A prediction model integrated genomic alterations and immune signatures of tumor immune microenvironment for early recurrence of stage I NSCLC after curative resection
Introduction
Lung cancer has the second-highest morbidity and the highest mortality of all malignant tumors worldwide. Non-small cell lung cancer (NSCLC) comprises 80–85% of lung cancer (1). The prevalence of stage I NSCLC has increased alongside the increased implication of low-dose computed tomographic screening (2). Surgical resection remains the standard treatment for these patients. However, the prognosis remains unsatisfactory because of local or distant recurrence. Almost one-third of patients relapse after surgery, and half of them succumb to the disease in the first year (3). Although some stage IB patients with high-risk factors received adjuvant chemotherapy, few benefited from the treatment (4). Perioperative targeted therapy and immunotherapy have made breakthroughs in recent years for NSCLC, while few studies enrolled stage I patients. Several phase III clinical trials, including ADAURA, IMpower-010, and Checkmate-816, demonstrated that perioperative targeted therapy and immunotherapy might improve the prognosis for stage IB NSCLC patients. However, it should be noted that the benefits for stage IB patients were not as good as stage II/IIIA (5-7). A better understanding of the role of genomic alterations and immune microenvironment may highlight potential novel avenues to develop perioperative a treatment for stage I NSCLC.
Genomic alterations, such as somatic mutations and copy number variations, are important factors that may underlie recurrence in early-stage NSCLC. “Driver mutations” confer a selective growth advantage to the tumor cells (8). It has been reported that driver gene mutations were associated with a higher risk of recurrence following surgery in stage I NSCLC (9). In contrast, other studies have reached the opposite conclusion (10,11). Similarly, studies have also examined the association between tumor mutation burden (TMB) and recurrence in early-stage lung cancers, yet the results have been controversial (12,13). Thus, the relationship between genomic alteration and recurrence for patients with stage I NSCLC require further study.
Programmed cell death-ligand 1 (PD-L1) is a common biomarker for the efficacy of immunotherapy, while its prognostic value for stage I NSCLC remains unclear. A few studies have reported that PD-L1 expression correlated with recurrence in stage I NSCLC, while the results were highly variable across different studies (14,15). Tumor-infiltrating immune cells, such as CD3+ and CD8+ tumor-infiltrating lymphocytes (TILs), play important roles in exerting anti-tumor immunity. According to the density and distribution of CD3+ and CD8+ TILs in tumor immune microenvironment (TIME), studies have proposed a classification system called “Immunoscore (IS)” in colon cancer, which significantly correlated with disease recurrence and even displayed a higher prognostic value than the TNM-classification system (16). However, it is unclear whether the IS could predict tumor recurrence in NSCLC.
Previous studies have built prognostic models based on clinical-pathological factors or radiomics features for stage I NSCLC (17-21). Recently, models based on tumor mutation or immune features also showed great prognostic value for predicting tumor recurrence in early-stage NSCLC (9,22-24). However, few studies integrated gene mutation and immune signatures to construct a model. In the present study, We developed and validated a model based on recurrence-associated genomic alterations and immune signatures in 130 stage I NSCLC to predict postoperative early recurrence. Our model showed excellent prognostic value and might provide evidence for the future study of perioperative treatment.
We present the following article in accordance with the TRIPOD reporting checklist (available at https://tlcr.amegroups.com/article/view/10.21037/tlcr-21-751/rc).
Methods
Study design and patients cohorts
We retrospectively analyzed data from the Second Xiangya Hospital of Central South University. From January 2011 to May 2020, a total of 130 resected stage I NSCLC patients, including 69 patients in early recurrence group (with recurrence within three years) and 61 patients in non-recurrence group (without recurrence more than five years). We reviewed the imaging system and surgical reports for all patients and restaged them according to the 8th edition of the American Joint Committee on Cancer (AJCC) TNM staging system. Tumor recurrence was defined as local relapse or distant metastasis in our study. All available samples were used to maximize the power and generalisability of the results. None of the patients received neoadjuvant therapy. Patients that met the following criteria were included in this study: (I) tumor recurrence within three years or without recurrence more than five years; (II) pathological diagnosis of stage I NSCLC; (III) surgical margins were negative; (IV) pathological tissue specimen were available. Patients with the following conditions were excluded from the study: (I) had any other malignancies; (II) received neoadjuvant therapy; (III) could not obtain qualified tumor tissue; (IV) refused to participate in this study. The outcome of interest was tumor early recurrence after surgery. Participants were followed by a varies methods, including telephone interviews, surveillance at our hospitals, and medical reports in our inpatient department after recurrence. Follow-up examination ways included radiological imaging with computed tomography (CT), magnetic resonance imaging (MRI), or positron emission tomography (PET-CT). The primary outcome was ascertained by two investigators blinded to the predictor variables. Disagreements were resolved by consensus. The following data were extracted for each patient: age, gender, histologic type, histologic grade, smoking history, tumor size, tumor site, the status of pleural invasion, the status of bronchus invasion, TNM stage. All these data were collected at the time of surgery. Besides, whole-exome sequencing data, CD3+, CD8+ TIL, and PD-L1 expression data were obtained from surgical specimens embedded in paraffin. The study was approved by the institutional review board of Second Xiangya Hospital of Central South University (No. 2020010), and all patients signed informed consent. All procedures performed in this study involving human participants conformed to the Declaration of Helsinki (revised in 2013).
Detection and analysis of genomic alterations
Each specimen was assessed by two lung cancer pathologists to confirm the histologic subtype and estimate tumor content (a minimum of 20% viable tumor cells required) before DNA extraction. Paired tumor and normal tissues were obtained. Whole exome sequencing (WES) was performed using the Illumina HiSeq4000 in the Clinical Laboratory Improvement Amendments (CLIA) lab. Qualified WES data were obtained in 74 patients. For those who were not qualified for WES (n=14), targeted gene panel sequencing consisting of 425 genes was performed to detect the somatic mutation. For WES samples, exonic regions in tumor and standard samples were read by an average of 150× and 60×, respectively. For targeted gene panel sequencing, regions in tumor and standard samples were read by an average of 1,000× and 200×. Details of DNA extraction and data processing for WES or targeted gene panel sequencing were described in supplementary methods (Appendix 1).
Using least absolute shrinkage and selection (Lasso), we selected recurrence-related mutations and built tumor mutation score (TMS) incorporating gene mutations. TMB was defined as the number of missense mutations per megabase of genome coding regions sequenced in WES. Genomic Identification of Significant Targets in Cancer (GISTIC) algorithm was applied to identify chromosomal gains and losses across samples, which was defined as chromosomal segments with log2 ratio of ≥0.25 (gain) and <−0.25 (loss), respectively. We separately uploaded all mutant genes to the DAVID database (https://david.ncifcrf.gov/) for early recurrence and non-recurrence groups. Gene ontology (GO) enrichment and Kyoto Encyclopedia of Genes and Genomes (KEGG) pathway analysis were performed using DAVID online for biological annotation.
Evaluation of immunohistochemical staining
Immunohistochemistry was performed to detect the expression of CD3 (Kit-0003, Fuzhou Maixin Biotech Co., Ltd.), CD8 (RMA-0514, Fuzhou Maixin Biotech Co., Ltd.), and PD-L1 (clone 22C3; DAKO) in whole section slides. The density of CD3+ and CD8+ TILs in the tumor center (CT) and invasive margin (IM) were semi-quantified by digital pathology (Image-Pro Plus software, version 6.0). For each region, one representative area presenting the highest infiltration and one presenting the lowest infiltration of cells were extracted under a 200× microscope. Cell density was calculated as the number of positive cells per square millimeter. The mean density of two areas was used as the final density of this region. A receiver operating characteristic curve (ROC) analysis was performed to evaluate the prognostic value of CD3+ and CD8+ TILs. The best cut-off was calculated according to the ROC curve. Patients were designated as high and low infiltration groups for CD3-CT+, CD3-IM+, CD8-CT+, and CD8-IM+ TILs. Immunoscore (IS) was built based on the density and location in the tumor microenvironment of CD3+ and CD8+ TILs. IS provides a score ranging from IS0 when low densities of both cell types are found in both regions to IS4 when high densities are found in both regions. All PD‐L1-stained slides were assessed with a tumor proportion score (TPS) and a combined proportion score (CPS). The TPS was defined as the number of positive tumor cells divided by the number of viable tumor cells multiplied by 100%. The CPS was defined as the total number of positive tumor cells and immune cells (including lymphocytes and macrophages) divided by the number of viable tumor cells, then multiplied by 100. Two experienced pathologists (SQF and QCL) evaluated samples independently without knowledge of clinicopathological data and patient outcomes.
Construction and validation of recurrence-model
A recurrence-model that integrated TMS and IS was built by logistic regression for early recurrence in stage I NSCLC. We randomly selected 70% of patients for training cohort and 30% for testing (internal validation) cohort by using the “caret” package in R. Two cohorts had similar clinicopathological features. To calculate the recurrence risk score, we used the following formula in both cohorts:
Recurrence risk score = TMS ×6.55658858436019−IS ×0.748938863395558+0.767461882293273
Patients were identified as having high early recurrence risk if their recurrence risk score was ≥−0.091. ROC curves were conducted to evaluate the discrimination of recurrence-model in both cohorts. Calibration curve and Hosmer-Lemeshow (HL) test were performed to evaluate the calibration of the model. Results of calibration were used to update the models if necessary.
External datasets from Western populations
To validate results from the local cohort, we enrolled 97 stage I NSCLC patients who had recurrence within three years (early recurrence group, n=59) and had no recurrence more than five years (non-recurrence group, n=38) from The Cancer Genome Atlas (TCGA) cohort.
Statistical analysis
Statistical analysis was conducted in GraphPad Prism software (version 7.00, La Jolla, CA) and R Statistical Software (version 4.0.5). For the continuous predictors such as age, TMB, TMS, and immune cell density, the Mann-Whitney U test was performed to analyze the correlations of early recurrence with them. For discrete variables such as gender, histologic type, and smoking history, chi-square or fisher’s exact tests were used. Lasso regression analysis was performed by the “glmnet” package (25) in R to screen recurrence-associated gene mutations and build TMS. Propensity matching score was performed by the “Matchit” package (26) in R to match the TCGA cohort and local cohort (1:1). Logistic regression was performed to identify independent predictors and construct a recurrence-model. We used the “missForest” package (27) to impute missing data algorithms. P<0.05 was considered statistically significant. And P<0.005 was considered statistically significant for correction of type I error when we performed chi-square or fisher’s exact tests to select recurrence-associated gene mutations.
Results
Clinical characteristics and early recurrence in local and TCGA cohort
A total of 130 patients were enrolled, with 69 (53.1%) in the early recurrence group and 61 (46.9%) in the non-recurrence group. The median follow-up was 13.5 months for the early recurrence group and 77 months for the non-recurrence group. The local cohort included 85 men and 45 women with a median age of 59.7 years (range from 29 to 75 years). 99 (76.2%) patients had lung adenocarcinoma (LUAD), and 31 (23.8%) had squamous cell carcinoma (LUSC). LUAD patients had a higher risk of recurrence compared with LUSC patients (P=0.008). Other clinical characteristics, including age, sex, smoking history, grade of differentiation, tumor site, pleura invasion, bronchial invasion, tumor size, and tumor stage, showed no significant association with early recurrence (all P>0.05; Table 1). In LUAD, smoking history correlated with a higher risk of early recurrence (P=0.029), while in LUSC, all clinical factors had no association with recurrence risk. Consistent with the local cohort, early recurrence also significantly correlated with histologic type and had no association with age, gender, smoking history, tumor sites, and stage in the TCGA cohort (P=0.001, 0.363, 0.605, 0.130, 0.114, and 0.516; Table S1).
Table 1
Characteristic | Early recurrence (n=69) | Non-recurrence (n=61) | P value |
---|---|---|---|
Age (mean, years) | 59 | 61 | 0.236 |
Gender (%) | 0.966 | ||
Male | 45 (65.2) | 40 (65.6) | |
Female | 24 (34.8) | 21 (34.4) | |
Grade (%) | 0.211 | ||
Well differentiated | 7 (10.1) | 11 (18.0) | |
Moderately differentiated | 34 (49.3) | 33 (54.1) | |
Poorly differentiated | 28 (40.6) | 17 (27.9) | |
Histologic type (%) | 0.008 | ||
Adenocarcinoma | 59 (85.5) | 40 (65.5) | |
Squamous carcinoma | 10 (14.5) | 21 (34.4) | |
Smoking history (%) | 0.278 | ||
Never smoker | 40 (58.0) | 41 (67.2) | |
Former or current smoker | 29 (42.0) | 20 (32.8) | |
Tumor site (%) | 0.568 | ||
Right lung | 35 (50.7) | 34 (55.7) | |
Left lung | 34 (49.3) | 27 (44.3) | |
Pleural invasion (%) | 0.094 | ||
No | 25 (36.2) | 31 (50.8) | |
Yes | 44 (63.8) | 30 (49.2) | |
Bronchus invasion (%) | 0.264 | ||
No | 62 (89.9) | 58 (95.1) | |
Yes | 7 (10.1) | 3 (4.9) | |
T stage (%) | 0.261 | ||
T1a | 1 (1.4) | 4 (6.6) | |
T1b | 21 (30.4) | 19 (31.1) | |
T1c | 30 (43.5) | 19 (31.1) | |
T2a | 17 (24.6) | 19 (31.1) | |
Stage (%) | 0.651 | ||
IA | 19 (27.5) | 19 (31.1) | |
IB | 50 (72.5) | 42 (68.9) | |
Surgical resection (%) | 0.498 | ||
Sublobar resection | 67 (97.1) | 61 (100.0) | |
Lobectomy | 2 (2.9) | 0 (0) | |
Adjuvant chemotherapy (%) | 0.218 | ||
No | 69 (100) | 59 (96.7) | |
Yes | 0 (0) | 2 (3.3) |
Comparing genomic background between local cohort and the TCGA cohort
Genomic profiling revealed a total of 1,080 and 478 mutant genes, non-overlapping mutant genes in the early recurrence and non-recurrence groups, and 150 overlapping mutant genes in both groups (Figure 1A). To compare the genomic background between two cohorts, we selected patients with WES data in the local cohort (n=74) and whole TCGA cohort. We compared the top 40 mutated genes and only found three common genes (TP53, AHNAK2, and TTN; Figure 1B). We noticed that some clinical features differed between the two cohorts (Table S2). In order to eliminate the influence of clinical features on genomic alteration. We selected matched patients with the same number (n=74) and similar clinical characteristics from the TCGA cohort by performing propensity score matching (PSM) (Table 2). When comparing the matched TCGA cohort and local cohort, we still only found three common genes (TP53, AHNAK2, and TTN; Figure 1C). Figure 1D,1E showed the top 40 genes in the local and TCGA cohort. In local cohort, Mucin-related antigen 4 (MUC4) was the most frequently altered gene (24%), followed by TP53 (23%). In TCGA cohort, TP53 had the highest mutation rate (67%), while only 5% of patients exhibited MUC4 mutation.
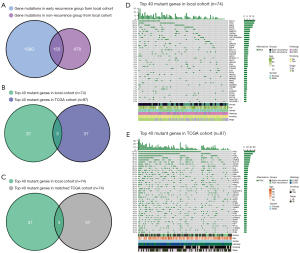
Table 2
Clinical characteristic | Local cohort (n=130) | TCGA cohort (n=97) | P value |
---|---|---|---|
Groups (%) | 0.244 | ||
Early recurrence | 69 (53.1) | 59 (60.8) | |
Non-recurrence | 61 (46.9) | 38 (39.2) | |
Age (mean) | 61 | 68 | <0.0001 |
Gender (%) | |||
Male | 85 (65.4) | 53 (54.6) | 0.101 |
Female | 45 (34.6) | 44 (45.4) | |
Histology (%) | 0.020 | ||
Adenocarcinoma | 99 (76.2) | 60 (61.9) | |
Squamous carcinoma | 31 (23.8) | 37 (38.1) | |
Smoking history (%) | <0.0001 | ||
No | 81 (62.3) | 11 (11.3) | |
Yes | 49 (37.7) | 86 (88.7) | |
Tumor sites (%) | 0.520 | ||
Left | 54 (42.4) | 46 (46.5) | |
Right | 74 (57.8) | 53 (53.5) | |
Stage (%) | 0.028 | ||
IA | 38 (29.2) | 42 (43.3) | |
IB | 92 (70.8) | 55 (56.7) |
TCGA, the Cancer Genome Atlas.
Genomic alteration and early recurrence
Identification and validation recurrence-associated gene mutations through univariate analysis
By chi-sq test or Fisher exact test, we analyzed the relationship between mutations and recurrence risk. MUC4 mutation was significantly associated with early recurrence in our cohort (P=0.0008; Figure 2). Notably, all of the MUC4 mutations identified were clustered in exon 2. MUC4 mutation also correlated with early recurrence in LUAD and LUSC (P=0.035 and 0.044, respectively; not show). EGFR mutation was exclusively found in LUAD (24.3% of LUAD) but had no association with early recurrence (P=0.1510; Figure 2). TP53 also displayed no correlation in the risk of early recurrence (P=0.4092; Figure 2). In the TCGA cohort, EGFR and TP53 did not correlate with early recurrence, consistent with the local cohort (P=0.6626 and 0.3764, respectively; Figure 2). While MUC4 mutation had no correlation with early recurrence in the TCGA cohort (P=0.6435; Figure 2).
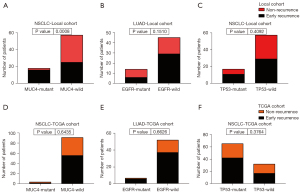
TMB and early recurrence
We calculated TMB in 74 samples from WES data and 14 samples from targeted sequencing panels. Considering the data from WES might be enough for analysis and the heterogeneity between different platforms might lead to more statistical errors even after adjustment, we did not use the TMB data from targeted sequencing panels. Median TMB for WES was 1.25 and 0.72 per Mb for early recurrence and non-recurrence groups, respectively. The early recurrence group exhibited higher TMB (P=0.0057; Figure 3). Further analysis showed that higher TMB correlated with higher recurrence risk in LUAD and LUSC (P=0.031 and 0.05, respectively; Figure 3). In the TCGA cohort, early recurrence only had a significant correlation with high TMB in LUAD patients (P=0.029; Figure 3), while early recurrence had no association with TMB in patients with NSCLC or LUSC (P=0.49 and 0.31; Figure 3).
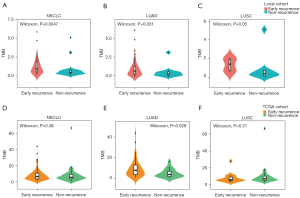
GO and KEGG enrichment analysis for mutant genes
KEGG enrichment analysis showed that Ras signaling, proteoglycans in cancer, cell adhesion molecules pathway were only and significantly enriched in the early recurrence group (Figure S1A). GO analysis also revealed some biological processes (BP) were only enriched in the early recurrence group, including DNA transcription, calcium-dependent cell-cell adhesion. For cell component (CC), nucleus and membrane were enriched in early recurrence group but not in the non-recurrence group (Figure S1B,S1C). In addition, molecular function (MF) analysis found that metal ion binding was exclusively enriched in the early recurrence group (Figure S1D).
Copy number variation and early recurrence
The early recurrence group showed more copy number amplifications and deletions (Figure S2A,S2B). Figure S2C showed the top 40 copy number variations. We also found that the chromosome sites of copy number variation differed in both groups. Amplifications in chromosome 8 and deletions in chromosomes 9, 14, and 17 were only identified in the early recurrence group (Figure S2D-S2G). KEGG pathway analysis showed pathways with copy number amplification were similar in both groups, while pathways with copy number deletion were much more in the early recurrence group compared with the non-recurrence group (Figure S2H,S2I). For example, copy number deletions in Wnt, TGF-beta, PI3K-Akt, P53, Notch, and mTOR signaling pathways were only identified in the recurrence group.
TMS based on the results of Lasso regression had significant association with early recurrence
We performed Lasso regression and identified 11 gene mutations associated with early recurrence: MUC4, KRTAP10-6, ZFHX4, TCF20, PRDM7, MEOX2, NPIPB5, FAM173B, ACP2, SCN5A, NSRP1 (Figure 4A-4C). We built TMS in 74 samples, since MUC4 was not included in targeted sequencing (Figure 4A-4C). The formula of TMS was in the supplementary method (Appendix 1). The TMS in the recurrence group was evenly distributed, while in the non-recurrence group, TMS was generally low (Figure 4D). ROC curve showed that TMS had a higher area under curve (AUC) value than MUC4 mutation and TMB (AUC =0.869, 0.717, and 0.671; Figure 4E). The early recurrence group showed significantly higher TMS in stage I NSCLC (P<0.0001), LUAD (P<0.0001), and LUSC (P=0.0028) in the local cohort (Figure 5). Consistent with local cohort, early recurrence also exhibited higher TMS in LUAD patients from the TGCA cohort (P=0.031; Figure 5). However, in LUSC and the whole cohort, TMS had no association with early recurrence (P=0.07 and 0.87, respectively; Figure 5).
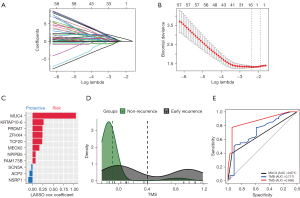
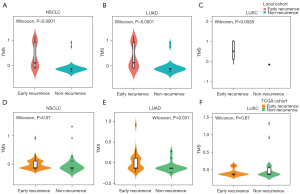
Immune signatures and early recurrence
We assessed tumor infiltration of CD3+ and CD8+ TILs in tumor center (CT) and invasive margin (IM). Figure 6 showed a representative immunochemical staining of CD3 and CD8+ TILs in CT and IM. Tumors from the non-recurrence group showed higher density of CD3-CT, CD3-IM, CD8-CT, and CD8-IM+ TILs (P=0.0202, 0.0025, 0.0170 and 0.0392, respectively; Figure 7A-7D). Eleven (9.6%) patients had PD-L1 TPS ≥1% and 30 (28.0%) patients had CPS ≥1. The early recurrence group had similar PD-L1 expression (P=0.8727 for TPS, P=0.3578 for CPS; Figure 7E,7F). IS was built based on the tumor infiltration location and density of CD3+ and CD8+ TILs (Figure 7G). IS0 and IS4 account for the largest proportion in the early recurrence and non-recurrence groups, respectively (Figure 7H). Patients with IS0 and IS1 showed similar recurrence risk. Recurrence risk decreased and negatively correlated with IS when IS ≥2 (Figure 7I). The early recurrence group showed significantly lower IS (P=0.0003; Figure 7J). ROC curve analysis suggested that IS had a better prognostic value than CD3+ or CD8+ TILs alone (AUC =0.717 for IS; all AUC <0.7 for CD3+ or CD8+ T cells; Figure 7K).
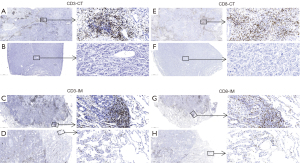
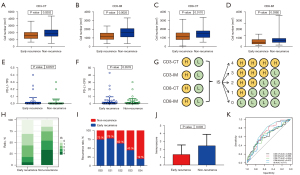
Development and validation of recurrence-model
For multivariate analysis, four factors that were statistically significant in the univariate analysis were enrolled, including histologic type, IS, TMS, and TMB. Besides, staging and grade were also enrolled since they are well-defined prognostic variables. The results showed that low IS (<2) and high TMS (<50%) were independent risk factors for early recurrence in stage I NSCLC (P=0.001 for IS, P<0.0001 for TMS; Figure 8A). Next, we built the following recurrence-model that integrated IS and TMS in the training cohort and visualized the model with a nomogram (Figure 8B):
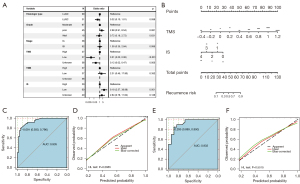
Prognostic index = 0.7674619−0.7489389 × immunoscore + 6.5565886 × TMS
The model had great discrimination (ROC curve, AUC =0.935) and calibration (HL test, P=0.2885) in the training cohort (Figure 8C,8D). In the testing cohort, the recurrence-model also had great discrimination (ROC curve, AUC =0.932) and calibration (HL test, P=0.5515; Figure 8E,8F).
The relationship between genomic alterations and immune signatures
We compared TMS in patients with different IS levels and found that TMS had no association with IS (Figure 9A). TMS had no association with PD-L1 TPS and CPS (P=0.2017 and 0.9527, respectively; Figure 9B,9C). Similarly, we found that TMB did not correlate with IS and PD-L1 expression (Figure 9D-9F). Patients with high TMB mainly exhibited high TMS, and patients with low TMB mainly had low TMS (Figure 9G). Next, we screened IS-associated gene mutations by Lasso regression (Figure 9H,9I). Fourteen genes were identified: C16orf3, FH, PER3, MUC20, RNF31, TMEM229A, ELN, IL4I1, ZNF208, ESRRA, ZAN, KALRN, MUC17, and OR2V2. Among these, C16orf3 had the highest coefficients. There was no intersection between the 14 IS-associated gene mutations and11 TMS-associated gene mutations (Figure 9J).
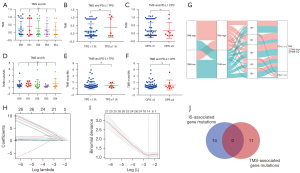
Discussion
Surgery is the primary treatment for stage I NSCLC. However, the prognosis of these patients remains unsatisfactory because of postoperative recurrence. Almost one-third of patients relapse after surgery, and half of them succumb to the disease in the first year (3). Until now, there has been no effective prognostic biomarker and perioperative treatment. Perioperative targeted therapy and immunotherapy have made breakthroughs in recent years for NSCLC, while few studies enrolled stage I patients. The phase III ADAURA study enrolled patients with stage IB NSCLC and found that Osimertinib improved disease-free survival (DFS) in patients with stage IB (28). Recently, the phase III study IMpower-010 reported that stage IB NSCLC patients did not benefit from atezolizumab compared with best supportive care after adjuvant chemotherapy (6). Another phase III study, Checkmate-816, reported that more patients had complete pathological remission in the treatment of nivolumab plus chemotherapy compared with chemotherapy in patients with stage IB NSCLC (7). These studies suggested stage I NSCLC might benefit from perioperative targeted therapy and immunotherapy, but not as good as stage II/III. The lack of understanding the relevant background of the genomic alteration and immune microenvironment limits the application of perioperative treatment in stage I NSCLC.
Among TMS 11 genes, MUC4 had the highest prognostic value. MUC4 gene is located in the q29 region of chromosome 3, containing 26 exons (29). Previous studies have reported that MUC4 overexpression was associated with tumor progression and metastasis in NSCLC (30,31). However, few studies reported the role of MUC4 mutation in NSCLC. The MUC4 mutation rate was 24.3% in the local cohort and correlated with early recurrence. We also noticed that all the mutating sites of MUC4 were clustered in exon 2. It has been reported that splicing of exon 2 in MUC4 gene could induce tumor metastasis in pancreatic cancer cells by activating the Notch signaling pathway (32). However, MUC4 mutation had no correlation with recurrence in the TCGA cohort, possibly because of the low mutation rate. In general, MUC4 mutation might play an important role in tumor recurrence for patients with stage I NSCLC, and the detailed mechanism needs to be further studied. Besides MUC4, MEOX2 was also closely associated with tumor progression and metastasis (33,34). In comparison, few studies reported the role of the other TMS 11 genes in cancer.
TMB is defined as the number of nonsynonymous mutations in a tumor (35). Owada-Ozaki et al. reported that higher TMB was associated with higher recurrence risk in 67 Japanese stage I NSCLC (12). On the contrary, Tian et al. found higher TMB correlated with lower recurrence rate in a cohort of 89 stage IA-IIIA Chinese NSCLC (36). In our study, high TMB correlated with early recurrence in stage I NSCLC, which was consistent with the results of Owada-Ozaki et al. However, no significant difference was found between TMB and early recurrence in multivariate analysis. To explore the relationship between gene mutations and early recurrence, we analyzed each gene separately and calculated the total mutation status-TMB. However, the separate analyses might miss some related genes because of the limited sample size. And TMB might involve genes that had no association with the early recurrence, which attenuated its prognostic value. Our study defined a novel TMS, a scoring system developed only by recurrence-associated gene mutations. The TMS had a much higher prognostic value than MUC4 gene mutation and TMB. Lu et al. and Li et al. constructed similar TMS to predict the efficacy of immunotherapy in NSCLC (37,38). However, few studies focused on tumor recurrence in stage I NSCLC. Generally, advanced NSCLC may present molecular and cellular features distinct from early-stage NSCLC (39). Taken together, we developed a novel, feasible, and powerful genomic biomarker for the prediction of early recurrence in stage I NSCLC.
Nevertheless, it should be noted that MUC4, TMB, and TMS all showed different prognostic values between the local and TCGA cohort. We also found there were few common genes between the two cohorts. Previous studies reported that significant ethnic differences exist between eastern and western NSCLC (40,41), which might attenuate the value of TMS in western NSCLC.
CD3+ and CD8+ TILs are frequently present in TIME, both implicated in tumor recurrence (42,43). However, the roles of CD3+ and CD8+ TILs were controversial (44,45). Recently, Enfield et al. reported that the prognostic value of CD8+ TILs in NSCLC might depend on the localization within TIME (42). Our results showed that high CD3+ and CD8 +TILs in CT and IM were significantly associated with lower early recurrence risk in stage I NSCLC. Galon et al. firstly reported that IS, which integrated the density of CD3+ and CD8+ TILs within tumor center and invasive margin, had excellent prognostic value for early-stage colon cancer (46). Further study showed that low IS significantly correlated with recurrence and its prognostic value was better than the TNM staging system in colon cancer (16). However, no relevant study reported the prognostic value of the IS in NSCLC. The present study found that the IS was an independent prognostic predictor for early recurrence in stage I NSCLC. Besides, IS had a higher prognostic value than CD3+ and CD8+ TILs alone. Thus, we used the IS to construct the model instead of CD3+ or CD8+ TILs alone, which simplified and improved the performance of our model.
Previous studies have proposed prediction models that consist of clinical-pathological features or radiomics signatures, which could improve the estimation of disease recurrence for early-stage NSCLC compared with conventional TNM staging systems (17,47). Several studies constructed models that integrated different molecular features, such as DNA mRNA expression, methylation, microRNA expression, gene mutation (24,48-50). A model based on 138 cancer-related gene mutations showed independent prognostic value for disease-free survival in patients with resected stage I–III NSCLC (9). Recently, our team reported that a model based on five-immune gene expression was related to tumor early recurrence in stage I NSCLC (23). This study developed and validated a comprehensive recurrence-model based on TMS and IS, both of which were independent predictors for early recurrence. The model had great discrimination in the training and testing cohort (AUC =0.935 and 0.932, respectively). The calibration curve and HL-test suggested the model also had great calibration. Although genomic and immune microenvironment alteration plays a critical role in tumor recurrence, few studies integrated gene mutation features and immune signatures to build a comprehensive model. Compared with previous models, our model was comprised of molecular features from both gene mutation and immune microenvironment aspects, which might provide a reliable biomarker for predicting early recurrence risk and evidence for studies of perioperative treatment. Nomograms are widely used as prognostic devices in oncology and medicine. We also created a nomogram to visualize the prediction mode, making it more convenient for clinical application. According to the nomogram, we can calculate the specific risk of early recurrence for patients with stage I NSLCC after surgical resection.
EGFR and PD-L1 were biomarkers for the efficacy of targeted therapy and immunotherapy (51,52), while their prognostic value for stage I NSCLC was unclear. Izar et al. reported that stage I NSCLC patients with EGFR mutation had better DFS (53). In contrast, Takamochi et al. reported that patients with EGFR mutation showed longer DFS in stage I-III LUAD patients but had no correlation with DFS in stage I LUAD (10). In this study, we found that EGFR mutation had no association with DFS in two cohorts, which was consistent with recent studies. A study showed that PD-L1 positive expression correlated with a lower recurrence risk in stage I LUAD (15). By contrast, another study reported that patients with positive PD-L1 positive expression exhibited higher recurrence risk in stage I NSCLC (54). Other studies also reported that PD-L1 expression correlated with tumor metastasis only in LUAD but not in LUSC (55). In our study, PD-L1 expression had no correlation with early recurrence in stage I NSCLC patients, neither in LUAD nor in LUSC. Taken together, the commonly used biomarkers for targeted therapy and immunotherapy had no prognostic value for stage I NSCLC, which might explain why stage I NSCLC did not benefit from perioperative treatment. In comparison, our model could distinguish patients with high recurrence risk very well.
Gene mutations could directly affect the immune microenvironment by recruiting or excluding immune cell infiltration (56). For example, TP53 mutation activates NF-κB signaling pathway and promotes tumor infiltration of immune cells (57). However, Kadara et al. reported that neither EGFR nor TP53 had a correlation with TILs in early-stage NSCLC (58). Similarly, we found that patients with EGFR and TP53 had no association with CD3+, CD8+ TILs in stage I NSCLC. To further explore the relationship between genomic and immune alterations, we identified 14 gene mutations correlated with IS. Among 14 IS-associated genes, some are closely related to the tumor immune microenvironment status. For example, IL4I1 inhibited antitumor T-cell proliferation and activation in vivo (59,60). PER3 was the core gene of the circadian clock. Studies reported that abnormal circadian clock contributes to T cell exhaustion and global upregulation of immune inhibitory molecules such as PD-L1 and CTLA-4 (61). RNF31 is up-regulated in regulatory T cells (Tregs) and positively regulates FOXP3 stability and Treg cell function (62). KALRN mutations promote antitumor immunity and immunotherapy response by regulating DNA damage repair pathways in cancer (63). Few studies reported the relationship between the rest of the 14 IS-associated genes and immune. However, there is no intersection between 14 IS-associated and 11 TMS-associated genes. Besides, TMS had no association with IS. These results suggested that the association between gene mutation and immune microenvironment might be weak in stage I NSCLC. They contributed to tumor early recurrence independently, which made it more necessary to construct an integrated model instead of a model consisting of gene mutation or immune signature alone. This paper had several limitations. First, the present study was a single-center study. The lack of external validation might attenuate the power of our model. Second, this study was retrospective, and some clinical-pathological features were missed.
In conclusion, we presented a comprehensive analysis of genomic alterations and immune signatures of TIME. We built an integrated recurrence-model that had high prognostic value for patients with stage I NSCLC. Our study provided a novel biomarker for identifying patients with high early recurrence risk and might provide evidence for future translational research about perioperative treatment.
Acknowledgments
Funding: This research was supported by: (I) CSCO-Merk Oncologic Research Foundation (Grant No. Y-MSDPU2021-0253); (II) The Health Commission of Hunan Province (Grant No. 202103100948); (III) CSCO-BMS Oncologic Research Foundation (Grant No. Y-BMS2019-100); (IV) Guangdong Provincial Key Lab of Translational Medicine in Lung Cancer (Grant No. 2017B030314120); (V) Guangdong Association of Clinical Trials (GACT)/Chinese Thoracic Oncology Group (CTONG); (VI) Natural Science Foundation of Hunan Province for Excellent Young Scholars (Grant No.2021JJ20088); (VII) Changsha Science and Technology Bureau (Grant No. kq1907077).
Footnote
Reporting Checklist: The authors have completed the TRIPOD reporting checklist. Available at https://tlcr.amegroups.com/article/view/10.21037/tlcr-21-751/rc
Data Sharing Statement: Available at https://tlcr.amegroups.com/article/view/10.21037/tlcr-21-751/dss
Peer Review File: Available at https://tlcr.amegroups.com/article/view/10.21037/tlcr-21-751/prf
Conflicts of Interest: All authors have completed the ICMJE uniform disclosure form (available at https://tlcr.amegroups.com/article/view/10.21037/tlcr-21-751/coif). The authors have no conflicts of interest to declare.
Ethical Statement: The authors are accountable for all aspects of the work in ensuring that questions related to the accuracy or integrity of any part of the work are appropriately investigated and resolved. The protocol of this study was approved by the institutional review board of Second Xiangya Hospital of Central South University (No. 2020010), and all patients signed informed consent. All procedures performed in this study involving human participants were conformed to the Declaration of Helsinki (as revised in 2013).
Open Access Statement: This is an Open Access article distributed in accordance with the Creative Commons Attribution-NonCommercial-NoDerivs 4.0 International License (CC BY-NC-ND 4.0), which permits the non-commercial replication and distribution of the article with the strict proviso that no changes or edits are made and the original work is properly cited (including links to both the formal publication through the relevant DOI and the license). See: https://creativecommons.org/licenses/by-nc-nd/4.0/.
References
- Siegel RL, Miller KD, Jemal A. Cancer statistics, 2020. CA Cancer J Clin 2020;70:7-30. [Crossref] [PubMed]
- National Lung Screening Trial Research Team. Reduced lung-cancer mortality with low-dose computed tomographic screening. N Engl J Med 2011;365:395-409. [Crossref] [PubMed]
- Consonni D, Pierobon M, Gail MH, et al. Lung cancer prognosis before and after recurrence in a population-based setting. J Natl Cancer Inst 2015;107:djv059. [Crossref] [PubMed]
- Pathak R, Goldberg SB, Canavan M, et al. Association of Survival With Adjuvant Chemotherapy Among Patients With Early-Stage Non-Small Cell Lung Cancer With vs Without High-Risk Clinicopathologic Features. JAMA Oncol 2020;6:1741-50. [Crossref] [PubMed]
- Forde PM, Chaft JE, Smith KN, et al. Neoadjuvant PD-1 Blockade in Resectable Lung Cancer. N Engl J Med 2018;378:1976-86. [Crossref] [PubMed]
- Wakelee HA, Altorki NK, Zhou C, et al. IMpower010: Primary results of a phase III global study of atezolizumab versus best supportive care after adjuvant chemotherapy in resected stage IB-IIIA non-small cell lung cancer (NSCLC). J Clin Oncol 2021;39:8500. [Crossref]
- Spicer J, Wang C, Tanaka F, et al. Surgical outcomes from the phase 3 CheckMate 816 trial: Nivolumab (NIVO) + platinum-doublet chemotherapy (chemo) vs chemo alone as neoadjuvant treatment for patients with resectable non-small cell lung cancer (NSCLC). J Clin Oncol 2021;39:8503. [Crossref]
- Vogelstein B, Papadopoulos N, Velculescu VE, et al. Cancer genome landscapes. Science 2013;339:1546-58. [Crossref] [PubMed]
- Ono A, Isaka M, Serizawa M, et al. Genetic alterations of driver genes as independent prognostic factors for disease-free survival in patients with resected non-small cell lung cancer. Lung Cancer 2019;128:152-7. [Crossref] [PubMed]
- Takamochi K, Oh S, Matsunaga T, et al. Prognostic impacts of EGFR mutation status and subtype in patients with surgically resected lung adenocarcinoma. J Thorac Cardiovasc Surg 2017;154:1768-1774.e1. [Crossref] [PubMed]
- Chaft JE, Dagogo-Jack I, Santini FC, et al. Clinical outcomes of patients with resected, early-stage ALK-positive lung cancer. Lung Cancer 2018;122:67-71. [Crossref] [PubMed]
- Owada-Ozaki Y, Muto S, Takagi H, et al. Prognostic Impact of Tumor Mutation Burden in Patients With Completely Resected Non-Small Cell Lung Cancer: Brief Report. J Thorac Oncol 2018;13:1217-21. [Crossref] [PubMed]
- Singal G, Miller PG, Agarwala V, et al. Association of Patient Characteristics and Tumor Genomics With Clinical Outcomes Among Patients With Non-Small Cell Lung Cancer Using a Clinicogenomic Database. JAMA 2019;321:1391-9. [Crossref] [PubMed]
- Keller MD, Neppl C, Irmak Y, et al. Adverse prognostic value of PD-L1 expression in primary resected pulmonary squamous cell carcinomas and paired mediastinal lymph node metastases. Mod Pathol 2018;31:101-10. [Crossref] [PubMed]
- Yang CY, Lin MW, Chang YL, et al. Programmed cell death-ligand 1 expression in surgically resected stage I pulmonary adenocarcinoma and its correlation with driver mutations and clinical outcomes. Eur J Cancer 2014;50:1361-9. [Crossref] [PubMed]
- Pagès F, Mlecnik B, Marliot F, et al. International validation of the consensus Immunoscore for the classification of colon cancer: a prognostic and accuracy study. Lancet 2018;391:2128-39. [Crossref] [PubMed]
- Kang YK, Song YS, Cho S, et al. Prognostic stratification model for patients with stage I non-small cell lung cancer adenocarcinoma treated with surgical resection without adjuvant therapies using metabolic features measured on F-18 FDG PET and postoperative pathologic factors. Lung Cancer 2018;119:1-6. [Crossref] [PubMed]
- Li J, Zheng Q, Zhao X, et al. Nomogram model for predicting cause-specific mortality in patients with stage I small-cell lung cancer: a competing risk analysis. BMC Cancer 2020;20:793. [Crossref] [PubMed]
- Qiu B, Ji Y, He H, et al. Three-dimensional reconstruction/personalized three-dimensional printed model for thoracoscopic anatomical partial-lobectomy in stage I lung cancer: a retrospective study. Transl Lung Cancer Res 2020;9:1235-46. [Crossref] [PubMed]
- Khorrami M, Bera K, Leo P, et al. Stable and discriminating radiomic predictor of recurrence in early stage non-small cell lung cancer: Multi-site study. Lung Cancer 2020;142:90-7. [Crossref] [PubMed]
- Dissaux G, Visvikis D, Da-Ano R, et al. Pretreatment 18F-FDG PET/CT Radiomics Predict Local Recurrence in Patients Treated with Stereotactic Body Radiotherapy for Early-Stage Non-Small Cell Lung Cancer: A Multicentric Study. J Nucl Med 2020;61:814-20. [Crossref] [PubMed]
- Chen P, Zhang L, Zhang W, et al. Galectin-9-based immune risk score model helps to predict relapse in stage I-III small cell lung cancer. J Immunother Cancer 2020;8:e001391. [Crossref] [PubMed]
- Wang Q, Zhou D, Wu F, et al. Immune Microenvironment Signatures as Biomarkers to Predict Early Recurrence of Stage Ia-b Lung Cancer. Front Oncol 2021;11:680287. [Crossref] [PubMed]
- Tamiya A, Koh Y, Isa SI, et al. Impact of somatic mutations on prognosis in resected non-small-cell lung cancer: The Japan Molecular Epidemiology for lung cancer study. Cancer Med 2020;9:2343-51. [Crossref] [PubMed]
- Friedman J, Hastie T, Tibshirani R. Regularization Paths for Generalized Linear Models via Coordinate Descent. J Stat Softw 2010;33:1-22. [Crossref] [PubMed]
- Austin PC. An Introduction to Propensity Score Methods for Reducing the Effects of Confounding in Observational Studies. Multivariate Behav Res 2011;46:399-424. [Crossref] [PubMed]
- Stekhoven DJ, Bühlmann P. MissForest--non-parametric missing value imputation for mixed-type data. Bioinformatics 2012;28:112-8. [Crossref] [PubMed]
- Wu YL, Tsuboi M, He J, et al. Osimertinib in Resected EGFR-Mutated Non-Small-Cell Lung Cancer. N Engl J Med 2020;383:1711-23. [Crossref] [PubMed]
- Gross MS, Guyonnet-Duperat V, Porchet N, et al. Mucin 4 (MUC4) gene: regional assignment (3q29) and RFLP analysis. Ann Genet 1992;35:21-6. [PubMed]
- Majhi PD, Lakshmanan I, Ponnusamy MP, et al. Pathobiological implications of MUC4 in non-small-cell lung cancer. J Thorac Oncol 2013;8:398-407. [Crossref] [PubMed]
- Tsutsumida H, Goto M, Kitajima S, et al. MUC4 expression correlates with poor prognosis in small-sized lung adenocarcinoma. Lung Cancer 2007;55:195-203. [Crossref] [PubMed]
- Tang J, Zhu Y, Xie K, et al. The role of the AMOP domain in MUC4/Y-promoted tumour angiogenesis and metastasis in pancreatic cancer. J Exp Clin Cancer Res 2016;35:91. [Crossref] [PubMed]
- Wang Z, Yang H, Zhang R, et al. MEOX2 serves as a novel biomarker associated with macrophage infiltration in oesophageal squamous cell carcinoma and other digestive system carcinomas. Autoimmunity 2021;54:373-83. [Crossref] [PubMed]
- Liu Y, Cheng L, Li C, et al. Identification of tumor microenvironment-related prognostic genes in colorectal cancer based on bioinformatic methods. Sci Rep 2021;11:15040. [Crossref] [PubMed]
- Meléndez B, Van Campenhout C, Rorive S, et al. Methods of measurement for tumor mutational burden in tumor tissue. Transl Lung Cancer Res 2018;7:661-7. [Crossref] [PubMed]
- Tian Y, Xu J, Chu Q, et al. A novel tumor mutational burden estimation model as a predictive and prognostic biomarker in NSCLC patients. BMC Med 2020;18:232. [Crossref] [PubMed]
- Li Y, Chen Z, Tao W, et al. Tumor mutation score is more powerful than tumor mutation burden in predicting response to immunotherapy in non-small cell lung cancer. Cancer Immunol Immunother 2021;70:2367-78. [Crossref] [PubMed]
- Lu M, Wu KH, Trudeau S, et al. A genomic signature for accurate classification and prediction of clinical outcomes in cancer patients treated with immune checkpoint blockade immunotherapy. Sci Rep 2020;10:20575. [Crossref] [PubMed]
- Kelemen O, Pla I, Sanchez A, et al. Proteomic analysis enables distinction of early- versus advanced-stage lung adenocarcinomas. Clin Transl Med 2020;10:e106. [Crossref] [PubMed]
- Chen J, Yang H, Teo ASM, et al. Genomic landscape of lung adenocarcinoma in East Asians. Nat Genet 2020;52:177-86. [Crossref] [PubMed]
- Jiang T, Shi J, Dong Z, et al. Genomic landscape and its correlations with tumor mutational burden, PD-L1 expression, and immune cells infiltration in Chinese lung squamous cell carcinoma. J Hematol Oncol 2019;12:75. [Crossref] [PubMed]
- Enfield KSS, Martin SD, Marshall EA, et al. Hyperspectral cell sociology reveals spatial tumor-immune cell interactions associated with lung cancer recurrence. J Immunother Cancer 2019;7:13. [Crossref] [PubMed]
- Karasaki T, Qiang G, Anraku M, et al. High CCR4 expression in the tumor microenvironment is a poor prognostic indicator in lung adenocarcinoma. J Thorac Dis 2018;10:4741-50. [Crossref] [PubMed]
- Zeng DQ, Yu YF, Ou QY, et al. Prognostic and predictive value of tumor-infiltrating lymphocytes for clinical therapeutic research in patients with non-small cell lung cancer. Oncotarget 2016;7:13765-81. [Crossref] [PubMed]
- Yan X, Jiao SC, Zhang GQ, et al. Tumor-associated immune factors are associated with recurrence and metastasis in non-small cell lung cancer. Cancer Gene Ther 2017;24:57-63. [Crossref] [PubMed]
- Galon J, Costes A, Sanchez-Cabo F, et al. Type, density, and location of immune cells within human colorectal tumors predict clinical outcome. Science 2006;313:1960-4. [Crossref] [PubMed]
- Kent MS, Mandrekar SJ, Landreneau R, et al. A Nomogram to Predict Recurrence and Survival of High-Risk Patients Undergoing Sublobar Resection for Lung Cancer: An Analysis of a Multicenter Prospective Study (ACOSOG Z4032). Ann Thorac Surg 2016;102:239-46. [Crossref] [PubMed]
- Haro GJ, Sheu B, Cook NR, et al. Comparison of Conventional TNM and Novel TNMB Staging Systems for Non-Small Cell Lung Cancer. JAMA Netw Open 2019;2:e1917062. [Crossref] [PubMed]
- Luo WM, Wang ZY, Zhang X. Identification of four differentially methylated genes as prognostic signatures for stage I lung adenocarcinoma. Cancer Cell Int 2018;18:60. [Crossref] [PubMed]
- Li W, Liu S, Su S, et al. Construction and validation of a novel prognostic signature of microRNAs in lung adenocarcinoma. PeerJ 2021;9:e10470. [Crossref] [PubMed]
- Sigismund S, Avanzato D, Lanzetti L. Emerging functions of the EGFR in cancer. Mol Oncol 2018;12:3-20. [Crossref] [PubMed]
- Yu H, Boyle TA, Zhou C, et al. PD-L1 Expression in Lung Cancer. J Thorac Oncol 2016;11:964-75. [Crossref] [PubMed]
- Izar B, Sequist L, Lee M, et al. The impact of EGFR mutation status on outcomes in patients with resected stage I non-small cell lung cancers. Ann Thorac Surg 2013;96:962-8. [Crossref] [PubMed]
- Handa Y, Tsutani Y, Shiroma N, et al. Prognostic Impact of Programmed Death-ligand 1 and Surrounding Immune Status on Stage I Lung Cancer. Clin Lung Cancer 2020;21:e302-14. [Crossref] [PubMed]
- Sun JM, Zhou W, Choi YL, et al. Prognostic Significance of PD-L1 in Patients with Non-Small Cell Lung Cancer: A Large Cohort Study of Surgically Resected Cases. J Thorac Oncol 2016;11:1003-11. [Crossref] [PubMed]
- Wellenstein MD, de Visser KE. Cancer-Cell-Intrinsic Mechanisms Shaping the Tumor Immune Landscape. Immunity 2018;48:399-416. [Crossref] [PubMed]
- Kastenhuber ER, Lowe SW. Putting p53 in Context. Cell 2017;170:1062-78. [Crossref] [PubMed]
- Kadara H, Choi M, Zhang J, et al. Whole-exome sequencing and immune profiling of early-stage lung adenocarcinoma with fully annotated clinical follow-up. Ann Oncol 2017;28:75-82. [Crossref] [PubMed]
- Lasoudris F, Cousin C, Prevost-Blondel A, et al. IL4I1: an inhibitor of the CD8+ antitumor T-cell response in vivo. Eur J Immunol 2011;41:1629-38. [Crossref] [PubMed]
- Puiffe ML, Dupont A, Sako N, et al. IL4I1 Accelerates the Expansion of Effector CD8+ T Cells at the Expense of Memory Precursors by Increasing the Threshold of T-Cell Activation. Front Immunol 2020;11:600012. [Crossref] [PubMed]
- Wu Y, Tao B, Zhang T, et al. Pan-Cancer Analysis Reveals Disrupted Circadian Clock Associates With T Cell Exhaustion. Front Immunol 2019;10:2451. [Crossref] [PubMed]
- Zhu F, Yi G, Liu X, et al. Ring finger protein 31-mediated atypical ubiquitination stabilizes forkhead box P3 and thereby stimulates regulatory T-cell function. J Biol Chem 2018;293:20099-111. [Crossref] [PubMed]
- Li M, Ma Y, Zhong Y, et al. KALRN mutations promote antitumor immunity and immunotherapy response in cancer. J Immunother Cancer 2020;8:e000293. [Crossref] [PubMed]