collect real-time amplification data in a highly partitioned microfluidics device, a real-time dPCR instrument. However, it is not a true dPCR instrument in comparison to the dPCR systems described above (18). Fluidigm’s platform only partitions samples into 770 compartments vs. 20 k individual partitions for endpoint dPCR systems, resulting in lower sensitivity due to limited number of partitions. Although sensitivity can be made comparable if a sample is run on 25 integrated fluidic circuits, each with 770 compartments, it would drive up the cost per sample by 25-fold (19).
We have successfully developed a real-time dPCR instrument, which continues to improve upon the sensitivity of endpoint dPCR by combining the absolute quantification of endpoint dPCR with the benefits of real-time amplification curve analysis of qPCR. Real-time dPCR eliminates a key limitation of PCR sensitivity, which is the random occurrence of positive PCR microreactions in negative samples due to non-specific PCR amplification and imaging issues (20). This is accomplished by using the real-time amplification curve data to identify and remove false positive datapoints based on their atypical amplification profiles (21). Since false positive datapoints are largely eliminated, the limit of detection (LoD) can be lowered. This leads to the reduction of false negative samples since true positive datapoints can be discerned at a lower LoD.
In different study, we have demonstrated improved sensitivity for viral detection. For SARS-CoV-2 LoD samples that were aged in −80 °C conditions for 2 months, real-time dPCR determined 86.36% of samples as positive while endpoint dPCR only determined 54.55% of samples as positive suggesting a significant improvement of sensitivity by real-time dPCR (21).
In this study, we focused on assays for cancer liquid biopsy. We compared the sensitivity and quantification accuracy of real-time dPCR and endpoint dPCR using contrived samples containing clinically important EGFR mutations: T790M, L858R, and exon 19 deletions, at different mutation allele frequencies (MAF). We also included an assay for human epidermal growth factor receptor 2 (HER2) amplification detection, which was used to determine if improvements to sensitivity and quantification accuracy are prevalent at high copy numbers. We expect that there will be improved sensitivity and quantification accuracy for real-time dPCR over the current endpoint dPCR at extremely low MAF. These results support the notion that the incorporation of real-time data with dPCR technology holds the potential to further improve the sensitivity and reduce false negative rates for cancer liquid biopsy assays. We present the following article in accordance with the MDAR reporting checklist (available at https://dx.doi.org/10.21037/tlcr-21-728).
Methods
DNA samples
Normal female human genomic DNA (gDNA) (catalog No. G1521) was purchased from Promega Corporation (Madison, WI, USA). All mutant cell lines were purchased from ResearchDX (Irvine, CA, USA). Genomic DNA from mutant cell lines for the four assays were as follows: (I) HER2 from mutant cell line SKBR3 gDNA, (II) T790M (NP_005219.2) and L858R (NP_005219.2) assays from mutant cell line NCIH1975 gDNA, and (III) 19del assay from mutant cell line HCC827. For each assay, the wildtype G1521 DNA was mixed with the mutant cell line gDNA in the indicated ratios based on QubitTM 4 Fluorometer (Thermo Fisher Scientific, Waltham, MA, USA; Cat. No. Q33238). Samples were stored at −20 °C.
dPCR Assays
The following four QuestGenomics assays were used in this study: (I) HER2 Amplification Detection Kit (dPCR) (QuestGenomics, Nanjing, China; Cat. No. Q0242110); (II) EGFR 19del Mutation Detection Kit (dPCR) (QuestGenomics; Cat. No. Q0242410); (III) EGFR T790M Mutation Detection Kit (dPCR) (QuestGenomics; Cat. No. Q0242310); (IV) EGFR L858R Mutation Detection Kit (dPCR) (QuestGenomics; Cat. No. Q0242210).
dPCR
All EGFR endpoint dPCR/real-time dPCR reactions were performed using 20ng DNA per sample. The HER2 assay used 5ng DNA per sample. All dPCR reaction volumes were 14.5 µL. Assays were run on dPCR chips (Thermo Fisher Scientific, Cat No. A26317) prepared following Thermo Fisher Scientific protocols. They were thermocycled on the Gnomegen Real-Time Digital PCR Instrument (Gnomegen LLC.; Cat No. INS1) which provides quantitative and qualitative detection of target nucleic acid sequences using real-time PCR analysis. PCR reactions were prepared using QuantStudioTM 3D Digital PCR master mix v2 (Thermo Fisher Scientific; Cat No. A26358). Cycling conditions for all assays were the following: preheat at 95 °C for 10 minutes followed by 39 cycles of 95 °C for 2 minutes and 60 °C for 30 seconds. Additional end point imaging was performed using the QuantStudioTM 3D Digital PCR Instrument (Thermo Fisher Scientific; Cat. No. 4489084) and analyzed using the QuantStudioTM 3D Analysis Suite Cloud Software (version 3.1.4-PRC-build1).
Data collection
During thermocycling in the Gnomegen Real-Time Digital PCR Instrument (Gnomegen LLC.; Cat. No. INS1), the chips were imaged every five cycles starting at cycle 5 after the extension step as well as imaging at the endpoint. After the thermocycling finished in the Gnomegen Real-Time Digital PCR Instrument, the chips were removed. Then endpoint images were taken in the QuantStudioTM 3D Digital PCR Instrument (Thermo Fisher Scientific; Cat. No. 4489084) following manufacturer’s protocol.
Sample batching
Samples were processed for each assay type in batches over multiple days. For the HER2 samples, each of the four different wildtype:mutant ratios (1.00, 1.09, 1.18, 1.30) were run in duplicate (8 chips per batch) across 7 batches (56 chips total). For the T790M samples, each of the four different wildtype:mutant ratios (0.00%, 0.03%, 0.10%, 1.00%) were run in duplicate (8 chips per batch) across 7 batches (56 chips total). For the L858R samples, each of the four different wildtype:mutant ratios (0.00%, 0.06%, 0.20%, 2.00%) was run in duplicate (8 chips per batch) across 6 batches (48 chips total). For the EGFR 19del samples, each of the four different wildtype:mutant ratios (0.00%, 0.06%, 0.20, 2.00%) was run in duplicate (8 chips per batch) across 7 batches (56 chips total). Additional chips at 0.00% and 0.06% were run for EGFR 19del samples to determine the LoD.
Statistical analysis
Data was organized and graphed using JMP®, Version 14 (SAS Institute Inc., Cary, NC, USA, 1989–2019). Each unique chip/array that was shared across endpoint dPCR and Gnomegen Real-Time Digital PCR Instrument per technical replication and concentration was described as an “experiment”. Real-time data was analyzed using a previously published algorithm (21). The average number of partitions for each chip were between 16,000 and 19,000 wells. The number of copies per partition was determined via the Poisson adjustment. Individual false positive datapoints within each dPCR chip were removed using the amplification curve generated by the Gnomegen Real-Time Digital PCR Instrument. Percent recovery was used as a measure of accuracy for the mutation detection assays, EGFR 19del, T790M, and L858R.
Percent recovery was used to measure accuracy for the amplification detection assay, HER2, with the ratio indicated as the ratio between the wildtype and mutant genes.
The mean percent ratio for both assay types was determined using the percent recovery equation with the mean observed values. Different plots were created and Wilcoxon rank test for matched pairs was performed to compare the distribution of paired data of endpoint and real-time dPCR for all concentrations measured. Then the data was exported in .csv format to be used in R. R functions from Vynck et al. (2016) was utilized to calculate generalized linear mixed model (GLMM)-based estimates for mean ±95% confidence intervals of relative expression estimates for technical replicates of dPCR reactions (22). RStudio (version 1.2.5042, RStudio Team 2020) with R 3.6.3 (R Core Team, 2014) was used for this part of the analysis. Datasets for this study are available upon request. We have also followed the recommendations for the Minimum Information for Publications of Quantitative Digital PCR Experiments (online table: https://cdn.amegroups.cn/static/public/tlcr-21-728-1.pdf).
Results
Experimental design
In this study, chips loaded with PCR reaction mix were first thermocycled on the Gnomegen Real-Time Digital PCR Instrument. During the thermocycling process, chips were imaged every 5 cycles to generate real-time data. Once thermocycling was completed, the chips were then read in Thermo Fisher Scientific’s QuantStudioTM 3D Digital PCR Instrument to generate endpoint data. By reading the same chip on the two instruments, any differences in results are due to the different endpoint vs. real-time analysis of each microreaction and not confounding variables such as differences in amplification, different chips, or potential variations in input amount of DNA. This allows for the goal of this study, direct comparison of endpoint and real-time dPCR, to be accomplished.
For the comparison of real-time and endpoint dPCR, two types of assays were used in this study, mutation detection assays and an amplification detection assay. The mutation detection assays encompassed three different EGFR mutations, exon 19 deletion (19del), T790M, and L858R. These mutations were chosen as they are highly prevalent in the development of NSCLC and have been extensively validated for CDx tests. Additionally, current CDx tests for these mutations lack sensitivity, especially at low allele frequencies, making any improvements to these tests important. These mutations were tested at different MAF ranging from 0.00% to 1.00%, for EGFR T790M, or 2.00%, for EGFR 19del and L858R. HER2 amplification was tested at different HER2 to reference gene ratios ranging from 1.00 to 1.30 to see if there are improvements to sensitivity and quantification accuracy when targets for detection were not extremely low.
Lower baseline, improved LoD, and more accurate MAF with real-time dPCR for EGFR 19del mutation detection assay
After data collection, a baseline was first established to set the threshold for the number of positive wells required to confidently identify a true positive sample. This step is necessary for both real-time and endpoint dPCR methods and is critical for evaluating the LoD for each assay. As expected, the amount of background signal was different between the two dPCR methods and for different EGFR mutation detection assays.
For EGFR 19del assay, when 20 replicates of wildtype samples (20 ng) were tested, endpoint dPCR had 1 sample with 4 FAM positive wells, 2 samples with 3 FAM positive wells, 4 samples with 2 FAM positive wells, 8 samples with 1 FAM positive wells, and 4 samples with 0 FAM positive wells (Table 1). One of the 20 samples failed to process due to quality control (QC) issues. In contrast, real-time dPCR had only 3 samples with 1 FAM positive well and the remaining 14 samples had 0 FAM positive wells. Three of the 20 samples failed to process due to QC issues. Thus, for real-time dPCR, 2 or more FAM positive wells for a sample was considered positive for the mutation; for endpoint dPCR, 5 or more positive wells was considered a positive sample. Going further, when looking at paired data, 10 out of 14 chips that had 0 FAM signal for real-time dPCR, had a range of 1–4 FMA signal, suggesting false positive signals from end point dPCR were successfully removed. Other 4 chips also had 0 FAM positive signal for endpoint dPCR. For samples that had 1 FAM positive signal with real-time dPCR, the corresponding endpoint dPCR results had 1 or 2 FAM positive signal (Table 1). These results confirmed that real-time dPCR can take out false positive datapoints, leading to a lower the baseline for real-time dPCR assays in comparison to end point dPCR.
Table 1
dPCR chip number | 0.00% nominal MAF | 0.06% nominal MAF | |||
---|---|---|---|---|---|
Endpoint dPCR FAM positive wells | Real-time dPCR FAM positive wells | Endpoint dPCR FAM positive wells | Real-time dPCR FAM positive wells | ||
1 | 0 | 0 | 4 | 3 | |
2 | 3 | 0 | 2 | 2 | |
3 | 2 | 0 | 4 | 3 | |
4 | 3 | 0 | 5 | 2 | |
5 | 1 | 1 | 5 | 2 | |
6 | 1 | 0 | 4 | 3 | |
7 | 2 | 0 | 5 | 4 | |
8 | 0 | 0 | 13 | 3 | |
9 | N/A | N/A | 6 | 5 | |
10 | N/A | N/A | 10 | 3 | |
11 | 0 | 0 | 6 | 6 | |
12 | 0 | 0 | 5 | 4 | |
13 | 1 | 0 | 8 | 6 | |
14 | 1 | 0 | 4 | 2 | |
15 | 1 | 0 | 5 | 4 | |
16 | 4 | 0 | 7 | 6 | |
17 | 1 | 1 | 5 | 4 | |
18 | 2 | 1 | 1 | 1 | |
19 | 1 | 0 | 7 | 2 | |
20 | N/A | N/A | 7 | 6 |
The comparison of endpoint and real-time dPCR was conducted on the same chip. Nineteen chips were analyzed for 0.00% nominal MAF, and 20 chips were analyzed for 0.06% nominal MAF. Real-time imaging data was collected with the Gnomegen Real-Time Digital PCR Instrument; endpoint data was collected using Thermo Fisher Scientific’s QuantStudioTM 3D instrument. The runs that failed were marked as N/A. dPCR, digital polymerase chain reaction; EGFR, epidermal growth factor receptor; FAM, fluorescein amidite; MAF, mutant allele frequency.
Through removing false positive signal, the baseline could be lowered which, in turn, improves the mutation detection capabilities at low allele frequencies. In Table 1, twenty repeats of contrived samples with EGFR 19del mutation at 0.06% MAF were tested similarly to the wildtype samples. At this MAF, 3–4 copies of mutant DNA are expected when 20 ng of gDNA were used based on 3.3 pg per one genome copy of human gDNA. Endpoint dPCR determined 14 samples as positive, with the baseline being 5 FAM positive wells. The number of FAM positive wells ranged from 5 to 13 wells, which is higher than the expected number of mutant copies. Real-time dPCR, by contrast, determined 19 out of 20 samples to be positive with the baseline of 2 FAM positive wells. More importantly, real-time dPCR found that positive samples had FAM positive wells ranging from 2 to 6 wells, which is closer to the expected 3–4 copies. Variations may be due to sampling error when extremely low copy numbers are expected.
When considering the baselines for EGFR 19del on real-time and endpoint dPCR, real-time dPCR would have a lower LoD than endpoint dPCR. The FDA defines LoD as the lowest concentration that can be detected as positive in 19 out of 20 replicates (23). Using this definition, real-time dPCR would have a LoD at or below 0.06% MAF for the EGFR 19del assay. In comparison, endpoint dPCR not only had an inconsistent number of mutant copies when compared to the expected values, but also found 14 out of 20 samples positive suggesting the LoD is higher than 0.06%. Figure 1 further confirms that a LoD cannot be established at 0.06% MAF for endpoint dPCR because there is overlap in signal range between 0.00% and 0.06% MAF (blue bars). In contrast, real-time dPCR does not have signal that overlaps making a LoD of around 0.06% MAF feasible (orange bars). While the difference between 14 and 19 positive samples, or an LoD below or above 0.06% MAF, does not seem particularly significant, it holds incredible clinical significance. If those were clinical samples, by removing false negative datapoints, 5 more patients would be found positive for the mutation and would benefit from target therapy. With the pressing need of liquid biopsy to be more sensitive, these improvements at extremely low MAF are critical.
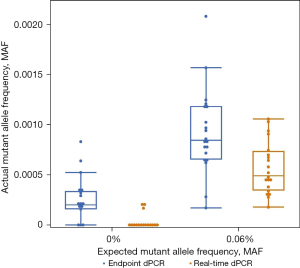
Another benefit of removing false positive datapoints and lowering the baseline is that the observed MAF is closer the expected MAF, making quantification more accurate. The comparison of expected and observed MAF are shown in a variety of ways. One way to compare the expected and observed MAF is to look at the mean values for each MAF. For EGFR 19del, at 0.06% expected MAF, the observed MAF for real-time dPCR from the same samples was 0.05% (95% CI: 0.04–0.05%) while the observed MAF for endpoint dPCR was 0.08% (95% CI: 0.07–0.09%) (Table 2). The percent change from endpoint dPCR to real-time dPCR was a 37.5% decrease. On the other hand, at 2.00% expected MAF, the observed MAF for real-time dPCR was 1.75% (95% CI: 1.62–1.89%), and the observed MAF for endpoint dPCR was 1.88% (95% CI: 1.74–2.02%). The percent change from endpoint dPCR to real-time dPCR was only 6.91%. GLMM calculated mean estimates of the observed MAF for real-time dPCR was closer to the expected value than endpoint dPCR which agrees with the improved detection. The differences in percent change for 0.06% MAF and 2.00% MAF show that the benefit of real-time dPCR is at detecting low allele frequencies; this point will be reiterated in the EGFR 19del data and investigated further when discussing results from HER2.
Table 2
Marker | Expected MAF or ratio | Endpoint dPCR | Real-time dPCR | |||
---|---|---|---|---|---|---|
Observed MAF or ratio | 95% CI | Observed MAF or ratio | 95% CI | |||
T790M | 0.00% | 0.04% | 0.03–0.04% | 0.01% | 0.01–0.02% | |
0.03% | 0.09% | 0.08–0.11% | 0.04% | 0.03–0.05% | ||
0.10% | 0.17% | 0.15–0.20% | 0.09% | 0.07–0.10% | ||
1.00% | 1.12% | 1.05–1.20% | 1.03% | 0.96–1.10% | ||
19del | 0.00% | 0.02% | 0.02–0.02% | 0.00% | 0.00–0.00% | |
0.06% | 0.08% | 0.07–0.09% | 0.05% | 0.04–0.05% | ||
0.20% | 0.26% | 0.22–0.30% | 0.19% | 0.16–0.21% | ||
2.00% | 1.88% | 1.74–2.02% | 1.75% | 1.62–1.89% | ||
L858R | 0.00% | 0.07% | 0.05–0.08% | 0.02% | 0.01–0.02% | |
0.06% | 0.10% | 0.09–0.12% | 0.05% | 0.04–0.06% | ||
0.20% | 0.21% | 0.19–0.24% | 0.14% | 0.12–0.16% | ||
2.00% | 2.06% | 1.97–2.16% | 1.87% | 1.79–1.97% | ||
HER2 | 1.00 | 1.04 | 1.02–1.07 | 1.02 | 1.00–1.04 | |
1.09 | 1.08 | 1.06–1.10 | 1.07 | 1.05–1.09 | ||
1.18 | 1.25 | 1.22–1.28 | 1.25 | 1.23–1.28 | ||
1.30 | 1.34 | 1.31–1.36 | 1.34 | 1.31–1.36 |
Each condition was replicated ≥12 times. Expected MAF is based on the ratios of wildtype and mutant cell line DNA prepared for the contrived samples. Observed MAF is the mean observed ratio of wildtype and mutant genotypes calculated from endpoint and real-time dPCR data of each condition. The 95% CI was calculated from the observed MAF data using GLMM method. CI, confidence interval; dPCR, digital polymerase chain reaction; EGFR, epidermal growth factor receptor; GLMM, generalized linear mixed model; HER2, human epidermal growth factor receptor 2; MAF, mutant allele frequency.
Another way to describe the comparison of expected and observed MAF is to compare the linearity and distribution of data. The regression analysis of endpoint and real-time dPCR shows that datapoints for real-time dPCR are more closely aligned to the predicted MAF than endpoint dPCR’s datapoints at low allele frequencies ranging from 0.00% to 0.20% MAF, as indicated by the closeness of the orange (real-time dPCR) and grey (reference) lines (Figure 2A). At a high allele frequency of 2.00% MAF, the results are comparable. The distribution of individually plotted datapoints visually show that the distribution of real-time MAF datapoints (orange) is closer to the expected MAF and has a smaller spread than endpoint MAF datapoints (blue), especially at low allele frequencies (Figure 2B). It is interesting to note that while the observed data for real-time dPCR is closer to the expected data, endpoint dPCR tends to overestimate the MAF (Figure 2C), suggesting the presence of false positive FAM signals. By subtracting the MAF of real-time dPCR from the MAF of endpoint dPCR, the degree of difference shows to what extent the values detected for both methods are different. These results show that endpoint dPCR consistently overestimates the MAF especially at low allele frequencies, as shown by negative values being present for all allele frequencies.
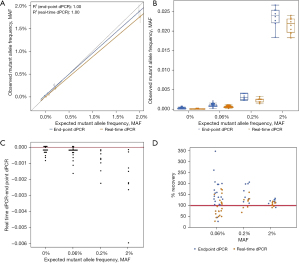
Lastly, by removing false positive datapoints, the accuracy of real-time dPCR is better than endpoint dPCR. Mean percent recovery was calculated to determine the accuracy of inter-assay measurements. For the EGFR 19del dataset, the mean percent recovery for real-time dPCR was 95% at 0.06 % MAF, 115% at 0.2% MAF, and 108% at 2% MAF (Table 3). The mean percent recovery for endpoint dPCR was 154% at 0.06% MAF, 159% at 0.2% MAF, and 118% at 2% MAF. The distribution of percent recovery is much wider for endpoint dPCR (blue datapoints) than real-time dPCR (orange datapoints, Figure 2D). The spread of datapoints for real-time dPCR is closer to the 100% accuracy line (red) than endpoint dPCR reiterating that real-time dPCR has improved accuracy. The Wilcoxon rank test for matched pairs was performed for all concentrations measured (0%, S=−60.0, P=0.0007; 0.06%, S=−103.0, P<0.0001; 0.2%, S=−18.0, P=0.0078; 2%, S=−18.0, P=0.0078) indicate that the distribution of technical replications is not similar across the two instruments. Together, the data presented corroborates that real-time dPCR has more accurate absolute quantification capabilities than endpoint dPCR for extremely low values; the ability to accurately detect the number of positive wells is shown to be consistently important for samples with low allele frequency.
Table 3
Marker | Expected MAF | Endpoint dPCR, mean % recovery | Real-time dPCR, mean % recovery |
---|---|---|---|
19del | 0.06% | 154 | 95 |
0.20% | 159 | 115 | |
2.00% | 118 | 108 | |
T790M | 0.03% | 352 | 167 |
0.10% | 201 | 101 | |
1.00% | 133 | 123 | |
L858R | 0.06% | 206 | 100 |
0.20% | 130 | 84 | |
2.00% | 126 | 113 | |
HER2 | 1.00 | 102 | 97 |
1.09 | 97 | 96 | |
1.18 | 99 | 99 | |
1.30 | 97 | 97 |
Mean recovery was closer to 100 in real-time dPCR results than endpoint dPCR. Differences in mean recovery was greater at lower allele frequencies than high allele frequencies. dPCR, digital polymerase chain reaction; HER2, human epidermal growth factor receptor 2; MAF, mutant allele frequency.
Improved sensitivity and quantification accuracy with real-time dPCR were also observed from other EGFR mutation detection assays
While EGFR 19del assay was the first one used to compare sensitivity and quantification accuracy, EGFR T790M and L858R were also tested to corroborate or disprove results determined by EGFR 19del. First, for both EGFR T790M and L858R, baselines needed to be established with wildtype samples. A single baseline could not be used for all assays as differences in assay functionality make this impossible. The results from wildtype samples for EGFR T790M and L858R confirm that real-time dPCR has a lower baseline than endpoint dPCR, validating the results from EGFR 19del, data not shown. After the baselines were determined for both mutations for real-time and endpoint dPCR, the lowest MAF was compared between endpoint and real-time dPCR for both mutations. Both EGFR T790M and L858R showed similar trends to 19del, the number of wells to determine a sample as positive was lower for real-time dPCR than endpoint dPCR, data not shown.
Similar to EGFR 19del, the observed MAF was closer to the expected MAF especially at low allele frequencies, and the percent change is larger between real-time and endpoint dPCR for low allele frequencies than for high allele frequencies. For EGFR T790M, at 0.03% expected MAF, the observed MAF for real-time dPCR was 0.04% (95% CI: 0.03–0.05%), and the observed MAF for endpoint dPCR was 0.09% (95% CI: 0.08–0.11%) (Table 2). The percent change from endpoint dPCR to real-time dPCR was a 55.56% decrease. On the other hand, at 1.00% expected MAF, the observed MAF for real-time dPCR was 1.03% (95% CI: 0.96–1.10%) while the observed MAF for endpoint dPCR was 1.12% (95% CI: 1.05–1.20%). The percent change was an 8.04% decrease from endpoint to real-time dPCR. For EGFR L858R, at the expected MAF of 0.06%, real-time dPCR has an observed MAF of 0.05% (95% CI: 0.04–0.06%) while endpoint dPCR has an observed MAF of 0.10% (95% CI: 0.09–0.12%). The percent change from endpoint to real-time dPCR was a 50% decrease. At the expected MAF of 2.00%, the observed MAF for real-time dPCR was 1.87% (95% CI: 1.79–1.97%) while endpoint dPCR has an observed MAF of 2.06% (95% CI: 1.97–2.16%). The percent change was a 9.22% decrease from endpoint to real-time dPCR. The results for both EGFR T790M and L858R confirm the findings from EGFR 19del.
Additionally, when comparing the linearity and distribution of data for EGFR T790M, real-time dPCR was closer to the expected MAF than endpoint dPCR with the distribution of real-time dPCR replicate datapoints being closer to the expected MAF as well (Figure 3A,3B). This result is comparable to the results seen with EGFR 19del. Endpoint dPCR overestimates the MAF while real-time dPCR underestimates the MAF and is more accurate (Figure 3C,3D). The results regarding linearity, distribution of data, overestimation of MAF, and accuracy were also seen with EGFR L858R (Figure 4). For both mutations, real-time dPCR is more accurate than endpoint dPCR with the percent recovery and mean percent recovery closer to 100% (Table 3). For the purposes for validating EGFR 19del data, both EGFR T790M and L858R, confirm all conclusions made by EGFR 19del.
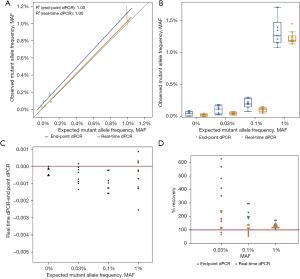
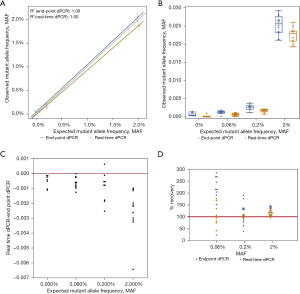
Comparable results for real-time and endpoint dPCR for HER2 amplification detection assay
Unlike EGFR T790M and L858R mutations, HER2 amplification was used to determine if sensitivity would improve when higher copies of target molecules are present. Dissimilar from the mutation detection assays tested, the observed HER2 to reference gene ratios shows that there is minimal difference in detection between real-time and endpoint dPCR. At an expected ratio of 1.00 for HER2 over reference gene, the observed ratio of endpoint dPCR was 1.04 (95% CI: 1.02–1.07), and the observed ratio of real-time dPCR was 1.02 (95% CI: 1.00–1.04) (Table 2). The percent change from endpoint to real-time dPCR is a decrease of 1.92%. At higher ratios such as 1.18 and 1.30, the ratios were the same for endpoint and real-time dPCR. Measurements of contrived samples yielded similar HER2 to reference gene ratio estimates for both instruments across different ratios (Figure 5A,5B). For analysis results between both instrument platforms, the observed HER2 to reference gene ratio for real-time and endpoint dPCR are closer to each other for this copy number variation (CNV) assay than previous mutation detection assays (Figure 5C). When looking at percent accuracy, endpoint dPCR tends to overestimate the MAF and real-time dPCR tends to underestimate the MAF, but the degree of over- and underestimation is not as extreme as the EGFR mutation detection assays (Figure 5D). Wilcoxon rank test for matched pairs was performed for all concentrations measured; however, values measured were not significantly different across endpoint dPCR and real-time dPCR instrument for HER2 marker (0%, S=−16.0000, P=0.1748; 1.5%, S=0.5000, P=1.0000; 3%, S=−6.0000, P=0.6377; 5%, S=4.5000, P=0.8077). These results support the notion that at higher allele frequencies the absolute quantification abilities of both mutation detection methods are comparable. The benefit of real-time dPCR lies in its detection at low allele frequencies. This is because the presence of a small number of false positive microreactions would occur for both HER2 (FAM labeled) and the reference gene [victoria (VIC) labeled] such that the removal of a small number of false positive datapoints on a dPCR chip will not have a significant impact on the ratio for HER2 over reference gene although the total number of positive FAM and VIC wells may change.
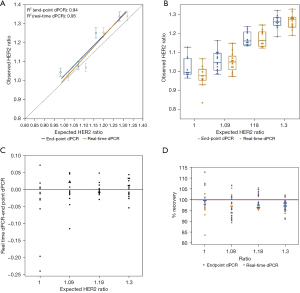
Discussion
In this study our goal was to demonstrate improved sensitivity and quantitative accuracy of real-time digital PCR to resolve the issue of poor sensitivity seen in many liquid biopsy tests. Most qPCR tests struggle to distinguish signal between 0.1% and 1% MAF (1); even endpoint dPCR has difficulty detecting signal at 0.1% or 0.2% MAF as seen in this paper for some assays. What distinguishes real-time dPCR is its ability to incorporate real-time amplification data into the final end-point measurements of dPCR. We used an algorithm for identifying and removing false positive datapoints based on amplification curve profiles for a clinical assay we validated (21); this enables a small but significant increase in sensitivity with a corresponding lower LoD for the assays we tested. One key point to remember is that each pairwise endpoint and real-time dPCR comparison (≥8 replicates per condition) comes from the same exact sample; dPCR chips were analyzed on two different dPCR platforms each with their own data processing algorithm. Thus, the differences we see are entirely the result of imaging and data processing differences between the Thermo Fisher QuantStudioTM 3D Digital PCR Instrument (endpoint dPCR) and the Gnomegen Real-Time Digital PCR Instrument. The cross compatibility of the dPCR chips on these two systems makes this possible.
The biggest improvement of real-time dPCR over endpoint dPCR is the lower baseline. This lower baseline brings out signal at low allele frequencies more effectively, thus improving assay sensitivity. As a result, assays tested utilizing this technology would have a lower LoD leading to fewer false negative samples because the positive signal that is present is more likely to represent true positives. At high allele frequencies, endpoint dPCR is comparable with real-time dPCR as the relatively small number of false positive signals represent only a small fraction of the total true positive signals.
Improving sensitivity allows real-time dPCR to benefit CDx in multiple avenues
For clinical applications, the amount of circulating tumor DNA (ctDNA) in plasma depends on tumor burden. In general, mutation bearing ctDNA from cancer cells only represents a small portion of cell-free circulating DNA derived from normal cells. For CDx liquid biopsy assays, the need for improved sensitivity is repeatedly demonstrated as many as 50% patients tested positive by tissue biopsy remain negative by liquid biopsy tests. Thus, the improvement of accurately determining 5–10 copies of mutant DNA as positive, for endpoint dPCR, to accurately determining 2–4 copies as positive, for real-time dPCR, becomes extremely helpful to identify more patients who would benefit from target therapy. This would make liquid biopsy more robust thus potentially reducing the need to retest patients with tissue biopsy when a negative result from liquid biopsy is obtained.
Although this study was conducted in a research setting, these findings can be extrapolated to patient populations. It is expected that improved sensitivity could be achieved in clinical samples, similar to the contrived samples used for this study. Patient populations that would benefit from real-time dPCR include patients diagnosed with late-stage cancers or who have undergone multiple lines of therapy as their test results are often falsely negative due to tumor heterogeneity. With liquid biopsy, many more mutations could be detected in the ctDNA but the reality of this occurring is dependent on how sensitive the test is. Another population is patients who need continuous monitoring for the presence of mutations, that had been previously found negative during therapy, as they may have developed resistance to the current therapeutic regimen. Their acquired mutations could make them eligible for targeted therapy. Because additional tissue biopsies are not possible at this point in time, if treatments were based off of previous tissue biopsy results, acquired mutations would go undetected. Real-time dPCR would more effectively be able to detect these mutations at low levels.
There is a growing number of target therapies on the market and more under development. Many different cancers would benefit greatly from real-time dPCR technology, not just the ones that are analyzed in this study. Besides the EGFR mutations for NSCLC that we presented in this paper, there are other mutations in KRAS, BRAF, PIK3CA, etc. that are tested using CDx assays for other cancers including breast, thyroid, gastric, colorectal cancers. While the benefit of real-time dPCR lies in its ability to detect low allele frequencies, the sensitivity at high allele frequencies is comparable with other PCR technologies on the market meaning that real-time dPCR can assess other types of assays such as gene amplification and gene fusion assays.
In the coming future, a new form of treatment will require liquid biopsy tests to be even more sensitive than they currently are. Antibody-drug conjugate (ADC) drugs, which consists of an antibody linked to a small molecule drug, will have a lower baseline than traditional target therapy, which still requires liquid biopsy tests with improved sensitivity (24). This is due to the improved therapeutic effects of ADC drugs (25-27). As more and more ADC drugs are developed there is now an additional clinical need for improved sensitivity for CDx assays.
The advantage of real-time dPCR over next generation sequencing (NGS) for CDx
Although there are many benefits to real-time dPCR, some would argue that NGS is the future of CDx. While NGS assays are extremely high throughput and tremendous efforts were put in to improve its sensitivity by reducing the amount of false positive reads, however, the biggest drawback for NGS lies in its pre-analytical process. Library preparation for NGS has two ligation steps which adds sequencing adaptors to either end of fragmented DNA. This issue with these ligation steps is that each has an efficiency of around 20% (28,29). Combined, the ligation steps have around 4% yield. As a result, if there are low copy numbers to be expected, such as 3–4 copies with 0.06% MAF, there is a very slim likelihood that these mutations will be present in the library. While there has been focus on improving the sensitivity of NGS, these measures are after the pre-analytical phase. Therefore, if the mutations are not present in the library, there is no way they are going to found after pre-analysis, regardless of the sensitivity after sequencing libraries are made. Additionally, NGS is prone to get random background due to a combination of the annealing step and low copy numbers. This worsens the sensitivity of NGS at low allele frequencies. While there are some benefits to NGS, such as calculating tumor mutation burden, for the purposes of CDx, it is not as sensitive as real-time dPCR at low allele frequencies.
Other considerations for real-time dPCR on CDx applications
While the LoD is low, it may seem that the input of DNA, at 20 ng, is high. However, with the lowest detected MAF at 0.06% or 0.03%, depending on the mutation, 3–4 copies of mutant alleles are expected. If the input amount of DNA were to decrease, fractions of copies would be expected making it impossible to detect mutations. In addition, it was found in a previously published paper that as the input amount of DNA decreases, the amount of background signal would increase in comparison to the signal (30). This would make it even more difficult to discern between real and false positive signal and to cut a threshold. For these reasons, 20 ng is an appropriate amount of input DNA for real time dPCR rare allele assays.
Another concern with an extremely sensitive assay is specificity. We have demonstrated in our tests that at the same baseline, our negative samples were determined negative for all mutations tested. This is because we have two thresholds. One threshold is for fluorescent intensity that allows us to determine if a well is positive or negative for PCR amplification; this determines the number of positive wells. This threshold is established using real-time amplification curves. A second threshold is used to call a sample positive or negative based on the MAF and the minimum number of positive wells required; this was established by negative samples during assay development. These two independent thresholds would address concerns that improved sensitivity would reduce specificity as in the case of qPCR when only one threshold is used to determine if a sample is positive.
Additionally, for each real-time dPCR-based CDx assay, an algorithm needs to be developed based on results from reference standards and contrived clinical samples. Algorithms need to be validated in clinical samples to ensure results from all samples are processed objectively to avoid human error and to maintain consistency among different test samples.
Due to the need for continuous monitoring of each individual partition during PCR amplification for real time dPCR, chip-based dPCR became the only option. ddPCR, as discussed in the Introduction, would not be easily developed to perform real time monitoring as droplets cannot be distinguished; the wells of chip-based dPCR are distinguishable because they remain in the same position throughout thermocycling and imaging.
Conclusions
It is clinically important to improve the sensitivity of liquid biopsy tests as providing health care workers with the knowledge of all the mutations present would provide a higher percentage of patients the most appropriate treatment options in a timely manner. Real-time digital PCR has been shown to improve sensitivity at low allele frequencies across contrived samples tested at low allele frequencies. After clinical validation, this technology would greatly benefit the landscape of CDx and, most importantly, would benefit the patients who otherwise would not receive specific targeted therapy.
Acknowledgments
Funding: Support for the study was provided by the National Key Research and Development Project (2019YFC1315700, to Dr. Jie Wang), the National Natural Sciences Foundation Key Program (81630071, to Dr. Jie Wang), CAMS Innovation Fund for Medical Sciences (CIFMS 2016-I2M-3-008, to Jie Wang; 2017-I2M-1-005, to Dr. Zhijie Wang), Aiyou Foundation (KY201701, to Dr. Jie Wang), CAMS Key Lab of Translational Research on Lung Cancer (2018PT31035, to Dr. Jie Wang), the National Natural Sciences Foundation (81871889 and 82072586 to Dr. Zhijie Wang), Beijing Natural Science Foundation (7212084 to Dr. Zhijie Wang), National Natural Sciences Foundation (82102886 to Dr. Jiachen Xu), Beijing Hope Run Special Fund of Cancer Foundation of China (LC2020B09 to Dr. Jiachen Xu), the Special Research Fund for Central Universities, Peking Union Medical College (3332021029 to Dr. Jiachen Xu).
Footnote
Reporting Checklist: The authors have completed the MDAR reporting checklist. Available at https://dx.doi.org/10.21037/tlcr-21-728
Data Sharing Statement: Available at https://dx.doi.org/10.21037/tlcr-21-728
Peer Review File: Available at https://dx.doi.org/10.21037/tlcr-21-728
Conflicts of Interest: All authors have completed the ICMJE uniform disclosure form (available at https://dx.doi.org/10.21037/tlcr-21-728). SRH, GC and PO were paid consultants during the time they worked on this manuscript. The other authors have no conflicts of interest to declare.
Ethical Statement: The authors are accountable for all aspects of the work in ensuring that questions related to the accuracy or integrity of any part of the work are appropriately investigated and resolved.
Open Access Statement: This is an Open Access article distributed in accordance with the Creative Commons Attribution-NonCommercial-NoDerivs 4.0 International License (CC BY-NC-ND 4.0), which permits the non-commercial replication and distribution of the article with the strict proviso that no changes or edits are made and the original work is properly cited (including links to both the formal publication through the relevant DOI and the license). See: https://creativecommons.org/licenses/by-nc-nd/4.0/.
References
- Xu J, Wu W, Wu C, et al. A large-scale, multicentered trial evaluating the sensitivity and specificity of digital PCR versus ARMS-PCR for detecting ctDNA-based EGFR p.T790M in non-small-cell lung cancer patients. Transl Lung Cancer Res 2021;10:3888-901. [Crossref] [PubMed]
- Wellmark. Circulating Tumor DNA for Management of Non-Small Cell Lung Cancer (Liquid Biopsy) [Internet]. Effective date 2019. Available online: http://www.wellmark.com/
- National Comprehensive Cancer Network. NCCN Guidelines In Oncology (NCCN Guidelines®): Non-Small Cell Lung Cancer, Metastatic [Internet]. Effective date 2020. Available online: http://www.nccn.org/patients/
- Malapelle U, Pisapia P, Rocco D, et al. Next generation sequencing techniques in liquid biopsy: focus on non-small cell lung cancer patients. Transl Lung Cancer Res 2016;5:505-10. [Crossref] [PubMed]
- Maltoni R, Casadio V, Ravaioli S, et al. Cell-free DNA detected by "liquid biopsy" as a potential prognostic biomarker in early breast cancer. Oncotarget 2017;8:16642-9. [Crossref] [PubMed]
- Tsui DWY, Blumenthal GM, Philip R, et al. Development, Validation, and Regulatory Considerations for a Liquid Biopsy Test. Clin Chem 2020;66:408-14. [Crossref] [PubMed]
- Malapelle U, Sirera R, Jantus-Lewintre E, et al. Profile of the Roche cobas® EGFR mutation test v2 for non-small cell lung cancer. Expert Rev Mol Diagn 2017;17:209-15. [Crossref] [PubMed]
- Han AL, Kim HR, Choi KH, et al. Comparison of cobas EGFR Mutation Test v2 and PANAMutyper-R-EGFR for Detection and Semi-Quantification of Epidermal Growth Factor Receptor Mutations in Plasma and Pleural Effusion Supernatant. Ann Lab Med 2019;39:478-87. [Crossref] [PubMed]
- Kang J, Lee A, Lee YS. Prediction of PIK3CA mutations from cancer gene expression data. PLoS One 2020;15:e0241514. [Crossref] [PubMed]
- Hindson CM, Chevillet JR, Briggs HA, et al. Absolute quantification by droplet digital PCR versus analog real-time PCR. Nat Methods 2013;10:1003-5. [Crossref] [PubMed]
- Brunetto GS, Massoud R, Leibovitch EC, et al. Digital droplet PCR (ddPCR) for the precise quantification of human T-lymphotropic virus 1 proviral loads in peripheral blood and cerebrospinal fluid of HAM/TSP patients and identification of viral mutations. J Neurovirol 2014;20:341-51. [Crossref] [PubMed]
- Strain MC, Lada SM, Luong T, et al. Highly precise measurement of HIV DNA by droplet digital PCR. PLoS One 2013;8:e55943. [Crossref] [PubMed]
- Sedlak RH, Cook L, Cheng A, et al. Clinical utility of droplet digital PCR for human cytomegalovirus. J Clin Microbiol 2014;52:2844-8. [Crossref] [PubMed]
- Belgrader P, Tanner SC, Regan JF, et al. Droplet digital PCR measurement of HER2 copy number alteration in formalin-fixed paraffin-embedded breast carcinoma tissue. Clin Chem 2013;59:991-4. [Crossref] [PubMed]
- Gevensleben H, Garcia-Murillas I, Graeser MK, et al. Noninvasive detection of HER2 amplification with plasma DNA digital PCR. Clin Cancer Res 2013;19:3276-84. [Crossref] [PubMed]
- Conte D, Verri C, Borzi C, et al. Novel method to detect microRNAs using chip-based QuantStudio 3D digital PCR. BMC Genomics 2015;16:849. [Crossref] [PubMed]
- Hindson BJ, Ness KD, Masquelier DA, et al. High-throughput droplet digital PCR system for absolute quantification of DNA copy number. Anal Chem 2011;83:8604-10. [Crossref] [PubMed]
- Spurgeon SL, Jones RC, Ramakrishnan R. High throughput gene expression measurement with real time PCR in a microfluidic dynamic array. PLoS One 2008;3:e1662. [Crossref] [PubMed]
- Quan PL, Sauzade M, Brouzes E. dPCR: A Technology Review. Sensors (Basel) 2018;18:1271. [Crossref] [PubMed]
- Ruiz-Villalba A, van Pelt-Verkuil E, Gunst QD, et al. Amplification of nonspecific products in quantitative polymerase chain reactions (qPCR). Biomol Detect Quantif 2017;14:7-18. [Crossref] [PubMed]
- Duong K, Ou J, Li Z, et al. Increased sensitivity using real-time dPCR for detection of SARS-CoV-2. Biotechniques 2021;70:7-20. [Crossref] [PubMed]
- Vynck M, Vandesompele J, Nijs N, et al. Flexible analysis of digital PCR experiments using generalized linear mixed models. Biomol Detect Quantif 2016;9:1-13. [Crossref] [PubMed]
- US Food and Drug Administration. Molecular Diagnostic Template for Laboratories [Internet]. 28 July 2020. Available online: http://www.fda.gov/
- Bouchard H, Viskov C, Garcia-Echeverria C. Antibody-drug conjugates—a new wave of cancer drugs. Bioorg Med Chem Lett 2014;24:5357-63. [Crossref] [PubMed]
- Nakada T, Sugihara K, Jikoh T, et al. The Latest Research and Development into the Antibody-Drug Conjugate, [fam-] Trastuzumab Deruxtecan (DS-8201a), for HER2 Cancer Therapy. Chem Pharm Bull (Tokyo) 2019;67:173-85. [Crossref] [PubMed]
- Amadori S, Suciu S, Selleslag D, et al. Gemtuzumab Ozogamicin Versus Best Supportive Care in Older Patients With Newly Diagnosed Acute Myeloid Leukemia Unsuitable for Intensive Chemotherapy: Results of the Randomized Phase III EORTC-GIMEMA AML-19 Trial. J Clin Oncol 2016;34:972-9. [Crossref] [PubMed]
- Castaigne S, Pautas C, Terré C, et al. Effect of gemtuzumab ozogamicin on survival of adult patients with de-novo acute myeloid leukaemia (ALFA-0701): a randomised, open-label, phase 3 study. Lancet 2012;379:1508-16. Erratum in: Lancet 2018 Mar 3;391(10123):838. doi: 10.1016/S0140-6736(17)32105-0. [Crossref] [PubMed]
- Aigrain L, Gu Y, Quail MA. Quantitation of next generation sequencing library preparation protocol efficiencies using droplet digital PCR assays - a systematic comparison of DNA library preparation kits for Illumina sequencing. BMC Genomics 2016;17:458. [Crossref] [PubMed]
- Nix DA, Hellwig S, Conley C, et al. The stochastic nature of errors in next-generation sequencing of circulating cell-free DNA. PLoS One 2020;15:e0229063. [Crossref] [PubMed]
- Zhou R, Cai Y, Li Z, et al. A digital PCR assay development to detect EGFR T790M mutation in NSCLC patients. Frontiers in Laboratory Medicine 2018;2:89-96. [Crossref]