Role of radiomics in predicting lung cancer spread through air spaces in a heterogeneous dataset
Introduction
Spread through air spaces (STAS) has been recently reported as a negative prognostic factor in patients with lung cancer (1,2). According to the 2015 World Health Organization classification (3), it is defined as the presence of micropapillary clusters, solid nests or single tumor cells spreading within air spaces beyond the edge of the main tumor. Several studies showed the impact of STAS on overall survival and risk of recurrence, particularly in patients with lung adenocarcinoma (ADC) undergoing limited resection (4,5). In fact, the presence of tumor cells beyond the main tumor edge could result in a non-radical surgical resection. Recently, sublobar resection of STAS-positive tumors has been associated with a higher rate of both distant and loco-regional recurrence when compared to lobectomy (6-8). Therefore, preoperative prediction of STAS may provide crucial information to properly plan the surgical treatment (9).
Previous studies suggested that STAS could be predicted by analysis of computed tomography (CT) imaging (10-12); it was associated with solid nodules, central low attenuation, ill-defined opacity, air bronchogram and high consolidation/tumor ratio. However, qualitative CT features are inevitably biased by personal interpretation potentially leading to a misclassification.
Radiomics is a process allowing the conversion of medical images characteristics into quantitative information. Using dedicated software, a large number of features are extracted from medical images, providing much more information than the “human eyes” and improving the accuracy of imaging decoding. Radiomics-based models to predict STAS in preoperative CT imaging have been recently proposed with encouraging results (13-15). However, in these studies both patients and CT images were highly selected, making the results strictly dependent on the quality and homogeneity of the data and, thus, hardly applicable in daily clinical practice.
The aim of this study is to test a radiomics-based prediction model of STAS using an unselected cohort of patients and images that are non-homogeneous for manufacture, model of scanner, pixel size, acquisition protocol, reconstruction and endovenous contrast phase. This unselected setting is more adherent to daily clinical practice and could help the future translation of radiomics. We present the following article in accordance with the TRIPOD reporting checklist (available at https://tlcr.amegroups.com/article/view/10.21037/tlcr-21-895/rc).
Methods
Patients’ selection and study design
This study was conducted in accordance with the Declaration of Helsinki (as revised in 2013). The study was approved by institutional ethics committee of Policlinico Umberto I, Sapienza University of Rome (No. Rif: 6453) and registered on clinicaltrials.gov (identifier: NCT04893200). Informed consent was taken from all individual participants. Our institutional database was searched for patients with diagnosis of lung ADC surgically treated at our Department from January 2018 to December 2019. Clinical and radiological data were collected and patients were selected according to the following inclusion criteria: age older than 18 years, pathologically confirmed lung ADC, STAS status available from the pathological report; accessible preoperative CT images. Patients with incomplete surgical resection (R1), chest wall or mediastinal infiltration and patients receiving induction radio-chemotherapy were excluded from this study. Finally, a cohort of 99 patients (61 M, 38 F) was selected to train the radiomics based predictor. For the training and internal validation phase the predictor was aware of the STAS status for each patient.
According to the Checklist for Artificial Intelligence in Medical Imaging (16) and the Radiomics Quality Score (17), the validation of the predictive model was planned on a prospective setting. To this purpose, a further series of 50 consecutive patients (24 M, 26 F) undergoing lung cancer surgery at our institution were prospectively enrolled as external validation cohort. Inclusion criteria were as follows: age older than 18 years; suspected or cito-histologically proven lung ADC undergoing lung cancer surgery; available preoperative CT images. Chest wall or mediastinal infiltration, induction radio or chemotherapy and incomplete surgical resection were considered exclusion criteria. For the external validation phase the predictor was not aware of the STAS status and results were compared after histological assessment.
The prediction model had no rule in the clinical work up and patients were treated according to standard protocols, regardless the radiomics outcome. A flowchart reporting the methodology of the study is shown in Figure 1.
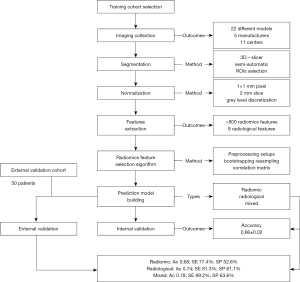
Histological evaluation
Multiple tissue samples were obtained from both the tumor and the surrounding lung parenchyma after formalin fixation. Several histological sections were then acquired from each paraffin block and stained with hematoxylin and eosin for light microscopy evaluation. A necessary requirement to reliably evaluate STAS on surgical samples was the presence of a rim of normal lung (at least 1 cm-thick) surrounding the entire tumor circumference. The morphological features of STAS were described according to the 2020 World Health Organization classification. Moreover, STAS was also classified based on the degree of circumferential extension. All slides were separately evaluated by two experienced pathologists (AP and GDA). Any discrepancy was discussed until consensus was reached.
Imaging acquisition and segmentation
CT scan images were obtained from the picture archiving and communication system (PACS) in digital imaging and communications in medicine (DICOM) format. To build a dataset as adherent as possible to daily clinical practice, images were taken from the last preoperative CT scan acquisition, regardless of model and manufacture of scanner, acquisition protocol, reconstruction, intravenous contrast phase and pixel size. If possible, images with intravenous contrast in venous phase were taken at the thinnest slice available. The sharpest (B70–B60) reconstruction kernel was chosen when possible.
Qualitative features were collected by two expert radiologists (MD and PR) including: tumor maximum diameter, density [solid, mixed, ground glass opacity (GGO)], consolidation-tumor ratio (C-T ratio), presence of air bronchogram, irregular margins, nodule excavation, pleural involvement and lymphadenopathy larger than 1 cm. The open-source platform 3D-slicer v4.8.1 (www.slicer.org) was used for tumor segmentation as already reported in previous studies (13-14). The tumor area was outlined independently by two different physicians with 5-year experience in lung cancer imaging (MB and MD) using a semi-automatic segmentation editor and marked as region of interest (ROI). ROIs were then reviewed and discrepancies resolved by additional corrections.
Imaging preprocessing and radiomics features extraction
Considering the heterogeneity of the dataset, a preprocessing algorithm was used to normalize the data in terms of voxel size (1×1×2 mm3) and gray level discretization (25 bin width) (18). ‘PyRadiomics’, an open-source package for standardizing the extraction of radiomics data (https://pyradiomics.readthedocs.io/en/latest/), was used to automatically extract more than 800 radiomics features from non-filtered imaging and images filtered for edge enhancement (wavelet transform). Detailed description and the computing algorithms of the radiomics features are available at https://pyradiomics.readthedocs.io/en/latest/features.html.
Feature selection
Features were selected according to the following process in order to select only features as independent as possible to preprocessing steps, correlation and sampling bias.
To create a model as stable as possible to preprocessing, we extracted and tested the features using 4 different preprocessing setups: (I) original images; (II) images normalized only for voxel size; (III) images normalized only for gray level; (IV) images normalized for both voxel size and gray level. Finally, we considered for further analysis only features showing a significant association with STAS (P value <0.05) in all four setups. Similarly, we discarded features extracted from discrete wavelet transforms (19).
To evaluate statistical significance stability in respect to subsampling, a bootstrap resampling algorithm was performed removing randomly 20% of the dataset. After discarding the outlier values (more than 3 sigma from the mean), each radiomics feature was compared using the Student’s t-test between STAS positive and STAS negative groups in the remaining dataset accordingly. Features showing a P value >0.05 after subsampling were removed from the analysis. The remaining features were sorted according to the P value of their respective univariate test. Moreover, when two features showed a high correlation (Pearson’s correlation >0.7), the one with the highest P value was excluded. At the end of this process, only the 5 features with the lowest P value were considered for the further analysis. This bootstrap resampling algorithm was repeated 100 times, randomly removing 20% of the dataset each run. Finally, features were ranked according to the number of times that have passed the test. The 5 features with the highest rank were considered for the prediction model building.
Model building and internal validation
Four machine-learning (ML) methods (Naıve Bayes, k-Nearest Neighbors, Random Forest, Logistic Regression) were tested to build the predictive model by incorporating the aforementioned radiomics features. A 3-fold cross internal validation was performed considering 70% of the dataset for training and 30% for test. Finally, the best ML method was selected according to the accuracy and the associated confusion matrix.
Statistical analysis
Statistical analysis was performed using Python (version 3.8) and R platform (R version 3.5.1). Continuous data were reported as median and interquartile range (IQR); categorical variables as number and percentage. Continuous and categorical clinical variables were analyzed using the Mann Whitney U test and Fisher exact test respectively. Features were compared using Student’s t-test and results of the multivariate analysis reported as mean ±2 standard deviations. A P value of <0.05 was considered statistically significant. To validate our predictor, the radiomics-based model was compared with the performance of a traditional radiological predictor and a mixed model. Accuracy, Sensitivity (SE) and Specificity (SP) were calculated after external validation according to the confusion matrix.
Results
Clinical data
Demographics and radiological characteristics of both training and external validation cohorts included in the study are shown in Table 1. The training cohort consists of 99 patients (61 M, 38 F) with a median age of 68.0 years (IQR, 61.5–74.0). Tumor stage, according to the 8th edition of the American Joint Commission on Cancer Tumor-Nodal-Metastasis (TNM) staging classification, was represented as follows: IA in 42 (42.4%) patients, IB in 17 (17.2%) patients, IIB in 19 (19.2%) patients, IIIA in 15 (15.2%) patients and IIIB in 6 patients (6.1%). STAS was present in 65 (65.7%) patients. No significant difference in terms of gender, smoking habits, histological subtype, tumor location, stage, N status and extent of surgical resection were found between STAS positive and STAS negative patients. However, STAS positive patients in training cohort were significantly associated with older age (P=0.04), larger tumor (P=0.03), solid nodule (P<0.01) and presence of lymphadenopathy larger than 1 cm (P=0.02) (Table 2).
Table 1
Characteristics | Training cohort | External validation cohort | P value |
---|---|---|---|
Patients (n) | 99 | 50 | |
Age (years) | 68.0 (61.5–74.0) | 69.0 (61.0–73.8) | 0.48 |
Gender, n (%) | 0.12 | ||
Male | 61 (61.6) | 24 (48.0) | |
Female | 38 (38.4) | 26 (52.0) | |
Smoker status, n (%) | 0.67 | ||
Smoker history | 77 (77.8) | 41 (82.0) | |
Non-smoker | 22 (22.2) | 9 (18.0) | |
Histological subtype, n (%) | 0.08 | ||
Acinar | 53 (53.5) | 23 (46.0) | |
Solid | 24 (24.3) | 10 (20.0) | |
Lepidic | 11 (11.1) | 5 (10.0) | |
Papillary | 5 (5.1) | 5 (10.0) | |
Others | 6 (6.1) | 7 (14.0) | |
T status, n (%) | 0.66 | ||
T1 | 48 (48.5) | 24 (48.0) | |
T2 | 29 (29.3) | 11 (22.0) | |
T3 | 15 (15.1) | 11 (22.0) | |
T4 | 7 (7.1) | 4 (8.0) | |
N status, n (%) | 0.29 | ||
N0 | 74 (74.7) | 43 (86.0) | |
N1 | 9 (9.1) | 3 (6.0) | |
N2 | 16 (16.2) | 4 (8.0) | |
STAS status, n (%) | 1.00 | ||
No | 34 (34.3) | 17 (34.0) | |
Yes | 65 (65.7) | 33 (66.0) | |
Tumor site, n (%) | 0.46 | ||
RUL | 33 (33.3) | 12 (24.0) | |
RML | 8 (8.1) | 2 (4.0) | |
RLL | 18 (18.2) | 12(24.0) | |
LUL | 25 (25.3) | 12 (24.0) | |
LLL | 15 (15.2) | 12 (24.0) | |
Surgical resection, n (%) | 0.21 | ||
Bilobectomy/pneumonectomy | 5 (5.1) | 3 (6.0) | |
Lobectomy | 66 (66.7) | 26 (52.0) | |
Sublobar resection | 28 (28.3) | 21 (42.0) | |
Radiological characteristics | |||
Density, n (%) | 0.06 | ||
Pure GGOs | 4 (4.0) | 7 (14.0) | |
Mixed | 18 (18.2) | 11 (22.0) | |
Solid | 77 (77.8) | 32 (64.0) | |
Tumor size (mm) | 25.0 (17.0–42.0) | 23.0 (16.0–31.8) | 0.20 |
Nodule excavation, n (%) | 19 (19.2) | 7 (14.0) | 0.50 |
Pleural invasion, n (%) | 33 (33.3) | 17 (34.0) | 0.97 |
Air bronchogram, n (%) | 32 (32.3) | 10 (20.0) | 0.13 |
Irregular margins | 77 (77.8) | 33 (66.0) | 0.17 |
Lymphadenopathy >1 cm, n (%) | 29 (29.3) | 15 (30.0) | 1.00 |
Continuous variables are reported as median (interquartile range); categorical variables as number (percentage). T, tumor; N, nodes; STAS, spread through air spaces; RUL, right upper lobe; RML, right middle lobe; RLL, right lower lobe; LUL, left upper lobe; LLL, left lower lobe; GGO, ground glass opacity.
Table 2
Training cohort | STAS positive | STAS negative | P value |
---|---|---|---|
Patients (n) | 65 | 34 | |
Age (years) | 69.0 (62.0–75.0) | 67.0 (57.8–72.5) | 0.04 |
Gender (male), n (%) | 38 (58.5) | 23 (67.6) | 0.39 |
Smoking habitus, n (%) | 50 (76.9) | 27 (79.4) | 1.00 |
Histological subtype, n (%) | 0.09 | ||
Acinar | 33 (50.8) | 20 (58.8) | |
Solid | 19 (29.2) | 6 (17.6) | |
Lepidic | 5 (7.7) | 6 (17.6) | |
Others | 8 (12.3) | 2 (5.9) | |
Tumor stage, n (%) | 0.26 | ||
I | 35 (53.8) | 24 (48.0) | |
II | 15 (23.1) | 4 (22.0) | |
III | 15 (23.1) | 6 (22.0) | |
N status, n (%) | 0.42 | ||
N0 | 46 (70.8) | 28 (82.4) | |
N1 | 6 (9.2) | 3 (8.8) | |
N2 | 13 (20.0) | 3 (8.8) | |
Radiological characteristics | |||
Density, n (%) | <0.01 | ||
Pure GGOs | 1 (1.5) | 3 (8.8) | |
Mixed | 7 (10.8) | 11 (32.4) | |
Solid | 57 (87.7) | 20 (58.8) | |
Tumor size (mm) | 27.0 (20.0–45.0) | 18.5 (16.0–32.8) | 0.03 |
Nodule excavation, n (%) | 10 (15.4) | 9 (26.5) | 0.19 |
Pleural invasion, n (%) | 24 (36.9) | 9 (26.5) | 0.37 |
Air bronchogram, n (%) | 25 (38.5) | 7 (20.6) | 0.11 |
Irregular margins, n (%) | 53 (81.5) | 26 (76.5) | 0.60 |
Lymphadenopathy >1 cm, n (%) | 24 (36.9) | 5 (14.7) | 0.02 |
Continuous variables are reported as median (interquartile range). Categorical variables as number (percentage). STAS, spread through air spaces; N, nodes; GGO, ground glass opacity.
The external validation cohort was prospectively enrolled and consists of 50 consecutive patients (24 M, 26 F) with a median age of 69.0 (IQR, 61.0–73.8). Tumor stage was represented as follows: 22 (44.0%) patients IA, 9 (18.0%) IB, 2 (4.0%) IIA, 8 (16.0%) IIB, 7 (14.0%) IIIA, 2 (4.0%) IIIB, 1 (2%) IV stage. No significant difference was found in the external validation group between STAS positive and STAS negative patients in terms of age, gender, smoking habits, tumor location, nodule diameter, stage, N status and extent of surgical resection. STAS was significantly associated with the presence of a solid nodule at preoperative CT images (P<0.01).
Internal and external validation
More than 40 radiomic features were found to be associated with the presence of STAS with a P value ≤0.05 after univariate analysis. According to the described selection process (i.e., subsection 2.5), the 5 most ranked features were considered for model building (Table 3). Three different predictors were created using respectively: (I) only radiomics features; (II) qualitative radiological features; (III) combined radiological and radiomics features. In the latter, radiological and radiomics features together entered the pipeline that automatically selected the best ones without knowing the origin of any single feature. Logistic regression was the ML method of choice in all cases.
Table 3
Type | Machine learning algorithm | Feature name | Accuracy on internal validation | Accuracy on external validation |
---|---|---|---|---|
Radiomics | Logistic regression | Autocorrelation | 0.66±0.02 | 0.68 |
Cluster prominence | ||||
Dependence entropy | ||||
Gray level non-uniformity | ||||
Long run high gray level emphasis | ||||
Radiological | Logistic regression | Maximum diameter | 0.66±0.02 | 0.74 |
Solid density | ||||
Bronchogram | ||||
Lymphadenopathy >1 cm | ||||
Pure GGO density | ||||
Mixed | Logistic regression | Autocorrelation | 0.66±0.02 | 0.78 |
Cluster prominence | ||||
Gray level non-uniformity | ||||
Solid density | ||||
Pure GGO density |
Accuracy in internal validation is reported as mean ±2 standard deviation. GGO, ground glass opacity.
The radiomics predictor showed an accuracy of 0.66±0.02 after internal validation and 0.68 after external validation (SE 77.4%; SP 52.6%). Similar results were shown by the radiological (0.66±0.02) and the mixed predictor (0.66±0.02) after internal validation with an accuracy at the external validation of 0.74 (SE 81.3%; SP 61.1%) and 0.78 (SE 89.2%; SP 63.6%) respectively (Figure 2, Table 4).
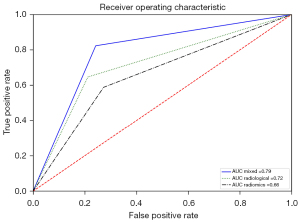
Table 4
Predictor | Accuracy | Sensitivity | Specificity | AUC |
---|---|---|---|---|
Clinical* | 0.64 | 0.73 | 0.47 | 0.59 |
Radiomics | 0.68 | 0.77 | 0.53 | 0.66 |
Radiological | 0.74 | 0.81 | 0.61 | 0.72 |
Mixed | 0.78 | 0.89 | 0.64 | 0.79 |
*, clinical variables include gender, age and smoking history. AUC, area under the curve.
As additional exploratory investigation, the models were tested in the subgroup of 31 stage I patients belonging to the validation cohort showing an accuracy of 0.65 (radiomics model), 0.71 (radiological model) and 0.74 (mixed model).
Discussion
STAS has gained relevance in recent years as a negative prognostic factor in patients with lung cancer, particularly ADC; it was considered an independent poor predictor of progression free survival, overall survival and lung cancer specific survival (1,2). It is present up to 64.2% of patients with lung cancer (2). Several studies examined the impact of STAS in patients undergoing sublobar resections. These studies showed an increased rate of both distant and locoregional metastasis in STAS positive patients undergoing limited resections (6-8). Therefore, the prediction of STAS in patients undergoing lung cancer surgery may provide crucial information to the surgeon to properly plan surgery and to avoid oncologically ineffective sublobar resections. Recently, efforts have been made to predict the presence of STAS preoperatively using intraoperative frozen sections (20), airway secretion cytology (21) or preoperative CT imaging (10-12). However, none of these methods has shown satisfying results and their application is far from clinical use to date.
In this context, radiomics could allow a deeper imaging analysis and potentially improve the accuracy of prediction models. It is an emergent analytical tool codifying into quantifiable features the qualitative characteristics of radiological images, reducing errors due to personal interpretation of data. The analysis can be performed using open-sourced platforms that allow the extraction of a large number of features from common medical imaging. This provides more information than traditional interpretation, allowing a deeper analysis of the data and improving the accuracy of imaging decoding. For these characteristics, the use of radiomics in medical imaging has rapidly gained popularity worldwide, including in lung cancer research.
In 2020, two parallel studies firstly proposed a radiomics-based model to predict STAS using preoperative CT imaging. Chen et al. (13) retrospectively studied a group of 233 patients with stage I lung ADC. The authors built a predictor model based on 5 radiomics features using the Naıve Bayes ML method. The predictor showed encouraging results with an area under the curve (AUC) of 0.63 (0.55–0.71) after internal validation and an accuracy of 0.69 in the external validation group.
Jiang et al. (14) performed a retrospective study on 462 patients surgically treated for lung ADC. They created a mixed random forest model including the age of patients and 12 radiomics features, showing an AUC of 0.75 (0.59–0.88) after internal validation. No external validation was performed. The authors concluded that the radiomics-based model has the potential to become a promising imaging biomarker to preoperatively predict STAS, facilitating surgeons’ decision making.
After that, a further study by Zhuo et al. (15) proposed a radiomics nomogram consisting of seven selected radiomics parameters and clinical features, showing good prediction in both training set (AUC, 0.98, 0.97–1.00) and test set (AUC, 0.99; 0.97–1.00). However, clinical features showed a high prediction power themselves (AUC, 0.98; 0.96–1.00), hiding the effective role of radiomics features.
Those studies presented some limitations that make the application of the predictor model difficult in daily clinical practice. First, all of them represent a single-institution study with highly selective criteria of CT imaging. In fact, images were taken from the same institution, with a controlled acquisition protocol and from the same scanners [“Brilliance 16” for Jiang et al. (14); “Somatom Definition AS” and “Brilliance 40” for Chen et al. (13)]. This helped to reduce the bias related to different acquisition protocols and scanners but makes the results strictly dependent on homogeneity of data. Second, Chen and Zhuo studied only stage I ADC, while Jiang did not assess the impact of tumor stage in relation to STAS. Third, only one study (13) reported an external validation, which has been performed on a retrospective cohort however.
We aimed to build a dataset of images adherent to daily clinical practice, with CT imaging obtained from different scanners in different centers and, thus, different acquisition protocols. Finally, images were taken from 22 different models of scanners from 5 distinct manufacturers, 11 different institutions and 19 different reconstruction kernel. Slice thickness ranged from 0.63 to 5 mm, pixel size from 0.55 mm to 1 mm and intravenous contrast was taken in a venous phase in 94, arterial phase in 3 and basal in 3. Similarly, we consecutively included patients of any tumor stage to assess the trend of radiomics features regardless tumor characteristics.
The first issue with such heterogeneous data was the imaging normalization. This preprocessing is recommended to obtain more reproducible CT features since some of them were dependent on voxel size and gray-level (18). We decided to normalize all CT images to the largest pixel size in our dataset (1 mm) and the median slice thickness (2 mm). This was, in our opinion, the best compromise between losing data (e.g., choosing a thickest slice) and creating information (e.g., normalizing to the smallest pixel size and thinnest slice). Similarly, gray level distribution was normalized to 25 bin width, a good balance between complexity of the imaging and stability of the features’ extraction. Imaging was checked out after normalization and no clinical differences were appreciable.
Secondly, we had to ensure that the features entering in the final model were stable over preprocessing setups and different acquisition protocols. To this purpose, we performed a univariate analysis in 4 different preprocessing setups and only features showing statistical significance stability in all configurations were considered for further analysis. Moreover, we discarded features extracted after discrete wavelet transform. In fact, this procedure may reduce the explainability of our final ML model generating images difficult to comprehend by clinicians (Figure 3).
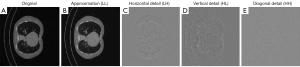
Third, in order to minimize the cohort selection bias, features were chosen after a bootstrapping subsampling algorithm and only the 5 features with the highest P value rank (e.g., more independent to subsampling) were considered for the predictor building. Interestingly, all 5 features selected for the final model are second-order texture features. Probably, first-order features and shape features are less informative than texture features in this analysis. Considering that a second-order feature (Gray Level Non-Uniformity) shows a high correlation with the tumor volume, all the shape features were automatically excluded by the classifier.
Furthermore, we aimed to validate our prediction model on a prospective cohort that provides the highest level of evidence with regard to the clinical validity and usefulness of the radiomics biomarker (17). The best accuracy in the prospective validation cohort was reached by the mixed model (78%) with an AUC of 0.79.
Although reliability, SE and SP are not suitable for a prompt translation, the use of radiomics-based models to predict STAS in patients with lung cancer may provide crucial information to properly plan surgical treatment in the future. More studies assessing the role of radiomics in STAS prediction are needed, especially in stage I lung cancer.
The classifiers should consider the heterogeneity of CT data in daily clinical practice and provide results that are reliable regardless of scanner model and manufacture and institutions’ protocols. This is the first study testing a model independent from radiological variance in image protocol acquisition, reconstruction and pre-processing. Moreover, this is the first radiomics study validating its prediction model of STAS on a prospective external cohort.
This study presents several limitations. First of all, the number of patients is relatively small in both the training cohort and the external validation cohort. Further multi-centric studies are needed to assess the reliability of our model. Second, we do not investigate the variability of features concerning different segmentation protocols nor the stability of the chosen features regarding segmentation discrepancies. Moreover, the effect of normalization process over the single features has not been investigated. Further studies are needed to state the stability of each feature in relation to different acquisition protocols and normalization settings.
Conclusions
Radiomics-based prediction models of STAS might be useful in the future to properly plan the surgical treatment and to avoid oncologically ineffective sublobar resections. This study supports a possible application of radiomics models using data with high variance in acquisition protocols, reconstruction and preprocessing. These findings represent a new chance for the use of radiomics-based models in the prediction of STAS despite the high variety of preoperative radiological data and could help its translation to clinical practice.
Acknowledgments
The abstract was presented at the 29th European Conference on General Thoracic Surgery of the European Society of Thoracic Surgeons 2021 – Bromptom Session (20 June 2021). The authors thank CONICYT (Fondecyt post-doctorado No. 3180167) and Rachele Giordano for independent validation of results.
Funding: None.
Footnote
Reporting Checklist: The authors have completed the TRIPOD reporting checklist. Available at https://tlcr.amegroups.com/article/view/10.21037/tlcr-21-895/rc
Data Sharing Statement: Available at https://tlcr.amegroups.com/article/view/10.21037/tlcr-21-895/dss
Peer Review File: Available at https://tlcr.amegroups.com/article/view/10.21037/tlcr-21-895/prf
Conflicts of Interest: All authors have completed the ICMJE uniform disclosure form (available at https://tlcr.amegroups.com/article/view/10.21037/tlcr-21-895/coif). The authors have no conflicts of interest to declare.
Ethical Statement: The authors are accountable for all aspects of the work in ensuring that questions related to the accuracy or integrity of any part of the work are appropriately investigated and resolved. This study was conducted in accordance with the Declaration of Helsinki (as revised in 2013). The study was approved by the institutional ethics committee of Policlinico Umberto I, Sapienza University of Rome (No. Rif: 6453) and registered on clinicaltrials.gov (identifier: NCT04893200). Informed consent was taken from all individual participants.
Open Access Statement: This is an Open Access article distributed in accordance with the Creative Commons Attribution-NonCommercial-NoDerivs 4.0 International License (CC BY-NC-ND 4.0), which permits the non-commercial replication and distribution of the article with the strict proviso that no changes or edits are made and the original work is properly cited (including links to both the formal publication through the relevant DOI and the license). See: https://creativecommons.org/licenses/by-nc-nd/4.0/.
References
- Toyokawa G, Yamada Y, Tagawa T, et al. Significance of Spread Through Air Spaces in Resected Pathological Stage I Lung Adenocarcinoma. Ann Thorac Surg 2018;105:1655-63. [Crossref] [PubMed]
- Mantovani S, Pernazza A, Bassi M, et al. Prognostic impact of spread through air spaces in lung adenocarcinoma. Interact Cardiovasc Thorac Surg 2021; Epub ahead of print. [Crossref] [PubMed]
- Travis WD, Brambilla E, Nicholson AG, et al. The 2015 World Health Organization Classification of Lung Tumors: Impact of Genetic, Clinical and Radiologic Advances Since the 2004 Classification. J Thorac Oncol 2015;10:1243-60. [Crossref] [PubMed]
- Kadota K, Nitadori JI, Sima CS, et al. Tumor Spread through Air Spaces is an Important Pattern of Invasion and Impacts the Frequency and Location of Recurrences after Limited Resection for Small Stage I Lung Adenocarcinomas. J Thorac Oncol 2015;10:806-14. [Crossref] [PubMed]
- Yi E, Lee JH, Jung Y, et al. Clinical implication of tumour spread through air spaces in pathological stage I lung adenocarcinoma treated with lobectomy. Interact Cardiovasc Thorac Surg 2021;32:64-72. [Crossref] [PubMed]
- Kadota K, Kushida Y, Kagawa S, et al. Limited Resection Is Associated With a Higher Risk of Locoregional Recurrence than Lobectomy in Stage I Lung Adenocarcinoma With Tumor Spread Through Air Spaces. Am J Surg Pathol 2019;43:1033-41. [Crossref] [PubMed]
- Ren Y, Xie H, Dai C, et al. Prognostic Impact of Tumor Spread Through Air Spaces in Sublobar Resection for 1A Lung Adenocarcinoma Patients. Ann Surg Oncol 2019;26:1901-8. [Crossref] [PubMed]
- Eguchi T, Kameda K, Lu S, et al. Lobectomy Is Associated with Better Outcomes than Sublobar Resection in Spread through Air Spaces (STAS)-Positive T1 Lung Adenocarcinoma: A Propensity Score-Matched Analysis. J Thorac Oncol 2019;14:87-98. [Crossref] [PubMed]
- Suh JW, Jeong YH, Cho A, et al. Stepwise flowchart for decision making on sublobar resection through the estimation of spread through air space in early stage lung cancer1. Lung Cancer 2020;142:28-33. [Crossref] [PubMed]
- Toyokawa G, Yamada Y, Tagawa T, et al. Computed tomography features of resected lung adenocarcinomas with spread through air spaces. J Thorac Cardiovasc Surg 2018;156:1670-1676.e4. [Crossref] [PubMed]
- de Margerie-Mellon C, Onken A, Heidinger BH, et al. CT Manifestations of Tumor Spread Through Airspaces in Pulmonary Adenocarcinomas Presenting as Subsolid Nodules. J Thorac Imaging 2018;33:402-8. [Crossref] [PubMed]
- Kim SK, Kim TJ, Chung MJ, et al. Lung Adenocarcinoma: CT Features Associated with Spread through Air Spaces. Radiology 2018;289:831-40. [Crossref] [PubMed]
- Chen D, She Y, Wang T, et al. Radiomics-based prediction for tumour spread through air spaces in stage I lung adenocarcinoma using machine learning. Eur J Cardiothorac Surg 2020;58:51-8. [Crossref] [PubMed]
- Jiang C, Luo Y, Yuan J, et al. CT-based radiomics and machine learning to predict spread through air space in lung adenocarcinoma. Eur Radiol 2020;30:4050-7. [Crossref] [PubMed]
- Zhuo Y, Feng M, Yang S, et al. Radiomics nomograms of tumors and peritumoral regions for the preoperative prediction of spread through air spaces in lung adenocarcinoma. Transl Oncol 2020;13:100820. [Crossref] [PubMed]
- Mongan J, Moy L, Kahn CE Jr. Checklist for Artificial Intelligence in Medical Imaging (CLAIM): A Guide for Authors and Reviewers. Radiol Artif Intell 2020;2:e200029. [Crossref] [PubMed]
- Lambin P, Leijenaar RTH, Deist TM, et al. Radiomics: the bridge between medical imaging and personalized medicine. Nat Rev Clin Oncol 2017;14:749-62. [Crossref] [PubMed]
- Shafiq-Ul-Hassan M, Latifi K, Zhang G, et al. Voxel size and gray level normalization of CT radiomic features in lung cancer. Sci Rep 2018;8:10545. [Crossref] [PubMed]
- van Timmeren JE, Leijenaar RTH, van Elmpt W, et al. Test-Retest Data for Radiomics Feature Stability Analysis: Generalizable or Study-Specific? Tomography 2016;2:361-5. [Crossref] [PubMed]
- Villalba JA, Shih AR, Sayo TMS, et al. Accuracy and Reproducibility of Intraoperative Assessment on Tumor Spread Through Air Spaces in Stage 1 Lung Adenocarcinomas. J Thorac Oncol 2021;16:619-29. [Crossref] [PubMed]
- Isaka T, Yokose T, Miyagi Y, et al. Detection of tumor spread through airspaces by airway secretion cytology from resected lung cancer specimens. Pathol Int 2017;67:487-94. [Crossref] [PubMed]