Implementation of artificial intelligence in the histological assessment of pulmonary subsolid nodules
Introduction
Previously, it has been reported that a reduction of mortality with low-dose computed tomography (CT) in a number of lung cancer screening trials (1-3). Consequently, lung cancer screening is more and more being implemented in the past two decades. With the increased use of CT and pulmonary subsolid nodules (SSNs), SSNs are increasingly being detected. Imaging assessment of invasiveness of SSNs is essential in the clinical management of patients. However, the histological prediction of SSNs, which has been reported to have a 9% detection rate in screening trials, poses several challenges (4,5). The degree of invasiveness is used as the basis for clinical management decisions. Lung adenocarcinoma appearing as SSN can present with a variety of morphological and imaging features, which can be related to different degrees of invasiveness and prognosis. Reported evidence of high intra-observer and interobserver variability in the invasiveness classification of SSNs has highlighted concerns about undertreatment and overtreatment. Therefore, an accurate diagnostic system or assistant tool can have a beneficial clinical impact (6).
To overcome these diagnostic challenges, a number of solutions for malignancy evaluation have been previously proposed (7-11), including radiological density, morphological features, and clinical features. Risk-assessing tools based on clinical and radiological features have been used to determine cancer risk and standardize clinical management recommendations (8,12). Additionally, quantitative analyses have been carried out to evaluate malignancy depending on accurate delineation of nodule borders and feature engineering (13-16). However, the application of previous methods relies on subjective interpretation or manual segmentation, indicating the implementation of automated approaches remains unsolved.
Recently advanced AI models have demonstrated specialist-level classification performance in medical image diagnosis (17-24). AI models which automatically correspond representative features from medical image data to specific task, have recently been introduced as a novel technique (25,26). The development of an accurate AI system could reduce the inconsistency among doctors with different expertise and provide management decision support. There is limited research on developing AI algorithm classifying invasiveness of pulmonary nodules (7,27,28). Attempts at assessing SSN invasiveness have been limited to binary classification or simple comparison with doctors (27,29). Previous researches constructed algorithms based on 2D image rather than 3D volume, which has limited the performance of AI techniques. Rare evidence has been reported in external validation in developing invasiveness classification AI system. Nevertheless, the clinical utility of AI-assisted diagnostic models needs to be investigated (6).
In the present study, we aimed to elucidate the applicability and reliability of a 3D AI algorithm to assess the invasiveness of SSNs by comparing both against the diagnostic performance of chest radiologists and thoracic surgeons and our previously developed feature-based radiomic signature (10). We investigated the practicality of our proposed AI algorithm by evaluating the improvement of prediction performance when the proposed method served as a second opinion. To further investigate the clinical utility, the proposed AI system was validated in an external cohort with chest radiologists and thoracic surgeons. To our knowledge, this is the first investigation of how AI assists doctors in SSN malignancy evaluation. We present the following article in accordance with the STARD reporting checklist (available at https://dx.doi.org/10.21037/tlcr-21-971).
Methods
Patient selection and study materials
Consecutive patients who underwent pulmonary resection for lung adenocarcinoma between January 2013 and December 2015 in Shanghai Pulmonary Hospital were retrospectively collected. Using the descriptive events including “subsolid nodule”, “part-solid nodule”, “non-solid nodule”, “mixed nodule”, “ground-glass nodule” or “ground glass opacity”, we retrieved the preoperative CT examinations of patients and 4,679 scans were confirmed. The CT scans were reviewed and SSNs were included under the following criteria: (I) the maximum diameter of lesion ≤3 cm on thin-section CT images (<1.5 mm) within 2 weeks prior to the surgery; (II) pulmonary nodules were histopathologically confirmed as atypical adenomatous hyperplasia (AAH), adenocarcinoma in situ (AIS), minimally invasive adenocarcinoma (MIA), or invasive adenocarcinoma (IA) according to the lung tumor classification; (III) patients without a history of malignancy or surgery. For patients with multiple lesions, cases without corresponding confirmed pathological diagnosis were excluded. A total of 1,471 patients with 1,589 SSNs from Shanghai Pulmonary Hospital (Shanghai, China) were included in the present study. A total of 1,349 (85%) nodules from 1,262 patients comprised the development set, including a training subset (n=1,191, 75%) and an internal subset (n=158, 10%); 240 nodules (15%) from 209 patients comprised a hold-out test dataset, of which data were unseen during the training course. To independently test the diagnostic value of the proposed framework, an external test dataset of 100 patients with 102 SSNs was included according to the same criteria from Hwa Mei Hospital (Ningbo, China). The workflow of patient inclusion is shown in Figure S1. In the internal test dataset, 14 (5.8%) patients were diagnosed with AAH, 67 (27.9%) with AIS, 55 (22.9%) with MIA, and 104 (43.4%) of with IA. In the external test dataset, 5 (4.9%) patients were diagnosed with AAH, 25 (24.5%) with AIS, 24 (23.5%) with MIA, and 48 (47.1%) with IA. The study was conducted in accordance with the Declaration of Helsinki (as revised in 2013). This retrospective study was approved by the Shanghai Pulmonary Hospital Institutional Review Board (No. L20-344). The need for informed consent was waived.
Data extraction and annotations
Chest CT images were acquired on two different scanners: Somatom Definition AS+ (Siemens Medical Systems, Germany, n=1,263) and iCT256 (Philips Medical Systems, Netherlands, n=308). All image data were reconstructed with slice thickness of <1.5 mm (30) and matrix of 512 mm × 512 mm. All CT scans were download form our picture archiving and communications systems (PACS) as digital imaging and communications in medicine (DICOM) images. The personal information of patients in CT images including name, medical number and hospital name were eliminated and images were transformed into NIfTI (NII) format by using an in-house software. The lung CT NII format images were imported into 3D slicer (version 4.8.0, Brigham and Women’s Hospital) for labelling. The region of interest (ROI) of SSNs was annotated with a bounding box including the SSN by 2 junior thoracic surgery doctors (Y.S. and J.D. with 4 and 2 years of experience, respectively), then the consensus of ROI was obtained by discussion with an expert chest radiologist (J.S. with 28 years of experience). Bronchi and pulmonary vessels were excluded as far as possible from the ROI. Then the image data of ROI was extracted in the “rcsv” format for further analysis. Each segmented ROI was annotated by a specific histopathologic label according to the specific histologic subtype of AAH, AIS, MIA, and IA. The histologic slides and results were reviewed by two experienced pathologists (L.H. and C.W.) separately with hematoxylin and eosin slides of the study cases in the absence of any clinical or radiologic information. And these histologic labels were reported in accordance with the updated classification of lung cancer (31).
Study design
As illustrated in Figure 1, an AI algorithm for invasiveness assessment, SSNet, was constructed for the histological classification of lung adenocarcinoma appearing as pulmonary SSNs on CT scans (Details of algorithm development are shown in Appendix 1). SSNet was developed and tested by using a retrospective dataset with patients with available CT images and corresponding histological diagnoses (31). The diagnostic performance of SSNet was evaluated by comparing with those of our previously reported radiomic feature-based signature (10) (Appendix 1) and three chest radiologists and three thoracic surgeons with experience ranging from junior to senior (clinical experience of three to more than 20 years). The 6 doctors were asked to evaluate the SSNs again with the prediction of SSNet to investigate the clinical utility based on performance improvements. Both the diagnostic accuracy and clinical utility for SSNs were further examined in an external test cohort.
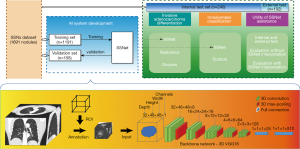
Clinical interpretation of CT images
All included cases were reviewed independently in a blinded fashion by six independent doctors from junior to senior degree in thoracic surgery and imaging (Y.S. and T.W. of junior degree with less than 5 years of experience, J.M. and J.S. of intermediate degree with 5–15 years of experience, D.X. and X.S. of senior degree with more than 25 years of experience in thoracic imaging, respectively). All readers subjectively were asked to provide categories of four histological subtypes, then the predicted labels were regrouped according to different tasks for evaluation. To evaluate the increments of diagnostic performance assisted by the SSNet, the six doctors were asked to re-evaluate the SSNs at least 4 weeks after the first evaluation with the predictions of SSNet as a second opinion. Inter-observer variability and diagnostic performance were compared against those from the first evaluation. Clinical interpretation was done without time constrain in RadiAnt Viewer (version 4.6.9, Medixant, https://www.radiantviewer.com). Raters were free to adjust the display window setting and use electronic caliper provided in the software.
Performance testing and statistical analysis
In the discrimination of IA from non-IA, including AAH, AIS, and MIA, the diagnostic performance of SSNet was compared to practicing doctors and radiomic signature using area under receiver-operating characteristic (ROC) curve (AUC) metric (Appendix 1). Comparisons of the diagnostic performance between SSNet and the practicing doctors were also done in 3- and 4-category classifications in a similar manner. Statistically significant differences in AUCs were assessed with Bonferroni-corrected confidence intervals (CIs; 1–0.05/n). Interobserver variability in participant level was evaluated by kappa concordance index. Performance metrics of sensitivity, specificity, accuracy, positive predictive value, and negative predictive value of each method were measured. Area under the precision-recall curve (AUPRC) and the F1 score were used to evaluate the multiple-category classification performance and reported as the macro average and micro average. Performance-evaluation metrics of practicing doctors were reported in group level and participant level, respectively. McNemar’s test was used to compare the statistical difference of sensitivity, specificity, and accuracy between the performance of SSNet and that of practicing doctors, as well as between the performance of practicing doctors with and without the interpretation assistance of SSNet in the binary classification task. Statistical analyses were performed in MedCalc (version 15.2.0; Mariakerke, Belgium), SPSS (version 23.0; IBM, Armonk, NY, USA), and R software (version 3.6.2; https://www.r-project.org/). P<0.05 was considered statistically significant.
Results
Baseline information
The internal dataset consisted of 1,589 SSNs from 471 patients (median age: 57 years, range, 23–82 years) and the external test dataset cohort included 102 SSNs from 100 patients (median age: 56 years, range, 28–75 years). The distribution of histological subtypes was similar between the 2 test datasets. There was no significant difference in age and sex of the 2 cohorts (Table S1).
Diagnostic performance in invasive classification
In the differentiation of IA from minimally invasive/pre-invasive lesions, the ROC curves for the 3 approaches are illustrated in Figure 2A, and comparisons of AUCs are reported in Table 1. The SSNet algorithm (AUC: 0.914, 95% CI: 0.813–0.987) performed as well as practicing doctors (AUC: 0.900, 95% CI: 0.867–0.922). The radiomic signature was inferior to the practicing doctors, with an AUC of 0.845 (95% CI: 0.806–0.883) and a statistically significant difference.
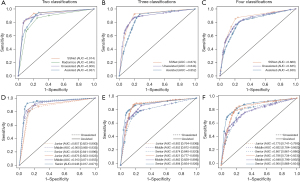
Table 1
Tasks | AUC | 95% CI | Difference (Bonferroni corrected CI) | Advantage |
---|---|---|---|---|
Internal test | ||||
Two classifications | ||||
SSNet | 0.914 | 0.813–0.987 | – | – |
Human (unassisted) | 0.900 | 0.867–0.922 | –0.014 (–0.090 to 0.060)* | No difference |
Human (assisted) | 0.937 | 0.911–0.970 | 0.037 (–0.078 to –0.014)† | Human (assisted) |
Radiomics | 0.845 | 0.806–0.883 | 0.067 (–0.034 to 0.145)* | No difference |
0.071 (0.032–0.110)‡ | Human (unassisted) | |||
Three classifications | ||||
SSNet | 0.874 | 0.832–0.909 | – | – |
Human (unassisted) | 0.844 | 0.816–0.864 | –0.030 (0.000–0.087)* | SSNet |
Human (assisted) | 0.852 | 0.825–0.882 | 0.008 (-0.015-0.042)† | No difference |
Four classifications | ||||
SSNet | 0.869 | 0.824–0.892 | – | – |
Human (unassisted) | 0.835 | 0.817–0.862 | –0.034 (0.012–0.098)* | SSNet |
Human (assisted) | 0.836 | 0.811–0.862 | 0.001 (–0.030 to 0.036)† | Human (assisted) |
External test | ||||
Two classifications | ||||
SSNet | 0.949 | 0.884–1.000 | – | |
Human (unassisted) | 0.883 | 0.826–0.939 | –0.066 (0.037–0.212)* | SSNet |
Human (assisted) | 0.908 | 0.847–0.982 | 0.025 (–0.092 to 0.029)† | Human (assisted) |
*, AUC difference was calculated as the AUC of the algorithm minus the AUC of the doctors (unassisted) or the AUC of radiomics. †, AUC difference was calculated as the AUC of the doctors (assisted) minus the AUC of the doctors (unassisted). ‡, AUC difference was calculated as the AUC of the doctors (unassisted) minus the AUC of the radiomics. To account for multiple hypothesis testing, the Bonferroni corrected CI (1−0.05/n, 97.5% for 2 classifications; 98.3% for 3 classifications; 98.8% for 4 classifications) around the difference was computed. AUC, area under the receiver-operating characteristic curve; CI, confidence interval.
In the differentiation of pre-invasive, minimally invasive, and invasive lesions, the ROC curves for SSNet and practicing doctors are illustrated in Figure 2B, and comparisons of AUCs are reported in Table 1. The SSNet algorithm (AUC: 0.874, 95% CI: 0.832–0.909) performed better than that of practicing doctors (AUC: 0.844, 95% CI: 0.816–0.864).
In the differentiation of all 4 histological subtypes of lung adenocarcinoma, the ROC curves for SSNet and practicing doctors are illustrated in Figure 2C, and comparisons of AUCs are reported in Table 1. The SSNet algorithm (AUC: 0.869, 95% CI: 0.824–0.892) performed better than practicing doctors (AUC: 0.835, 95% CI: 0.817–0.862).
Performance of SSNet in assisting readers
In the performance test using the SSNet algorithm, AUCs of clinicians were 0.937, 0.852, and 0.836 for differentiating IA from non-IA, MIA, AIS, and for differentiating IA from AAH and MIA, AIS, and AAH, respectively (Figure 2D-2F; Table 1). Compared with the diagnostic performance of subjective evaluation only, increments of AUCs were 0.037, 0.008, and 0.001, respectively, and those in multiple-category classification were statistically significant (Table 1). Specifically, in the differentiation of IA, the sensitivity of 1 of the junior doctors improved from 0.750 to 0.885 (P=0.004), and the specificity of 1 of the senior doctors increased from 0.897 to 0.949 (P=0.039). In the multiple-category classification, improvements in multiple statistics were observed in 3 practicing doctors (Tables S2,S3). Improvements were seen in the evaluation metrics in all classes for 3-category classification and were more often in classes of lower-grade invasiveness in the 4-category classification. Kappa statistics improved from 0.480 to 0.496 for 4-category classification but decreased from 0.601 to 0.596 for 3 classifications, respectively.
Performance evaluation in details
In discriminating invasive lesions, performance-evaluating results, including sensitivity, specificity, and accuracy were shown in Figure 3A and Table 2. In terms of the approach level, SSNet achieved a sensitivity of 0.933, which was higher than the micro-average sensitivity of practicing doctors (0.846), and a radiomic signature of 0.885. SSNet accuracy was 0.921, which was higher than the micro-average accuracy of practicing doctors (0.919) and that of the radiomic signature (0.866). For the 3-category classification, SSNet had better performance for most of the evaluation metrics when compared with practicing doctors as a group and individually (Figure 3B-3D; Tables S2,S4). For the 4-category classification, SSNet demonstrated better performance for all evaluation metrics when compared with group or individual performance of practicing doctors (Figure 3E-3H; Tables S3,S4). SSNet maintained better performance in terms of micro-average AUPRC than junior doctors and 1 of the mid-career doctors. A mid-career and a senior doctor achieved a higher micro-average AUPRC than SSNet.
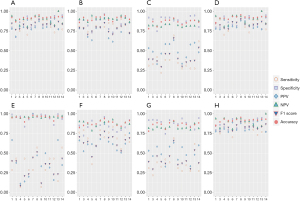
Table 2
Performance metrics | SSNet | Radiomics | Unassisted | Assisted | |||||||||||||||||
---|---|---|---|---|---|---|---|---|---|---|---|---|---|---|---|---|---|---|---|---|---|
Junior | Middle | Senior | Micro average | Junior | Middle | Senior | Micro average | ||||||||||||||
1 | 2 | 1 | 2 | 1 | 2 | 1 | 2 | 1 | 2 | 1 | 2 | ||||||||||
Retrospective | |||||||||||||||||||||
Sensitivity | 0.933 | 0.885 | 0.750 | 0.875 | 0.702 | 0.894 | 0.933 | 0.923 | 0.846 | 0.885 | 0.885 | 0.798 | 0.731 | 1.000 | 0.769 | 0.845 | |||||
McNemar’s test | <0.001* | 0.146* | <0.001* | 0.424* | 1.000* | 1.000* | 0.004† | 1.000† | 0.052† | 0.001† | 0.016† | 0.002† | |||||||||
Specificity | 0.794 | 0.673 | 0.860 | 0.831 | 0.934 | 0.794 | 0.816 | 0.897 | 0.855 | 0.816 | 0.831 | 0.912 | 0.941 | 0.772 | 0.949 | 0.870 | |||||
McNemar’s test | 0.049* | 0.302* | <0.001* | 1.000* | 0.250* | 0.003* | 0.146† | 1.000† | 0.508† | <0.001† | 0.180† | 0.039† | |||||||||
Accuracy | 0.921 | 0.866 | 0.897 | 0.919 | 0.909 | 0.912 | 0.929 | 0.952 | 0.919 | 0.916 | 0.921 | 0.926 | 0.919 | 0.931 | 0.931 | 0.880 | |||||
McNemar’s test | <0.001* | 0.054 | <0.001* | 0.596* | 0.250* | 0.012* | 0.001† | 1.000† | 0.031† | <0.001† | 0.007† | <0.001† | |||||||||
Kappa‡ | 0.718 | 0.701 | |||||||||||||||||||
Prospective | |||||||||||||||||||||
Sensitivity | 0.958 | 0.708 | 0.854 | 0.625 | 0.875 | 0.958 | 0.896 | 0.819 | 0.0.875 | 0.813 | 0.729 | 0.667 | 1.000 | 0.729 | 0.802 | ||||||
McNemar’s test | <0.001* | 0.063* | <0.001* | 0.289* | 1.000* | 0.375* | 0.039† | 0.688† | 0.227† | 0.006† | 0.500† | 0.039† | |||||||||
Specificity | 0.796 | 0.815 | 0.852 | 0.907 | 0.759 | 0.815 | 0.833 | 0.830 | 0.759 | 0.778 | 0.870 | 0.944 | 0.778 | 0.944 | 0.846 | ||||||
McNemar’s test | 1.000* | 0.453* | 0.031* | 0.727* | 1.000* | 0.688* | 0.453† | 0.289† | 0.727† | 0.006† | 0.727† | 0.031† | |||||||||
Accuracy | 0.932 | 0.867 | 0.921 | 0.873 | 0.897 | 0.938 | 0.926 | 0.904 | 0.897 | 0.885 | 0.891 | 0.897 | 0.938 | 0.915 | 0.904 | ||||||
McNemar’s test | 0.004* | 0.039* | 0.001* | 0.804* | 1.000 * | 0.227* | 0.019† | 0.791† | 0.167† | <0.001† | 0.344† | 0.001† | |||||||||
Kappa‡ | 0.632 | 0.649 |
1, 2 represents doctors 1 and 2. *, McNemar’s test P value for comparison of evaluation metrics between SSNet and practicing doctors alone; †, McNemar’s test P-value for comparison of evaluation metrics between practicing doctors with and without the assistance of SSNet; ‡, Kappa value was calculated as Fleiss’ kappa for the 6 readers.
External test for diagnostic performance
The primary outcome was evaluated for 102 SSNs. SSNet demonstrated excellent diagnostic performance, with an AUC of 0.949 (95% CI: 0.884–1.000), and was better than that of the practicing doctors (AUC: 0.883, 95% CI: 0.826–0.982) (Table 1; Figure 4). The sensitivity and accuracy of the differentiation for IA by SSNet was 0.958 and 0.932, respectively, which was significantly higher than the micro-average sensitivity (0.819) and accuracy (0.904) of practicing doctors as a group, respectively. In the evaluation of clinical utility, the AUC of practicing doctors was significantly improved from 0.883 to 0.908 with the assistance of SSNet (Table 1; Figure 4). Sensitivity and specificity micro averages of practicing doctors also improved. For the participant level of performance improvement, the accuracy of a junior doctor significantly improved from 0.867 to 0.897 (Table 2). The kappa statistic also improved from 0.632 to 0.649.
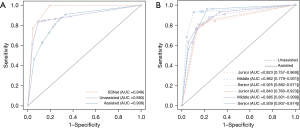
Discussion
Clinical management for SSNs between invasive and pre-invasive lesions is different. Therefore, the use of a risk-prediction tool that distinguishes invasive lesions from pre-invasive ones is significant. In the present study, we demonstrated that a simple 3D AI diagnostic tool, SSNet, based on CT images enabled the differentiation of IA from pre-invasive/minimally invasive lesions and histological subtype classification in lesions that appeared as SSNs (<3 cm) on chest CT. Performance was equal between SSNet, radiomic signature and doctors in binary discrimination on an internal test but better for SSNet than doctors on external test in the classification of more than two categories. In addition, the use of SSNet enhanced doctors’ SSN interpretations.
Evaluation by radiomic signature, which was previously developed for discriminating IAs, did not reach its optimal performance in our internal test. A possible reason for performance discrepancy would be the spectrum effect (25,32). The population used for the construction of a radiomic signature had a higher proportion of invasive lesions (>50%), while the rate of IA was relatively low in the present study (<50%) (10). A similar performance drop was also seen in another validation experiment using a similar population (33). Previous models developed to differentiate invasive lesions by CT features had an AUC of 0.64–0.91 (7,10,34-38). However, these models have not been validated in an external cohort. The performance of the SSN evaluation model based on predefined features was limited by the subjectivity and proficiency in CT interpretation, whereas the AI-based evaluation model is able to learn representative features from raw medical image without specifying radiological features. Recently, Wang et al. proposed an AI system using a 3D convolutional neural network for differentiating pre-invasive lesions from IA appearing as SSNs no larger than 3 cm (29). In their study, the proposed architecture with an AUC of 0.892 outperformed the performances of 4 radiologists, who yielded an AUC between 0.805 and 0.867. However, the model was not designed for further discrimination of specific lung adenocarcinoma histological subtypes and the model was not fully investigated or externally validated for its clinical utility. In our study, the 3D SSNet utilized the volumetric data of thin-section CT from 1,471 patients and the proposed AI system achieved a competitive AUC of 0.914 in terms of differentiating IA from pre-invasive and minimally invasive lesions compared with doctors. In addition, the external diagnostic evaluation found that SSNet outperformed practicing doctors in IA discrimination.
Concerns remain regarding the actual help of an AI-based evaluation system in clinical practice. To date, limited studies have investigated the benefits of an AI system in assessing invasiveness (7,20,39,40). In the present study, we investigated the improvement with the assistance of AI interpretation as a reference. Based on our results, the performance of practicing doctors improved with the assistance of SSNet in invasive discrimination and multiple-category invasiveness assessments. Although the AUPRC, a metric evaluating a classifier’s performance in imbalanced data, of SSNet was lower than some of the practicing doctors in terms of multiple-category classification, the accuracy of doctors improved with SSNet. These findings support the role of the AI system as a second viewer that would increase the AUPRC in diagnosing cases that doctors might misinterpret or miss.
In the present study, SSNet achieved better performance than practicing doctors in identifying lesions with lower grade of invasiveness, which was shown in the 3- and 4-category classifications. The F1 scores for AAH class and MIA class were relatively low due to the number of samples, which could limit the performance of the AI system. It is recommended that patients with AAH are routinely followed up or undergo resection after comprehensive evaluation. MIA is defined as small nodules with ≤5 mm predominantly lepidic invasion, Lim et al. inferred that invasion ≤5 mm might not greatly contribute in the emergence of increased attenuation on CT scan (41). Therefore, MIA appearing as SSNs can easily be misclassified as AIS or IA by doctors and the AI system (Figure S2).
In the present study, there was no incorrect prediction of SSNet as AAH being misclassified as IA or IA as AAH (Figure S2). SSNet achieved a higher AUPRC in differentiating histological subtypes than junior doctor in multiple-category classification. Although the AUPRC of SSNet was not higher than those of intermediate and senior doctors, the reduced time in interpretation of SSNs and the improved diagnostic performance would streamline the workflow and reduce subjectivity bias. Additionally, several underestimated predictions can be corrected by the AI system, as radiological features indicating malignancy could be absent or not fully identified (Figure S2). This was also proved by the highest sensitivity by SSNet and the confusion matrices. The SSN evaluation process is simple. Only a cuboid box that fully embraces SSN at its mass center is required for the invasiveness evaluation. Therefore, the incorporation of SSNet into the workflow would improve efficiency and accuracy in identifying SSNs and the workload of radiologists.
The present study has several limitations. First, data of this study only reflects this particular study population and cannot extrapolated to a screening setting. Secondly, though the proposed AI algorithm showed discriminative ability in classifying invasiveness of SSNs, the relevant image features that dominate model for decision-making were not reflected due to the black box nature of deep learning algorithm. Further explainable deep learning modes with good performance are necessary to improve the transparency and re reliability for humans. Additionally, the generalizability of our proposed method should be confirmed with more external and prospective validation. In the present study, the test dataset was split prior to model development and treated as a hold-out dataset to evaluate diagnostic performance. An external test cohort was used for validation. It should be acknowledged that although geographically external validation as applied, the SSN interpretation was done in a non-clinical environment and would not change clinical decisions that happened to the included patients. There is a potential decision threshold shift that could bias the actual clinical utility of the AI diagnostic tool. Therefore, further prospective studies for clinical decision making with long-time follow-up are needed to warrant the clinical utility of this diagnostic AI system and the value of improving patient outcome for such an AI diagnostic system designed for clinical management decision support. Multi-institutional randomized controlled trials are critical to test the benefit of incorporating AI into workflow.
In conclusion, the proposed SSNet is helpful in evaluating SSNs and can be used to assess invasiveness. The implementation of SSNet in practice has the potential to improve patient care workflow and optimize clinical decision support. The safety and feasibility of AI-assisted tools in supporting clinical decisions for SSNs are warranted in long-term and multi-institutional trials.
Acknowledgments
The authors appreciate the academic support from the AME Thoracic Surgery Collaborative Group. The authors would like to thank biostatistician, Professor Aihong Zhang (Department of Medical Statistics, Tongji University School of Medicine, Shanghai, China), for guidance on the statistical analysis in this research.
Funding: This study was supported by National Natural Science Foundation of China (No. 8210071009); Shanghai Science and Technology Commission (No. 21YF1438200); Shanghai Municipal Health Commission (No. 2019SY072); the Science-Technology Foundation for Young Scientists of Gansu Province (No. 18JR3RA305, 21JR1RA107); and the Natural Science Foundation of Gansu Province (No. 21JR1RA118, 21JR1RA092).
Footnote
Reporting Checklist: The authors have completed the STARD reporting checklist. Available at https://dx.doi.org/10.21037/tlcr-21-971
Data Sharing Statement: Available at https://dx.doi.org/10.21037/tlcr-21-971
Conflicts of Interest: All authors have completed the ICMJE uniform disclosure form (available at https://dx.doi.org/10.21037/tlcr-21-971). SZ reports employment at Tailai Biosciences Inc., Shenzhen, Chinia. SJ reports employment at Dianei Technology, Shanghai, China. The other authors have no conflicts of interest to declare.
Ethical Statement: The authors are accountable for all aspects of the work in ensuring that questions related to the accuracy or integrity of any part of the work are appropriately investigated and resolved. The study was conducted in accordance with the Declaration of Helsinki (as revised in 2013). This retrospective study was approved by the Shanghai Pulmonary Hospital Institutional Review Board (No. L20-344). The need for informed consent was waived.
Open Access Statement: This is an Open Access article distributed in accordance with the Creative Commons Attribution-NonCommercial-NoDerivs 4.0 International License (CC BY-NC-ND 4.0), which permits the non-commercial replication and distribution of the article with the strict proviso that no changes or edits are made and the original work is properly cited (including links to both the formal publication through the relevant DOI and the license). See: https://creativecommons.org/licenses/by-nc-nd/4.0/.
References
- Field JK, Duffy SW, Baldwin DR, et al. UK Lung Cancer RCT Pilot Screening Trial: baseline findings from the screening arm provide evidence for the potential implementation of lung cancer screening. Thorax 2016;71:161-70. [Crossref] [PubMed]
- de Koning HJ, van der Aalst CM, de Jong PA, et al. Reduced Lung-Cancer Mortality with Volume CT Screening in a Randomized Trial. N Engl J Med 2020;382:503-13. [Crossref] [PubMed]
- Rzyman W, Szurowska E, Adamek M. Implementation of lung cancer screening at the national level: Polish example. Transl Lung Cancer Res 2019;8:S95-105. [Crossref] [PubMed]
- Yankelevitz DF, Yip R, Smith JP, et al. CT Screening for Lung Cancer: Nonsolid Nodules in Baseline and Annual Repeat Rounds. Radiology 2015;277:555-64. [Crossref] [PubMed]
- Henschke CI, Yip R, Smith JP, et al. CT Screening for Lung Cancer: Part-Solid Nodules in Baseline and Annual Repeat Rounds. AJR Am J Roentgenol 2016;207:1176-84. [Crossref] [PubMed]
- Kauczor HU, von Stackelberg O. Subsolid Lung Nodules: Potential for Overdiagnosis. Radiology 2019;293:449-50. [Crossref] [PubMed]
- Varghese C, Rajagopalan S, Karwoski RA, et al. Computed Tomography-Based Score Indicative of Lung Cancer Aggression (SILA) Predicts the Degree of Histologic Tissue Invasion and Patient Survival in Lung Adenocarcinoma Spectrum. J Thorac Oncol 2019;14:1419-29. [Crossref] [PubMed]
- McWilliams A, Tammemagi MC, Mayo JR, et al. Probability of cancer in pulmonary nodules detected on first screening CT. N Engl J Med 2013;369:910-9. [Crossref] [PubMed]
- Li Y, Chen KZ, Wang J. Development and validation of a clinical prediction model to estimate the probability of malignancy in solitary pulmonary nodules in Chinese people. Clin Lung Cancer 2011;12:313-9. [Crossref] [PubMed]
- She Y, Zhang L, Zhu H, et al. The predictive value of CT-based radiomics in differentiating indolent from invasive lung adenocarcinoma in patients with pulmonary nodules. Eur Radiol 2018;28:5121-8. [Crossref] [PubMed]
- Maldonado F, Boland JM, Raghunath S, et al. Noninvasive characterization of the histopathologic features of pulmonary nodules of the lung adenocarcinoma spectrum using computer-aided nodule assessment and risk yield (CANARY)--a pilot study. J Thorac Oncol 2013;8:452-60. [Crossref] [PubMed]
- American College of Radiology. Lung-RADS Version 1.1. 2019. Accessed 13 Jan 2019. Available online: https://www.acr.org/-/media/ACR/Files/RADS/Lung-RADS/LungRADSAssessmentCategoriesv1-1.pdf?la=en
- Lee SM, Park CM, Goo JM, et al. Invasive pulmonary adenocarcinomas versus preinvasive lesions appearing as ground-glass nodules: differentiation by using CT features. Radiology 2013;268:265-73. [Crossref] [PubMed]
- Heidinger BH, Anderson KR, Nemec U, et al. Lung Adenocarcinoma Manifesting as Pure Ground-Glass Nodules: Correlating CT Size, Volume, Density, and Roundness with Histopathologic Invasion and Size. J Thorac Oncol 2017;12:1288-98. [Crossref] [PubMed]
- Qi L, Xue K, Li C, et al. Analysis of CT morphologic features and attenuation for differentiating among transient lesions, atypical adenomatous hyperplasia, adenocarcinoma in situ, minimally invasive and invasive adenocarcinoma presenting as pure ground-glass nodules. Sci Rep 2019;9:14586. [Crossref] [PubMed]
- Kim H, Goo JM, Kim YT, et al. Consolidation-to-tumor ratio and tumor disappearance ratio are not independent prognostic factors for the patients with resected lung adenocarcinomas. Lung Cancer 2019;137:123-8. [Crossref] [PubMed]
- Esteva A, Kuprel B, Novoa RA, et al. Corrigendum: Dermatologist-level classification of skin cancer with deep neural networks. Nature 2017;546:686. [Crossref] [PubMed]
- Rajpurkar P, Irvin J, Ball RL, et al. Deep learning for chest radiograph diagnosis: A retrospective comparison of the CheXNeXt algorithm to practicing radiologists. PLoS Med 2018;15:e1002686. [Crossref] [PubMed]
- Nam JG, Park S, Hwang EJ, et al. Development and Validation of Deep Learning-based Automatic Detection Algorithm for Malignant Pulmonary Nodules on Chest Radiographs. Radiology 2019;290:218-28. [Crossref] [PubMed]
- Zhang Y, Wu X, He L, et al. Applications of hyperspectral imaging in the detection and diagnosis of solid tumors. Transl Cancer Res 2020;9:1265-77. [Crossref]
- Tschandl P, Codella N, Akay BN, et al. Comparison of the accuracy of human readers versus machine-learning algorithms for pigmented skin lesion classification: an open, web-based, international, diagnostic study. Lancet Oncol 2019;20:938-47. [Crossref] [PubMed]
- Gulshan V, Peng L, Coram M, et al. Development and Validation of a Deep Learning Algorithm for Detection of Diabetic Retinopathy in Retinal Fundus Photographs. JAMA 2016;316:2402-10. [Crossref] [PubMed]
- Ehteshami Bejnordi B, Veta M, Johannes van Diest P, et al. Diagnostic Assessment of Deep Learning Algorithms for Detection of Lymph Node Metastases in Women With Breast Cancer. JAMA 2017;318:2199-210. [Crossref] [PubMed]
- Li X, Zhang S, Zhang Q, et al. Diagnosis of thyroid cancer using deep convolutional neural network models applied to sonographic images: a retrospective, multicohort, diagnostic study. Lancet Oncol 2019;20:193-201. [Crossref] [PubMed]
- Faes L, Wagner SK, Fu DJ, et al. Automated deep learning design for medical image classification by health-care professionals with no coding experience: a feasibility study. Lancet Digit Health 2019;1:e232-42. [Crossref] [PubMed]
- Castiglioni I, Rundo L, Codari M, et al. AI applications to medical images: From machine learning to deep learning. Phys Med 2021;83:9-24. [Crossref] [PubMed]
- Zhao W, Yang J, Sun Y, et al. 3D Deep Learning from CT Scans Predicts Tumor Invasiveness of Subcentimeter Pulmonary Adenocarcinomas. Cancer Res 2018;78:6881-9. [Crossref] [PubMed]
- Kim H, Lee D, Cho WS, et al. CT-based deep learning model to differentiate invasive pulmonary adenocarcinomas appearing as subsolid nodules among surgical candidates: comparison of the diagnostic performance with a size-based logistic model and radiologists. Eur Radiol 2020;30:3295-305. [Crossref] [PubMed]
- Wang S, Wang R, Zhang S, et al. 3D convolutional neural network for differentiating pre-invasive lesions from invasive adenocarcinomas appearing as ground-glass nodules with diameters ≤3 cm using HRCT. Quant Imaging Med Surg 2018;8:491-9. [Crossref] [PubMed]
- Guchlerner L, Wichmann JL, Tischendorf P, et al. Comparison of thick- and thin-slice images in thoracoabdominal trauma CT: a retrospective analysis. Eur J Trauma Emerg Surg 2020;46:187-95. [Crossref] [PubMed]
- Travis WD, Brambilla E, Nicholson AG, et al. The 2015 World Health Organization Classification of Lung Tumors: Impact of Genetic, Clinical and Radiologic Advances Since the 2004 Classification. J Thorac Oncol 2015;10:1243-60. [Crossref] [PubMed]
- Riley RD, Ensor J, Snell KI, et al. External validation of clinical prediction models using big datasets from e-health records or IPD meta-analysis: opportunities and challenges. BMJ 2016;353:i3140. [Crossref] [PubMed]
- Ransohoff DF, Feinstein AR. Problems of spectrum and bias in evaluating the efficacy of diagnostic tests. N Engl J Med 1978;299:926-30. [Crossref] [PubMed]
- Liang J, Xu XQ, Xu H, et al. Using the CT features to differentiate invasive pulmonary adenocarcinoma from pre-invasive lesion appearing as pure or mixed ground-glass nodules. Br J Radiol 2015;88:20140811. [Crossref] [PubMed]
- Son JY, Lee HY, Kim JH, et al. Quantitative CT analysis of pulmonary ground-glass opacity nodules for distinguishing invasive adenocarcinoma from non-invasive or minimally invasive adenocarcinoma: the added value of using iodine mapping. Eur Radiol 2016;26:43-54. [Crossref] [PubMed]
- Zhang Y, Shen Y, Qiang JW, et al. HRCT features distinguishing pre-invasive from invasive pulmonary adenocarcinomas appearing as ground-glass nodules. Eur Radiol 2016;26:2921-8. [Crossref] [PubMed]
- Jin C, Cao J, Cai Y, et al. A nomogram for predicting the risk of invasive pulmonary adenocarcinoma for patients with solitary peripheral subsolid nodules. J Thorac Cardiovasc Surg 2017;153:462-469.e1. [Crossref] [PubMed]
- Baldwin DR, Gustafson J, Pickup L, et al. External validation of a convolutional neural network artificial intelligence tool to predict malignancy in pulmonary nodules. Thorax 2020;75:306-12. [Crossref] [PubMed]
- Hwang EJ, Nam JG, Lim WH, et al. Deep Learning for Chest Radiograph Diagnosis in the Emergency Department. Radiology 2019;293:573-80. [Crossref] [PubMed]
- Hwang EJ, Park S, Jin KN, et al. Development and Validation of a Deep Learning-based Automatic Detection Algorithm for Active Pulmonary Tuberculosis on Chest Radiographs. Clin Infect Dis 2019;69:739-47. [Crossref] [PubMed]
- Lim HJ, Ahn S, Lee KS, et al. Persistent pure ground-glass opacity lung nodules ≥ 10 mm in diameter at CT scan: histopathologic comparisons and prognostic implications. Chest 2013;144:1291-9. [Crossref] [PubMed]
(English Language Editor: R. Scott)