Systematic analysis of ferroptosis-related long non-coding RNA predicting prognosis in patients with lung squamous cell carcinoma
Introduction
Lung cancer is one of the most common cancers globally and has the highest mortality rate (1,2), with about 2 million new cases and 1.76 million deaths per year (3). The disease is broadly divided into non-small cell lung cancer (NSCLC) (85% of total diagnoses) and small cell lung cancer (SCLC) (15% of total diagnoses) (4), and among the former, the most common subtype is adenocarcinoma, followed by lung squamous cell carcinoma (LUSC). LUSC accounts for approximately 40% of all lung cancers, and most patients present early with clinical symptoms and regional metastases at the time of pathological confirmation (5). LUSC is more common in smokers and men compared to other histological types (6), although its incidence has now declined dramatically, partly due to a decline in smoking rates. Advances in diagnosis and treatment have been made in recent years, but the 5-year overall survival (OS) rate for LUSC remains low. Cancer patients with low OS usually have specific markers, that is, markers with cancer susceptibility and risk, that are at high risk of cancer and are expected to benefit from prevention studies (7). To date, various studies have reported on potential prognostic markers for LUSC. CCNA2, AURKA, AURKB, MUC22, and KLK6 were found to be associated with poor prognosis in LUSC and was associated with immune cell infiltration (8,9). However, these studies have only understood the involvement of biomarkers in influencing the immune microenvironment and have not fully explored the underlying mechanisms of biomarkers. Therefore, it is particularly important to find a comprehensive and effective prognostic marker for the disease.
Ferroptosis is a novel type of cell death, which unlike autophagy and apoptosis, is an iron- and reactive oxygen species (ROS)-dependent cell death (10). These cellular abnormalities are due to membrane lipid peroxidation and oxidative stress resulting in the loss of selective permeability of the plasma membrane (11). Compared to normal cells, cancer cells exhibit iron addiction (12), and in contrast to the abnormal iron metabolism in cancer, ferroptosis appears to be an antitumor process (13,14). In recent years, ferroptosis inducers are expected to be potential therapeutic options to induce cancer cell death, especially for malignancies resistant to radiotherapy and chemotherapy (15,16). Increasing evidence indicates long non-coding RNAs (lncRNAs) play a crucial regulatory role in the occurrence and development of cancer, as they can regulate the proliferation, invasion, and metastasis of cancer cells and metabolic reprogramming (17). A few reports have suggested lncRNAs are involved in ferroptosis. Ferroptosis-related lncRNAs have been reported to be associated with prognosis of pancreatic, colon, and liver cancers (18-20). It was also been reported in lung adenocarcinoma that ferroptosis-related lncRNAs affect patient prognosis (21). However, no corresponding studies have been reported in LUSC.
This study systematically analyzed the association between ferroptosis-related lncRNAs expression and clinicopathological features of LUSC patients in The Cancer Genome Atlas (TCGA) database. We also constructed prognostic signatures based on 29 ferroptosis-related lncRNAs and assessed their ability to independently and accurately predict factors for LUSC. This study provides new insights into the underlying mechanisms of LUSC and the identification of 29 ferroptosis-related lncRNAs could serve as diagnostic and prognostic biomarkers. We present the following article in accordance with the TRIPOD reporting checklist (available at https://tlcr.amegroups.com/article/view/10.21037/tlcr-22-224/rc).
Methods
The flow chart of systematic bioinformatics analysis in this study was showed in Figure 1. The study was conducted in accordance with the Declaration of Helsinki (as revised in 2013).
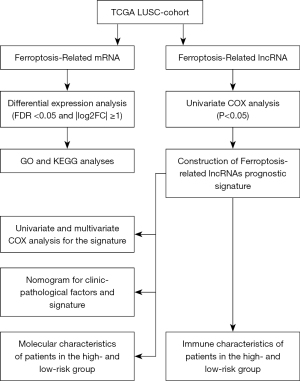
Data acquisition
Gene expression and clinical data on LUSC patients were obtained from TCGA database (https://portal.gdc.cancer.gov/repository) and analyzed in R language (R version 4.1.2). Gene expression profiles were normalized, and patients with a clear pathological diagnosis, gene expression, and clinical data were included, resulting in a final total of 504 samples. Clinical data collected from the 504 LUSC patients included clinicopathological stage, gender, age, pathologic stage, smoking, survival status, and survival time, and their baseline characteristics are summarized in Table 1. Ethics approval was not required, as TCGA is a public database.
Table 1
Characteristics | Levels | Overall |
---|---|---|
n | 504 | |
Gender, n (%) | Female | 131 (26.0) |
Male | 373 (74.0) | |
T, n (%) | T1 | 50 (9.9) |
T1a | 24 (4.8) | |
T1b | 40 (7.9) | |
T2 | 174 (34.5) | |
T2a | 87 (17.3) | |
T2b | 34 (6.7) | |
T3 | 71 (14.1) | |
T4 | 24 (4.8) | |
M, n (%) | M0 | 414 (82.1) |
M1 | 5 (1.0) | |
M1a | 1 (0.2) | |
M1b | 1 (0.2) | |
MX | 79 (15.7) | |
Unknown | 4 (0.8) | |
N, n (%) | N0 | 320 (63.5) |
N1 | 133 (26.4) | |
N2 | 40 (7.9) | |
N3 | 5 (1.0) | |
NX | 6 (1.2) | |
Age (years), median [IQR] | 68 [62, 73] |
TCGA, The Cancer Genome Atlas; IQR, interquartile range.
Identification of ferroptosis-related lncRNAs
To search for ferroptosis-related genes in LUSC, gene expression profiles from TCGA database and ferroptosis-related genes from the FerrDb website (11) were combined. Finally, 382 ferroptosis-related genes were identified in LUSC. Pearson correlation analysis was used to search for lncRNAs associated with ferroptosis-related genes, and the screening criteria were correlation coefficient |R2|>0.4 and P<0.001. Significant differential expression of ferroptosis-related lncRNAs was set at false discovery rate (FDR) <0.05 and |log2FC| ≥1 by using the “limma” R package, and the biological function of ferroptosis-related differentially expressed genes (DEGs) was explored using Gene Ontology (GO) and Kyoto Encyclopedia of Genes and Genomes (KEGG) analysis.
Construction and evaluation of the prognostic signature
Univariate Cox regression models were used to identify the most relevant ferroptosis-related lncRNAs for OS in LUSC patients (P<0.05), and 29 target ferroptosis-associated lncRNAs were identified as candidates for a prognostic signature model (Figure S1). We stratified characteristic lncRNAs using the following formula to compute the risk score (22): risk score = (coefficient lncRNA1 × expression of lncRNA1) + (coefficient lncRNA2 × expression of lncRNA2) + … + (coefficient lncRNAn × expression lncRNA). The associated risk score for each LUSC patient was also evaluated and patients were divided into high-risk and low-risk groups using the median risk score as the cut-off point.
Receiver operating characteristic (ROC) analysis (“survival ROC” R package) and decision curve analysis (DCA) were then used to assess the accuracy and clinical applicability of the prognostic risk model (23). Univariate analysis was performed on risk model and clinicopathological parameters to determine that age, stage, and risk model were associated with prognosis. Next, multifactorial Cox regression analysis of these three factors was performed and all were found to be associated with prognosis. The nomogram was used to further determine whether the prognostic risk model was an independent prognostic factor for LUSC patients.
Bioinformatic analysis
The Tumor Immune Estimation Resource (TIMER) (24), CIBERSORT (25), CIBERSORT-ABS (26), QUANTISEQ (27), Microenvironment Cell Populations-counter (MCP-counter) (28), XCELL (29), and EPIC (30) algorithms were applied to assess the infiltration of various immune cells between high-and low-risk groups based on the ferroptosis-related lncRNAs prognostic signature. The differences in immune cell infiltration under different algorithms were displayed in a Heatmap, and single-sample gene set enrichment analysis (ssGSEA) was used to quantify immune cell subpopulations and related functions between the two groups (31). The potential immune checkpoint was also displayed.
We used the gene set enrichment analysis (GSEA) method based on the KEGG and HALLMARK gene sets with the “clusterProfiler” R package (P<0.05 and FDR <0.25) to determine in which signalling pathways the differentially expressed genes were involved. The gene mutation analysis obtained information on genetic alterations from the cBioPortal database (http://www.cbioportal.org/) and the quantity and quality of gene mutations were analyzed in the two groups by using the “Maftools” R package (Mutation Annotation Formatted files).
Statistical analysis
Spearman correlation analysis was used to analyze the correlation between ferroptosis-related genes and ferroptosis-related lncRNAs, and chi-square test was used to analyze the difference in the proportion of clinical features. Wilcox test was used to compare the proportion of tumor-infiltrating immune cells. The OS between different groups was plotted using the Kaplan-Meier product-limit method, with P values based on the log-rank test. Univariable and multifactor Cox regression analyses were implemented to identify its independent predictors. All P values were based on a two-sided statistical test, and P<0.05 was considered statistically significant.
Results
Functional enrichment analysis of ferroptosis-related DEGs
Combining univariate and multifactorial analyses, we identified 29 ferroptosis-related lncRNAs associated with prognosis. These were evaluated using GO and KEGG analysis to understand the potential biological pathways of ferroptosis-related DEGs (Figure 2). Biological processes were mainly involved in the production of oxidative stress, metabolism, and homeostasis of superoxide, while molecular functions were implicated in influencing apical and basal plasma membrane functions and nicotinamide adenine dinucleotide phosphate (NADPH) oxidase. Cellular components were mainly up-regulated in the NADPH oxidoreductase complex and iron ion binding. KEGG analysis showed that these DEGs were mainly involved in ferroptosis, microRNAs in cancer, the hypoxia-inducible factor-1 (HIF-1) signaling pathway, the NOD-like receptor (NLR) signaling pathway, and energy metabolism.
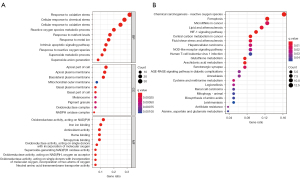
Identification of prognostically significant ferroptosis-related lncRNAs
Based on the ferroptosis-related lncRNAs obtained in the previous period, we calculated risk scores and constructed prognostic signatures to predict the survival of 504 enrolled LUSC patients. As shown in Figure 3A, compared with low-risk patients, high-risk patients had significantly poorer OS (P<0.001). The time-dependent ROC curve demonstrated the area under the curve (AUC) value for the ferroptosis-related lncRNAs prognostic signature was 0.658, which had superior performance than the AUC values for age (AUC =0.536), gender (AUC =0.496), and stage (AUC =0.562) in predicting the prognosis of LUSC (Figure 3B). Patients were then ranked according to the risk scores based on the ferroptosis-related lncRNAs prognosis signature (Figure 3C, upper), and the scatter plot showed the survival rate correlated with the risk scores, where patients with higher risk scores had shorter survival times (Figure 3C, middle). Interestingly, the heatmap suggested that most characteristic lncRNAs were negatively correlated with our risk model, so more studies are needed to explore the reasons for this (Figure 3C, bottom). The time-dependent ROC curve showed the AUC of characteristic lncRNAs for 1-, 2-, and 3-year survival were 0.658, 0.693, and 0.687, respectively (Figure 3D). DCA graphically illustrated that ferroptosis-related lncRNAs prognostic signature brought more net benefit of survival than other parameters (Figure 3E).
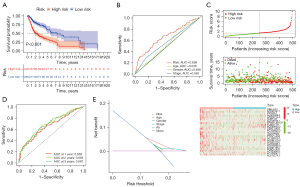
Description of the ferroptosis-related lncRNAs as an independent prognostic factor
Multivariate Cox regression analysis was then performed to determine whether the ferroptosis-related lncRNAs prognostic signature was an independent prognostic factor for LUSC. Univariate analysis showed that age (P<0.05), stage (P<0.05), and the signature risk score (P<0.001) were associated with OS, in which the risk score was statistically significant, and this was confirmed by multivariate analyses (Figure 4). The heatmap for the association between the ferroptosis-related lncRNAs prognostic signature and clinicopathological features was also analyzed, as shown in Figure 5. A nomogram was then constructed by using the signature and traditional clinicopathological features, including gender, age, and stage (Figure 6), and the results demonstrated the nomogram using the signature risk score was reliable and accurate. The above results indicated that the ferroptosis-related lncRNAs signature could be identified as an independent prognostic factor for LUSC patients.
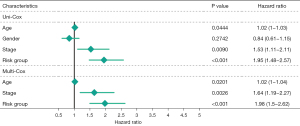
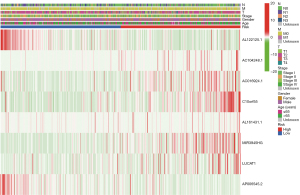
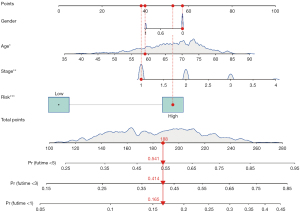
Molecular characteristics of patients in the high- and low-risk group
We performed GSEA to determine the gene sets enriched between the high- and low-risk group. In the high-risk group, the enriched gene sets included angiogenesis, apical surface, cholesterol homeostasis, hedgehog signaling, and TGF-β signaling (Figure 7A), where DNA repair, E2F targets, G2M checkpoint, and MYC targets were enriched in the low-risk group (Figure 7B) (P<0.05, FDR <0.29).
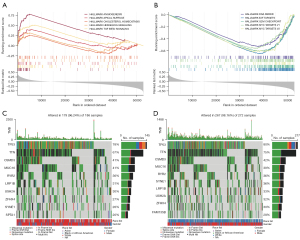
Gene mutations were then analyzed to better understand the biology of the characteristic lncRNAs. The top 10 genes were identified with the highest mutation rate in two separate groups (Figure 7C,7D) and showed significantly higher mutation counts in the high-risk group than in the low-risk group. The mutation rate of each gene was more than 20% in both the high- and low-risk group. Missense variations were the frequent mutation type, followed by nonsense, and frameshift deletions. Despite the difference in mutation rate, the mutated genes were approximately the same in both groups.
Ferroptosis-related lncRNAs and tumor immune microenvironment (TIME)
Figure 2B shows the pathways associated with ferroptosis-related DEGs using KEGG analysis. Of interest is the HIF-1 pathway, which is closely associated with TIME. To further explore the correlation between the ferroptosis-related lncRNAs and TIME, different algorithms were used to determine the proportion of tumor-infiltrating immune cells (TIICs), including TIMER, CIBERSORT, CIBERSORT-ABS, QUANTISEQ, MCP-counter, XCELL, and EPIC. The heatmap showed the TIICs in high- and low-risk groups under various algorithms (Figure 8) and revealed differences in the various TIICs in the high- and low- group, such as CD4+ T cell, CD8+ T cell, Neutrophil, macrophage, and myeloid dendritic cells.
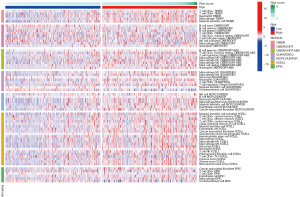
We next used ssGSEA to analyze the correlation between the function of the above immune cell subpopulations and the high- and low-risk group. The result showed significant differences in T cell functions, including antigen presentation process, checkpoint inhibition, cytokine receptor (CCR), T cell activation and inhibition, inflammatory response, and release of types I and II IFN (Figure 9A). The immune checkpoint is closely related to immune cell function. Although there are three popular immune checkpoints at present: PD-1, CTLA-4, and PD-L1, there are still much literature reporting that a variety of immunosuppressive molecules and costimulatory molecules are involved in tumor immune escape. Therefore, we used ssGSEA to look for immune checkpoints significantly associated with the constructed risk model of ferroptosis-related lncRNAs to look for lncRNAs upstream of ferroptosis and immune response. We found significant differences in immune checkpoint molecules between the two groups (P<0.001), including the classic PDCD-1 (PD-1), CD276, ICOS, and CTLA4 (Figure 9B).
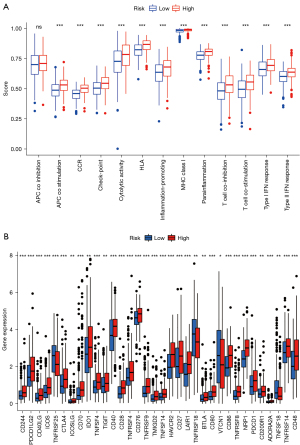
Ferroptosis-related lncRNAs and m6A methylation
Various data have shown that lncRNA is closely related to methylation in the occurrence and development of cancer. Through analysis, we found m6A-related mRNA expression was significantly different between the high- and low-risk groups, including YTHDF1, YTHDC1, HNRNPC, YTHDC2, METTL3, ALKBH5, and FTO (Figure 10). This provides a new idea for studying methylation and ferroptosis in LUSC.
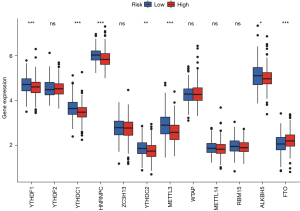
Discussion
Resistance to radiotherapy and chemotherapy remains the key challenge facing cancer treatment today, and ferroptosis appears to hold potential for reversing this process. As lncRNA has been found to participate in the ferroptosis process of tumor cells, targeting ferroptosis-associated lncRNAs could benefit patients whose cancer progresses after treatment. In this study, we systematically investigated the association between the expression of 29 ferroptosis-associated lncRNAs and prognosis in LUSC tumor tissues and constructed and verified a new prognostic model integrating them differently.
Firstly, through GO and KEGG analysis, it was found that ferroptosis-associated lncRNAs were not only enriched in the ferroptosis process, oxidative stress, and lipid metabolism-related pathways, but were related to the HIF-1 signaling pathway, microRNAs in cancer, central carbon metabolism in cancer, and NLR signaling pathway, which are involved in the development of cancer. microRNA-9 has been reported to regulate ferroptosis in melanoma by targeting glutamic oxaloacetate transaminase (32), and HIF-1 and central carbon metabolism were associated with non-tumorigenic ferroptosis (33,34). Although there is no direct evidence for the relationship between NLR and ferroptosis, it has been documented that high intracellular ROS levels can activate the NLR pathway, which in turn promotes cellular scorching (35). This may provide a possible theoretical basis for another mode of cell death, ferroptosis.
A total of 29 ferroptosis-related lncRNAs with prognostic significance were identified in this study. MYOSLID modulates epithelial-mesenchymal transition in head and neck squamous cell carcinoma to promote invasion and metastasis (36), and stress-induced LASTR promotes breast cancer metastasis by regulating the activity of U4/U6 recycling factor SART3 (37). MIR22HG can regulate the miR-486/PTEN axis to promote cell proliferation in bladder cancer (38), while LUCAT1 promotes colorectal cancer tumorigenesis by targeting the ribosomal protein L40-MDM2-p53 pathway by binding UBA52 (39). Although some of the 29 ferroptosis-related lncRNAs have not been studied, it can be hypothesized that they promote tumor progression based on the above studies, which is consistent with our conclusions (Figure 3A). There are no studies on the role of ferroptosis-related lncRNAs in the prognosis of LUSC patients, and our findings may provide new ideas for exploring the mechanism of the disease.
Risk scores were calculated for each LUSC patient based on the expression of 29 ferroptosis-related lncRNAs in the prognostic signatures, and patients were divided into high- and low-risk groups based on the median risk score. The survival time of those with high-risk scores was shorter compared with low-risk scores. ROC and DCA curve analysis validated the prognostic accuracy of ferroptosis-related lncRNAs prognostic signatures in LUSC patients, and the prognostic signatures risk scores were an independent prognostic factor in univariate and multifactor Cox regression analyses. A nomogram showed risk scores for ferroptosis-related lncRNAs prognostic signatures were more predictive of prognosis in LUSC patients compared with clinicopathological parameters (gender, age, and stage), and calibration plots showed the predicted 1-, 3-, and 5-year survival rates were similar according to the column line plots. Overall, in our study, ferroptosis-related lncRNAs prognostic signatures independently and accurately predicted survival outcomes in LUSC patients.
By performing GSEA on the high- and low-risk groups, we found the high-risk group was enriched in angiogenesis, TGF-β, and hedgehog signalling pathways, while the low-risk group tended to be enriched in cell cycle signalling pathways. Targeting ATF4 has been reported to inhibit tumor angiogenesis and promote ferroptosis in glioma cells (40), and in hepatocellular carcinoma, TGF-β inhibited System Xc− suppressing ferroptosis (41). Although no clear reports show the association of the hedgehog pathway with ferroptosis, there is potential to tap the former in ferroptosis based on its ability to promote lipid peroxidation as well as maintain the stemness characteristics of cancer cells (42). TP53 is an important known oncogene that can regulate apoptosis and cell cycle and inhibit tumor development by regulating ferroptosis (43). We identified high-frequency mutations in TP53 in both groups at the time of mutation prediction, which suggests that in addition to activating glutathione peroxidase 4 (GPX4), which is a key protein of the classical ferroptosis pathway, ferroptosis can also be driven through the TP53 pathway.
Immune escape is the main cause of failure in tumor immunotherapy. Therefore, restoring or enhancing immune cells in recognition of tumor cells and killing capability is the main direction of current immunization therapy and research. A previous study has shown that the ferroptosis-specific lipid peroxidation level is significantly increased after anti-PD-L1 treatment, while the sensitivity of tumor cells to immunotherapy is significantly reduced after blocking the ferroptosis pathway (44). In this study, TIICs that differed in high- and low-risk groups were found by different immune cell infiltration analysis methods, and differences in immune cell subpopulations and corresponding functions in the two groups were analyzed using ssGSEA. We also found the most relevant immune checkpoints for ferroptosis-related lncRNAs by ssGSEA, including the classic PDCD-1 (PD-1), CD276, ICOS, and CTLA4. This suggests combining ferroptosis inducers with immune checkpoint inhibitors could be a boon for LUSC patients with poor immunotherapy results.
Since methylation is also associated with the development of ferroptosis in tumor cells (45), this study also explored the differential methylation-related genes in the high and low groups, and a total of seven genes were identified, including YTHDF1, YTHDC1, HNRNPC, YTHDC2, METTL3, ALKBH5, and FTO. This provides new ideas for the exploration of the ferroptosis mechanism in LUSC.
Our study has several limitations. First, our findings were not validated through other independent cohorts to further determine the stability and accuracy of the prognostic model. Second, our study was based on a retrospective study from the publicly available TCGA database and was not conducted in a prospective cohort study with a clinical sample to determine clinical applicability. Finally, further biochemical experiments, such as immunohistochemistry, quantitative real-time fluorescence, quantitative PCR and flow cytometry, and clinical data analysis, are needed to confirm our findings. In a word, we investigated the potential mechanisms of LUSC development from the perspective of ferroptosis-related lncRNAs, providing new insights into LUSC research, and identified 29 lncRNAs as biomarkers to predict the prognosis of LUSC patients.
Acknowledgments
The authors appreciate the academic support from the AME Lung Cancer Collaborative Group.
Funding: This work was supported by grants from the National Natural Scientific Funding (81702608) to NSJ and the Science and Technology Project of Nantong (MSZ20207) to YNH.
Footnote
Reporting Checklist: The authors have completed the TRIPOD reporting checklist. Available at https://tlcr.amegroups.com/article/view/10.21037/tlcr-22-224/rc
Conflicts of Interest: All authors have completed the ICMJE uniform disclosure form (available at https://tlcr.amegroups.com/article/view/10.21037/tlcr-22-224/coif). Sujie Ni serves as an unpaid editorial board member of Translational Lung Cancer Research from June 2017 to June 2022. The other authors have no conflicts of interest to declare.
Ethical Statement: The authors are accountable for all aspects of the work in ensuring that questions related to the accuracy or integrity of any part of the work are appropriately investigated and resolved. The study was conducted in accordance with the Declaration of Helsinki (as revised in 2013).
Open Access Statement: This is an Open Access article distributed in accordance with the Creative Commons Attribution-NonCommercial-NoDerivs 4.0 International License (CC BY-NC-ND 4.0), which permits the non-commercial replication and distribution of the article with the strict proviso that no changes or edits are made and the original work is properly cited (including links to both the formal publication through the relevant DOI and the license). See: https://creativecommons.org/licenses/by-nc-nd/4.0/.
References
- Jemal A, Center MM, DeSantis C, et al. Global patterns of cancer incidence and mortality rates and trends. Cancer Epidemiol Biomarkers Prev 2010;19:1893-907. [Crossref] [PubMed]
- Siegel RL, Miller KD, Jemal A. Cancer statistics, 2020. CA Cancer J Clin 2020;70:7-30. [Crossref] [PubMed]
- Howlader N, Forjaz G, Mooradian MJ, et al. The Effect of Advances in Lung-Cancer Treatment on Population Mortality. N Engl J Med 2020;383:640-9. [Crossref] [PubMed]
- Travis WD, Brambilla E, Burke AP, et al. Introduction to The 2015 World Health Organization Classification of Tumors of the Lung, Pleura, Thymus, and Heart. J Thorac Oncol 2015;10:1240-2. [Crossref] [PubMed]
- Herbst RS, Morgensztern D, Boshoff C. The biology and management of non-small cell lung cancer. Nature 2018;553:446-54. [Crossref] [PubMed]
- Auerbach O, Garfinkel L, Parks VR. Histologic type of lung cancer in relation to smoking habits, year of diagnosis and sites of metastases. Chest 1975;67:382-7. [Crossref] [PubMed]
- Kucuk O. Cancer biomarkers. Mol Aspects Med 2015;45:1-2. [Crossref] [PubMed]
- Gao M, Kong W, Huang Z, et al. Identification of Key Genes Related to Lung Squamous Cell Carcinoma Using Bioinformatics Analysis. Int J Mol Sci 2020;21:2994. [Crossref] [PubMed]
- Xu F, Lin H, He P, et al. A TP53-associated gene signature for prediction of prognosis and therapeutic responses in lung squamous cell carcinoma. Oncoimmunology 2020;9:1731943. [Crossref] [PubMed]
- Dixon SJ, Lemberg KM, Lamprecht MR, et al. Ferroptosis: an iron-dependent form of nonapoptotic cell death. Cell 2012;149:1060-72. [Crossref] [PubMed]
- Zhou N, Bao J. FerrDb: a manually curated resource for regulators and markers of ferroptosis and ferroptosis-disease associations. Database (Oxford) 2020;2020:baaa021.
- Torti SV, Manz DH, Paul BT, et al. Iron and Cancer. Annu Rev Nutr 2018;38:97-125. [Crossref] [PubMed]
- Zhang Y, Shi J, Liu X, et al. BAP1 links metabolic regulation of ferroptosis to tumour suppression. Nat Cell Biol 2018;20:1181-92. [Crossref] [PubMed]
- Hassannia B, Vandenabeele P, Vanden Berghe T. Targeting Ferroptosis to Iron Out Cancer. Cancer Cell 2019;35:830-49. [Crossref] [PubMed]
- Sleire L, Skeie BS, Netland IA, et al. Drug repurposing: sulfasalazine sensitizes gliomas to gamma knife radiosurgery by blocking cystine uptake through system Xc-, leading to glutathione depletion. Oncogene 2015;34:5951-9. [Crossref] [PubMed]
- Roh JL, Kim EH, Jang HJ, et al. Induction of ferroptotic cell death for overcoming cisplatin resistance of head and neck cancer. Cancer Lett 2016;381:96-103. [Crossref] [PubMed]
- Huarte M. The emerging role of lncRNAs in cancer. Nat Med 2015;21:1253-61. [Crossref] [PubMed]
- Chen D, Gao W, Zang L, et al. Ferroptosis-Related IncRNAs Are Prognostic Biomarker of Overall Survival in Pancreatic Cancer Patients. Front Cell Dev Biol 2022;10:819724. [Crossref] [PubMed]
- Wu Z, Lu Z, Li L, et al. Identification and Validation of Ferroptosis-Related LncRNA Signatures as a Novel Prognostic Model for Colon Cancer. Front Immunol 2022;12:783362. [Crossref] [PubMed]
- Xu Z, Peng B, Liang Q, et al. Construction of a Ferroptosis-Related Nine-lncRNA Signature for Predicting Prognosis and Immune Response in Hepatocellular Carcinoma. Front Immunol 2021;12:719175. [Crossref] [PubMed]
- Fei X, Hu C, Wang X, et al. Construction of a Ferroptosis-Related Long Non-coding RNA Prognostic Signature and Competing Endogenous RNA Network in Lung Adenocarcinoma. Front Cell Dev Biol 2021;9:751490. [Crossref] [PubMed]
- Fan CN, Ma L, Liu N. Systematic analysis of lncRNA-miRNA-mRNA competing endogenous RNA network identifies four-lncRNA signature as a prognostic biomarker for breast cancer. J Transl Med 2018;16:264. [Crossref] [PubMed]
- Vickers AJ, Elkin EB. Decision curve analysis: a novel method for evaluating prediction models. Med Decis Making 2006;26:565-74. [Crossref] [PubMed]
- Li T, Fan J, Wang B, et al. TIMER: A Web Server for Comprehensive Analysis of Tumor-Infiltrating Immune Cells. Cancer Res 2017;77:e108-10. [Crossref] [PubMed]
- Charoentong P, Finotello F, Angelova M, et al. Pan-cancer Immunogenomic Analyses Reveal Genotype-Immunophenotype Relationships and Predictors of Response to Checkpoint Blockade. Cell Rep 2017;18:248-62. [Crossref] [PubMed]
- Tamminga M, Hiltermann TJN, Schuuring E, et al. Immune microenvironment composition in non-small cell lung cancer and its association with survival. Clin Transl Immunology 2020;9:e1142. [Crossref] [PubMed]
- Finotello F, Mayer C, Plattner C, et al. Molecular and pharmacological modulators of the tumor immune contexture revealed by deconvolution of RNA-seq data. Genome Med 2019;11:34. [Crossref] [PubMed]
- Becht E, Giraldo NA, Lacroix L, et al. Estimating the population abundance of tissue-infiltrating immune and stromal cell populations using gene expression. Genome Biol 2016;17:218. [Crossref] [PubMed]
- Aran D, Hu Z, Butte AJ. xCell: digitally portraying the tissue cellular heterogeneity landscape. Genome Biol 2017;18:220. [Crossref] [PubMed]
- Racle J, de Jonge K, Baumgaertner P, et al. Simultaneous enumeration of cancer and immune cell types from bulk tumor gene expression data. Elife 2017;6:26476. [Crossref] [PubMed]
- Finotello F, Trajanoski Z. Quantifying tumor-infiltrating immune cells from transcriptomics data. Cancer Immunol Immunother 2018;67:1031-40. [Crossref] [PubMed]
- Luo M, Wu L, Zhang K, et al. miR-137 regulates ferroptosis by targeting glutamine transporter SLC1A5 in melanoma. Cell Death Differ 2018;25:1457-72. [Crossref] [PubMed]
- Li X, Zou Y, Xing J, et al. Pretreatment with Roxadustat (FG-4592) Attenuates Folic Acid-Induced Kidney Injury through Antiferroptosis via Akt/GSK-3β/Nrf2 Pathway. Oxid Med Cell Longev 2020;2020:6286984. [Crossref] [PubMed]
- Ryter SW. Heme Oxgenase-1, a Cardinal Modulator of Regulated Cell Death and Inflammation. Cells 2021;10:515. [Crossref] [PubMed]
- Morris G, Walker AJ, Berk M, et al. Cell Death Pathways: a Novel Therapeutic Approach for Neuroscientists. Mol Neurobiol 2018;55:5767-86. [Crossref] [PubMed]
- Xiong HG, Li H, Xiao Y, et al. Long noncoding RNA MYOSLID promotes invasion and metastasis by modulating the partial epithelial-mesenchymal transition program in head and neck squamous cell carcinoma. J Exp Clin Cancer Res 2019;38:278. [Crossref] [PubMed]
- De Troyer L, Zhao P, Pastor T, et al. Stress-induced lncRNA LASTR fosters cancer cell fitness by regulating the activity of the U4/U6 recycling factor SART3. Nucleic Acids Res 2020;48:2502-17. [Crossref] [PubMed]
- Tang Q, Jiang X, Ma S, et al. MIR22HG regulates miR-486/PTEN axis in bladder cancer to promote cell proliferation. Biosci Rep 2020;40:BSR20193991. [Crossref] [PubMed]
- Zhou Q, Hou Z, Zuo S, et al. LUCAT1 promotes colorectal cancer tumorigenesis by targeting the ribosomal protein L40-MDM2-p53 pathway through binding with UBA52. Cancer Sci 2019;110:1194-207. [Crossref] [PubMed]
- Chen D, Fan Z, Rauh M, et al. ATF4 promotes angiogenesis and neuronal cell death and confers ferroptosis in a xCT-dependent manner. Oncogene 2017;36:5593-608. [Crossref] [PubMed]
- Kim DH, Kim WD, Kim SK, et al. TGF-β1-mediated repression of SLC7A11 drives vulnerability to GPX4 inhibition in hepatocellular carcinoma cells. Cell Death Dis 2020;11:406. [Crossref] [PubMed]
- Yang Y, Li X, Wang T, et al. Emerging agents that target signaling pathways in cancer stem cells. J Hematol Oncol 2020;13:60. [Crossref] [PubMed]
- Ou Y, Wang SJ, Li D, et al. Activation of SAT1 engages polyamine metabolism with p53-mediated ferroptotic responses. Proc Natl Acad Sci U S A 2016;113:E6806-12. [Crossref] [PubMed]
- Wang W, Green M, Choi JE, et al. CD8+ T cells regulate tumour ferroptosis during cancer immunotherapy. Nature. 2019;569:270-4. [Crossref] [PubMed]
- Shen M, Li Y, Wang Y, et al. N6-methyladenosine modification regulates ferroptosis through autophagy signaling pathway in hepatic stellate cells. Redox Biol 2021;47:102151. [Crossref] [PubMed]