Cross-talk of pyroptosis and tumor immune landscape in lung adenocarcinoma
Introduction
As a hallmark of cancer, the ability to escape cell death not only contributes to the origin of cancer, but also plays a crucial role in acquisition of therapy-resistance, progression and metastasis (1). Pyroptosis, a lytic pro-inflammatory type of regulated cell death that activates the immune system, which was characterized by membrane perforation, cell swelling induced by cleaved gasdermin family members (GSDMs), the release of cellular content, nuclear condensation and DNA fragmentation (2). Recent studies indicated that pyroptosis may act as a double-edged sword in promoting and inhibiting tumor cell growth in tumors. Pyroptosis leads to chronic inflammation activation which facilitates tumor growth, angiogenesis, invasion, and metastasis (3). It has been shown that caspase-1-deficient mice demonstrate increased colonic epithelial cell proliferation in early stages of injury-induced tumor formation formation (4). Conversely, recent studies have proved that pyroptosis in tumor cells may function as a tumor suppression mechanism (5). Gasdermin E (GSDME) is silenced in most cancer cells. GSDME can function as a tumor suppressor by converting caspase-3-mediated apoptosis to pyroptosis in melanoma, breast cancer and colon cancer (6). Moreover, investigators have found that some anti-cancer agents can induce pyroptosis to inhibit tumorigenesis and development.
With over 2.2 million new cases and 1.8 million deaths worldwide in 2020, lung cancer is currently the most common malignancy and the leading cause of cancer-related mortality worldwide (7,8). Adenocarcinoma is the most common histologic type, which accounts for approximately 40% of lung cancers. Most patients are diagnosed with locally advanced and metastatic disease, resulting in poor prognosis with less than 20% 5-year overall survival (OS) rate of lung adenocarcinoma (LUAD) (9). Molecular targeted therapies, mainly tyrosine kinase inhibitors (TKIs), have improved the overall survival for LUAD patients with actionable driver mutations, such as epidermal growth factor receptor (EGFR), anaplastic lymphoma kinase (ALK), c-ros oncogene 1 (ROS1). On the other hand, the development of immune checkpoint inhibitors (ICIs) has been extensively renovated the standard treatment for patients with driver-independent LUAD (10,11). However, the biomarkers for predicting the response to immunotherapy are not satisfactory (12), which still do not completely reflect the intratumor heterogeneity of LUAD (13). Accordingly, there is an urgent need for better prognostic tools and biomarkers that are capable of accurately predicting the behaviors of tumor.
Emerging evidence reveals a close relationship between pyroptosis and adaptive immunity. When pyroptosis occurs, a variety of danger-associated signaling molecules and cytokines are activated and released, lead to extensive effects on the tumor microenvironment (TME), immune cell recruitment and tumor growth. A few studies have uncovered that the potent proinflammatory effects of pyroptosis are correlated with the regulation of TME. GSDMB-dependent pyroptosis formed a CTL-mediated killing mechanism, which might contribute to enhancing antitumor immunity (14,15). The pyroptotic effector GSDMD was positively associated with the number and activity of CD8+ T cell markers in NSCLC samples (16). GSDME-expressing tumors had more tumor-infiltrating immune cells, including CD8+ T lymphocytes, NK cells and tumor-associated macrophages (TAMs) (6), subjected to intrinsic stresses (i.e., hypoxia, ER stress) or extrinsic challenges (i.e., chemotherapy, radiation, cytotoxic lymphocyte attack). Additionally, pyroptosis is characterized by releasing of many proinflammatory factors, including IL-1β, IL-18, ATP, and HMGB1 (4,17), a well-known process for shaping an immunosuppressive TME during the progression of various tumors (18,19). Notably, several pyroptosis inducers exert an obvious synergistic effect with PD-(L)1 inhibitors on tumor inhibition. However, the previous studies were restricted to one or two pyroptosis related genes, and the study of correlations between pyroptosis and TME is still in its infancy and need further investigation. Therefore, a comprehensive understanding of the TME cell infiltration characteristics mediated by pyroptosis will contribute to enhancing our understanding of cancer immunity and the development of immunotherapeutic strategies.
According to recent findings, we hypothesized that as a highly immunogenic form of cell death, pyroptosis could cause local inflammation and attract inflammatory cell infiltration, providing a great opportunity to relieve immunosuppression of tumor microenvironments (TME) and induce a systemic immune response in treating LUAD.
In this study, we comprehensively explored the association between pyroptosis patterns and TME cell-infiltrating characteristics by integrating the transcriptomic and genomic data of 915 LUAD samples from TCGA and GEO databases. We found that pyroptosis patterns were not only associated with the immune cell infiltration, but also with immunogenicity, immunomodulating properties and the activation of epithelial mesenchymal transition (EMT). Moreover, based on expressions of pyroptosis related genes (PRGs), we constructed a scoring scheme to predict clinical outcomes and ICIs therapy response for LUAD patients. These findings suggested that pyroptosis plays an indispensable role in shaping diverse tumor immune during the lung cancer development.
We present the following article in accordance with the MDAR reporting checklist (available at at https://dx.doi.org/10.21037/tlcr-21-715).
Methods
Public cohorts
Genomic, transcriptomic, and clinical data of LUAD from The Cancer Genome Atlas Project (TCGA) were downloaded from the GDC data portal (https://portal.gdc.cancer.gov). The fragments per kilobase million (FPKM) data were transformed into transcripts per kilobase million (TPM) values. A total of 500 patients with both RNA-seq data and corresponding clinical information were enrolled as the training cohort. Three independent LUAD cohorts, including GSE30219 (20) (n=83), GSE31210 (21) (n=226) and GSE37745 (22) (n=106), were obtained from the Gene Expression Omnibus (https://www.ncbi.nlm.nih.gov/geo) database via the “GEOquery” R package. The detailed clinical information of four patient sets was shown in Table S1.
Clinical specimens
We retrospectively collected surgically resected, formalin-fixed, paraffin-embedded LUAD samples from the biobank of National Cancer Center/National Clinical Research Center for Cancer/Cancer Hospital in Chinese Academy of Medical Sciences and Peking Union Medical College (Beijing, China). A total of 142 patients pathologically and clinically diagnosed with LUAD from 2006 to 2015 were selected as an independent cohort in this study and none of the patients received antitumor or immunosuppressive treatments before surgery. The clinical data were obtained by reviewing the patients’ medical histories, which are summarized in Table S2. Pathological staging was evaluated according to the 8th edition of the American Joint Committee on Cancer/Union for International Cancer Control TNM classification system (23).
The study was conducted in accordance with the Declaration of Helsinki (as revised in 2013). All procedures involving human participants were reviewed and approved by the Medical Ethics Committee of Cancer Hospital, Chinese Academy of Medical Sciences (NCC2017ZDXM-001) and individual consent for this retrospective analysis was waived.
Pyroptosis-based consensus clustering analysis
We retrieved the literatures related to pyroptosis, and a total of 48 genes including GSDM family members, inflammasome-related genes, pyroptosis-related caspase family members and cytokines were extracted for further analysis (24-30) (Table S3). Unsupervised clustering analysis was conducted to identify distinct cancer subtypes based on the expression profiles of 48 pyroptosis-related genes. The “CancerSubtypes” R package (31) was applied to execute the consensus clustering and 1,000 times repetitions were performed for stable classification.
Gene set variation analysis (GSVA) and functional enrichment analysis
To investigate the variation of different pyrotosis clusters in biological process, we utilized “GSVA” R package (32) to performed GSVA enrichment analysis. The “h.all.v7.2” gene sets (33) obtained from the MSigDB database and the gene sets constructed by Mariathasan et al. (34) were used for running GSVA analysis. Gene Ontology (GO) (35) annotation for pyroptosis-related genes was analyzed by the “clusterProfiler” (36) R package, with the cutoff value of P<0.05. Gene set enrichment analysis (GSEA) (33) was also conducted in the javaGSEA desktop application (GSEA 4.1.0) to identify the underlying pathways or processes between pyroptosis gene subtypes. Normalized enrichment score (NES) >1.5 and P<0.05 were regarded as significantly enrichment.
Estimation of immune cell infiltration
We quantified the relative abundance of 28 immune cell types in LUAD TME by introducing the single sample gene set enrichment analysis (ssGSEA) algorithm. The gene signature for marking immune cell types were derived from the study of Charoentong et al. (37). The relative infiltration level of each immune cell type in each patient was represented by the enrichment score calculated by ssGSEA.
EMT score
The epithelial-to-mesenchymal transition (EMT) gene signature, including 25 epithelial and 52 mesenchymal marker genes, was derived from Mak et al. (38,39). We calculated the EMT score of each sample using the formula , as described in previous studies. In this formula, M and E indicate the expression of mesenchymal marker genes and epithelial marker genes mentioned above, N and n represent the gene number of mesenchymal marker and epithelial marker, respectively.
Identification of differentially expressed genes (DEGs) among pyroptosis clusters
To identify pyroptosis-related genes, we classified patients into three pyroptosis clusters based on the expression of 48 PRGs. The DEGs among these clusters were screened out using the R package “limma”. The significance criteria for filtering DEGs were set as FDR <0.001.
Construction of pyroptosis-related prognosis signature
Univariate Cox regression analysis were first applied to assess the association between the gene expression of PRGs and overall survival, and a total of 13 genes with P<0.1 were selected as candidate genes. To further shrink the number of candidate genes, we performed the random survival forest analysis using the “randomForestSRC” R package (40). The 6 prognostic genes with importance >0.005 were chosen to performed combination analysis. The 6 genes generated 63 combinations, and for each combination, multivariate Cox regression analysis was conducted and the corresponding multivariate Cox regression coefficient was used to calculate the risk score of each patient. Patients were stratified into high- and low-risk group according the median risk score of each model and Kaplan-Meier analysis was performed to screen the best risk model. Comparing the -log10 P value of 63 models, we found that a 4-gene risk model ranked the top, which was considered as the final risk model. We calculated the risk score of each patient in TCGA-LUAD cohort based on the multivariate Cox regression coefficient of the 4-gene risk model. Patients were then classified into high and low-risk group according to the optimal cut-off for further analysis.
Evaluation of key immune characteristics and immune response predictor: TIDE, immunophenoscore and ESTIMATE
We downloaded values of key immune characteristics (41), including proliferation, wound healing, B-cell receptor (BCR) shannon, T-cell receptor (TCR) shannon, intratumor heterogeneity (ITH), cancer testis antigens (CTA) score, loss of heterozygosity (LOH; number of segments with LOH events, and fraction of bases with LOH events, respectively), homologous recombination deficiency(HRD), and mutation load (non-silent mutation) from the following website: https://gdc.cancer.gov/about-data/publications/panimmune, which is an extensive immunogenomic analysis of over 10,000 tumors comprising 33 diverse cancer types utilizing data compiled by TCGA. The values of clonal neoantigen and subclonal neoantigen were obtained from https://tcia.at. The Tumor Immune Dysfunction and Exclusion (42,43) (TIDE, http://tide.dfci.harvard.edu/) algorithm, which integrates tumor immune evasion mechanism including T cell dysfunction and T cell exclusion, was validated to be a superior predictor of immunotherapy response. The TIDE score was obtained after uploading the gene expression file as the instruction. Immunophenoscore (37) (IPS, https://tcia.at), calculated based on a panel of immune-related genes, was another outstanding immune response molecular marker. The Estimation of Stromal and Immune Cells in Malignant Tumors using Expression Data (44) (ESTIMATE, https://bioinformatics.mdanderson.org/estimate/index.html) website provides the stromal score, immune score, and ESTIMATE score of samples in TCGA database. We downloaded the data of TCGA-LUAD cohort to predict the level of infiltrating immune and stromal cells and tumor purity between subgroups.
Drug sensitivity prediction
The chemotherapeutic response of each patient was predicted based on the Genomics of Drug Sensitivity in Cancer (45) (GDSC, https://www.cancerrxgene.org) database. The patients’ half-maximal inhibitory concentration (IC50) to each chemotherapy drug was quantified using the “pRRophetic” R package (46).
Immunohistochemistry and evaluation
IHC was performed to investigate the expression of GBP1 and GBP2 in human LUAD. After deparaffinization, rehydration, the tissue microarray (TMA) slides were incubated overnight at 4 °C using primary antibodies against GBP1 (1:200, proteintech), GBP2 (1:200, proteintech), IRF8 (1:100, proteintech), and NLRC3 (1:100, Bioss) respectively. The slides were then incubated with corresponding secondary antibody and visualized with DAKO EnVision Detection System. The immunohistochemical staining was scored based on the staining intensity and percentage of positive cells. Signal intensity was scored as 0 (negative), 1 (weak), 2 (moderate) or 3 (strong) and the expression proportion was scored as 1 (0–25%), 2 (26–50%), 3 (51–75%) or 4 (76–100%). The intensity and proportion scores were then multiplied to obtain a final immunostaining score of each tissue. All the immune-stained sections were scored independently and blindly by two pathologists.
Statistical analysis
In this study, data were analyzed using the R-3.6.1 and GraphPad Prism 8.0 software. Comparison between two groups was calculated using Wilcoxon rank-sum test, while three group comparison was estimated by Kruskal-Wallis test. Spearman’s correlation analysis was used to calculate the correlation coefficient (47). The surv_cutpoint function in “survminer” R package was applied to determine the cutoff point and patients were stratified into high- and low-risk groups based on the optimal cutoff value. We then used the Kaplan-Meier survival analysis to generate the survival curves and determined the significance of the differences via the log-rank test. The receiver operating characteristic (ROC) curve, generated by the “timeROC” R package, was used to assess the specificity and sensitivity of the Pyroptosis score. Multivariate Cox regression analysis was utilized to evaluate the independent prognostic factor. The “maftools” (48) and “GenVisR” (49) R package was used to depict the mutation landscape of the TCGA-LUAD cohort. All statistical test was two-side and P<0.05 was considered statistically significant.
Results
Landscape of genetic and transcriptional variation of PRGs in LUAD
To characterize pyroptosis patterns and screen out potential targets, we developed a framework among 915 LUAD samples as the workflow shown in Figure S1A. Public gene-expression data and full clinical annotation were collected in Cancer Genome Atlas (TCGA) database and Gene-Expression Omnibus (GEO). After removing the patients without survival information, 500 LUAD patients from TCGA and 3 eligible LUAD cohorts (GSE30219, GSE31210 and GSE37745) were enrolled in this study for further analysis. In this study, after reviewing the literature, we identified 48 genes that mainly regulate pyroptosis in 4 pathways: two inflammasome dependent pathways including the canonical and non-canonical pathways, and two inflammasome independent manner including caspase-3 mediated pathway and granzymes proteases mediated pathway. Figure 1A depicts the cross-talks between tumors and immune cells under a potential influence of pyroptosis, as well as a summary of tumor progression mediated by pyroptosis including tumor growth, metastasis, and angiogenesis (29,50-52). GO enrichment analysis of 48 PRGs was conducted, and pyroptosis related biological processes were significantly enriched such as interleukin-1 beta (IL-1β) production, positive regulation of cytokine production pathways, summarized in Figure S1B.
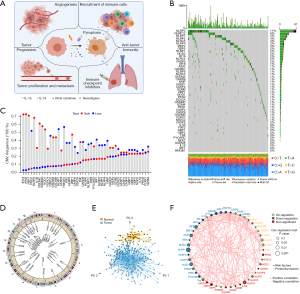
We first determined the prevalence of copy number variations and somatic mutations of 48 PRGs in LUAD. Among TCGA LUAD samples, NLRP3 showed the highest mutation frequency (11%), followed by NLRP12, NLRP7 and NLRP2 (Figure 1B). We also examined mutation co-occurrence across all PRGs and found the significant mutation co-occurrence relationships between GBP1 and NLRP7, NOD1 and NLRP12, CASP4 and NLRP3, as well as ZBP1 and GBP5 (Figure S1C). Further analysis of 48 PRGs revealed that CNV alterations were prevalent. AIM2, GSDMA, GSDMB, GSDMC, ZBP1, and CASP8 showed widespread CNV amplification. In contrast, PKN1, IRF2, DDX3X, and NAIP had prevalent CNV deletions (Figure 1C). The locations of CNV alterations of 48 PRGs on chromosomes were shown in Figure 1D. We also performed principal component analysis (PCA) based on tumor and normal specimens and found that the 48 PRGs completely distinguished LUAD samples from normal samples (Figure 1E). These analyses demonstrated a high heterogeneity of genetic landscape and expression of PRGs between LUAD and normal samples.
Furthermore, we explored the correlation of mRNA expression among PRGs. The comprehensive landscape of the interactions of the 48 PRGs and their prognostic significance in LUAD patients were illustrated in Figure 1F, which showed significant correlations among PRGs. A univariate Cox regression model showed that several PRGs (e.g., HMGB1 and CASP3) recognized as risk factors are related to poor prognosis in LUAD patients. In contrast, other PRGs (e.g., NAIP and NLRC3) presented characteristics of tumor suppressors, correlated with favorable prognosis in LUAD patients (Figure 1F, Figure S1D, Table S4). The above findings demonstrated the significant differences and correlations in the genomic and transcriptomic landscape of PRGs between normal and LUAD samples. Therefore, the expression imbalance of PRGs has a crucial role in tumor initiation and development of LUAD.
Pyroptosis-related molecular patterns with distinct prognosis, biological processes and TME characteristics in LUAD
Based on the mRNA expression profiles of 48 PRGs in LUAD samples from TCGA database, the patients were classified into the three molecular patterns, termed as pyroptosis cluster 1, 2, 3 (C1, Cluster 1: n=213; C2, Cluster 2: n=159; C3, Cluster 3: n=128) by unsupervised clustering analysis (Figure 2A, Figure S2A-S2F). The three pyroptosis clusters with distinct clinical outcomes were established. Compared with C1 and C2, Patients in C3 exserted a significantly longer survival time (Figure 2B, P=0.0099). PCA analysis confirmed the cluster assignments (Figure 2C).
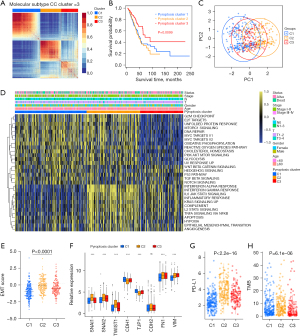
Furthermore, we evaluated the extensive biological functions and tumor immune microenvironment features among the three distinct pyroptosis patterns. We performed Gene Set Variation Analysis (GSVA) based on the hallmark gene sets and the known signatures to compare the differences of biological processes among the three clusters. As shown in Figure 2D, pyroptosis cluster 1 was enriched in stromal and carcinogenic activation pathways such as the MYC-TARGETS V1/V2, TGF-β pathway, and hedgehog signaling pathways, suggesting that pyroptosis cluster 1 may be associated with tumorigenesis, indicating malignant cell content was the highest in cluster 1. Pyroptosis cluster 3 was enriched in pathways associated with immune regulation but down-regulated in carcinogenic activation pathways. Meanwhile, pyroptosis cluster 2 was markedly enriched in both immune regulation and stromal-related signaling pathways such as interferon gamma/alpha response, IL-6/JAK/STAT3 pathway and EMT. Moreover, the pan-fibroblast TGF-β response signature (Pan-F-TBRS) pathway, as the measurement of TGF-β pathway activity specifically in fibroblasts, was the highest in C2, moderate in C3, and the lowest in C1, indicating C2 was significantly associated with stromal activation (Figure S2G). Therefore, we hypothesized that stromal activation in pyroptosis C2 inhibited the antitumor effect of immune cells. The activation of EMT in pyroptosis C2 (Figure 2D, Figure S2G) was also validated by the results of EMT score and EMT related genes (Figure 2E,2F). Most human solid tumors exhibit as three distinct immunological phenotypes: immune inflamed, immune excluded and immune desert (53). The stromal activity had been reported enhancing in immune excluded phenotype with the activation of EMT and TGF-β pathways (34), indicating pyroptosis C2 was mainly classified as immune-excluded phenotype. Similarly, pyroptosis C1 and C3 exhibited as immune desert and immune inflamed, respectively. Further, we evaluated the extent of infiltration of immune cells (Immune Score) and stromal cells (Stromal Score) across three distinct patterns. Further analyses revealed that pyroptosis C1, as immune desert, exhibited the lowest immune score compared with C2 and C3, meanwhile pyroptosis C1 had lower stromal score than C2 and C3, suggesting that pyroptosis C2 and C3 tumors contained more nontumor compositions, such as immune cells and stromal cells (Figure S2H,S2I).
To further validate our speculation, we compared the relative abundances of 28 immune infiltrating cell subpopulations among distinct pyroptosis molecular patterns (Figure S2J). Consistent with previous results, most immune infiltrating cell subpopulations, such as effector memory CD8+ T cells, activated CD4+/CD8+ T cells, NK cells, and regulatory T cells were markedly enriched in the pyroptosis C2 and C3, lowest in C1. In addition, considering that PD-L1 and TMB played as the well-established biomarkers for predicting the response of ICB, we also compared the PD-L1 expression and TMB level in different pyroptosis patterns and observed a significant increase in the pyroptosis C2 (Figure 2G,2H). Taken together, we confirmed that the three pyroptosis patterns were characterized by distinct TME characteristics, including immune cell infiltrate, immunogenicity and checkpoint expressions.
Pyroptosis gene subtypes correlated with distinct prognosis, immune landscape and EMT in LUAD
Although the consensus clustering algorithm based on pyroptosis expression classified LUAD patients into three pyroptosis phenotypes, patients with distinct patterns did not show a matching survival advantage (Figure 2B), and the underlying genetic and expression variations within these patterns were not well clarified. Thus, we further explored the potential PRGs transcriptional expression change across three pyroptosis patterns in LUAD. We first determined the overlapping DEGs)among the three pyroptosis patterns. A total of 470 DEGs that represented the critical distinguishing index of the three pyroptosis molecular patterns were identified and illustrated in a Venn diagram (Figure 3A, available online: https://cdn.amegroups.cn/static/public/tlcr-21-715-01.pdf), and Go analysis indicated that DEGs exhibit immune regulation pathways enrichment, such as T cell activation, regulation T cell of activation, positive regulation of cytokine activation and regulation of cell-to-cell adhesion (Figure S3A). These findings mirrored and confirmed that pyroptosis played a necessary role in regulation of adapted and innate immunity in TME. Based on the expression profiles of the 119 prognostic DEGs with an optimal k of 2 (Figure S3B-S3H), we divided 500 LUAD samples into two pyroptosis gene subtypes, termed as pyroptosis gene-S1 and pyroptosis gene-S2. And pyroptosis gene-S1 showed significantly longer survival than pyroptosis gene-S2 (Figure 3B). Moreover, the two subgroups exhibited discrepancy by PCA analysis (Figure 3C).
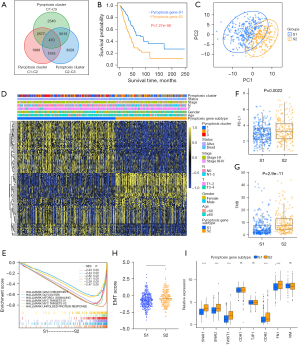
Furthermore, we explored the clinicopathological characteristics and biological processes between the two pyroptosis gene subtypes. We found that an advanced clinical stage and lymph node metastasis (N1-3) were mainly concentrated in patients of the pyroptosis gene-S2 subgroup (Figure 3D), reflecting the prognostic differences between the pyroptosis gene-S1 and S2 in LUAD. GSEA analysis showed that some critical hallmark gene sets including G2M checkpoints, glycolysis and MYC_targets were significantly enriched in pyroptosis gene-S2 (Figure 3E).
Additionally, patients in pyroptosis gene-S2 showed up-regulated PD-L1 expression and high TMB (Figure 3F,3G). To further explore the biological characteristics, we uncovered that pyroptosis S2 displayed the higher EMT score and increasing expression of EMT related genes (Figure 3H,3I, Figure S3I). Collectively, the coherence between the prognostic and biological features in the two gene subtypes indicated that this classification was reliable and reasonable.
Construction of the pyroptosis scoring system for LUAD and exploration of its clinical relevance
To accurately predict the patterns of pyroptosis in individual tumors, we developed a scoring system based on the identified PRGs. We first performed univariate Cox regression analysis for primary screening of the survival-related genes. The 13 genes (NLRP1, NLRC3, IRF8, NOD1, NAIP, HMGB1, CASP6, NLRC4, GBP2, GBP1, CASP3, NLRP3, IL18) that met the criteria of P<0.1 were retained for further analysis (Figure S1D). Next, the random survival forest algorithm was used to identify the most robust prognostic genes among the 13 prognostic PRGs (Figure S4A). The 6 prognostic genes with importance >0.005 were chosen to performed combination analysis. And finally, an ensemble of 4 genes obtained the minimum P value (Figure S4B), which were integrated to build a pyroptosis -related prognostic signature. The risk score was calculated as follows: Pyro score = (-0.2425 * expression value of IRF8) + (0.2271 * expression value of GBP1) + (0.1444 * expression value of GBP2) + (-0.3261 *expression value of NLRC3). Using the established formula, a pyroptosis-related prognostic risk score (Pyro score) for each sample was calculated in each cohort. As shown in Figure 4A,4B, patients with higher Pyro score exhibited worse overall survival in the TCGA cohort (HR =2.048, 95% CI: 1.529–2.743, P=1.428e-06). To further evaluate the prognostic and predictive capacities of Pyro score in depth, Area Under the Curves (AUCs) were quantified. AUCs for 2-, 3- and 5-year survival time were 0.640, 0.658 and 0.633, suggesting that Pyro score possessed a robust and reliable capacity for predicting the prognosis for LUAD patients (Figure 4C). Our results showed that in TCGA-LUAD cohort, Pyro score was significantly correlated to survival outcomes (P=7.7e-06), TNM stage (P=1.7e-05), T stage (P=0.0026), N stage (P=0.0004) and M stage (P=0.044) in Figure S4C. Moreover, multivariate cox regression analysis displayed that Pyro score was confirmed as an independent prognostic biomarker for evaluating LUAD patient outcomes [hazard ratio (HR): 2.343, 95% confidence interval (CI): 1.585–3.465, P<0.001; Figure 4D].
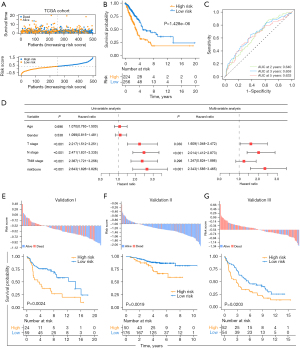
In order to further test the stability of Pyro score model, the prognostic value of Pyro score was validated in three independent GEO cohorts (Validation I: HR =2.438, 95% CI: 1.217–4.884, P=0.0024; Validation II: HR =2.780, 95% CI: 1.202–6.432, P=0.0019; Validation III: HR =1.687, 95% CI: 1.072–2.654, P=0.0203; Figure 4E-4G). We also validated the prognostic effects of Pyro score genes (GBP1, GBP2, IRF8 and NLRC3) in our NCC cohort (n=142) by IHC (Figure 5A-5D). GBP1 and GBP2 levels also grew along with stage and N stage of LUAD, portending a worse prognosis (Figure 5E-5G). The patients with lower GBP1 and GBP2 protein expression or with higher IRF8 and NLRC3 protein expression showed longer survival of LUAD patients (Figure 5H), which were confirmed in TCGA cohort, Validation I, II, III cohorts, respectively (Figure S4D-S4G). To determine the utility of Pyro score in clinical practice, we stratified patients in NCC cohort into high and low Pyro score subgroups, and as expected, the low Pyro score subgroup presented longer OS (Figure 5I, P=0.0308). Collectively, Pyro score may serve as a promising and accurate prognostic factor for LUAD patients.
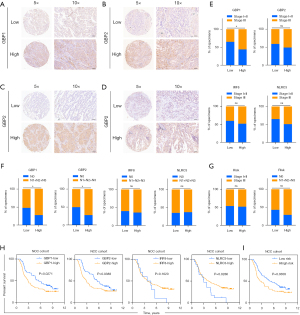
To better illustrate the crosstalk of Pyro score and pyroptosis patterns, the alluvial diagram was used to visualize the attribute changes of individual patients (Figure 6A). Intriguingly, almost pyroptosis S2 patients were composed of C1 (44.3%, characterized as immune desert subtype) and C2 (48.6%, characterized as immune excluded), which correlated with worse prognosis (Figure 6A). Compared with Pyroptosis C1 and C3, Pyroptosis C2 showed the highest median score which indicated Pyroptosis C2 is correlated with poor prognosis (Figure 6B). Meanwhile Pyroptosis gene S2 had the higher median score than Pyroptosis gene S1, indicating that high Pyro score could be linked to stromal activation related signatures (Figure 6C). Box plots showed the expression distribution of 4 genes (IRF8, NLRC3, GBP1, GBP2) across three pyroptosis patterns and pyroptosis gene subgroups (Figure S5A,S5B). And we also compared the expression of 4 genes (IRF8, NLRC3, GBP1, GBP2) between high and low pyro score groups, the results showed that the expressions of IRF8, NLRC3 decreased, in contrast GBP1 and GBP2 elevated in high-risk groups (Figure S5C).
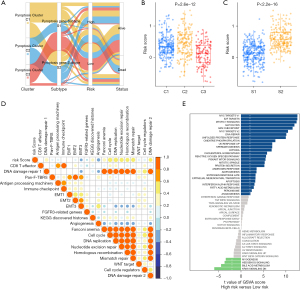
To further analysis the Pyro score related biological processes, we first evaluated the DEGs between the distinct Pyro score groups. With a threshold of FDR <0.05 and | log2FC | >1,209 significantly upregulated genes and 532 significantly downregulated genes were identified in TCGA cohort (Figure S5D). Subsequently, Gene Ontology enrichment analysis showed that DEGs were mainly enriched in immune regulation pathways, such as immune response, myeloid leukocyte migration, leukocyte chemotaxis and B cell receptor pathway (Figure S5E). GSVA enrichment analysis of known signatures and hallmark gene sets was also performed, which also revealed distinct biological processes between high- and low-risk group (Figure 6D,6E).
The role of Pyro score in immunotherapy
Accumulated evidence showed ICIs treatment represented by CTLA-4/PD-(L)1 inhibitors has undoubtedly revolutionized antitumor therapy of LUAD. To demonstrated the role of Pyro score in ICIs treatment, we first explored the relationships between Pyro score and TMB, which reflects immunogenicity and serves as a predictor of ICB. As a result, the TMB presented a pronounced elevation in the high Pyro score group, and Pyro score was positively correlated with TMB (r=0.3, P<0.0001), as shown in Figure 7A,7B. Next, we considered Pyro score in combinations with TMB or immune checkpoints expression to assess whether Pyro score influences OS in patients with similar immune checkpoints expression. Survival analyses of the four groups stratified by Pyro score, TMB or immune checkpoint genes expression were conducted. As depicted in Figure 7C,7D, patients with low TMB and high Pyro score had worst prognosis. Meanwhile patients with low PD-L1 and low Pyro score had prolonged OS compared to those with low PD-L1 and high Pyro score (P=0.0074). Among patients with high PD-L1 expression, a lower Pyro score signified a remarkably better survival (P=0.0003). Similar survival patterns were also observed among the four patient groups stratified by Pyro score and PD-1 or CTLA4 expression in TCGA cohort (Figure 7E,7F). Consistent with TCGA dataset, patients with low Pyro scores had significantly better survival than those with high Pyro-score scores regardless of the expression level of immune checkpoint genes in validation I, II, III cohorts (Figure S6A-S6C). These results confirmed that the combinations of Pyro score and TMB or immune checkpoint expression showed better prognosis stratifications than each biomarker alone.
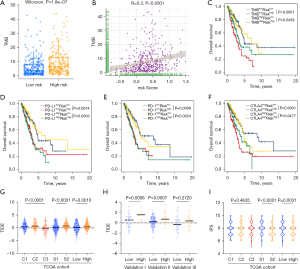
In addition to well-known predictors for ICIs, newly identified predictors, such as TIDE and IPS, are widely used and strongly recommended to evaluate the immune response and immune evasion. Our analysis also revealed that the TIDE was significantly decreased in the low Pyro score group, and IPS was significantly elevated in the low Pyro score group (Figure 7G-7I). Therefore, the above results indirectly demonstrated that the difference in tumor pyroptosis patterns could play a crucial role in mediating the clinical response to ICIs treatment.
Additionally, to further screen out potential small molecule compounds besides ICB for the treatment of LUAD, we compared the estimated IC50 levels of 138 drugs between high and low Pyro score groups in Genomics of Drug Sensitivity in Cancer (GDSC) database. As shown in Figure S7A, the top 15 drugs had distinctly higher estimated IC50 values in the high Pyro score group compared to the low Pyro score group, indicating that low Pyro score patients were more sensitive to these small molecule drugs (such as GTPases inhibitor, DNA synthesis inhibitor, PARP inhibitor, and IGF-1R pathway inhibitor).
Potential mechanisms associated with pyroptosis patterns, Pyro score in predicting the efficacy of immunotherapy
Considering the previously identified correlations of PD-L1 expression and TMB with the clinical benefits of ICIs, the associations of pyroptosis with the tumor immunogenicity and anti-tumor immunity were analyzed to investigate the possible mechanisms among pyroptosis patterns, pyroptosis gene subtypes and between high and low Pyro score tumors. Immune modulators (IMs) are critical for tumor immunotherapy including numerous IM agonists and antagonists (54). To deeply understand their expression and modes of control in different states of the pyroptosis, we evaluated IM genes expression in distinct pyroptosis patterns, pyroptosis genes subtypes and high/low Pyroscore groups. Gene expression of IMs (Figure 8A) varied across pyroptosis patterns. In line with immune infiltration and signatures, many immunomodulators were generally downregulated in the Pyro score-high tumors, such as co-stimulator (CD80, CD28, ICOSLG), antigen presentation related genes (HLA-DRA, HLA-DRB1, HLA-DPB1, HLA-DPA1) and cytolytic activity associated gene (PRF1), indicating Pyroscore high tumors exhibit immune escape through antigen-presentation machinery dysfunction and T cell dysfunction. Additionally, IMs showed differences between subtypes including CD274, CD276, CD70, CXCL9, CXCL10, GZMA, HAVCR2, HMGB1, IFNG and IL1B, most highly expressed in S2. The observed differences in regulation of IMs might have implications for immunotherapeutic and combination strategies.
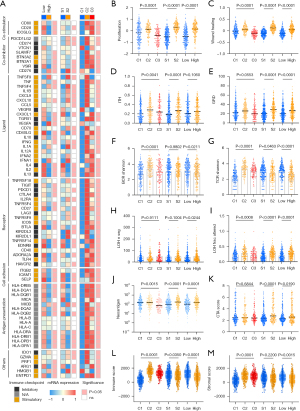
As shown in Figure 8B-8K, pyroptosis C2 and S2 had the highest proliferation rate, ITH, HRD, as well as a high neoantigen load, indicating a higher heterogeneity leading to poor prognosis, which were consistent with previous findings. According to the level of Pyro score, as expected, the high Pyro score group showed higher proliferation rate, ITH, HRD, and a high neoantigen load, as well as subclonal neoantigen fraction than in the low Pyro score group (Figure 8B-8K, Figure S7B).
Additionally, we used the ESTIMATE algorithm to quantify the overall infiltration of immune cells (Immune score) and stromal cells (Stromal score) across three pyroptosis patterns. Patients in high Pyro score group showed lower immune score and ESTIMATE score (Figure 8L-8M, Figure S7C). Taken together, these findings indirectly demonstrated that the representation of tumor pyroptosis patterns plays a crucial role in mediating the immune response, and Pyro score was associated with the response to immunotherapies and can further predict the prognosis of patients.
Discussion
Growing evidence displayed that pyroptosis has a wide-ranging impact on various biological processes including inflammation, innate immunity, metabolism, therapeutic resistance (5). In recent years, cancer immunotherapy based on ICIs has achieved considerable success in clinic (11). However, ICIs are significantly limited by the fact that only one third of patients with most types of cancer respond to these agents (55). The induction of immune cell death mechanisms has gradually emerged as a new cancer treatment strategy. However, to date, the possibility of combining these two modalities has not been discussed systematically. Additionally, the effects of pyroptosis on LUAD progression remain unclear, and studies on the biological mechanisms as well as prognostic biomarkers of LUAD concerning PRGs are still limited. In the present study, we were inspired by identifying the role of distinct pyroptosis patterns in the tumor immune landscape of LUAD and constructed an independent model to assess the efficacy of PRGs in individual patients, contributing to the personalized immunotherapy strategies.
As the crosstalk of cancer, pyroptosis and the immune environment is largely underexplored in LUAD patients, it is important to gain more extensive insight into their interactions. In this study, our results can be summarized as follows: (I) we identified three distinct pyroptosis patterns characterized by different biological process and immune phenotypes, which were correlated with diverse pro- and anti-tumor immunity; (II) the pyroptosis patterns featured by distinct immune cell infiltration characterization, expression of immunomodulatory genes, extent of ITH, HRD score, TCR/BCR diversity, EMT score and prognosis; (III) accordingly, the DEGs between different patterns were enriched in immune-related biological processes and pathways. And two gene clusters with distinct clinical outcomes and immune characteristics were proposed for LUAD. This demonstrated again that the pyroptosis was of great significance in shaping different TME landscapes (IV); by random forest algorithm, Pyro score was constructed for accurately evaluating prognosis of individual LUAD patients. Intriguingly, patients with high Pyro score usually experienced shorter survival time, with higher proliferation rate, extent of ITH, and HRD score (V); as expected, Pyro score showed significant correlations with PD-L1, TMB and other biomarkers of immunotherapy, including IPS and TIDE, indicating that Pyro score possessed the potential to predict the responsiveness to immunotherapy. Overall, our study provides insights into pyroptosis as the crucial role in the regulation of the immune microenvironment in LUAD and highlights various potential immunotherapeutic targets.
Previous studies demonstrated that the tumor microenvironment contexture plays a crucial role in tumor progression and immunotherapeutic efficacy (41). Intriguingly, in our study, the observed pyroptosis patterns share multiple similarities with previous study that the stromal component refines the immune clusters into distinct two subtypes (IE/F and IE), which are strikingly different in immunosuppressive profile and prognosis (56). Similarly, we observed both immune regulation and stromal-related signaling pathways activating in pyroptosis C2. Recent studies showed that the activation of TGF-β and EMT related pathways impeded the penetration of lymphocyte cells into the tumor parenchyma as well as weakened tumor killing effects (34). The co-existence of immune infiltration cells and stromal context in immune-excluded tumors and the relevance of TGF-β signaling in this pattern may be the driver due to its impact on stromal cells. And TGF-β is a pleiotropic cytokine associated with poor prognosis in cancers, which plays as a pro-tumorigenic factor in cancers through promoting immunosuppression, EMT, fibroblast activation, angiogenesis and metastasis of tumors (57,58) . Consistent with previous reports, pyroptosis C2 was characterized by a significant stroma activation status, including the highly expressed angiogenesis, EMT and TGF-β pathways, corresponding to immune-excluded phenotype, with the highest ITH, EMT score, proliferation, suggesting worst prognosis. Specific molecular inhibitors targeting TGF-β have been shown to reshape the tumor microenvironment (e.g., reprogram peritumoral stromal fibroblasts) and restore the anti-tumor immunity (59). Based on these findings, we speculated that LUAD patients with the pyroptosis-C2 pattern may benefit from combinations with ICB and anti-TGFβ therapies, which needs to be validated in mouse model and clinical settings in the future.
Gasdermin E (GSDME) and gasdermin A3 (GSDMA3) as tumor suppressors by activating pyroptosis were reported to enhance functional properties of tumor-infiltrating NK and CD8+ T killer lymphocytes in cancers (5,6). On the contrary, the activation of pyroptosis could lead to the release of inflammasome such as IL-1 and IL-18, which could promote tumorigenesis (4,60). Given the extensive heterogeneity of pyroptosis, we also constructed a scoring system to accurately assess the efficacy of pyroptosis related genes in individual LUAD patients, defined as Pyro score. Pyro score, constructed based on four genes (NLRC3, IRF8, GBP1 and GBP2), was an independent prognostic factor for OS. Moreover, the four genes also involved in immune-related regulation. NLRC3 is a key transcriptional coactivator of MHC class I genes. Reduced NLRC3 expression is associated with impaired CD8+ T-cell activation and immune evasion in cancers (61). GBP1and GBP2, as the most interferon-induced genes in the GTPase superfamily, participates in membrane, cytoskeleton and cell verification reactions. GBP1 facilitates extracellular secretion of IDO1 to inhibit the activity of T cells, and its expression level is related to the poor prognosis of NSCLC patients (62), while GBP2 promotes glioblastoma tumor growth and invasion in vivo and in vitro (63). Herein, immunogenetic characteristics such as TCR/BCR diversity, IMs, IPS, TIDE scores were utilized to quantify immunogenicity and response of ICIs for LUAD patients. Notably, the patients with high Pyro score markedly exhibited high TIDE score as well as high ITH score, which was characterized by poor prognosis (41), accompanied by low immune score and lower IPS scores. Intriguingly, the highest Pyroscore was observed in C2 and S2, which exhibited activation of EMT and TGF-β pathway, underlying the core role of stromal activation in resistance to checkpoint immunotherapy in high Pyroscore group. This indicated that suppressive factors including angiogenesis, fibroblast activation, EMT and TGF-β pathway components may play a key role in the resistance to immunotherapy.
In clinical practice, our results provide novel insights in personalized treatment of LUAD patients. On one hand, comprehensive analyses revealed that Pyro score is a robust and independent prognostic biomarker for LUAD. Significantly prolonged survival was observed for patients with low Pyro score, suggesting that high Pyro score patients should receive more frequent clinical surveillance and corresponding measures to prevent disease recurrence and progression. On the other hand, our data also displayed a markedly correlation between Pyro score and TMB as well as PD-L1. Combinations of Pyro score, TMB and PD-L1 could be applied not only as prognostic stratification tools but also as more refined predictive biomarkers for personalized immunotherapy treatment. Besides, previous studies have demonstrated that many clinical drugs induce and regulate pyroptotic pathways and inhibit tumor growth including the doxorubicin, cisplatin, topotecan, etoposide and BRAF inhibitors/MEK inhibitors (29), which could reverse immunosuppressive microenvironment and reestablish local or systemic anti-tumor immunity (64). Therefore, comprehensive explorations of pyroptosis and the corresponding TME characterization within individual patient aid identifying the immune phenotypes of tumors and guide the more accurate clinical practice.
In spite of some new perspectives on pyroptosis-immune-oncology crosstalk in LUAD, several limitations still remained in our study. First, our pyroptosis signature was constructed based on public datasets. Besides, we used the IPS, TIDE values to mimic patients’ response to ICI treatment. which have been proved correlated with response to ICI therapy in several independent datasets. The predictive capability needs further verification in LUAD patients with immunotherapy. Second, the regulatory mechanisms of PRGs remodeling the TIME need to be further investigated in vivo and in vitro.
In conclusion, we comprehensively analyzed the pyroptosis landscape and the extensive regulation mechanisms of pyroptosis on tumor microenvironment in LUAD. The difference of pyroptosis patterns enhanced our understanding of the tumor heterogeneity and complexity of TIME, and provided the distinct implications of the clinical outcomes. We also constructed Pyroscore model to document the crosstalk and regulatory roles of the pyroptosis related genes and identify their utility in immunotherapy. Consequently, our improved understanding of pyroptosis and their interconnectivity with adaptive immunity may pave the way to the personalized immunotherapy strategies for LUAD patients.
Acknowledgments
The authors would like to thank the staff members of the TCGA Research Network, the GEO data as well as all the investigators for making their valuable research data public. Figure 1A is created with BioRender.com.
Funding: This work was supported by National Key R&D Program of China (2020AAA0109500), the Beijing Municipal Science & Technology Commission (Z191100006619116), R&D Program of Beijing Municipal Education commission (KJZD20191002302), CAMS Initiative for Innovative Medicine (2021-1-I2M-012, 2021-1-I2M-015), Non-profit Central Research Institute Fund of Chinese Academy of Medical Sciences (2021-PT310-001), and Aiyou Foundation (KY201701).
Footnote
Reporting Checklist: The authors have completed the MDAR reporting checklist. Available at https://dx.doi.org/10.21037/tlcr-21-715
Conflicts of Interest: All authors have completed the ICMJE uniform disclosure form (available at https://dx.doi.org/10.21037/tlcr-21-715). The authors have no conflicts of interest to declare.
Ethical Statement: The authors are accountable for all aspects of the work in ensuring that questions related to the accuracy or integrity of any part of the work are appropriately investigated and resolved. The study was conducted in accordance with the Declaration of Helsinki (as revised in 2013). All procedures involving human participants were reviewed and approved by The Medical Ethics Committee of Cancer Hospital, Chinese Academy of Medical Sciences (NCC2017ZDXM-001) and individual consent for this retrospective analysis was waived.
Open Access Statement: This is an Open Access article distributed in accordance with the Creative Commons Attribution-NonCommercial-NoDerivs 4.0 International License (CC BY-NC-ND 4.0), which permits the non-commercial replication and distribution of the article with the strict proviso that no changes or edits are made and the original work is properly cited (including links to both the formal publication through the relevant DOI and the license). See: https://creativecommons.org/licenses/by-nc-nd/4.0/.
References
- Hanahan D, Weinberg RA. Hallmarks of cancer: the next generation. Cell 2011;144:646-74. [Crossref] [PubMed]
- Fink SL, Cookson BT. Pillars Article: Caspase-1-dependent pore formation during pyroptosis leads to osmotic lysis of infected host macrophages. Cell Microbiol. 2006. 8: 1812-1825. J Immunol 2019;202:1913-26. [PubMed]
- Grivennikov SI, Greten FR, Karin M. Immunity, inflammation, and cancer. Cell 2010;140:883-99. [Crossref] [PubMed]
- Hu B, Elinav E, Huber S, et al. Inflammation-induced tumorigenesis in the colon is regulated by caspase-1 and NLRC4. Proc Natl Acad Sci U S A 2010;107:21635-40. [Crossref] [PubMed]
- Wang Q, Wang Y, Ding J, et al. A bioorthogonal system reveals antitumour immune function of pyroptosis. Nature 2020;579:421-6. [Crossref] [PubMed]
- Zhang Z, Zhang Y, Xia S, et al. Gasdermin E suppresses tumour growth by activating anti-tumour immunity. Nature 2020;579:415-20. [Crossref] [PubMed]
- Siegel RL, Miller KD, Fuchs HE, et al. Cancer Statistics, 2021. CA Cancer J Clin 2021;71:7-33. [Crossref] [PubMed]
- Sung H, Ferlay J, Siegel RL, et al. Global Cancer Statistics 2020: GLOBOCAN Estimates of Incidence and Mortality Worldwide for 36 Cancers in 185 Countries. CA Cancer J Clin 2021;71:209-49. [Crossref] [PubMed]
- Miller KD, Nogueira L, Mariotto AB, et al. Cancer treatment and survivorship statistics, 2019. CA Cancer J Clin 2019;69:363-85. [Crossref] [PubMed]
- Dafni U, Tsourti Z, Vervita K, et al. Immune checkpoint inhibitors, alone or in combination with chemotherapy, as first-line treatment for advanced non-small cell lung cancer. A systematic review and network meta-analysis. Lung Cancer 2019;134:127-40. [Crossref] [PubMed]
- Gandhi L, Rodríguez-Abreu D, Gadgeel S, et al. Pembrolizumab plus Chemotherapy in Metastatic Non-Small-Cell Lung Cancer. N Engl J Med 2018;378:2078-92. [Crossref] [PubMed]
- Ren D, Hua Y, Yu B, et al. Predictive biomarkers and mechanisms underlying resistance to PD1/PD-L1 blockade cancer immunotherapy. Mol Cancer 2020;19:19. [Crossref] [PubMed]
- Gerlinger M, Rowan AJ, Horswell S, et al. Intratumor heterogeneity and branched evolution revealed by multiregion sequencing. N Engl J Med 2012;366:883-92. [Crossref] [PubMed]
- Zhou Z, He H, Wang K, et al. Granzyme A from cytotoxic lymphocytes cleaves GSDMB to trigger pyroptosis in target cells. Science 2020;368:eaaz7548. [Crossref] [PubMed]
- Pardo J, Wallich R, Martin P, et al. Granzyme B-induced cell death exerted by ex vivo CTL: discriminating requirements for cell death and some of its signs. Cell Death Differ 2008;15:567-79. [Crossref] [PubMed]
- Xi G, Gao J, Wan B, et al. GSDMD is required for effector CD8+ T cell responses to lung cancer cells. Int Immunopharmacol 2019;74:105713. [Crossref] [PubMed]
- Yamazaki T, Hannani D, Poirier-Colame V, et al. Defective immunogenic cell death of HMGB1-deficient tumors: compensatory therapy with TLR4 agonists. Cell Death Differ 2014;21:69-78. [Crossref] [PubMed]
- Balahura LR, Selaru A, Dinescu S, et al. Inflammation and Inflammasomes: Pros and Cons in Tumorigenesis. J Immunol Res 2020;2020:2549763. [Crossref] [PubMed]
- Baker KJ, Houston A, Brint E. IL-1 Family Members in Cancer; Two Sides to Every Story. Front Immunol 2019;10:1197. [Crossref] [PubMed]
- Rousseaux S, Debernardi A, Jacquiau B, et al. Ectopic activation of germline and placental genes identifies aggressive metastasis-prone lung cancers. Sci Transl Med 2013;5:186ra66. [Crossref] [PubMed]
- Okayama H, Kohno T, Ishii Y, et al. Identification of genes upregulated in ALK-positive and EGFR/KRAS/ALK-negative lung adenocarcinomas. Cancer Res 2012;72:100-11. [Crossref] [PubMed]
- Botling J, Edlund K, Lohr M, et al. Biomarker discovery in non-small cell lung cancer: integrating gene expression profiling, meta-analysis, and tissue microarray validation. Clin Cancer Res 2013;19:194-204. [Crossref] [PubMed]
- Detterbeck FC. The eighth edition TNM stage classification for lung cancer: What does it mean on main street? J Thorac Cardiovasc Surg 2018;155:356-9.
- Karki R, Kanneganti TD. Diverging inflammasome signals in tumorigenesis and potential targeting. Nat Rev Cancer 2019;19:197-214. [Crossref] [PubMed]
- Christgen S, Place DE, Kanneganti TD. Toward targeting inflammasomes: insights into their regulation and activation. Cell Res 2020;30:315-27. [Crossref] [PubMed]
- Fernandes FP, Leal VNC, Souza de Lima D, et al. Inflammasome genetics and complex diseases: a comprehensive review. Eur J Hum Genet 2020;28:1307-21. [Crossref] [PubMed]
- Downs KP, Nguyen H, Dorfleutner A, et al. An overview of the non-canonical inflammasome. Mol Aspects Med 2020;76:100924. [Crossref] [PubMed]
- Orning P, Lien E, Fitzgerald KA. Gasdermins and their role in immunity and inflammation. J Exp Med 2019;216:2453-65. [Crossref] [PubMed]
- Tan Y, Chen Q, Li X, et al. Pyroptosis: a new paradigm of cell death for fighting against cancer. J Exp Clin Cancer Res 2021;40:153. [Crossref] [PubMed]
- Xia X, Wang X, Cheng Z, et al. The role of pyroptosis in cancer: pro-cancer or pro-"host"? Cell Death Dis 2019;10:650. [Crossref] [PubMed]
- Xu T, Le TD, Liu L, et al. CancerSubtypes: an R/Bioconductor package for molecular cancer subtype identification, validation and visualization. Bioinformatics 2017;33:3131-3. [Crossref] [PubMed]
- Hänzelmann S, Castelo R, Guinney J. GSVA: gene set variation analysis for microarray and RNA-seq data. BMC Bioinformatics 2013;14:7. [Crossref] [PubMed]
- Subramanian A, Tamayo P, Mootha VK, et al. Gene set enrichment analysis: a knowledge-based approach for interpreting genome-wide expression profiles. Proc Natl Acad Sci U S A 2005;102:15545-50. [Crossref] [PubMed]
- Mariathasan S, Turley SJ, Nickles D, et al. TGFβ attenuates tumour response to PD-L1 blockade by contributing to exclusion of T cells. Nature 2018;554:544-8. [Crossref] [PubMed]
- The Gene Ontology Consortium. The Gene Ontology Resource: 20 years and still GOing strong. Nucleic Acids Res 2019;47:D330-8. [Crossref] [PubMed]
- Yu G, Wang LG, Han Y, et al. clusterProfiler: an R package for comparing biological themes among gene clusters. OMICS 2012;16:284-7. [Crossref] [PubMed]
- Charoentong P, Finotello F, Angelova M, et al. Pan-cancer Immunogenomic Analyses Reveal Genotype-Immunophenotype Relationships and Predictors of Response to Checkpoint Blockade. Cell Rep 2017;18:248-62. [Crossref] [PubMed]
- Mak MP, Tong P, Diao L, et al. A Patient-Derived, Pan-Cancer EMT Signature Identifies Global Molecular Alterations and Immune Target Enrichment Following Epithelial-to-Mesenchymal Transition. Clin Cancer Res 2016;22:609-20. [Crossref] [PubMed]
- Chen H, Yao J, Bao R, et al. Cross-talk of four types of RNA modification writers defines tumor microenvironment and pharmacogenomic landscape in colorectal cancer. Mol Cancer 2021;20:29. [Crossref] [PubMed]
- Ishwaran H, Kogalur U. Fast Unified Random Forests for Survival, Regression, and Classification (RF-SRC). R package version 2.12.0. Available online: https://cran.r-project.org/package=randomForestSRC. 2021.
- Thorsson V, Gibbs DL, Brown SD, et al. The Immune Landscape of Cancer. Immunity 2018;48:812-830.e14. [Crossref] [PubMed]
- Fu J, Li K, Zhang W, et al. Large-scale public data reuse to model immunotherapy response and resistance. Genome Med 2020;12:21. [Crossref] [PubMed]
- Jiang P, Gu S, Pan D, et al. Signatures of T cell dysfunction and exclusion predict cancer immunotherapy response. Nat Med 2018;24:1550-8. [Crossref] [PubMed]
- Yoshihara K, Shahmoradgoli M, Martínez E, et al. Inferring tumour purity and stromal and immune cell admixture from expression data. Nat Commun 2013;4:2612. [Crossref] [PubMed]
- Yang W, Soares J, Greninger P, et al. Genomics of Drug Sensitivity in Cancer (GDSC): a resource for therapeutic biomarker discovery in cancer cells. Nucleic Acids Res 2013;41:D955-61. [Crossref] [PubMed]
- Geeleher P, Cox N, Huang RS. pRRophetic: an R package for prediction of clinical chemotherapeutic response from tumor gene expression levels. PLoS One 2014;9:e107468. [Crossref] [PubMed]
- Hazra A, Gogtay N. Biostatistics Series Module 3: Comparing Groups: Numerical Variables. Indian J Dermatol 2016;61:251-60. [Crossref] [PubMed]
- Mayakonda A, Lin DC, Assenov Y, et al. Maftools: efficient and comprehensive analysis of somatic variants in cancer. Genome Res 2018;28:1747-56. [Crossref] [PubMed]
- Skidmore ZL, Wagner AH, Lesurf R, et al. GenVisR: Genomic Visualizations in R. Bioinformatics 2016;32:3012-4. [Crossref] [PubMed]
- Hsu SK, Li CY, Lin IL, et al. Inflammation-related pyroptosis, a novel programmed cell death pathway, and its crosstalk with immune therapy in cancer treatment. Theranostics 2021;11:8813-35. [Crossref] [PubMed]
- Ju X, Yang Z, Zhang H, et al. Role of pyroptosis in cancer cells and clinical applications. Biochimie 2021;185:78-86. [Crossref] [PubMed]
- Tang R, Xu J, Zhang B, et al. Ferroptosis, necroptosis, and pyroptosis in anticancer immunity. J Hematol Oncol 2020;13:110. [Crossref] [PubMed]
- Herbst RS, Soria JC, Kowanetz M, et al. Predictive correlates of response to the anti-PD-L1 antibody MPDL3280A in cancer patients. Nature 2014;515:563-7. [Crossref] [PubMed]
- Tang J, Shalabi A, Hubbard-Lucey VM. Comprehensive analysis of the clinical immuno-oncology landscape. Ann Oncol 2018;29:84-91. [Crossref] [PubMed]
- Samstein RM, Lee CH, Shoushtari AN, et al. Tumor mutational load predicts survival after immunotherapy across multiple cancer types. Nat Genet 2019;51:202-6. [Crossref] [PubMed]
- Bagaev A, Kotlov N, Nomie K, et al. Conserved pan-cancer microenvironment subtypes predict response to immunotherapy. Cancer Cell 2021;39:845-865.e7. [Crossref] [PubMed]
- David CJ, Massagué J. Contextual determinants of TGFβ action in development, immunity and cancer. Nat Rev Mol Cell Biol 2018;19:419-35. [Crossref] [PubMed]
- Batlle E, Massagué J. Transforming Growth Factor-β Signaling in Immunity and Cancer. Immunity 2019;50:924-40. [Crossref] [PubMed]
- Liu S, Ren J, Ten Dijke P. Targeting TGFβ signal transduction for cancer therapy. Signal Transduct Target Ther 2021;6:8. [Crossref] [PubMed]
- Dupaul-Chicoine J, Yeretssian G, Doiron K, et al. Control of intestinal homeostasis, colitis, and colitis-associated colorectal cancer by the inflammatory caspases. Immunity 2010;32:367-78. [Crossref] [PubMed]
- Gültekin Y, Eren E, Özören N. Overexpressed NLRC3 acts as an anti-inflammatory cytosolic protein. J Innate Immun 2015;7:25-36. [Crossref] [PubMed]
- Meng Y, Wang W, Chen M, et al. GBP1 Facilitates Indoleamine 2,3-Dioxygenase Extracellular Secretion to Promote the Malignant Progression of Lung Cancer. Front Immunol 2021;11:622467. [Crossref] [PubMed]
- Yu S, Yu X, Sun L, et al. GBP2 enhances glioblastoma invasion through Stat3/fibronectin pathway. Oncogene 2020;39:5042-55. [Crossref] [PubMed]
- Erkes DA, Cai W, Sanchez IM, et al. Mutant BRAF and MEK Inhibitors Regulate the Tumor Immune Microenvironment via Pyroptosis. Cancer Discov 2020;10:254-69. [Crossref] [PubMed]