Deep learning for predicting immunotherapeutic efficacy in advanced non-small cell lung cancer patients: a retrospective study combining progression-free survival risk and overall survival risk
Introduction
Immune checkpoint inhibitors (ICIs) targeting programmed cell death 1 (PD-1) and its ligand (PD-L1) have been shown to confer durable antitumor efficacy, dramatically revolutionizing the therapeutic paradigms of various types of malignancies, including advanced non-small cell lung cancer (NSCLC) (1-4). Despite this important breakthrough, an objective response to immunotherapy occurs in only approximately 20% of unselected patients with advanced NSCLC (5-8). Therefore, accurately identifying patients who potentially benefit from ICIs is of paramount importance for the treatment optimization of advanced NSCLC.
Previous studies have analyzed several predictive biomarkers of response to ICIs in advanced NSCLC, including tumor mutation burden (TMB) (9-11), PD-L1 expression (12-14), tumor-infiltrating lymphocytes (15,16), and inflammatory cytokines (17). The PD-L1 expression represents the only biomarker in clinical practice capable of guide the decision in first line treatment NSCLC. However, the current standard for identifying PD-L1 expression mainly relies on biopsy, which cannot characterize the whole landscape of tumor microenvironment due to the small size of biopsy specimens, therefore, potentially leading to the limitation in diagnostic accuracy. In addition, several trials demonstrated that PD-L1 expression could not accurately recognize patients sensitive to immunotherapy, ICIs might benefit patients with negative PD-L1 expression, its predictive accuracy for immunotherapeutic efficacy was unsatisfactory (18,19). Thus, there is a significant unmet need for a robust and noninvasive biomarker to predict the efficacy of ICIs in patients with advanced NSCLC.
The use of radiomics for quantitative analysis of solid tumors has been recently proposed (20). This method explores the deep-level tumor imaging features that cannot be discovered by the human eye, constructing a corresponding auxiliary diagnostic model based on different clinical problems (21). Deep learning, as a new branch of radiomics, has also developed rapidly (22), playing an increasingly significant role in clinical application of various solid tumors, including gastric cancer (23), breast cancer (24,25), and NSCLC (26). The combination of medical imaging research with deep learning technology has led to further development in many clinical fields. The application range of this technology includes disease diagnosis (27), treatment selection (28), and prognosis prediction (29,30). In addition, a study has confirmed that there are significant differences in computed tomography (CT) images of patients in different ICI treatment cycles (31).
Previous studies have usually relied on existing proven prognostic factors as the prediction target of deep learning to predict the prognosis of immunotherapy (32-35). In this study, we aimed to use CT images combined with deep learning to find a more accurate radiomic score construction method using multiple prognostic indicators for evaluating the clinical outcome of advanced NSCLC patients treated with ICIs. We present the following article in accordance with the TRIPOD reporting checklist (available at https://tlcr.amegroups.com/article/view/10.21037/tlcr-22-244/rc).
Methods
Patients
The study was conducted in accordance with the Declaration of Helsinki (as revised in 2013) and was approved by the ethics committee of Shanghai Pulmonary Hospital (L20-333-1). Informed consent was waived considering the retrospective nature of this study. Patients who received anti-PD-1/PD-L1 monotherapy for advanced NSCLC and had undergone chest CT scans within 2 weeks before immunotherapy at the Shanghai Pulmonary Hospital between January 2015 and December 2019 were retrospectively included. Patients were excluded if any one of the following criteria was met: poor quality of CT images, incomplete baseline data, mixed tumor histologic type, and lost to follow-up. The baseline characteristics, including gender, age, Eastern Cooperative Oncology Group performance-status score (ECOG PS), pathological stage, and tumor histologic type, were retrospectively collected. Follow-up information was acquired from outpatient records and telephone interviews. Response in general to immune checkpoint blockade was assessed according to the Response Evaluation Criteria in Solid Tumors (RECIST) version 1.1 (36). Progression-free survival (PFS) was calculated as the time from immunotherapy administration to tumor progression or death from any cause or last follow-up. Overall survival (OS) was estimated as the time from tumor diagnosis until death or last follow-up.
The patients were divided into a modelling cohort (n=164), validation cohort (n=24), and testing cohort (n=48) using stratified randomization. The modelling cohort was used for deep network training, the validation cohort was used to optimize network parameters, and the test cohort was used to evaluate the network. Since both the modelling cohort and the validation cohort were used in the training phase of the network, we collectively refer to them as the training cohort (n=188). The construction, optimization, and evaluation of each network used the same modelling cohort, validation cohort, and test cohort.
CT image and tumor segmentation
Chest CT scans were performed using instruments by Siemens (Somatom Definition AS+, Biograph 64, Munich, Germany), Philips (Brilliance 40, iCT 256, Ingenuity Flex, MX 16-slice, Amsterdam, Netherlands), GE Medical System (Bright Speed, Boston, USA), and United Imaging (uCT 510, uCT 760, uCT S-160, Shanghai, China). All images were reconstructed and then imported into 3D Slicer (http://www.slicer.org) for segmentation.
The region of interest (ROI) was annotated by a bounding box including the entire tumor volume. Two radiologists (T.T.W and Y.Y) independently performed tumor segmentation in the lung window setting [mean, −450 Hounsfield unit (HU); width, 1,500 HU], and interobserver disagreements were resolved by consulting a senior radiologist (X.W.S) with more than 10 years of experience.
The segmented 3-dimensional (3D) tumor images were preprocessed before training the networks. The upper and lower bounds of HU values in CT images were set as 1,024 and −1,024, respectively, and we used 3D tumor images for z-score normalization based on the dataset. In addition, we performed multi-view data augmentation in order to increase the number of samples and improve the generalization ability of the network (37). The data augmentation method is detailed in Appendix 1 & Figure S1.
Experimental design and main flow overview
In this study, we aimed to find prognosis evaluation differences when various prognostic indicators were combined by deep learning as prediction targets and also to construct a deep learning network for follow-up information to obtain accurate risks. Based on these goals, we collected 2 types of prognosis-related information: optimal immune response [partial response (PR), stable disease (SD), progressive disease (PD)] and follow-up information.
Our research consisted of 3 parts. The first was to build a survival network for follow-up information to obtain an OS risk and PFS risk for patients. Later, we combined OS risk and PFS risk to conduct more in-depth exploration of patient prognosis assessment to improve precision. Meanwhile, we constructed a classification network based on the optimal immune response to obtain the PR and PD probabilities of patients, and we compared the difference between the optimal immune response model and the prognostic information model in prognostic evaluation (Figure 1). The inputs of all networks were the CT images. At the end of the study, we exported the class activation maps of all risk scores to observe the differences in the areas of concern when predicting PFS and OS risks.
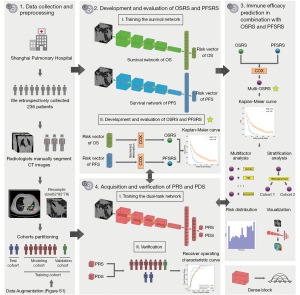
Acquisition and verification of OS risk score (OSRS) and PFS risk score (PFSRS)
The survival network, which was different from previous studies (38,39), contained 2 modules: a convolutional module and classification module. The convolutional module was a dense-like network (the number of convolutional layers included in each dense block was 6, 12, 12, and 6), and the main function was to extract deep learning features. The classification network was a fully connected network. A total of 508 deep learning features extracted by the convolutional module reached a hidden layer containing 256 nodes after passing through the input layer. The classification network was designed to directly output a risk vector with 3 scores, with each score related to whether the patient had an endpoint in the time interval. The points of trisection in the time dimension of the sample with endpoint in the training cohort were selected as the cut-off points. The OS cut-off points in this study were 135 days and 282 days, and the PFS cut-off points were 98 and 213 days. Meanwhile, we designed different loss functions for patients with multiple goals and single goals in the risk vector. For patients with multiple goals, we defined the sample loss function as follows:
For patients with single goals, we defined the sample loss as follows:
Information about the survival network is detailed in Appendix 2. After obtaining the risk vectors, we employed backward selection via the Cox regression model to fuse the risk vectors to acquire accurate patient risks. OSRS was the risk value obtained by using the fusion OS risk vector, and PFSRS was the risk value obtained by using the fusion PFS risk vector.
In the evaluation stage, we used the macro-accuracy that could better evaluate each category to assess the risk vector, and we mapped each patient’s risk vector to 3D coordinates to observe its spatial differences. For OSRS and PFSRS, we chose the Kaplan-Meier curve and log-rank test to evaluate the risk stratification ability of OSRS and PFSRS. Otherwise, concordance index (C-index) and hazard ratio (HR) were used as evaluation indicators.
Immune efficacy prediction in combination with OSRS and PFSRS
As PFS and OS are closely related, it is necessary to combine them for analysis. Therefore, we performed Cox regression analysis to merge PFSRS into OSRS to restore the original appearance of survival prognosis, and the final score was named Multi-OSRS (MOSRS). In addition, we displayed the distribution maps of OSRS, PFSRS, and MOSRS based on patient follow-up information. The class activation maps of PFSRS and OSRS were generated and the structural similarity between them was calculated. Finally, the similarity coefficient and all risk scores were analyzed by Spearman’s correlation to quantitatively analyze differences in the observation areas of risk scores when predicting PFS and OS.
To verify the ability of MOSRS to divide patients into high- and low-risk groups, we used the Kaplan-Meier curve, HR, C-index, and log-rank test for evaluation. Further, we used MOSRS to test subgroups of different tumor sizes (the 3D maximum diameter of the tumor) and different tumor histologic type to evaluate the prognostic value of MOSRS.
Acquisition and verification of PR score (PRS) and PD score (PDS)
We constructed PRS and PDS to simultaneously predict the PR and PD of patients via a dual-task network. The advantage of multitask learning is the ability to process multiple tasks through 1 network to identify the expression of common features among network learning tasks, thereby improving the generalization ability of the results (40). The dual-task network in this study had similar components to the survival network, with both including a convolutional module and classification module. In the output layer, the network directly predicted the PR and PD of patients. The training process and parameters are shown in Appendix 3.
When verifying the PRS and PDS, the receiver operating characteristic curve (ROC) was used to evaluate the performance of PRS and PDS, and area under the curve (AUC) was selected as indicator of quantitative evaluation. The best cut-off point was chosen by the Youden index. In addition, to comprehensively evaluate the predictive power of PRS and PDS, we included the thickness, voxel spacing, and tumor histologic type that might be potential influencing factors for subgroup analysis. We also performed the log-rank test to evaluate the ability of PRS and PDS to stratify patient risk.
Statistical analysis
Discrete and continuous baseline characteristics of patients were compared through Chi-square test and Mann-Whitney U test, respectively. For categorical variables output by the network, ROC and AUC were employed to evaluate PRS and PDS, and macro-accuracy was used to measure the performance of risk vectors. For survival risk, we chose Kaplan-Meier curve, log-rank test, C-index, and HR to evaluate the stratification ability of OSRS, PFSRS, and MOSRS. X-tile was used to select the best cut-off point (41). Otherwise, all analyses were performed in R (version 3.5.2; http://www.R-project.org) and Python (version 3.6.7; http://www.python.org/). A two-sided P value less than 0.05 was considered a significant difference, an AUC more than 0.75 was considered as a satisfactory predictive efficiency. The Python and R packages are summarized in Appendix 4.
Results
Clinicopathological characteristics
In order to assess the role of CT in predicting the efficacy of immunotherapy as accurately as possible, 236 patients who had received the first-line ICIs were retrospectively enrolled in this study. The clinical characteristics of the patients are summarized in Table 1.
Table 1
Characteristics | Total (N=236) | Training cohort (N=188) | P value* | Test cohort (N=48) | P value** | |
---|---|---|---|---|---|---|
Modelling cohort (N=164) | Validation cohort (N=24) | |||||
Gender | 0.84 | 0.56 | ||||
Female | 40 (0.17) | 26 (0.16) | 4 (0.17) | 10 (0.21) | ||
Male | 196 (0.83) | 138 (0.84) | 20 (0.83) | 38 (0.79) | ||
Median age [range] (years) | 64 [57–70] | 64 [57–70] | 64 [61–67] | 0.34 | 64 [59–69] | 0.34 |
Smoking status | 0.66 | 0.75 | ||||
Never smoked | 45 (0.19) | 33 (0.20) | 3 (0.12) | 9 (0.19) | ||
Current or former smoker | 83 (0.35) | 59 (0.36) | 9 (0.38) | 15 (0.31) | ||
Unknown | 108 (0.46) | 72 (0.44) | 12 (0.50) | 24 (0.50) | ||
ECOG performance-status score | 0.02 | 0.69 | ||||
0 | 11 (0.05) | 9 (0.05) | 0 (0.0) | 2 (0.04) | ||
1 | 112 (0.47) | 81 (0.49) | 9 (0.38) | 22 (0.46) | ||
2 | 6 (0.03) | 3 (0.02) | 3 (0.12) | 0 (0.0) | ||
Unknown | 107 (0.45) | 71 (0.43) | 12 (0.50) | 24 (0.50) | ||
Clinical stage | 0.80 | 0.67 | ||||
III | 13 (0.06) | 10 (0.06) | 1 (0.04) | 2 (0.04) | ||
IV | 116 (0.49) | 83 (0.51) | 11 (0.46) | 22 (0.46) | ||
Unknown | 107 (0.45) | 71 (0.43) | 12 (0.50) | 24 (0.50) | ||
Tumor histologic type | 0.35 | 0.69 | ||||
Squamous cell carcinoma | 71 (0.30) | 51 (0.31) | 4 (0.17) | 16 (0.33) | ||
Adenocarcinoma | 119 (0.50) | 80 (0.49) | 14 (0.58) | 25 (0.52) | ||
Others | 46 (0.19) | 33 (0.20) | 6 (0.25) | 7 (0.15) | ||
Tumor mutation | 0.68 | 0.50 | ||||
No mutation | 104 (0.44) | 77 (0.47) | 9 (0.38) | 18 (0.38) | ||
Mutation | 25 (0.11) | 16 (0.10) | 3 (0.12) | 6 (0.12) | ||
Unknown | 107 (0.45) | 71 (0.43) | 12 (0.50) | 24 (0.50) | ||
Optimal immune response | 0.96 | 0.93 | ||||
Progressive disease | 62 (0.26) | 44 (0.27) | 6 (0.25) | 12 (0.25) | ||
Stable disease | 119 (0.50) | 83 (0.51) | 12 (0.50) | 24 (0.50) | ||
Partial response | 55 (0.23) | 37 (0.23) | 6 (0.25) | 12 (0.25) | ||
Progression-free survival outcome | 0.84 | 0.15 | ||||
No event | 94 (0.40) | 61 (0.37) | 9 (0.38) | 24 (0.50) | ||
Event | 142 (0.60) | 103 (0.63) | 15 (0.62) | 24 (0.50) | ||
Progression-free survival time (days) | ||||||
No event | 352.48±222.19 | 353.87±216.33 | 411.67±259.73 | 0.31 | 326.75±217.10 | 0.32 |
Overall survival outcome | 0.40 | 0.34 | ||||
No event | 174 (0.74) | 120 (0.73) | 15 (0.62) | 39 (0.81) | ||
Event | 62 (0.26) | 44 (0.27) | 9 (0.38) | 9 (0.19) | ||
Overall survival time (days) | ||||||
No event | 374.11±232.58 | 379.69±237.26 | 429.33±254.56 | 0.27 | 249.43±198.05 | 0.19 |
Event | 221.94±184.21 | 233.75±201.12 | 208.44±133.77 | 0.50 | 177.67± 123.68 | 0.28 |
Voxel spacing (mm) | 0.75±0.09 | 0.75±0.09 | 0.75±0.09 | 0.37 | 0.76±0.09 | 0.37 |
Thickness (mm) | 0.82±0.24 | 0.82±0.26 | 0.82±0.24 | 0.20 | 0.83±0.22 | 0.20 |
Categorical data are shown as numbers (proportion) and continuous data as mean ± SD or median [range]. *, P value is the test result of the training cohort and the validation cohort; **, P value is the test result of the training cohort and the test cohort. ECOG, Eastern Cooperative Oncology Group.
The proportion of male patients in the dataset was larger (83%). The median age of all patients was 64 years, and the most common histologic type was adenocarcinoma (50%). For the clinical characteristics with incomplete data, stage IV (49%) accounted for the highest proportion of clinical stage, the majority of patients were classified as current or former smokers (35%), and the number of patients with oncogenic alterations was lower (11%) than those without alterations. With respect to immune response, the number of patients with disease progression (26%) was slightly more than that of patients with PR (23%). In terms of prognostic information, progress in PFS was found in 142 (60%) patients and 62 (26%) patients had an endpoint event in OS. The median OS and PFS of all patients were 296.5 (range, 16–1,128) days and 181 (range, 15–1,010) days, respectively.
Risk assessment of OSRS and PFSRS
We trained survival networks for OS and PFS to obtain risk vectors of OS and PFS. The macro-accuracy of the OS risk vector and PFS risk vector were 77.4% and 81.6% in the training cohort, respectively, and 83.3% and 77.5% in the test cohort, respectively, indicating good multicategory prediction ability. Meanwhile, we constructed a 3D space to visualize the risk vectors of patients who had an endpoint event (Figure 2A,2B). Whether predicting PFS or OS, we observed that all patients were aggregated into 3 clusters with spatial differences in the 3D space. Since the event time in some patients was near the cut-off time, there were also some intertwined samples in the figure.
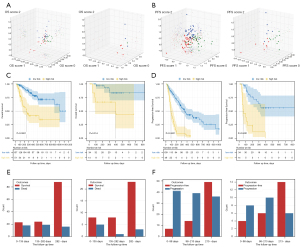
To develop the OSRS, we used an OS risk vector which was composed of OS score 1, OS score 2, and OS score 3 for backward selection via the Cox regression model. In the end, only OS score 3 (multivariate P<0.001) was retained and formed into OSRS. The results showed that OSRS for patients in the training cohort [cut-off point =0.64; HR: 4.14, 95% confidence interval (CI): 2.40–7.15; log-rank P<0.001; Figure 2C] and test cohort (HR: 4.54, 95% CI: 1.21–16.94; log-rank P=0.014; Figure 2C) had excellent risk stratification ability, with the C-index in the training cohort and test cohort 0.73 (95% CI: 0.66–0.80) and 0.75 (95% CI: 0.59–0.90), respectively. To develop the PFSRS, we used a PFS risk vector which was composed of PFS score 1, PFS score 2, and PFS score 3 for backward selection via the Cox regression model. PFS score 2 (multivariate P=0.002) and PFS score 3 (multivariate P<0.001) were singled out for the PFSRS. The results showed that PFSRS could significantly divide patients into high-risk and low-risk groups in both the training cohort (cut-off point =0.51; HR: 4.52, 95% CI: 3.04–6.70; log-rank P<0.001; Figure 2D) and test cohort (HR: 6.64, 95% CI: 2.89–15.29; log-rank P<0.001; Figure 2D). The C-index of the training cohort and test cohort were 0.72 (95% CI: 0.68–0.77) and 0.70 (95% CI: 0.59–0.81), respectively. Clinical characteristics based on OSRS and PFSRS grouping are displayed in Tables S1,S2, respectively.
To explore the reasons why score 3 was more easily selected in OS and PFS risk vectors, we performed statistical analysis on samples that contributed to different scores (Figure 2E,2F). We found that for the third category, the nonclinical endpoint sample was the largest no matter which risk vector was trained. These samples included the patients in which the endpoint event occurred and also the patients in which the event did not appear in the time period. This was in line with the principle of our survival network training.
Multivariable deep learning signatures for prediction of patient outcomes
Although OSRS and PFSRS had significant stratification capabilities, they did not make full use of the prognostic information of patients when used alone. We integrated OSRS and PFSRS to predict the OS. Both OSRS (multivariate P<0.001) and PFSRS (multivariate P<0.001) were significant in the Cox regression model. The MOSRS obtained by the fusion of OSRS and PFSRS could significantly stratify patients in the training cohort (cut-off point =0.76; HR: 8.44, 95% CI: 4.62–15.44; C-index: 0.77, 95% CI: 0.71–0.83; log-rank P<0.001; Figure 3A) and test cohort (HR: 6.79, 95% CI: 1.69–27.28; C-index: 0.79, 95% CI: 0.63–0.94; log-rank P<0.001; Figure 3A). We also combined OSRS and PFSRS to analyse PFS, and the results showed that OSRS was not significant in the model (multivariate P=0.848). Clinical characteristics based on MOSRS grouping are displayed in Table S3.
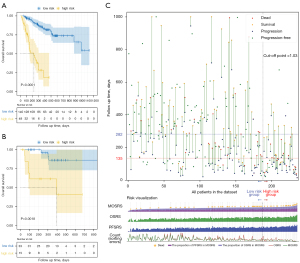
Then, we combined prognostic information, OSRS, PFSRS, and MOSRS to draw Figure 3B, in order to show the better-quality details of MOSRS compared to OSRS. Figure 3B is divided into upper and lower modules. The abscissas of the two modules represent different patients, and all patients are sorted in order of MOSRS, from small to large. The upper module reflects the survival of all patients, and the ordinate represents the follow-up time (the upper limit set in the figure is 1,000 days). The lower module of the figure is a bar graph of each score and a line graph of the number of sorting errors which is based on the C-index. We observed that with an increase in MOSRS, the density of events per unit time gradually increased, and the time of death was gradually reduced. In addition, 1 patient progressed and died only 16 days after treatment, and his MOSRS was obviously higher than the remaining high-risk patients. Further, we found that that OSRS was uneven in the MOSRS-based arrangement, and PFSRS had a potential corrective effect, especially for the 161th patient. This patient had a lower OSRS than others but a higher PFSRS. The line graph (Figure 3C) of the wrong sorting shows that the number of incorrectly sorted patients decreased from 153 to 44 (the red line represents OSRS and the green line represents MOSRS). A similar situation also occurred in patients with higher MOSRS. We observed that some patients had significantly reduced sequencing errors. Therefore, when analysing the efficacy of ICI treatment, judgments and studies should be made in conjunction with variables related to patient progress and survival.
In addition, we performed univariate analysis of clinical characteristics and multivariate analysis combined with MOSRS, and all scores were normalized to nonnegative numbers via a nomogram. (Figure 4A,4B). The results showed that in the univariate analysis, only ECOG PS was significantly related to the patient’s OS, and no characteristics were significant in the multivariate analysis with MOSRS. Further, we tested the stratified analysis of MOSRS in different tumor histologic type (squamous cell carcinoma and adenocarcinoma) and different tumor size (3D maximum diameter). The results showed that MOSRS showed excellent stratification effects in all subgroups (all log-rank P values were less than 0.001; Figure 4C,4D).
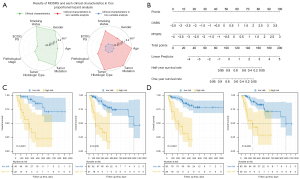
Using PRS and PDS to predict immunotherapy response
We obtained the PRS and PDS of the patients by training the dual-task network. The results of the dual-task network are displayed in Figure S2. PRS could significantly predict the optimal immune efficacy, whether it was verified in the training cohort (cut-off point: 0.36; AUC: 0.81, 95% CI: 0.74–0.87) or the test cohort (AUC: 0.78, 95% CI: 0.63–0.91). Compared with PRS, PDS also showed excellent predictive performance, which was a good indicator of whether the patient was progressing in both the training cohort (cut-off point: 0.55; AUC: 0.78, 95% CI: 0.70–0.85) and the test cohort (AUC: 0.78, 95% CI: 0.65–0.91). Meanwhile, the results of 2 scores at different tumor histologic type cohorts, thicknesses cohorts, and voxel spacing cohorts indicated that these factors would not affect the score performance.
We also attempted to stratify patient risk using PRS and PDS. The results showed that PRS could not significantly stratify the risk of patients for OS (log-rank P=0.441), even if it could distinguish whether the patient was PR. However, PDS differed from PR as it could classify patients well and also stratify patients with high and low risks for PFS (log-rank P<0.001). To further explore the association of different prognostic indicators, we conducted a multivariable analysis of these scores. PRS was significant when analysed with OSRS (log-rank P=0.045), but it was not significant when combined with MOSRS (log-rank P=0.082). PDS was not significant in the models combined with PFSRS (log-rank P=0.738) and OSRS (log-rank P=0.170). The results showed that there was potential collinearity among PRS, PDS, and PFSRS, which may reflect the tumor’s response to early immunotherapy. In addition, the optimal immune response may be affected by the follow-up time dimension, and the lack of a fixed time may have introduced image differences. Therefore, modelling with follow-up time and endpoint may be more accurate in prognosis assessment.
Visual analysis
The development process of MOSRS is summarized in Figure 5. We selected the dual-task network, OS survival network, and PFS survival network for visualization by gradient-weighted class activation mapping (Grad-Cam) (42).
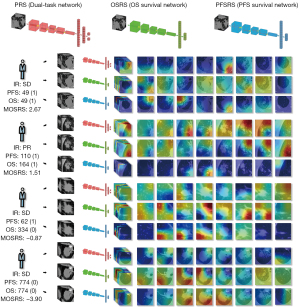
We selected 4 patients with representative prognostic information and displayed the results of the 3 models on the patient unit. We found that regardless of whether we used optimal immune response or prognostic information as our deep learning training goals, the key areas of the 3 networks were the tumor microenvironment with certain similarities. This result was consistent with our previous research conclusions (33). Further, the results of the quantitative evaluation showed that the structural similarity of the regions concerned with OSRS and PFSRS showed a significant negative correlation with the 3 risk scores (Figure 6). This was a very interesting finding and characterized the greater the risk, the smaller the similarity of the observation area. In other words, in the mechanism of immune prognosis, there are many differences in the factors that affect OS and PFS which bear resemblance to the corrective effect of PFSRS on OSRS in high-risk patients. In short, these factors are worth exploring in future research.
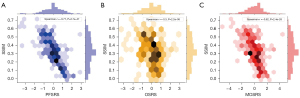
Discussion
As immunotherapy plays an increasingly crucial role in the field of cancer treatment, CT image analysis based on deep learning technique can screen out patients who will benefit from immunotherapy (32-35). In our study, 236 patients who received ICI treatment were divided into a modelling cohort (n=164), validation cohort (n=24), and test cohort (n=48), and their 3D tumor images were extracted by manual segmentation. We first used patient follow-up information to directly construct a survival network for modelling and obtain the OS risk vector (macro-accuracy of training cohort: 77.4%, macro-accuracy of test cohort: 83.3%) and PFS risk vector (macro-accuracy of training cohort: 81.6%, macro-accuracy of test cohort: 77.5%) that could classify patient endpoint time. These risk vectors were fused through the Cox regression model to get OSRS (training cohort log-rank P<0.001; test cohort log-rank, P=0.014) and PFSRS (training cohort log-rank P<0.001; test cohort log-rank P<0.001) with significant risk stratification performance. In the meantime, we used OSRS combined with PFSRS to optimize patient risk and obtain MOSRS. MOSRS demonstrated superiority to OSRS in both the training (log-rank P<0.001) and test (log-rank P=0.002) cohorts. Finally, we constructed a dual-task network with PR and PD which showed significant risk stratification ability in the pre-experiment to obtain PRS and PDS capable of predicting the patient's optimal immune efficacy. Both PRS and PDS showed excellent performance in predicting the optimal immune response in patients. However, when performing risk stratification, PRS could not significantly stratify patients in OS (log-rank P=0.441), while PDS could significantly stratify both (log-rank P<0.001).
PFS and OS follow-up information potentially contains the short-term and long-term response of tumors to immunotherapy. We innovatively combined OSRS and PFSRS to obtain MOSRS in order to make full use of patient prognosis information. MOSRS was better than OSRS in C-index, log-rank test, and other indicators. Based on these results, we speculated that the mechanism of immunotherapy was complicated, and the early response of tumors to immunotherapy was crucial in predicting patient OS. In addition, we used bar graphs and line graphs to display the MOSRS, PFSRS, and MOSRS of each patient. The bar graph showed that the MOSRS of the patient with the shortest survival time (16 days) was obviously greater than that of other high-risk patients, while the line graph showed that the ability of PFSRS to correct MOSRS was mainly reflected in the middle-high-risk patients.
Mounting evidence suggests the role of radiomics in the evaluation of immune response of patients, illustrating its importance in predicting the efficacy of immunotherapy. A previous study has employed deep learning technology combined with prognostic factors to indirectly predict immune response (32). Clinically, the validation of stable, accurate, and more targeted prediction methods represent, nowadays, an unmet need. CT, which provides easy-to-obtain and noninvasive medical data, combined with deep learning technology is one of the better choices to fill this demand.
To the best of our knowledge, this is the first study to directly build a bridge between deep learning and prognostic information. In other words, MOSRS does not rely on any factors with predictive performance. In the preliminary experiment, we used a survival network to directly train the network and did not obtain acceptable results in either the training cohort (C-index =0.60) or test cohort (C-index =0.59). The loss of the network declined but not in exchange for an improvement of C-index. We speculated that the underlying reason for this was that the total number of patients in the study of immune efficacy was small, and there were fewer patients with endpoints.
In addition, in the multivariate analysis of clinical characteristics, we found that no clinical variables were significant using MOSRS. Further, we used TMB radiomic biomarker (TMBRB) with TMB classification and prognostic stratification capabilities from our previous study to compare with MOSRS (33). TMBRB (cut-off point =0.61; log-rank P=0.023) showed a stratification effect lower than that of MOSRS (log-rank P<0.001), and the multivariable P value of TMBRB was 0.73. These results are sufficient to prove the powerful potential of MOSRS as an independent prognostic factor.
In order to prove performance reliability of the method, we selected 4 patients with distinctive prognostic information and output the areas deemed important by the network through the visualization method. Although we selected different types of prognostic information as the target of our network training, they all had a similar region. Regarding the visualization results, we found that whether a dual-task network or survival network was used, the tumor microenvironment played an irreplaceable role in predicting tumor progression and patient OS, which was consistent with the conclusions of previous studies (32-34). Considering that the abundance of CD8 cells was related to immune efficacy, Sun et al. constructed a radiomic signature from CT images of 135 patients in the MOSCATO dataset (34). Three of the 8 features extracted to construct the signature were from the tumor peripheral, and this signature could better assess the patient’s immunophenotype and OS. Trebeschi et al. also used CT combined with radiomics to develop a radiomic biomarker at the level of the lesion (35). They found that this biomarker had good predictive ability and was also related to cell cycle progression and mitosis. In addition, the irregular blood vessels in the tumor microenvironment could lead to uneven tumor growth patterns, which in turn hinders the penetration of T cells (43).
We found that the OSRS and PFSRS regions had differences in high-risk patients, and the structural similarity was negatively correlated with all risk scores. These results indicated that CT images, which provide the macroscopic characterization of multifactorial effects of human immunity, showed that there were different factors affecting immune-related PFS and OS. This also explained why PFSRS had a strong corrective effect on OSRS for high-risk patients when counting the number of incorrect rankings.
Our research had several limitations that should be acknowledged. First, our research involved a single-center retrospective collection of small sample size of Chinese patients. There may have been potential deviations in the survival distribution of patients, and larger multiethnic samples are needed. From the perspective of the loss function, we increased the constraints on sample scores with endpoints and only constrained the scores of negative classes for samples without endpoints. Therefore, room for improvement in the precision of the network remains. However, this this survival network had great value for predicting the efficacy of immunotherapy. In subsequent studies, we will increase the number of patients and integrate more ethnic groups with prospective experiments for verification. For the survival network, we will optimize the selection of the time cutoff point and the loss function to obtain a more accurate survival network for predicting immune efficacy.
Our research has proven that CT image analysis combined with deep learning technology may provide an accurate, noninvasive, and reliable method for evaluating patient response to immunotherapy. Although further investigation of the relationship between immune efficacy and tumor biology is needed, we have found a way to study this matter in depth. Once verification with a larger dataset is provided, the method can be applied clinically.
Conclusions
In conclusion, our research has shown that deep learning can play an important role in predicting the immune efficacy of patients, and the scores obtained by CT images combined with deep learning technology can be effectively correlated with the clinical endpoints of patients treated with ICIs.
Acknowledgments
The authors acknowledge the pulmonary hospital team for their data support and Institute of Automation, Chinese Academy of Sciences (CASIA) for their research resources. The authors appreciate the academic support from the AME Thoracic Surgery Collaborative Group.
Funding: The study was funded by the National Key R&D Program of China (2017YFA0205200), National Natural Science Foundation of China (91959126, 82022036, 91959130, 81971776, 81771924, 6202790004, 81930053, 9195910169), Beijing Natural Science Foundation (L182061), Strategic Priority Research Program of Chinese Academy of Sciences (XDB38040200), Chinese Academy of Sciences (GJJSTD20170004, QYZDJ-SSW-JSC005), Shanghai Municipal Health Commission (2018ZHYL0102), Tongji University AI Program (22120190216), Youth Innovation Promotion Association CAS (2017175), and China Postdoctoral Science Foundation (2021M700341).
Footnote
Reporting Checklist: The authors have completed the TRIPOD reporting checklist. Available at https://tlcr.amegroups.com/article/view/10.21037/tlcr-22-244/rc
Data Sharing Statement: Available at https://tlcr.amegroups.com/article/view/10.21037/tlcr-22-244/dss
Conflicts of Interest: All authors have completed the ICMJE uniform disclosure form (available at https://tlcr.amegroups.com/article/view/10.21037/tlcr-22-244/coif). SW reports the payment or honoraria for lectures, presentations, speakers bureaus, manuscript writing or educational events from Eli Lilly, Pfizer, Novartis Pharma, AstraZeneca, Chugai Pharma, Bristol-Myers, Boehringer Ingelheim, MSD, Ono Pharmaceutical, Daiichi Sankyo, Taiho Pharmaceutical. The other authors have no conflicts of interest to declare.
Ethical Statement: The authors are accountable for all aspects of the work in ensuring that questions related to the accuracy or integrity of any part of the work are appropriately investigated and resolved. The study was conducted in accordance with the Declaration of Helsinki (as revised in 2013) and was approved by the ethics committee of Shanghai Pulmonary Hospital (L20-333-1). Informed consent was waived considering the retrospective nature of this study.
Open Access Statement: This is an Open Access article distributed in accordance with the Creative Commons Attribution-NonCommercial-NoDerivs 4.0 International License (CC BY-NC-ND 4.0), which permits the non-commercial replication and distribution of the article with the strict proviso that no changes or edits are made and the original work is properly cited (including links to both the formal publication through the relevant DOI and the license). See: https://creativecommons.org/licenses/by-nc-nd/4.0/.
References
- Ribas A, Wolchok JD. Cancer immunotherapy using checkpoint blockade. Science 2018;359:1350-5. [Crossref] [PubMed]
- Okazaki T, Chikuma S, Iwai Y, et al. A rheostat for immune responses: the unique properties of PD-1 and their advantages for clinical application. Nat Immunol 2013;14:1212-8. [Crossref] [PubMed]
- Kim ES, Herbst RS, Wistuba II, et al. The BATTLE trial: personalizing therapy for lung cancer. Cancer Discov 2011;1:44-53. [Crossref] [PubMed]
- Kwak EL, Bang YJ, Camidge DR, et al. Anaplastic lymphoma kinase inhibition in non-small-cell lung cancer. N Engl J Med 2010;363:1693-703. [Crossref] [PubMed]
- Borghaei H, Paz-Ares L, Horn L, et al. Nivolumab versus Docetaxel in Advanced Nonsquamous Non-Small-Cell Lung Cancer. N Engl J Med 2015;373:1627-39. [Crossref] [PubMed]
- Brahmer J, Reckamp KL, Baas P, et al. Nivolumab versus Docetaxel in Advanced Squamous-Cell Non-Small-Cell Lung Cancer. N Engl J Med 2015;373:123-35. [Crossref] [PubMed]
- Herbst RS, Baas P, Kim DW, et al. Pembrolizumab versus docetaxel for previously treated, PD-L1-positive, advanced non-small-cell lung cancer (KEYNOTE-010): a randomised controlled trial. Lancet 2016;387:1540-50. [Crossref] [PubMed]
- Reck M, Rodríguez-Abreu D, Robinson AG, et al. Pembrolizumab versus Chemotherapy for PD-L1-Positive Non-Small-Cell Lung Cancer. N Engl J Med 2016;375:1823-33. [Crossref] [PubMed]
- Rizvi H, Sanchez-Vega F, La K, et al. Molecular Determinants of Response to Anti-Programmed Cell Death (PD)-1 and Anti-Programmed Death-Ligand 1 (PD-L1) Blockade in Patients With Non-Small-Cell Lung Cancer Profiled With Targeted Next-Generation Sequencing. J Clin Oncol 2018;36:633-41. [Crossref] [PubMed]
- High TMB Predicts Immunotherapy Benefit. Cancer Discov 2018;8:668. [PubMed]
- Goodman AM, Piccioni D, Kato S, et al. Prevalence of PDL1 Amplification and Preliminary Response to Immune Checkpoint Blockade in Solid Tumors. JAMA Oncol 2018;4:1237-44. [Crossref] [PubMed]
- Ma W, Gilligan BM, Yuan J, et al. Current status and perspectives in translational biomarker research for PD-1/PD-L1 immune checkpoint blockade therapy. J Hematol Oncol 2016;9:47. [Crossref] [PubMed]
- Meng X, Huang Z, Teng F, et al. Predictive biomarkers in PD-1/PD-L1 checkpoint blockade immunotherapy. Cancer Treat Rev 2015;41:868-76. [Crossref] [PubMed]
- Kerr KM, Tsao MS, Nicholson AG, et al. Programmed Death-Ligand 1 Immunohistochemistry in Lung Cancer: In what state is this art? J Thorac Oncol 2015;10:985-9. [Crossref] [PubMed]
- Topalian SL, Hodi FS, Brahmer JR, et al. Safety, activity, and immune correlates of anti-PD-1 antibody in cancer. N Engl J Med 2012;366:2443-54. [Crossref] [PubMed]
- Herbst RS, Soria JC, Kowanetz M, et al. Predictive correlates of response to the anti-PD-L1 antibody MPDL3280A in cancer patients. Nature 2014;515:563-7. [Crossref] [PubMed]
- Ayers M, Lunceford J, Nebozhyn M, et al. IFN-γ-related mRNA profile predicts clinical response to PD-1 blockade. J Clin Invest 2017;127:2930-40. [Crossref] [PubMed]
- Borghaei H, Gettinger S, Vokes EE, et al. Five-Year Outcomes From the Randomized, Phase III Trials CheckMate 017 and 057: Nivolumab Versus Docetaxel in Previously Treated Non-Small-Cell Lung Cancer. J Clin Oncol 2021;39:723-33. [Crossref] [PubMed]
- Rittmeyer A, Barlesi F, Waterkamp D, et al. Atezolizumab versus docetaxel in patients with previously treated non-small-cell lung cancer (OAK): a phase 3, open-label, multicentre randomised controlled trial. Lancet 2017;389:255-65. [Crossref] [PubMed]
- Kumar V, Gu Y, Basu S, et al. Radiomics: the process and the challenges. Magn Reson Imaging 2012;30:1234-48. [Crossref] [PubMed]
- Parekh VS, Jacobs MA. Deep learning and radiomics in precision medicine. Expert Rev Precis Med Drug Dev 2019;4:59-72. [Crossref] [PubMed]
- Ardila D, Kiraly AP, Bharadwaj S, et al. End-to-end lung cancer screening with three-dimensional deep learning on low-dose chest computed tomography. Nat Med 2019;25:954-61. [Crossref] [PubMed]
- Jiang Y, Chen C, Xie J, et al. Radiomics signature of computed tomography imaging for prediction of survival and chemotherapeutic benefits in gastric cancer. EBioMedicine 2018;36:171-82. [Crossref] [PubMed]
- Liu Z, Wang S, Dong D, et al. The Applications of Radiomics in Precision Diagnosis and Treatment of Oncology: Opportunities and Challenges. Theranostics 2019;9:1303-22. [Crossref] [PubMed]
- Li H, Zhu Y, Burnside ES, et al. MR Imaging Radiomics Signatures for Predicting the Risk of Breast Cancer Recurrence as Given by Research Versions of MammaPrint, Oncotype DX, and PAM50 Gene Assays. Radiology 2016;281:382-91. [Crossref] [PubMed]
- Coudray N, Ocampo PS, Sakellaropoulos T, et al. Classification and mutation prediction from non-small cell lung cancer histopathology images using deep learning. Nat Med 2018;24:1559-67. [Crossref] [PubMed]
- Dong D, Fang MJ, Tang L, et al. Deep learning radiomic nomogram can predict the number of lymph node metastasis in locally advanced gastric cancer: an international multicenter study. Ann Oncol 2020;31:912-20. [Crossref] [PubMed]
- Zhang L, Dong D, Zhang W, et al. A deep learning risk prediction model for overall survival in patients with gastric cancer: A multicenter study. Radiother Oncol 2020;150:73-80. [Crossref] [PubMed]
- Song J, Shi J, Dong D, et al. A New Approach to Predict Progression-free Survival in Stage IV EGFR-mutant NSCLC Patients with EGFR-TKI Therapy. Clin Cancer Res 2018;24:3583-92. [Crossref] [PubMed]
- Peng H, Dong D, Fang MJ, et al. Prognostic Value of Deep Learning PET/CT-Based Radiomics: Potential Role for Future Individual Induction Chemotherapy in Advanced Nasopharyngeal Carcinoma. Clin Cancer Res 2019;25:4271-9. [Crossref] [PubMed]
- Khorrami M, Prasanna P, Gupta A, et al. Changes in CT Radiomic Features Associated with Lymphocyte Distribution Predict Overall Survival and Response to Immunotherapy in Non-Small Cell Lung Cancer. Cancer Immunol Res 2020;8:108-19. [Crossref] [PubMed]
- Mu W, Tunali I, Gray JE, et al. Radiomics of 18F-FDG PET/CT images predicts clinical benefit of advanced NSCLC patients to checkpoint blockade immunotherapy. Eur J Nucl Med Mol Imaging 2020;47:1168-82. [Crossref] [PubMed]
- He B, Dong D, She Y, et al. Predicting response to immunotherapy in advanced non-small-cell lung cancer using tumor mutational burden radiomic biomarker. J Immunother Cancer 2020;8:e000550. [Crossref] [PubMed]
- Sun R, Limkin EJ, Vakalopoulou M, et al. A radiomics approach to assess tumour-infiltrating CD8 cells and response to anti-PD-1 or anti-PD-L1 immunotherapy: an imaging biomarker, retrospective multicohort study. Lancet Oncol 2018;19:1180-91. [Crossref] [PubMed]
- Trebeschi S, Drago SG, Birkbak NJ, et al. Predicting response to cancer immunotherapy using noninvasive radiomic biomarkers. Ann Oncol 2019;30:998-1004. [Crossref] [PubMed]
- Eisenhauer EA, Therasse P, Bogaerts J, et al. New response evaluation criteria in solid tumours: revised RECIST guideline (version 1.1). Eur J Cancer 2009;45:228-47. [Crossref] [PubMed]
- Xie Y, Xia Y, Zhang J, et al. Knowledge-based Collaborative Deep Learning for Benign-Malignant Lung Nodule Classification on Chest CT. IEEE Trans Med Imaging 2019;38:991-1004. [Crossref] [PubMed]
- Katzman JL, Shaham U, Cloninger A, et al. DeepSurv: personalized treatment recommender system using a Cox proportional hazards deep neural network. BMC Med Res Methodol 2018;18:24. [Crossref] [PubMed]
- Lee C, Zame WR, Yoon J, et al. DeepHit: A Deep Learning Approach to Survival Analysis With Competing Risks. Proc Conf AAAI Artif Intell 2018;8:
-
Ruder S. An overview of multi-task learning in deep neural networks. (2017 ). Available online: https://arxiv.org/abs/1706.05098 - Camp RL, Dolled-Filhart M, Rimm DL. X-tile: a new bio-informatics tool for biomarker assessment and outcome-based cut-point optimization. Clin Cancer Res 2004;10:7252-9. [Crossref] [PubMed]
- Selvaraju RR, Cogswell M, Das A, et al. Grad-cam: Visual explanations from deep networks via gradient-based localization. Proceedings of the IEEE international conference on computer vision; 2017:618-26.
- Huang Y, Goel S, Duda DG, et al. Vascular normalization as an emerging strategy to enhance cancer immunotherapy. Cancer Res 2013;73:2943-8. [Crossref] [PubMed]