Plasma extracellular vesicle long RNA profiling identifies a diagnostic signature for stage I lung adenocarcinoma
Introduction
Lung adenocarcinoma (LUAD) is the most common histologic type of lung carcinoma, with an average 5-year survival rate of 15% (1,2). After surgical resection, early LUAD, such as adenocarcinomas in situ (AIS) and minimally invasive adenocarcinomas (MIA), and stage I LUAD, have an 80–100% survival rate (3-6). Currently, low-dose computed tomography (LDCT) is recommended as the only method of clinical lung carcinoma detection for cases with a high risk of lung carcinoma (7). However, due to the significant cost and high false discovery rate of computed tomography (CT) screening, early detection remains unsatisfactory (8). Recently, liquid biopsy has shown promising results for the early diagnosis of LUAD and is superior to conventional biopsy due to its noninvasiveness (9). Discovering effective circulating diagnostic biomarkers for early LUAD is imperative.
Extracellular vesicles (EVs), including exosomes and microvesicles, comprise protein, lipids, and RNA, and stably exist in various body fluids [e.g., blood and urine (10,11)]. Thus, the contents of EVs may have potential as diagnostic biomarkers via liquid biopsies for human carcinomas. Melo et al. reported that the EV protein marker, Glypican 1 (GPC1), could be a promising diagnosis-related marker of early pancreatic carcinoma, with high specificity and sensitivity (12). EV microRNAs (miRNAs) have been well characterized and studied (13). According to Huang et al., exosomal miR-1290 and miR-375 are prognosis-related markers in castration-resistant prostate carcinoma (14). In recent years, long RNA species [e.g., messenger RNA (mRNA), circular RNA (circRNA), and long noncoding RNA (lncRNA)] have also been identified in human blood EVs and exhibit function-related and clinically related significance (15). Yu et al. built an EV long RNA-based diagnostic signature for detecting pancreatic ductal adenocarcinoma (PDAC), which showed a high accuracy (16). Del Re et al. reported that PD-L1 mRNA in plasma EVs is related to the response towards anti-PD-1 antibodies in non-small cell lung carcinoma (NSCLC) and melanoma (17).
For lung carcinoma, studies have confirmed that EV miRNAs and proteins could act as potential diagnostic biomarkers (18-22). However, there are few studies on the profile of EV long RNA (exLR) in LUAD and whether exLR could serve as a biomarker in early LUAD cases. The present study performed exLR sequencing on plasma samples collected from 110 participants (64 cancer samples and 46 controls) to determine the diagnosis-related significance and molecular characteristics of exLR profiles in early LUAD. We also evaluate the performance and stability of the exLR-based diagnostic signature in a cohort comprising 30 cancer samples and 16 controls by RT-qPCR. We present the following article in accordance with the STARD reporting checklist (available at https://tlcr.amegroups.com/article/view/10.21037/tlcr-21-729/rc).
Methods
Patient cohort
In total, 110 participants [including stage I LUAD cases, patients with benign pulmonary nodules (BPN), and health controls (HCs)] were enrolled in this study. Non-cancerous controls (NCs) were defined as a combination of BPN and HCs. The training (n=48) and internal validation (n=32) cohorts were composed of patients from the National Cancer Center/Cancer Hospital, and the external validation cohort (n=30) was composed of patients from the Peking Union Medical College Hospital and the China-Japan Friendship Hospital. The patients’ clinical features, including age, gender, smoking history, family history of lung cancer, pathological type, and nodule size, are shown in Table 1.
Table 1
Characteristics | Training cohort (n=48) | Internal validation cohort (n=32) | External validation cohort (n=30) | |||||
---|---|---|---|---|---|---|---|---|
NC (n=24) | LUAD (n=24) | NC (n=12) | LUAD (n=20) | NC (n=10) | LUAD (n=20) | |||
Age (years), mean (SD) | 53.00 (7.49) | 51.96 (7.97) | 51.25 (9.14) | 55.20 (7.41) | 57.00 (11.17) | 53.25 (10.92) | ||
Gender, n (%) | ||||||||
Female | 9 (37.50) | 15 (62.50) | 3 (25.00) | 10 (50.00) | 6 (60.00) | 16 (80.00) | ||
Male | 15 (62.50) | 9 (37.50) | 9 (75.00) | 10 (50.00) | 4 (40.00) | 4 (20.00) | ||
Smoking history, n (%) | ||||||||
Yes | 8 (33.33) | 2 (8.33) | 6 (50.00) | 10 (50.00) | 4 (40.00) | 6 (30.00) | ||
No | 16 (66.67) | 22 (91.67) | 6 (50.00) | 10 (50.00) | 6 (60.00) | 14 (70.00) | ||
Family history, n (%) | ||||||||
Yes | 4 (16.67) | 3 (12.50) | 3 (25.00) | 6 (30.00) | 2 (20.00) | 4 (20.00) | ||
No | 20 (83.33) | 21 (87.50) | 9 (75.00) | 14 (70.00) | 8 (80.00) | 16 (80.00) | ||
Nodule size (cm), mean (SD) | 1.35 (0.66) | 1.22 (0.48) | 1.23 (0.32) | 0.93 (0.44) | 0.9 (0.26) | 1.11 (0.52) | ||
Pathology, n (%) | ||||||||
HC | 13 (54.17) | 0 | 6 (50.00) | 0 | 5 (50.00) | 0 | ||
BPN | 11(45.83) | 0 | 6 (50.00) | 0 | 5 (50.00) | 0 | ||
AIS | 0 | 8 (33.33) | 0 | 7 (35.00) | 0 | 7 (35.00) | ||
MIA | 0 | 5 (20.83) | 0 | 6 (30.00) | 0 | 6 (30.00) | ||
IAC | 0 | 11 (45.83) | 0 | 7 (35.00) | 0 | 7 (35.00) |
NC, non-cancerous control; LUAD, lung adenocarcinoma; SD, standard deviation; HC, health control; BPN, benign pulmonary nodule; AIS, adenocarcinoma in situ; MIA, minimal invasive adenocarcinoma; IAC, invasive carcinoma.
The study was conducted in accordance with the Declaration of Helsinki (as revised in 2013). The study was approved by the clinical research ethics committee of National Cancer Center/Cancer Hospital, Chinese Academy of Medical Sciences (Approval number: 20/370-2155). All patients provided written informed consent.
Plasma specimen collection
Based on the regular venipuncture process, 2 mL of peripheral blood specimens was collected from each participant using ethylenediaminetetraacetic acid (EDTA) tubes. After centrifugation for 10 minutes at 2,000 ×g and 4 ℃, the plasma was aspirated and stored at −80 ℃ for subsequent use.
Isolation of EVs
The ultracentrifugation method was optimized according to the process outlined in a previous study (23). After melting at 37 ℃, the plasma specimens underwent centrifugation for 15 minutes at 3,000 ×g to remove cell debris. Subsequently, the supernatant was diluted with phosphate-buffered saline (PBS) at 8-fold volume, and centrifuged for 15 minutes at 13,000 ×g to remove significant-scale particles. The supernatant then underwent ultracentrifugation for 4 hours in a P50A72-986 rotor (CP100NX; Hitachi, Tokyo, Japan) at 150,000 ×g and at 4 ℃ for exosome pelleting. The pellet was then resuspended in PBS and recentrifuged for 2 hours at 150,000 ×g and 4 ℃. Subsequently, the exosome pellets were washed with PBS and resuspended in 200 µL of PBS. For a full description of this process, please see the EV-TRACK protocol(EV200082) (24).
Nanoparticle tracking analysis (NTA)
Vesicle suspensions at concentrations ranging from 1×107 to 1×109 particles/mL were examined using the 405 nm laser-equipped ZetaView PMX 110 (Particle Metrix, Meerbusch, Germany) to determine the sizes and quantities of the separated particles. A 60-second video was taken at a frame ratio of 30 frames/s. Furthermore, particle movement was analyzed using NTA software (ZetaView 8.02.28, Particle Metrix).
Transmission electron microscopy
A 10 µL exosome solution was placed onto a copper mesh and incubated for 1 minute at ambient temperature. The exosome solution was then washed using sterile distilled water, and was contrasted for 1 minute using uranyl acetate solution. Subsequently, the specimen was dried for 2 minutes under an incandescent light. The copper mesh was observed and photographed with a transmission electron microscope (TEM; H-7650, Hitachi).
Western blotting assay
The exosome supernatant was denatured in a 5× sodium dodecyl sulfonate (SDS) buffer and then underwent western blotting assay (50 µg protein/lane; 10% SDS-polyacrylamide gel electrophoresis) with calnexin (10427-2-AP, Promega, Madison, WI, USA), TSG101 (sc-13611, Santa Cruz, CA, USA), Alix (sc-53540, Santa Cruz Biotechnology, Inc., Dallas, TX, USA), HSP90 (60318-I-Ig, Proteintech, Rosemont, IL, USA), CD9 (60232-I-Ig, Proteintech), and rabbit polyclonal antibody CD63 (sc-5275, Santa Cruz). The proteins were visualized using the Tanon4600 Automatic chemiluminescence image analyzing method (Tanon, Shanghai, China).
EV RNA isolation and RNA analyses
Total RNA was extracted and purified based on the plasma exosome using the miRNeasy Mini tool (cat. no. 217004, Qiagen, Hilden, Germany) according to the manufacturer’s instructions. RNA degradation and contamination, especially DNA contamination, was monitored on 1.5% agarose gels. RNA concentration and purity were evaluated using the RNA Nano 6000 Assaying Tool based on the Agilent Bioanalyzer 2100 Method (Agilent Technologies, Santa Clara, CA, USA).
RNA isolation and qRT-PCR
The total RNA from EVs was extracted using miRNeasy Serum/Plasma Advanced Kit (Qiagen, cat. No. 217004) according to the manufacturer’s protocol. The total RNA was then reverse transcribed to synthesize cDNA using PrimeScript™ RT reagent Kit (Perfect Real Time) (TAKARA, RR037A). The abundance of target gene expression was detected by TaqMan® probe using real-time qPCR. Two microliters of cDNA was used as the template for each PCR reaction. The sequence of primers and probes were shown as Table S1.
Library preparation and sequencing
With the Ovation SoLo RNA-Seq Library Preparation Kit (NuGEN, San Carlos, CA, USA), 5 ng of RNA per specimen was used as the input material for the sequencing libraries, and the index codes were added into the attribute sequences of the respective specimens according to the manufacturer’s instructions. Then, PCR outcomes were purified (AMPure XP method), and the library quality was assessed using the Agilent Bioanalyzer 2100 and qPCR. The index-coded specimens were clustered on acBot Cluster Generating Method using TruSeq PE Cluster Kitv3-cBot-HS (Illumina, San Diego, CA, USA) according to the manufacturer’s instructions. When the cluster was generated, the library prepared products were sequenced onto an Illumina Hiseq platform, and subsequently, the paired-end reads were produced.
RNA-sequencing analysis
The transcriptome was aligned to the reference genome with Hisat2 and was assembled using StringTie according to the reads mapped to the GRCh38 human genome. The transcripts under the assembly were annotated using known mRNA gff by employing the Cuffcompare program from the Cufflinks package.
This study employed transcripts unknown for screening putative lncRNAs. We considered transcripts over 200 nt in length and having over 2 exons to be lncRNA candidates. These were subsequently screened with CPC/CNCI/CPAT/Pfam, which is capable of distinguishing protein-coding genes in noncoding genes.
Statistical analyses
LncRNA and mRNA raw read counts were converted to FPKM values using Cuffdiff (v2.1.1). In order to improve the reliability of the analysis results, we used only RNA (mean FPKM ≥1 and in more than 1/4 sample FPKM >0) to perform downstream analysis. The ExLR FPKM was further standardized using the TMM method via R package “edgeR”. Differential expression analysis of the controls compared with LUAD samples was performed using the Mann Whitney U test, with a cutoff TMM ≥5, fold change ≥1.5, and P value ≤0.05. In the training cohort, 136 exLRs were differentially expressed in LUAD samples compared with the controls. Although the integration of data was carried out together, in fact, samples (especially plasma samples of tumor patients) are collected by different investigators in three hospitals at different time periods. So, Information about the three cohorts is relatively independent and not easy to leak.
We used LASSO regularization to narrow the number of candidate markers. LASSO regularization was performed using the “glmnet” package in R software, and the training cohort was employed to process LASSO regression in 1000 repetitions. We used 5-fold cross-validation and the Akaike information criterion to estimate the expected generalization error and then selected the optimal value of “1-se”” lambda parameter to construct an adaptive general linear model for marker selection. Following LASSO regularization analysis, the frequency statistics of the top 20 differential exLRs were selected to a construct diagnostic model by logistic regression using the “glm” function in R software. R package “pROC” was used to evaluate the performance of the model and visualize the result. We selected results with better comprehensive performance by comparing the area under the ROC curve (AUC), sensitivity, specificity, and negative predictive value (NPV). The same evaluation was also performed on the validation cohort. Finally, 8 exLR markers (NFKBIA, NDUFB10, SLC7A7, ARPC5, SEPTIN9, HMGN1, H4C2, and lnc-PLA2G1B-2:3) were selected.
All statistical tests were two-sided and performed using R 3.2.3. P<0.05 was considered statistically significant.
Results
Human blood exSR-seq and exLR-seq
The clinical information of the patients corresponding to each sample has been shown in Table 1. This study presented an optimized strategy for exLR-seq analysis involving 64 LUAD plasma samples, 22 BPN plasma samples, and 24 HCs plasma samples (Figure S1). TEM and NTA showed that EVs were in the rounding, cup-shaping, and double-membrane-bound vesiclelike configurations (Figure 1A), with a size range of 70–200 nm (Figure 1B). Western blot analysis demonstrated the expression of the EV markers (Alix, TSG101, and CD9) in the aforementioned EVs. Calnexin, a negative EV marker, was not detected (Figure 1C).
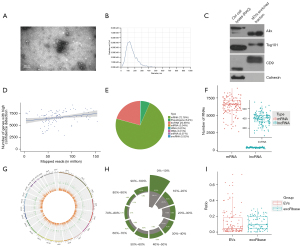
ExLR-seq generated a median read count of 35.3 million mapped reads for each sample. Although the number of reads in each sample was different, there was no significant change in the number of valid long RNAs identified with the increasing number of reads. Despite the broad range of mapped reads, nearly 9,871 annotated genes and 1,237 lncRNAs were consistently detected (Figure 1D). Protein-coding RNAs (mRNAs) constituted 72.78% of the total mapped reads, and lncRNAs accounted for 20.66% of the overall mapped reads. Other RNA types accounted for small fractions of the isolated sequences (6.2% for pseudogenes and 0.22% for rRNA alone; Figure 1E). In terms of the respective exLR-seq sample, this study performed median detection of 6,304 mRNAs and 399 lncRNAs (Figure 1F). In addition, no noticeable location preferences across chromosomes were identified between the 2 EV long RNA species (Figure 1G). The basic coverage of exLRs was also analyzed, and the results showed that the coverage of most mRNAs and lncRNAs in EVs was not 100% (Figure 1H).
During the pretreatment of plasma EVs, the interference of platelets and platelet-derived EVs might have been introduced, and hence, the expression level of mitochondrial genes in platelets would be significantly higher than that in other tissues and cells. Therefore, we used the mitochondrial gene expression ratio to evaluate the impact of platelets on our samples. This study compared the mitochondrial gene expression ratio in our data set with that of exoRbase (25), and found that the ratio in our samples was similar to that of exoRbase (Figure 1I). These results suggested that platelet effects were removed during sample processing in this study.
Comparison of exLRs between LUAD and NCs
RNA concentration (ng per mL plasma) of EVs enriched fractions isolated from HC, BPN and LUAD groups was analyzed. Total RNA isolated from EVs enriched fractions was analyzed and quantitated. No significant difference was identified in the RNA concentration of EVs enriched fractions (ng/mL plasma) between HC, BPN patients and LUAD cases (Figure 2A). The LUAD group had a significantly higher number of detected RNA species, including both mRNAs and lncRNAs, than did the HCs. However, in terms of the number of detected RNA species, the LUAD group was not significantly different from the BPN group (Figure 2B). According to t-distributed stochastic neighbor embedding (T-SNE) and principal component analysis (PCA) analyses, generally, in terms of the exLR profiles, there were differences between the LUAD cases and healthy individuals, as well as some BPN cases (Figure 2C,2D). A total of 117 exLRs were identified as being differentially expressed in LUAD compared to noncancerous control cases (Table S2). Among these, 3 lncRNAs and 80 mRNAs were found to be upregulated, and 23 lncRNAs and 11 mRNAs were found to be downregulated in LUAD cases compared to NCs. As per the unsupervised hierarchical clustering of the differentially expressed exLRs (DEexLRs), LUAD and NCs were clearly separated (Figure 2E). By Kyoto Encyclopedia of Genes and Genomes (KEGG) pathway analysis, enrichment of the mentioned DEexLRs could be observed in a few pathways that were involved in carcinoma, such as the estrogen signaling pathway, viral carcinogenesis, the cGMP-PKG signaling pathway, the cytosolic DNA-sensing signaling pathway, and the glucagon signaling pathway (Figure 2F). According to these findings, biomarkers in exLRs may be used to detect early LUAD.
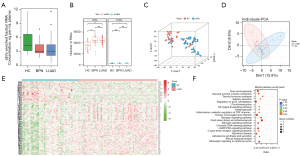
Establishment of the exLR d-signature for LUAD
The workflow applied for identifying the exLR d-signature for the diagnosis of LUAD is shown in Figure 3. The choice of the exLRs (n=136) that were differentially expressed in LUAD cases compared to the NCs was made from a training cohort of 24 NC cases (BPN + healthy) individuals and 24 LUAD cases. The selected exLR markers were analysed using the LASSO method to shrink the number of variables. Finally, 8 exLR markers (NFKBIA, NDUFB10, SLC7A7, ARPC5, SEPTIN9, HMGN1, H4C2, and lnc-PLA2G1B-2:3) were selected (Table 2).
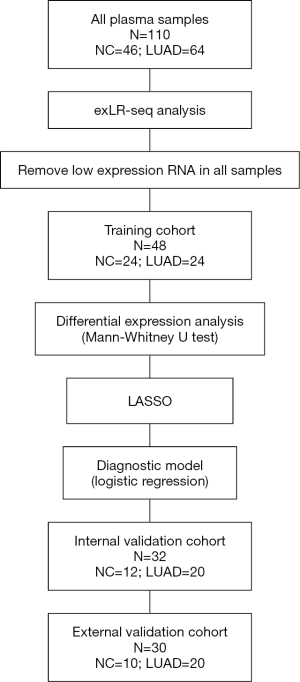
Table 2
Biomarker | P value | log2FC | FDR |
---|---|---|---|
NFKBIA | 0.0017 | 0.8666 | 0.4843 |
NDUFB10 | 0.0019 | 0.6927 | 0.4843 |
SLC7A7 | 0.0072 | 1.0254 | 0.5651 |
ARPC5 | 0.0001 | 1.2126 | 0.4338 |
SEPTIN9 | 0.0007 | 0.6908 | 0.4450 |
HMGN1 | 0.0004 | 2.0347 | 0.4421 |
H4C2 | 0.0020 | −0.7178 | 0.4843 |
lnc-PLA2G1B-2:3 | 0.0001 | −1.6102 | 0.4338 |
FDR, false discovery rate; LUAD, lung adenocarcinoma; FC, fold change.
Based on logistic regression, this study built an exLR-based diagnostic signature (exLR d-signature) for LUAD. Detailed parameters of the model and the cutoff value:
Z = (–15.439212538296 + 0.276596940044276*NFKBIA + 0.116677939851743*NDUFB10 + 0.287717146886391*SLC7A7 + 0.10254802626883*ARPC5 + 0.152102286464288*SEPTIN9 + 0.43111045527139*HMGN1 + -0.00489333268680212*H4C2 + –0.226760347812566*lnc.PLA2G1B-2:3).
Probability=e^Z/(1+e^Z). Cutoff value: 0.21. Probability is taken as the predicted value of the final model, if Probability > Cutoff, the sample is cancer patient.
In the training cohort, the exLR d-signature had the ability to distinguish LUAD from NCs, with a specificity of 91.7%, a sensitivity of 95.8%, and an AUC of 0.991 (95% CI: 0.973 to 1; Figure 4A,4B). Next, the exLR d-signature was used in the internal validation cohort of 12 NCs and 20 LUAD cases. LUAD was detected with a specificity of 83.3%, a sensitivity of 87.5%, and an AUC of 0.921 (95% CI: 0.832 to 1; Figure 4A,4B). The exLR d-signature was then used in the external validation cohort of 10 NCs and 20 LUAD cases: LUAD was detected with a specificity of 90%, a sensitivity of 75%, and an AUC of 0.9 (95% CI: 0.793 to 1; Figure 4A,4B).
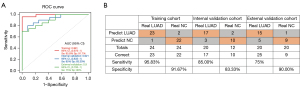
Unsupervised hierarchical clustering with the 8 exLRs effectively distinguished LUAD from the NCs with high specificity and sensitivity (Figure S2).
The exLR d-signature could detect early LUAD from NCs
The ability of a biomarker to detect LUAD at an early stage determines its true value for LUAD diagnosis. To assess the diagnostic significance of the exLR d-signature in the different stages of early LUAD, we separated the LUAD cases into AIS, MIA, and invasive adenocarcinoma (IAC) cases. We found that the d-signature had no correlation with tumor stage, indicating that the d-signature’s diagnostic performance was determined by the tumor burden in stage I LUAD (Figure 5A). The d-signature exhibited the ability to identify LUAD cases from the NCs, HCs and BPN cases, with AUCs of 0.948, 0.969, and 0.925, respectively, in the combined cohorts (Figure 5B). Further, the LUAD was found to exhibit a high median exLR d-signature score compared to that of the BPN (P<0.001) and HC (P<0.001) cases (Figure 5C). In the combined cohorts, the d-signature had the ability to distinguish AIS, MIA, and the remaining IAC cases from the HCs with AUCs of 0.934, 0.909, and 0.987, respectively (Figure 5D). According to these findings, the exLR d-signature could be applied for the early detection of LUAD with high accuracy.
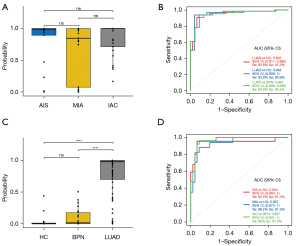
Validation of the 8 genes in the exLR d-signature by RT-qPCR
qPCR was used to evaluate the 8 exLRs using a cohort comprising 30 LUAD samples and 16 noncancerous controls. The expression levels of the 8 exLRs are shown in Figure 6. There were 6 exLRs (NFKBIA, NDUFB10, ARPC5, SEPTIN9, H4C2, and lnc-PLA2G1B-2:3) with upregulated expression in LUAD patients compared to NC controls (P<0.05). Moreover, in order to confirm the origin of these exLRs, we performed degradation assay using Proteinase K and RNase prior to RNA extraction. Coomassie blue staining verified that the proteins in sEVs enriched fractions were largely degraded by Proteinase K (Figure 7A). Agilent 2100 Bioanalyzer results suggested that sEVs enriched fraction derived RNA was slightly decreased (Figure 7B). We also verified that in sEVs enriched fraction NFKBIA, NDUFB10, SLC7A7, ARPC5, SEPTIN9, HMGN1, H4C2, and lnc-PLA2G1B-2:3 could not be degraded by the pretreatment of Proteinase K and RNase A (Figure 7C). Those results suggested that these 8 exLRs were all well protected by sEV membrane in sEVs enriched fractions.
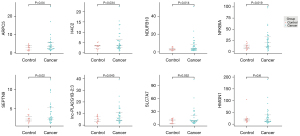
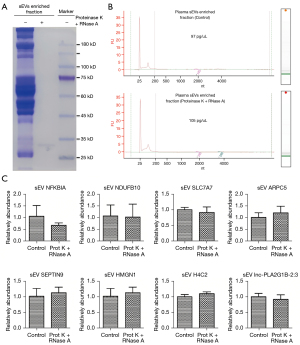
Biological process network of 8 genes in the exLR d-signature
We conducted a network analysis relative to the 8 RNAs in our signature of the GO: Biological Process signature by considering the union of 7 mRNAs and target genes of 1 lncRNA. The interaction network resulted very dense with 483 nodes and 596 edges. For a selective visualization of the most important pathways in the network, we highlighted the interactions of the following relevant pathways: epidermal growth factor receptor signaling pathway, positive regulation of intrinsic apoptotic signaling pathway, epithelial to mesenchymal transition, Notch signaling pathway, activation of MAPK activity and apoptotic signaling pathway (Figure 8). A complete list of the signature 8 RNAs, target genes and the pathways detected by the target network analysis are displayed in https://cdn.amegroups.cn/static/public/tlcr-21-729-1.xlsx.

Discussion
This work acquired the exLR-seq expression profiles from 110 human plasma EV samples, including 64 LUAD plasma samples, 22 BPN plasma samples, and 24 HCs plasma samples. To the best of our knowledge, this is the first published EV long RNA-sequencing expression profile for LUAD cases. Moreover, this work first compared the differences in exLRs levels between LUAD, BPN, and HCs, and then built an EV diagnostic signature for early LUAD.
Early diagnosis can help to reduce lung carcinoma mortality. According to recently conducted analyses, EVs represent an attractive source of diagnosis-related biomarkers for early stage lung carcinoma. Jin et al. created a panel with 4 EV miRNAs (let-7b-5p, let-7e-5p, miR-23a-3p, and miR-486-5p), which displayed a promising diagnosis-related performance in identifying stage I NSCLC, with a specificity of 92.31%, a sensitivity of 80.25%, and an AUC of 0.899 (19). Furthermore, Yao et al. developed an EV miRNA signature for stage I/II LUAD, with an AUC of 0.993 (26). As reconfirmed by the studies mentioned above, plasma EV miRNA exhibited comparatively higher diagnosis-related accuracy in terms of LUAD, especially for early diagnosis. In addition to EV miRNA, EV proteins may also be employed to identify lung carcinoma (27). Sandfeld-Paulsen et al. reported that EV membrane-attached proteins, such as CD151, CD171, and tetraspanin 8, may be potential biomarkers that can distinguish between cases with and without lung carcinoma (28). Moreover, according to An et al., fibronectin on EVs was found to perform well in the diagnosis of NSCLC cases and shows promise for future clinical use (29). Other molecules, such as mRNAs, lncRNAs, and circRNAs, have also been identified inside EVs. However, given our currently limited understanding of EV long RNAs, extensive studies should be conducted to determine their potential as lung carcinoma biomarkers. Zhang et al. reported that the EV lncRNA, MALAT-1, is highly expressed in NSCLC cases compared to healthy individuals (30). Xian et al. recently reported that 3 EV circRNAs (circ_0047921, circ_0056285, and circ_0007761) are promising biomarkers for NSCLC diagnosis (31). It is clear from these studies that EVs have long RNAs, which could be biomarkers for the noninvasive diagnosis of lung carcinoma.
In this study, nearly 11,000 exLRs could be detected in the respective samples. Among them, mRNAs and lncRNAs constituted a significant portion of the mapped reads. According to the T-SNE and PCA analyses, in terms of the exLR profile, LUAD cases were generally different from healthy individuals and some BPN cases. Moreover, through KEGG pathway analysis, enrichment of the mentioned DELRs could be seen in a few pathways that were involved in cancer progression. We then built a d-signature consisting of 8 exLRs (NFKBIA, NDUFB10, SLC7A7, ARPC5, SEPTIN9, HMGN1, H4C2, and lnc-PLA2G1B-2:3) for LUAD detection. This signature showed high diagnostic performance in the training (AUC 0.991), internal validation (AUC 0.921), and external validation (AUC 0.9) cohorts.
In a previous cancer study, Wei et al. reported that serum high-mobility group nucleosome-binding protein 1 (HMGN1) was a novel clinical biomarker of non-small cell lung cancer. Patients suffering with NSCLC were found to have significantly higher serum levels of HMGN1 than the HCs (32). In patients with NSCLC, HMGN1 overexpression may correlate with tumor development, invasion, and metastasis (33-35). Nuclear factor of κ-light polypeptide gene enhancer in B cells inhibitor α (NFKBIA), a tumor suppressor gene, was found to be silenced in LUADs (36,37). Also, Zhang et al. reported that cytoplasmic NFKBIA expression were associated with a poorer prognosis in NSCLC patients (38). Actin-related protein 2/3 complex subunit 5 (ARPC5) was found to be helpful for cell migration and invasion in head and neck squamous cell carcinoma (39). Xiong et al. demonstrated there to be a correlation between patients in the ARPC5 high expression group and poor overall survival in multiple myeloma cases; thus, they considered ARPC5 to be an independent prognostic factor (40). Furthermore, there has been dysregulation of SLC7A7 observed in varied types of cancers, including ovarian cancer, NSCLC, and glioblastoma (41-43). Overexpression of SLC7A7 enables cells to have an advantage in growth and survival under limited amino acid availability, which may lead to tumorigenesis (44). Dai et al. reported that elevated SLC7A7 expression is correlated with poor prognosis and enhanced infiltration of macrophages, neutrophils and DCs in multiple cancers, especially in NSCLC (42). The Septin 9 (SEPT9) gene has been found to be associated with a variety of human diseases, and it plays a role in the development of tumors (45). Warren et al. revealed that Septin 9 methylated DNA is a sensitive and specific blood test for colorectal cancer (46). According to Zhang et al., glioma has higher transcription levels of SEPTIN9 than does normal tissue, which may be a tumor-promoting factor in glioma (47). At present, little is known about H4C2, NDUFB10 and lnc-PLA2G1B-2:3. There are also relatively few studies on the relationship between these 8 genes and EVs.
Lung carcinoma is the leading cause of carcinoma-related mortality worldwide. Early diagnosis could help to improve the survival rate of lung carcinoma. For LUAD, if the disease is detected at the stage stage and treated with curative resection, the 5-year survival may be 80% or higher (3). However, early-stage diagnosis is difficult. This study built an exLR d-signature with the ability to distinguish stage II LUAD cases from healthy individuals and BPN cases. It is worth noting that the tumor burden was found to have little influence on the exLR d-signature scores of cases, indicating that the d-signature may be helpful for detecting tumors that are still AIS or MIA. In fact, the d-signature detected stage I LUAD from noncancerous controls (NCs) with an AUC of 0.948, a sensitivity of 85.9%, and a specificity of 89.1% in the combined 3 cohorts. Thus, from this finding, we can conclude that the exLR d-signature could be a potential biomarker for the clinical diagnosis of stage I LUAD.
There were several strengths in the present study compared to other biomarker signature studies. First, in the LUAD group, we included several cases that were in a very early stage (AIS and MIA), which is extremely difficult to diagnose by other approaches. Our results showed that the d-signature could detect AIS from noncancerous controls with a specificity of 89.1%, a sensitivity of 86.4%, and an AUC of 0.934. It also could detect MIA with a specificity of 89.1%, a sensitivity of 70.6%, and an AUC of 0.909. Identification of the mentioned cases with a noninvasive tool could improve the overall prognostic process and 5-year survival rate of LUAD. Moreover, in the NC group, we not only recruited healthy individuals (as was the case in most previous studies), but also recruited cases with pulmonary benign nodules as noncancerous controls. Thus, the possible exLR disturbance attributed to other factors was considered in advance in the screening of biomarker. Also, the low false-positive rate in this study suggests that it has the ability to prevent unnecessary lung resection for BPN cases.
However, there were several limitations to this study that should be noted. Firstly, this study may be limited by selection bias due to the limited number of participants. Second, there was a multicentric validation study performed based on the patient samples from 3 different centers in Beijing and a validation of the exLR d-signature in large cohorts from other regions in China. However, the efficacy and stability of this diagnostic method may be improved by its application in other ethnic populations or other countries. Third, the non-cancerous control samples in our study are from the healthy crowd in our hospital and the patients who underwent surgery in our department but proved to be benign nodule by pathological evaluation after surgery. So, the study participants are generally younger and the majority have no smoking history. Thus, validation of the signature using a larger cohort that matches the screening criteria of being at high risk of lung cancer is needed in the future. Finally, our study lacked prognostic information, as all of the included cases were newly diagnosed. Thus, the prognostic value of this exLR signature could not be evaluated.
Conclusions
Stage I LUAD cases exhibited a unique plasma exLR profile compared with NCs. The exLR d-signature demonstrated relatively high sensitivity and specificity in distinguishing cases with early LUAD from both HCs and BPN cases. The exLR d-signature is a promising potential noninvasive biomarker for the early detection and routine screening of LUAD.
Acknowledgments
We appreciate the support of the physicians and patients who participated in this study.
Funding: This study was supported by the National Key R&D Program of China (Nos. 2017YFC1311000, 2018YFC1312100, 2019YFC1315700), the National Natural Science Foundation of China (Nos. 82002451, 82122053), the Beijing Municipal Science & Technology Commission (No. Z191100006619117), R&D Program of Beijing Municipal Education commission (No. KJZD20191002302), CAMS Initiative for Innovative Medicine (Nos. 2017-I2M-1-005, 2017-I2M-2-003, 2019-I2M-2-002, 2021-1-I2M-012, 2021-1-I2M-015), Non-profit Central Research Institute Fund of Chinese Academy of Medical Sciences (Nos. 2018PT32033, 2017PT32017, 2021-PT310-001), Innovation team development project of Ministry of Education (No. IRT_17R10), and the Beijing Hope Run Special Fund of Cancer Foundation of China (No. LC2019B15).
Footnote
Reporting Checklist: The authors have completed the STARD reporting checklist. Available at https://tlcr.amegroups.com/article/view/10.21037/tlcr-21-729/rc
Data Sharing Statement: Available at https://tlcr.amegroups.com/article/view/10.21037/tlcr-21-729/dss
Peer Review File: Available at https://tlcr.amegroups.com/article/view/10.21037/tlcr-21-729/prf
Conflicts of Interest: All authors have completed the ICMJE uniform disclosure form (available at https://tlcr.amegroups.com/article/view/10.21037/tlcr-21-729/coif). XL is an employee of Echo Biotech Co., Ltd. The other authors have no conflicts of interest to declare.
Ethical Statement: The authors are accountable for all aspects of the work in ensuring that questions related to the accuracy or integrity of any part of the work are appropriately investigated and resolved. The study was conducted in accordance with the Declaration of Helsinki (as revised in 2013). The study was approved by the clinical research ethics committee of National Cancer Center/Cancer Hospital, Chinese Academy of Medical Sciences (Approval number: 20/370-2155). All patients provided written informed consent.
Open Access Statement: This is an Open Access article distributed in accordance with the Creative Commons Attribution-NonCommercial-NoDerivs 4.0 International License (CC BY-NC-ND 4.0), which permits the non-commercial replication and distribution of the article with the strict proviso that no changes or edits are made and the original work is properly cited (including links to both the formal publication through the relevant DOI and the license). See: https://creativecommons.org/licenses/by-nc-nd/4.0/.
References
- Siegel RL, Miller KD, Jemal A. Cancer statistics, 2019. CA Cancer J Clin 2019;69:7-34. [Crossref] [PubMed]
- Chen W, Zheng R, Baade PD, et al. Cancer statistics in China, 2015. CA Cancer J Clin 2016;66:115-32. [Crossref] [PubMed]
- Goldstraw P, Chansky K, Crowley J, et al. The IASLC Lung Cancer Staging Project: Proposals for Revision of the TNM Stage Groupings in the Forthcoming (Eighth) Edition of the TNM Classification for Lung Cancer. J Thorac Oncol 2016;11:39-51. [Crossref] [PubMed]
- Maeshima AM, Tochigi N, Yoshida A, et al. Histological scoring for small lung adenocarcinomas 2 cm or less in diameter: a reliable prognostic indicator. J Thorac Oncol 2010;5:333-9. [Crossref] [PubMed]
- Yim J, Zhu LC, Chiriboga L, et al. Histologic features are important prognostic indicators in early stages lung adenocarcinomas. Mod Pathol 2007;20:233-41. [Crossref] [PubMed]
- Travis WD, Brambilla E, Noguchi M, et al. International association for the study of lung cancer/american thoracic society/european respiratory society international multidisciplinary classification of lung adenocarcinoma. J Thorac Oncol 2011;6:244-85. [Crossref] [PubMed]
- Zhao W, Yang J, Sun Y, et al. 3D Deep Learning from CT Scans Predicts Tumor Invasiveness of Subcentimeter Pulmonary Adenocarcinomas. Cancer Res 2018;78:6881-9. [Crossref] [PubMed]
- Patz EF Jr, Pinsky P, Gatsonis C, et al. Overdiagnosis in low-dose computed tomography screening for lung cancer. JAMA Intern Med 2014;174:269-74. [Crossref] [PubMed]
- Rijavec E, Coco S, Genova C, et al. Liquid Biopsy in Non-Small Cell Lung Cancer: Highlights and Challenges. Cancers (Basel) 2019;12:17. [Crossref] [PubMed]
- Guo W, Gao Y, Li N, et al. Exosomes: New players in cancer Oncol Rep 2017;38:665-75. (Review). [Crossref] [PubMed]
- van Niel G, D'Angelo G, Raposo G. Shedding light on the cell biology of extracellular vesicles. Nat Rev Mol Cell Biol 2018;19:213-28. [Crossref] [PubMed]
- Melo SA, Luecke LB, Kahlert C, et al. Glypican-1 identifies cancer exosomes and detects early pancreatic cancer. Nature 2015;523:177-82. [Crossref] [PubMed]
- Sun Z, Shi K, Yang S, et al. Effect of exosomal miRNA on cancer biology and clinical applications. Mol Cancer 2018;17:147. [Crossref] [PubMed]
- Huang X, Yuan T, Liang M, et al. Exosomal miR-1290 and miR-375 as prognostic markers in castration-resistant prostate cancer. Eur Urol 2015;67:33-41. [Crossref] [PubMed]
- Zhou R, Chen KK, Zhang J, et al. The decade of exosomal long RNA species: an emerging cancer antagonist. Mol Cancer 2018;17:75. [Crossref] [PubMed]
- Yu S, Li Y, Liao Z, et al. Plasma extracellular vesicle long RNA profiling identifies a diagnostic signature for the detection of pancreatic ductal adenocarcinoma. Gut 2020;69:540-50. [Crossref] [PubMed]
- Del Re M, Marconcini R, Pasquini G, et al. PD-L1 mRNA expression in plasma-derived exosomes is associated with response to anti-PD-1 antibodies in melanoma and NSCLC. Br J Cancer 2018;118:820-4. [Crossref] [PubMed]
- Sandfeld-Paulsen B, Aggerholm-Pedersen N, Bæk R, et al. Exosomal proteins as prognostic biomarkers in non-small cell lung cancer. Mol Oncol 2016;10:1595-602. [Crossref] [PubMed]
- Jin X, Chen Y, Chen H, et al. Evaluation of Tumor-Derived Exosomal miRNA as Potential Diagnostic Biomarkers for Early-Stage Non-Small Cell Lung Cancer Using Next-Generation Sequencing. Clin Cancer Res 2017;23:5311-9. [Crossref] [PubMed]
- Cazzoli R, Buttitta F, Di Nicola M, et al. microRNAs derived from circulating exosomes as noninvasive biomarkers for screening and diagnosing lung cancer. J Thorac Oncol 2013;8:1156-62. [Crossref] [PubMed]
- Silva J, García V, Zaballos Á, et al. Vesicle-related microRNAs in plasma of nonsmall cell lung cancer patients and correlation with survival. Eur Respir J 2011;37:617-23. [Crossref] [PubMed]
- Zhang JT, Qin H, Man Cheung FK, et al. Plasma extracellular vesicle microRNAs for pulmonary ground-glass nodules. J Extracell Vesicles 2019;8:1663666. [Crossref] [PubMed]
- Théry C, Amigorena S, Raposo G, et al. Isolation and characterization of exosomes from cell culture supernatants and biological fluids. Curr Protoc Cell Biol 2006;Chapter 3:Unit 3.22.
- EV-TRACK Consortium. EV-TRACK: transparent reporting and centralizing knowledge in extracellular vesicle research. Nat Methods 2017;14:228-32. [Crossref] [PubMed]
- Li S, Li Y, Chen B, et al. exoRBase: a database of circRNA, lncRNA and mRNA in human blood exosomes. Nucleic Acids Res 2018;46:D106-12. [Crossref] [PubMed]
- Yao B, Qu S, Hu R, et al. A panel of miRNAs derived from plasma extracellular vesicles as novel diagnostic biomarkers of lung adenocarcinoma. FEBS Open Bio 2019;9:2149-58. [Crossref] [PubMed]
- Cui S, Cheng Z, Qin W, et al. Exosomes as a liquid biopsy for lung cancer. Lung Cancer 2018;116:46-54. [Crossref] [PubMed]
- Sandfeld-Paulsen B, Jakobsen KR, Bæk R, et al. Exosomal Proteins as Diagnostic Biomarkers in Lung Cancer. J Thorac Oncol 2016;11:1701-10. [Crossref] [PubMed]
- An T, Qin S, Sun D, et al. Unique Protein Profiles of Extracellular Vesicles as Diagnostic Biomarkers for Early and Advanced Non-Small Cell Lung Cancer. Proteomics 2019;19:e1800160. [Crossref] [PubMed]
- Zhang R, Xia Y, Wang Z, et al. Serum long non coding RNA MALAT-1 protected by exosomes is up-regulated and promotes cell proliferation and migration in non-small cell lung cancer. Biochem Biophys Res Commun 2017;490:406-14. [Crossref] [PubMed]
- Xian J, Su W, Liu L, et al. Identification of Three Circular RNA Cargoes in Serum Exosomes as Diagnostic Biomarkers of Non-Small-Cell Lung Cancer in the Chinese Population. J Mol Diagn 2020;22:1096-108. [Crossref] [PubMed]
- Wei F, Yang F, Jiang X, et al. High-mobility group nucleosome-binding protein 1 is a novel clinical biomarker in non-small cell lung cancer. Tumour Biol 2015;36:9405-10. [Crossref] [PubMed]
- Shang GH, Jia CQ, Tian H, et al. Serum high mobility group box protein 1 as a clinical marker for non-small cell lung cancer. Respir Med 2009;103:1949-53. [Crossref] [PubMed]
- Wang JL, Wu DW, Cheng ZZ, et al. Expression of high mobility group box - B1 (HMGB-1) and matrix metalloproteinase-9 (MMP-9) in non-small cell lung cancer (NSCLC). Asian Pac J Cancer Prev 2014;15:4865-9. [Crossref] [PubMed]
- Zhang X, Wang H, Wang J. Expression of HMGB1 and NF-κB p65 and its significance in non-small cell lung cancer. Contemp Oncol (Pozn) 2013;17:350-5. [Crossref] [PubMed]
- Karin M, Greten FR. NF-kappaB: linking inflammation and immunity to cancer development and progression. Nat Rev Immunol 2005;5:749-59. [Crossref] [PubMed]
- Furukawa M, Soh J, Yamamoto H, et al. Silenced expression of NFKBIA in lung adenocarcinoma patients with a never-smoking history. Acta Med Okayama 2013;67:19-24. [PubMed]
- Zhang D, Jin X, Wang F, et al. Combined prognostic value of both RelA and IkappaB-alpha expression in human non-small cell lung cancer. Ann Surg Oncol 2007;14:3581-92. [Crossref] [PubMed]
- Kinoshita T, Nohata N, Watanabe-Takano H, et al. Actin-related protein 2/3 complex subunit 5 (ARPC5) contributes to cell migration and invasion and is directly regulated by tumor-suppressive microRNA-133a in head and neck squamous cell carcinoma. Int J Oncol 2012;40:1770-8. [PubMed]
- Xiong T, Luo Z. The Expression of Actin-Related Protein 2/3 Complex Subunit 5 (ARPC5) Expression in Multiple Myeloma and its Prognostic Significance. Med Sci Monit 2018;24:6340-8. [Crossref] [PubMed]
- Fan S, Meng D, Xu T, et al. Overexpression of SLC7A7 predicts poor progression-free and overall survival in patients with glioblastoma. Med Oncol 2013;30:384. [Crossref] [PubMed]
- Dai W, Feng J, Hu X, et al. SLC7A7 is a prognostic biomarker correlated with immune infiltrates in non-small cell lung cancer. Cancer Cell Int 2021;21:106. [Crossref] [PubMed]
- Sun T, Bi F, Liu Z, et al. SLC7A2 serves as a potential biomarker and therapeutic target for ovarian cancer. Aging (Albany NY) 2020;12:13281-96. [Crossref] [PubMed]
- Wang Q, Bailey CG, Ng C, et al. Androgen receptor and nutrient signaling pathways coordinate the demand for increased amino acid transport during prostate cancer progression. Cancer Res 2011;71:7525-36. [Crossref] [PubMed]
- Sun J, Zheng MY, Li YW, et al. Structure and function of Septin 9 and its role in human malignant tumors. World J Gastrointest Oncol 2020;12:619-31. [Crossref] [PubMed]
- Warren JD, Xiong W, Bunker AM, et al. Septin 9 methylated DNA is a sensitive and specific blood test for colorectal cancer. BMC Med 2011;9:133. [Crossref] [PubMed]
- Zhang G, Feng W, Wu J. Down-regulation of SEPT9 inhibits glioma progression through suppressing TGF-β-induced epithelial-mesenchymal transition (EMT). Biomed Pharmacother 2020;125:109768. [Crossref] [PubMed]