Circulating tumor cell methylation profiles reveal the classification and evolution of non-small cell lung cancer
Introduction
Lung cancer is one of the most common and deadliest forms of cancer worldwide (1). Approximately 85% of lung cancers are non-small cell lung cancers (NSCLCs), which include lung adenocarcinoma (LUAD) and lung squamous cell carcinoma (LUSC). As the main treatment options are determined according to the histologic features and the molecular profile, pathological diagnosis is key in NSCLC treatment. Currently, pathological diagnoses are based on the morphologic features or on immune-cytochemical and immune-histochemical analyses of NSCLC tissues, or cytologic samples obtained from a surgical biopsy, bronchoscopy, or bronchial brushing (2), and it is difficult to make a pathological diagnosis in cases in which tumor biopsy or cytology material is not adequate.
Circulating tumor cells (CTCs) are cancer cells that disseminate from primary or metastatic sites into the peripheral blood. CTCs have great potential as diagnostic and prognostic biomarkers, and could be used to guide the individualized treatment of lung cancer patients. In the lung cancer diagnostic field, the CTC detection rate is approximately 87% among those who have >3 CTCs per 3.2 mL of blood (3). Further, CTCs carry information about the primary tumor cells and could be considered an alternative means of tumor subtype classification (4).
In metastasis, the methylation status of the primary tumor is inherited by the metastatic tumor. Previous studies have shown that deoxyribonucleic acid (DNA) methylation exhibits cell and tissue specificity (5), and changes in DNA methylation play an important regulatory role in the development of cancer (6,7). Indeed, both genome-wide hypomethylation and hypermethylation modifications have the ability to alter the expression of neighboring genes and contribute to cancer phenotypes (7,8). DNA methylation has been extensively investigated in primary tumors (9); however, the events that shape the DNA methylome during metastatic dissemination are largely uncharacterized (10,11). Overall, knowledge of the methylation profile of CTC DNA may broaden our understanding of tumor cell origin and evolution.
In this study, we combined immunostaining fluorescence in situ hybridization (FISH)-based CTC identification, laser capture microdissection-based CTC capture, and single-cell resolution DNA methylation to explore CTC methylation signatures in the origin, classification, and evolution of these cells in NSCLC. Our study provides a genome-wide DNA methylation landscape of primary tumor tissues, CTCs, matched normal lung tissues, and white blood cells (WBCs) in 6 NSCLC patients. The results demonstrate that CTCs can be used as an effective blood-based method for the classification of LUAD and LUSC. The results also showed that both CTC primary tumor inheritance and CTC evolution affect metastasis and immune escape. We present the following article in accordance with the MDAR reporting checklist (available at https://tlcr.amegroups.com/article/view/10.21037/tlcr-22-50/rc).
Methods
Characteristics of patients and samples
The clinical characteristics and molecular profiles, including methylation data for a training cohort of 553 tumor samples and 60 matched adjacent normal tissue samples, and a validation cohort of 288 tumor and 14 matched normal samples, were obtained from The Cancer Genome Atlas (TCGA). A separate validation cohort of 37 tumor samples and 74 normal samples was obtained from Gene Expression Omnibus (GEO). Another separate validation cohort of 6 tumor and 6 matched normal samples was obtained from Zhongshan Hospital of Fudan University, Shanghai, China. Matched adjacent normal tissue samples and WBCs were collected at the same time as the tumor tissue from each patient, and subjected to a histological analysis to confirm that there was no evidence of cancer. The clinical characteristics of all the patients are summarized in Table S1. None of the 6 patients received any additional treatment apart from the surgery. Written informed consent was obtained from the patients, and ethical approval was obtained from the Zhongshan Hospital Research Ethics Committee (No. Y2019-187). All procedures performed in this study involving human participants were in accordance with the Declaration of Helsinki (as revised in 2013).
Experimental method details
Subtraction enrichment of CTCs and identification of aneuploid CTCs
The enrichment and identification of CTCs were performed in accordance with the instructions of the CTCseqTM kit (Majorbio). The samples were fixed on slides, and then counted and photographed. Each suspicious tumor cell coordinate was recorded to facilitate subsequent target cell identification. The identification principle of CTCs is that they are (I) negative for cluster of differentiation (CD)45 and (II) positive for chromosome 8 heteroploidy. The slides were stored at –20 °C.
Laser capture microdissection
The samples were loaded onto the stage of a Zeiss PALM MicroBeam (Zeiss) under a ×40 objective. After microdissection with a 355-nm laser beam, the target cells were collected on an AdhesiveCap 200 opaque cover (Zeiss). The 10-µL reaction volume contained 5 µL of M-Digestion Buffer (2×), 0.5 µL of proteinase K (EZ DNA Methylation-Direct™ Kit, Zymo), and 4.5 µL of nuclease-free water (Ambion). The reagents were mixed and placed on the AdhesiveCap 200 opaque cover; the tube was briefly microcentrifuged, and the reaction was incubated in a thermal cycler for 20 min at 50 °C, with a 4 °C hold. The samples were stored at –20 °C.
WGBS analysis
Tumor DNA extraction
Genomic DNA extraction from freshly frozen normal or cancer tissues or WBCs was performed with a QIAamp DNA Mini Kit (Qiagen) in accordance with the manufacturer’s recommendations. DNA was extracted from approximately 0.5 mg of tissue and stored at –20 °C; the samples were analyzed within 1 week of preparation.
Bisulfite conversion of genomic DNA and WGBS
Bisulfite conversion was performed using the EZ DNA Methylation-Lightning™ Kit (Zymo Research). Whole-genome bisulfite sequencing (WGBS) was performed using the KAPA Hyper Prep Kit (Roche) with several modifications, as previously described (12). The WGBS libraries of tissue and WBCs were sequenced with paired-end flow cell lanes in the HiSeq4000 system (Illumina) for 150 cycles.
Capture and sequencing
Capture was performed using the SeqCap Epi CpGiant Enrichment Kit (Roche) in accordance with the manufacturer’s instructions. Briefly, 4–6 bisulfite-treated libraries (200 ng/sample) were hybridized to the SeqCap Epi probe pool; the beads were captured, washed, amplified, quantified, and qualified as directed in the protocol. The captured pooled library (tissue 2N) was sequenced using the Illumina HiSeq X Ten system with a 150-bp paired-end model.
Bisulfite conversion and single-cell whole-genome bisulfite library preparation
The library was produced according to a previously published protocol (12). In brief, after cell lysis for 20 min, the CTC samples were subjected to bisulfite conversion using the EZ DNA Methylation-Direct™ Kit (Zymo) in accordance with the manufacturer’s instructions. The bisulfite-converted DNA was then synthesized using Klenow exo-(Enzymatics) with a truncated Illumina P5 adapter (5'-CTACACGACGCT CTT CC GATCTNNNNNN-3') followed by a random hexamer at the 3'end. This step was repeated 4 additional times for preamplification. The excess primers were removed using exonuclease I (New England Biolabs). Following purification, the 2nd strands were synthesized similarly but using a truncated P7 Illumina adapter (5'-AGACGTGTGCTCTTCCGATCTNNNNNN-3'). The final library was amplified using the KAPA HiFi HotStart ReadyMix (Kapabiosystems) with NEB primers (universal primer and index primer). The amplified libraries were purified twice with 0.9× AMPure XP beads (Beckman Coulter), and quantified using Qubit ds HS dye and a 2100 Bioanalyzer (Agilent Technologies). The final quality-ensured libraries were sequenced with a HiSeq4000 system (Illumina) for 150 cycles.
Quantification and statistical analysis
Processing methylation microarray data
The DNA methylation data were obtained from TCGA analysis of 485,000 sites generated using Infinium 450K Methylation Array, and the following GSE datasets: GSE85845, GSE83842, GSE66087, GSE63704, and GSE53051. The microarray data (level 3 in TCGA and processed matrix files in the GEO database) provided the methylation levels of the individual CpG sites. The methylation levels for the two cancer subtypes (i.e., LUAD and LUSC) and normal lung tissues were extracted. Six matched cohorts (cancer, normal, bulk WBCs, and CTCs per patient) were obtained by WGBS and analyzed as described.
Building the multiclass classifier
For each of the three subtypes of LUAD and LUSC cancer and corresponding normal tissue samples from TCGA, we randomly split the full TCGA 450K data set into training and validation sets at a 2:1 ratio. We first performed prescreening to remove excessive noise from the training data using the Dunn test. First, a CpG site methylation level was marked as “not available” (NA) if methylation measurements were not available for more than half of the CpG sites. Second, any samples that had missing methylation levels for more than 5 k CpG sites were marked as “NA”. Third, for each set of comparisons, 1 type of sample was compared against the other two types of samples. A list of markers with significant methylation differences ≥0.2 and P values <0.05 between LUAD and LUSC, and significant methylation differences ≥0.1 and P values <0.05 between LUAD and normal tissues or LUSC and normal tissues were retained for future analysis. The Benjamini-Hochberg procedure was used to control the false discovery rate at a significance level of 0.05. For the multinomial classification, we used logistic regression with the L2 regularization model (Ridge), and the tuning parameter was determined by the expected generalization error estimated from the 5-fold cross-validation. A multiclass prediction system was constructed to predict a cancer subtype or normal sample in the validation data using the selected features. A confusion matrix and receiver operating characteristic curves were also produced to evaluate sensitivity and specificity in addition to prediction accuracy.
All the data analyses were conducted by custom-made bash and R and Python scripts (R version =3.4.2, Python version =3.7.2) with the dunn.test (R) and sklearn (Python) packages.
WGBS processing
After tissue and CTC WGBS sequencing, an initial quality assessment of the data was performed using FastQC (https://www.bioinformatics.babraham.ac.uk/projects/fastqc). Adaptor sequences, low-quality ends, and 6 bp from both the 5' and 3' ends of reads were removed with Trim Galore (v0.4.2, http://www.bioinformatics.babraham.ac.uk/projects/trim_galore/; parameters: --clip_R1 6 --clip_R2 6 --three_prime_clip_R1 6 --three_prime_clip_R2 6). Trimmed reads were aligned to the hg19/GRCh37 human genome using Bismark with the alignment tool Bowtie2 (v2.2.9) (main parameter: --score-min L, 0, 0.2) (13,14). Finally, methylation cells were extracted after deduplication using Bismark. Only CpG sites with a depth of coverage ≥3× were considered for the methylation analysis.
Differential methylation analysis and enrichment analysis
Differentially methylated CpGs were assessed using a methylKit (R package) (15). Under the sliding linear model (SLIM) method, a P value <0.05 indicated differential methylenation (16). We selected differentially methylated CpG sites (DMCs) detected in 2 of the 6 patients for the future functional pathway analysis. Transcription factor binding sites (TFBSs) in DMCs were calculated using i-cisTarget (https://gbiomed.kuleuven.be/apps/lcb/i-cisTarget/) with a full motif analysis (17). The Kyoto Encyclopedia of Genes and Genomes (KEGG) analysis with a hypergeometric test implemented in the clusterProfiler R/bioconductor package was performed using ClueGO (Vocci and London, 1997). The annotation used for the CpGIslands and RefSeq genes was performed using the genomation toolkit in the R/bioconductor package.
KEGG pathway enrichment analysis based on genomic features
Individual CpGs were mapped to genes and their promoters using the RefSeq gene annotation from the University of California Santa Cruz genome browser (https://genome.ucsc.edu; date: 10/10/2020). Promoters were defined as the ±2 kb region around the transcription start site. Mapping to superenhancer regions was based on dbSUPER (http://asntech.org/dbsuper/), an integrated database of superenhancers that provides a list of genes associated with each region. Each genomic feature was interrogated for differential methylation in the same manner as that for genomic tiles. Similar genes corresponding to genomic features with a normal P value >0.05 and a methylation difference <10% were considered for the enrichment analysis between CTCs and matched tumor tissues. Differential genes corresponding to genomic features with a normal P value <0.05 and an absolute methylation difference >0.1 were considered for the enrichment analysis between CTCs and matched tumor tissues. The gene set enrichment analysis was performed using a hypergeometric test implemented in the clusterProfiler R/bioconductor package. Gene sets with an adjusted P value <0.05 were considered statistically significant.
Results
Identification and validation of cancer-specific differential methylation CpG sites
To explore NSCLC-specific DNA methylation markers, we first analyzed Infinium 450K methylation profiles obtained from TCGA (see Figure 1). We hypothesized that the most appropriate methylation differences (LUSC vs. LUAD vs. normal) would lead to the best performance in both clustering and classification. Thus, we started with 5 cutoff parameters of beta differences (greater than the cutoff) of 0.10, 0.15, 0.20, 0.25, and 0.30 among LUAD versus LUSC versus normal. The same cutoff parameters were used for LUAD and LUSC compared to normal controls. Four groups of features with each fixed parameter set were identified according to whether the difference in methylation met the cutoff for: (I) LUAD_LUSC_Normal specific; (II) LUAD_specific; (III) LUSC_specific; and (IV) Normal_specific. We assessed 4 combinations of specific probes [(I); (I) + (II) + (III), (I) + (II) + (III) + (IV), and (I) + (IV)] under each fixed parameter set, resulting in 5×5×4=100 mixed samples. Finally, we obtained the optimal parameters for LUAD_LUSC_diff ≥0.20, LUAD_Normal_diff ≥0.10, and LUSC_Normal_diff >0.10 (P<0.05). The clustering results are shown in Figure 2A.
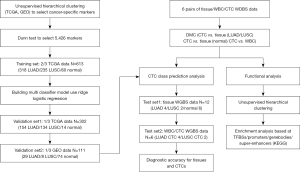
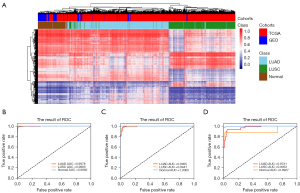
Under the optimal parameters, we detected 5,426 DMCs, including 5,426 LUAD-LUSC cancer-specific DMCs, 1,409 LUAD-normal specific DMCs, and 2,919 LUSC-normal specific DMCs (see https://cdn.amegroups.cn/static/public/tlcr-22-50-1.xlsx). Based on the differential methylation of the CpG sites, we were able to distinguish LUAD, LUSC, and normal tissues with diagnostic accuracies of 97.5%, 95.7%, and 100%, respectively (see Figure 2B and Table S2).
To assess the diagnostic accuracy of the methylation marker panel, we then applied the methylation panel to 1/3 of TCGA validation cohort 1 and GEO validation cohort 2 (see Figure 2C,2D). The diagnostic accuracy of the panel for LUAD, LUSC, and normal tissues was 98.1%, 94.8%, and 100%, respectively, in 1/3 of TCGA validation cohort 1 (see Figure 2C and Table S3), and 86.2%, 87.5%, and 98.6% in GEO validation cohort 2 (see Figure 2D and Table S4), respectively. These results demonstrate the robust nature of the methylation panel in identifying the presence of malignancy and its NSCLC subtype classification.
Cancer-specific methylation signature validation in tissues and CTCs
To validate the clinical value of the methylation panel, we next performed the WGBS analysis of the tumor tissue samples, matched normal lung tissue samples, CTCs, and WBCs from 6 NSCLC patients, including 4 LUAD and 2 LUSC cases (see Table S1). To obtain CTCs, blood samples were drawn from the 6 patients who had been newly diagnosed with lung cancer and processed using the immunostaining-FISH-based CTC technique (18-21), a CD45-based immunomagnetic system that combines leukocyte common antigen (CD45) immunostaining, and FISH using unprocessed blood samples that was specifically adapted to achieve a capture rate of >98% for single CTC and CTC clusters (18,22). Upon capture, the fixed CTCs were stained with antibodies against CD45 to identify contaminating leukocytes (see Figure S1). Upon staining verification, we identified CTCs in the 6 patients; the CTCs from each patient were individually captured and deposited in 10 µL of lysis buffer for WGBS (18,22). The WGBS sequencing data comprised 30 G raw data (10×) for the tissue samples and bulk WBCs, and 90 G raw data (30×) for the CTC samples. On average, we achieved 47.3% CpG coverage for CTCs, which is in line with a recent single-cell WGBS study (12). For each individual methylation profile, only CpG sites ≥3× coverage were used for the clustering and functional analyses (see Table S5).
To refine the tumor methylation signature in the matched CTCs, we identified similar methylation CpGs (SMCs) with methylation differences <10% and P values >0.05 between CTCs and the matched tumor tissue for each patient and included 450K CpG sites (see Table S6). Thus, we identified the CT450K SMCs present in both the CTCs and tumor methylation profiles with similar methylation levels that were also included in the 450K CpG sites. Next, we merged the CT450K SMCs from the 6 patients and used these CpG sites as features to cluster matched normal and lung cancer tissues, WBCs, and CTCs. After the refinement of the CT450K methylation signatures, all 6 pairs of CTCs and matched tumor tissues clustered together, and the Pearson correlation coefficient of CTCs and tumor tissues for each patient was >0.994 (see Figure 3A). These results suggest that CTCs inherit most of their methylation signatures from the primary tumors.
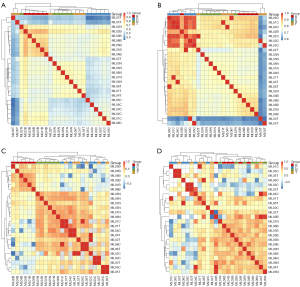
However, clustering of the CT450K methylation analysis did not group the CTCs and tumors together. The high Pearson correlation coefficient of CTCs and WBCs/normal led us to consider the existence of WBCs and normal tissue backgrounds in the CTC methylation pattern. To eliminate WBC backgrounds in the CTC methylation profile, we identified CTC/WBC/Tumor (CBT)450K DMCs for each patient (see Table S6). Next, we merged the CBT450K DMCs of the 6 patients together, and used these merged DMCs to cluster the lung cancer tissues and matched normal tissues/WBCs/CTCs. The Pearson correlation coefficient of the CTCs and WBCs for each patient decreased from a minimum of 0.831 (see Figure 3B) to a maximum of –0.696 (see Figure 3C). The results showed that almost all 6 pairs of CTCs and matched tumors clustered together, except for the clustering of 2T and 2C due to the ineffective bisulfite conversion of 2B (conversion ratio 91.46%). Figure 3C shows that the WBC background is an important component of the CTC methylation pattern.
To further eliminate the normal tissue background from the CTC methylation profile, we identified CTC/WBC/Tumor/Normal (CBTN)450K DMCs in each patient (see Table S6), and then merged the CBTN450K DMCs of the 6 patients together and used these merged DMCs to cluster the matched normal and lung cancer tissues, WBCs, and CTCs (see Figure 3D). The poor clustering performance suggested that a normal tissue background is not an effective component of the CTC methylation pattern.
Based on the above methylation profiles, our NSCLC tissue cohort showed that the methylation panel for all LUAD, LUSC, and normal tissues had a diagnostic accuracy of 100% (see Table S7); after removing the matched WBC background, the diagnostic accuracy of the methylation panel for LUAD and LUSC was also 100% based on the CTC cohort (see Table S8) (C&B diff >0.1, P<0.05).
Inheritance of CTCs
To explore the characteristics of CTCs, we specifically investigated methylation profile distribution according to functional genomic features between CTCs and matched tumor tissues (see Figure 4). We observed that the number of CpG sites in each CTC/Tumor (CT) similar group was far larger than that in the matched CT difference group (see Figure 4A), which implies that CTCs have a large proportion of methylation signatures inherited from the primary tumor. In addition, 5-methylcytosine (5-mC) was most common in TFBSs and intronic and intergenic regions, accounting for 42.8%, 47.3%, and 46.0% of all CpG sites in tumors, and 47.4%, 47.6%, and 42.8% of all CpG sites in CTCs, respectively (see Figure 4A). 5-mC was also commonly found in enhancers, superenhancers, promoters, and CpG shores, accounting for 5.6%, 24.8%, 5.5%, and 6.6% of all CpG sites in tumors, and 6.1%, 28.6%, 9.1%, and 8.0% of all CpG sites in CTCs, respectively (see Figure 4A).
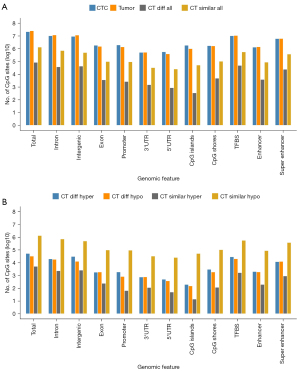
For regulatory elements, the loss of DNA methylation at TFBSs can designate active transcription factor networks or networks primed for activation at later stages [e.g., during processes such as the derivation of induced pluripotent stem cells from differentiated cells (23) or cancer progression (9)]. We then analyzed SMCs at TFBSs using i-Cistarget (17), and used the clusterProfile R package to analyze the KEGG pathways of global CTC hypomethylated TFBSs coexisting in CTCs and matched tumor tissues with methylation differences <10% and P values >0.05 (see Figure 5A). Our DNA methylation analysis revealed the mitogen-activated protein kinase signaling pathway and pathways regulating the pluripotency of stem cells, epithelial growth factor receptor (EGFR) tyrosine kinase inhibitor resistance, and the cell cycle. These pathways coexisted in both CTCs and matched tumor tissues, suggesting that CTC methylation originates from primary tumor tissues and is inherited as the cells move from the primary tumor tissues to the peripheral blood (see Figure 5A). We also found that binding sites for stemness-associated transcription factors are specifically hypomethylated in SMCs in both CTCs and matched tumor tissues, including binding sites for NANOG and SOX2, which in previous reports were associated with CTC clusters compared to single CTCs (24).
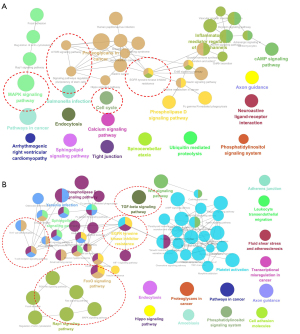
To explore other subtle changes in DNA methylation occurring specifically within promoters, gene bodies, and superenhancer regions, we carried out a hypergeometric-based gene set enrichment analysis of genomic features. Consistently, this analysis revealed hypomethylation and cell cycle progression (see Figure S2), as previously observed for cancer specimens with stem-like and proliferative features.
Evolution of CTCs
The CTCs showed many DMCs differed to those of the primary tumor (see Figure 4B). To identify whether the characteristic-related transcription factor networks were also transcriptionally active in CTCs compared to matched tumors, we performed a single-cell resolution methylation sequencing analysis of CTCs and matched tumors isolated from the 6 NSCLC patients (see Figure 5). We used the cluster Profile R package to analyze the KEGG pathways of global hypomethylated TFBSs in CTCs rather than matched tumor tissues with an absolute methylation difference >0.1 and a P value <0.05 (see Figure 5B). The KEGG analysis of TFBS DMCs in patient CTCs compared to matched tumors revealed the enrichment of genes related to the T cell receptor signaling pathway, Th17 cell differentiation, transforming growth factor (TGF)-beta signaling pathway, EGFR tyrosine kinase inhibitor resistance, and canonical pathways (RAS/MAPK/PI3K/AKT) (see Figure 5B).
In relation to the other subtle changes in DNA methylation occurring specifically in promoters, gene bodies, and superenhancer regions, the KEGG analysis revealed the enrichment of gene groups related to the TGF-beta signaling pathway, Th17 cell differentiation, T cell receptor signaling pathway, and EGFR tyrosine kinase inhibitor resistance (see Figure S3).
These results show that in the processes of dissemination from the primary tumor tissue to the peripheral blood, CTCs gradually develop their own unique methylation signatures with some unique characteristics that differ to those of the primary tumor.
Discussion
Our study shows that CTC methylation signatures can be used as an alternative non-invasive approach to biopsy for the pathological classification of NSCLC patients. We found that these methylation signatures can identify LUAD and LUSC with extremely high accuracy. The histologic definition of NSCLC is of huge importance to drive molecular testing and optimize treatment selection, which is a non-invasive method for histologic diagnosis. Our results raise the possibility that the detection of CTC methylation in peripheral blood may be expanded to aid in the diagnosis of a much larger number of tumor types. In addition, we uncovered 2 CTC methylation patterns of inheritance and evolution during the CTC migration process. These features of CTCs provide new insights into the mechanism of NSCLC metastasis.
In practice, the amount of genomic DNA in CTCs per patient is typically limited to a range of 10s of picograms. Moreover, the amplification of damaged DNA beginning with a fixed CTC cell is even more difficult than the amplification of integrated genomic DNA beginning with a live cell. In our study, we collected all the CTCs from each patient into 1 tube. We then amplified and achieved an average of 47.3% CpG coverage for CTCs, which is similar to the 50% CpG site coverage in a single cell reported by a recent study (12).
Our study suggests that CTCs share several properties common to immune escape and in mesenchymal-shifted cells compared to matched tumors. For example, the T-cell receptor signaling pathway and Th17 cell differentiation contributes to tumor immune escape (25-33). The TGF-beta pathway promotes epithelial-mesenchymal transition (EMT) in tumor cells, which plays an important role in mediating tumor invasion and metastasis (34,35). EGFR tyrosine kinase inhibitor resistance suggests a form of acquired drug resistance, which is associated with the tumor cell EMT phase (36-38). The canonical RAS/MAPK/PI3K/AKT signaling pathway is involved in EMT progression (39). Previous reports have demonstrated that the enhancement of mesenchymal-like features epigenetically reprograms epithelial cancer cells to adapt well to new microenvironments, and thus may contribute to distant metastasis (40). Several reports have focused on the relationship between EMT and immune escape (39,41-44), especially in NSCLC. However, previous studies have only focused on a few genes or proteins in CTCs associated with immune escape, such as the upregulation of CD47 expression as a potential escape mechanism in colorectal cancer based on quantitative polymerase chain reaction (45) or the downregulation of ULBP1 protein expression as a potential CTC evasion mechanism from natural killer cells (46). Our study uncovered a CTC immune escape mechanism through CTC methylation signatures on a genome-wide scale, and we propose that the EMT status of CTCs and T-cell receptor signaling ultimately leads to tumor immune escape and invasion.
The methylation profiling of circulating tumor DNA has been investigated in cancer diagnostics and in the assessment of therapeutic outcomes (47-52), but to date, few methylation profiles of CTCs have been studied and derived a DNA methylation signature in CTCS of patients with lung cancer. We used CTC methylation profiles to identify LUAD and LUSC with extremely high accuracy in 6 NSCLC patients. In our study, CTCs, as 1 of the 3 liquid biopsy biomarker types, showed strong potential in terms of methylation origin and classification. Compared to circulating tumor DNA and exosomes, CTCs carry a complete genome, which provides an incomparable advantage. Our study demonstrated that CTC traceability only requires deducing the matched WBCs. Conversely, circulating tumor DNA traceability needs a large training set and complex algorithm due to its rarity in the blood.
As an auxiliary diagnosis tool for benign and malignant lesions, CTC techniques should be strengthened. However, the capture of CTC remains a huge challenge for the widely available in the cancer diagnosis and other technologies, such as microfluidic technology, may be used to count and capture CTCs with both high sensitivity and specificity and low damage to the cells. Further, more analytical methods and models should be explored to improve coverage or change the analysis units from methylated cytosines to methylated regions (24,53).
Conclusions
In summary, our study provides insights into the potential of CTCs to replace invasive biopsy for the pathological classification of NSCLC patients. Further, we also found that CTC primary tumor inheritance and CTC evolution affect metastasis and immune escape.
Acknowledgments
The authors appreciate the academic support from the AME Lung Cancer Collaborative Group.
Funding: This work was supported by the National Natural Science Foundation of China (81972168), and the National Key Research and Development Program of China (2016YFA0502202, HW).
Footnote
Reporting Checklist: The authors have completed the MDAR reporting checklist. Available at https://tlcr.amegroups.com/article/view/10.21037/tlcr-22-50/rc
Data Sharing Statement: Available at https://tlcr.amegroups.com/article/view/10.21037/tlcr-22-50/dss
Conflicts of Interest: All authors have completed the ICMJE uniform disclosure form (available at https://tlcr.amegroups.com/article/view/10.21037/tlcr-22-50/coif). CYC, JL, and YL are from Shanghai Zhiyi Biomedical Technology Company, WF is from Jilin Province JinKangAn Pharmaceutical Company. The other authors have no conflicts of interest to declare.
Ethical Statement: The authors are accountable for all aspects of the work in ensuring that questions related to the accuracy or integrity of any part of the work are appropriately investigated and resolved. Written informed consent was obtained from the patients, and ethical approval was obtained from the Zhongshan Hospital Research Ethics Committee (No. Y2019-187). All procedures performed in this study involving human participants were in accordance with the Declaration of Helsinki (as revised in 2013).
Open Access Statement: This is an Open Access article distributed in accordance with the Creative Commons Attribution-NonCommercial-NoDerivs 4.0 International License (CC BY-NC-ND 4.0), which permits the non-commercial replication and distribution of the article with the strict proviso that no changes or edits are made and the original work is properly cited (including links to both the formal publication through the relevant DOI and the license). See: https://creativecommons.org/licenses/by-nc-nd/4.0/.
References
- Sung H, Ferlay J, Siegel RL, et al. Global Cancer Statistics 2020: GLOBOCAN Estimates of Incidence and Mortality Worldwide for 36 Cancers in 185 Countries. CA Cancer J Clin 2021;71:209-49. [Crossref] [PubMed]
- Reck M, Rabe KF. Precision Diagnosis and Treatment for Advanced Non-Small-Cell Lung Cancer. N Engl J Med 2017;377:849-61. [Crossref] [PubMed]
- Zhang Z, Xiao Y, Zhao J, et al. Relationship between circulating tumour cell count and prognosis following chemotherapy in patients with advanced non-small-cell lung cancer. Respirology 2016;21:519-25. [Crossref] [PubMed]
- Matthew EM, Zhou L, Yang Z, et al. A multiplexed marker-based algorithm for diagnosis of carcinoma of unknown primary using circulating tumor cells. Oncotarget 2016;7:3662-76. [Crossref] [PubMed]
- Vidal E, Sayols S, Moran S, et al. A DNA methylation map of human cancer at single base-pair resolution. Oncogene 2017;36:5648-57. [Crossref] [PubMed]
- Ehrlich M. DNA methylation in cancer: too much, but also too little. Oncogene 2002;21:5400-13. [Crossref] [PubMed]
- Ehrlich M. DNA hypomethylation in cancer cells. Epigenomics 2009;1:239-59. [Crossref] [PubMed]
- Farlik M, Halbritter F, Müller F, et al. DNA Methylation Dynamics of Human Hematopoietic Stem Cell Differentiation. Cell Stem Cell 2016;19:808-22. [Crossref] [PubMed]
- Feinberg AP, Vogelstein B. Hypomethylation distinguishes genes of some human cancers from their normal counterparts. Nature 1983;301:89-92. [Crossref] [PubMed]
- Fernandez AF, Assenov Y, Martin-Subero JI, et al. A DNA methylation fingerprint of 1628 human samples. Genome Res 2012;22:407-19. [Crossref] [PubMed]
- Moran S, Martínez-Cardús A, Sayols S, et al. Epigenetic profiling to classify cancer of unknown primary: a multicentre, retrospective analysis. Lancet Oncol 2016;17:1386-95. [Crossref] [PubMed]
- Clark SJ, Smallwood SA, Lee HJ, et al. Genome-wide base-resolution mapping of DNA methylation in single cells using single-cell bisulfite sequencing (scBS-seq). Nat Protoc 2017;12:534-47. [Crossref] [PubMed]
- Krueger F, Andrews SR. Bismark: a flexible aligner and methylation caller for Bisulfite-Seq applications. Bioinformatics 2011;27:1571-2. [Crossref] [PubMed]
- Langmead B, Salzberg SL. Fast gapped-read alignment with Bowtie 2. Nat Methods 2012;9:357-9. [Crossref] [PubMed]
- Akalin A, Kormaksson M, Li S, et al. methylKit: a comprehensive R package for the analysis of genome-wide DNA methylation profiles. Genome Biol 2012;13:R87. [Crossref] [PubMed]
- Wang HQ, Tuominen LK, Tsai CJ. SLIM: a sliding linear model for estimating the proportion of true null hypotheses in datasets with dependence structures. Bioinformatics 2011;27:225-31. [Crossref] [PubMed]
- Herrmann C, Van de Sande B, Potier D, et al. i-cisTarget: an integrative genomics method for the prediction of regulatory features and cis-regulatory modules. Nucleic Acids Res 2012;40:e114. [Crossref] [PubMed]
- Wan JF, Li XQ, Zhang J, et al. Aneuploidy of chromosome 8 and mutation of circulating tumor cells predict pathologic complete response in the treatment of locally advanced rectal cancer. Oncol Lett 2018;16:1863-8. [Crossref] [PubMed]
- Lin PP, Gires O, Wang DD, et al. Comprehensive in situ co-detection of aneuploid circulating endothelial and tumor cells. Sci Rep 2017;7:9789. [Crossref] [PubMed]
- Lin PP. Erratum to: Integrated EpCAM-independent subtraction enrichment and iFISH strategies to detect and classify disseminated and circulating tumors cells. Clin Transl Med 2016;5:6. [Crossref] [PubMed]
- Lin PP. Integrated EpCAM-independent subtraction enrichment and iFISH strategies to detect and classify disseminated and circulating tumors cells. Clin Transl Med 2015;4:38. [Crossref] [PubMed]
- Chen C, Li J, Wan J, et al. A low cost and input tailing method of quality control on multiple annealing, and looping-based amplification cycles-based whole-genome amplification products. J Clin Lab Anal 2019;33:e22697. [Crossref] [PubMed]
- Lee DS, Shin JY, Tonge PD, et al. An epigenomic roadmap to induced pluripotency reveals DNA methylation as a reprogramming modulator. Nat Commun 2014;5:5619. [Crossref] [PubMed]
- Gkountela S, Castro-Giner F, Szczerba BM, et al. Circulating Tumor Cell Clustering Shapes DNA Methylation to Enable Metastasis Seeding. Cell 2019;176:98-112.e14. [Crossref] [PubMed]
- Han Y, Ye A, Bi L, et al. Th17 cells and interleukin-17 increase with poor prognosis in patients with acute myeloid leukemia. Cancer Sci 2014;105:933-42. [Crossref] [PubMed]
- Kim JM, Chen DS. Immune escape to PD-L1/PD-1 blockade: seven steps to success (or failure). Ann Oncol 2016;27:1492-504. [Crossref] [PubMed]
- Grywalska E, Pasiarski M, Góźdź S, et al. Immune-checkpoint inhibitors for combating T-cell dysfunction in cancer. Onco Targets Ther 2018;11:6505-24. [Crossref] [PubMed]
- He X, Xu C. Immune checkpoint signaling and cancer immunotherapy. Cell Res 2020;30:660-9. [Crossref] [PubMed]
- Price DA, West SM, Betts MR, et al. T cell receptor recognition motifs govern immune escape patterns in acute SIV infection. Immunity 2004;21:793-803. [Crossref] [PubMed]
- Qin A, Coffey DG, Warren EH, et al. Mechanisms of immune evasion and current status of checkpoint inhibitors in non-small cell lung cancer. Cancer Med 2016;5:2567-78. [Crossref] [PubMed]
- Reuben A, Zhang J, Chiou SH, et al. Comprehensive T cell repertoire characterization of non-small cell lung cancer. Nat Commun 2020;11:603. [Crossref] [PubMed]
- Spranger S. Mechanisms of tumor escape in the context of the T-cell-inflamed and the non-T-cell-inflamed tumor microenvironment. Int Immunol 2016;28:383-91. [Crossref] [PubMed]
- Tian Y, Zhai X, Han A, et al. Potential immune escape mechanisms underlying the distinct clinical outcome of immune checkpoint blockades in small cell lung cancer. J Hematol Oncol 2019;12:67. [Crossref] [PubMed]
- Colak S, Ten Dijke P. Targeting TGF-beta signaling in cancer. Trends Cancer 2017;3:56-71. [Crossref] [PubMed]
- Drabsch Y, Ten Dijke P. TGF-beta signalling and its role in cancer progression and metastasis. Cancer Metastasis Rev 2012;31:553-68. [Crossref] [PubMed]
- Gainor JF, Dardaei L, Yoda S, et al. Molecular Mechanisms of Resistance to First- and Second-Generation ALK Inhibitors in ALK-Rearranged Lung Cancer. Cancer Discov 2016;6:1118-33. [Crossref] [PubMed]
- Liu X, Li J, Cadilha BL, et al. Epithelial-type systemic breast carcinoma cells with a restricted mesenchymal transition are a major source of metastasis. Sci Adv 2019;5:eaav4275. [Crossref] [PubMed]
- Fischer KR, Durrans A, Lee S, et al. Epithelial-to-mesenchymal transition is not required for lung metastasis but contributes to chemoresistance. Nature 2015;527:472-6. [Crossref] [PubMed]
- Jiang Y, Zhan H. Communication between EMT and PD-L1 signaling: New insights into tumor immune evasion. Cancer Lett 2020;468:72-81. [Crossref] [PubMed]
- Mitra A, Mishra L, Li S. EMT, CTCs and CSCs in tumor relapse and drug-resistance. Oncotarget 2015;6:10697-711. [Crossref] [PubMed]
- Mak MP, Tong P, Diao L, et al. A Patient-Derived, Pan-Cancer EMT Signature Identifies Global Molecular Alterations and Immune Target Enrichment Following Epithelial-to-Mesenchymal Transition. Clin Cancer Res 2016;22:609-20. [Crossref] [PubMed]
- Lou Y, Diao L, Cuentas ER, et al. Epithelial-Mesenchymal Transition Is Associated with a Distinct Tumor Microenvironment Including Elevation of Inflammatory Signals and Multiple Immune Checkpoints in Lung Adenocarcinoma. Clin Cancer Res 2016;22:3630-42. [Crossref] [PubMed]
- Kim S, Koh J, Kim MY, et al. PD-L1 expression is associated with epithelial-to-mesenchymal transition in adenocarcinoma of the lung. Hum Pathol 2016;58:7-14. [Crossref] [PubMed]
- Tiwari N, Gheldof A, Tatari M, et al. EMT as the ultimate survival mechanism of cancer cells. Semin Cancer Biol 2012;22:194-207. [Crossref] [PubMed]
- Steinert G, Schölch S, Niemietz T, et al. Immune escape and survival mechanisms in circulating tumor cells of colorectal cancer. Cancer Res 2014;74:1694-704. [Crossref] [PubMed]
- Hu B, Tian X, Li Y, et al. Epithelial-mesenchymal transition may be involved in the immune evasion of circulating gastric tumor cells via downregulation of ULBP1. Cancer Med 2020;9:2686-97. [Crossref] [PubMed]
- Taylor WC. Comment on 'Sensitive and specific multi-cancer detection and localization using methylation signatures in cell-free DNA' by M. C. Liu et al. Ann Oncol 2020;31:1266-7. [Crossref] [PubMed]
- Liu L, Toung JM, Jassowicz AF, et al. Targeted methylation sequencing of plasma cell-free DNA for cancer detection and classification. Ann Oncol 2018;29:1445-53. [Crossref] [PubMed]
- Jiang P, Sun K, Tong YK, et al. Preferred end coordinates and somatic variants as signatures of circulating tumor DNA associated with hepatocellular carcinoma. Proc Natl Acad Sci U S A 2018;115:E10925-33. [Crossref] [PubMed]
- Xu RH, Wei W, Krawczyk M, et al. Circulating tumour DNA methylation markers for diagnosis and prognosis of hepatocellular carcinoma. Nat Mater 2017;16:1155-61. [Crossref] [PubMed]
- Guo S, Diep D, Plongthongkum N, et al. Identification of methylation haplotype blocks aids in deconvolution of heterogeneous tissue samples and tumor tissue-of-origin mapping from plasma DNA. Nat Genet 2017;49:635-42. [Crossref] [PubMed]
- Lee EJ, Luo J, Wilson JM, et al. Analyzing the cancer methylome through targeted bisulfite sequencing. Cancer Lett 2013;340:171-8. [Crossref] [PubMed]
- Hao X, Luo H, Krawczyk M, et al. DNA methylation markers for diagnosis and prognosis of common cancers. Proc Natl Acad Sci U S A 2017;114:7414-9. [Crossref] [PubMed]