Single nucleotide polymorphisms in FOXP1 and RORA of the lymphocyte activation-related pathway affect survival of lung cancer patients
Introduction
Lung cancer is a significant public health challenge and the leading cause of cancer-related mortality (1). In 2021, approximately 235,760 cases of lung cancer and 131,880 associated-deaths were reported in the United States (2). Non-small cell lung cancer (NSCLC), as the dominant histological subtype, represents approximately 85% of all lung cancer cases (3). Despite major advances in therapeutic strategies in the past decades, such as minimally invasive surgery, targeted therapy, and immunotherapy, lung cancer survival was improving marginally, with a dismal 5-year survival rate around 20% (4). Despite the same stage, histology, and therapy (5), individual lung cancer patients can experience different survival rates, suggesting that potential genetic variations, such as single nucleotide polymorphisms (SNPs), may be involved (6). Thus, identifying genetic factors in key genes and pathways that might affect cancer progression is an important step in providing patients with an optimized and personalized management and treatment plan.
Solid tumors are not merely an accumulation of neoplastic cells but also include a microenvironment containing immune cells, the extracellular matrix, cytokines, and other macromolecules, which play crucial roles in cancer development, invasion, and metastasis (7). Lymphocytes are an essential group of immune cells capable of infiltrating the tumor microenvironment (8). Lymphocyte activation is part of the host immune response against malignant cells. It may be involved in both immunostimulatory and immunosuppressive effects, with major functions in the control of antitumor immunity (9). Tumor-infiltrating lymphocytes are commonly detected in human malignancies, including lung cancer (10). Thus, targeting lymphocyte activation may be an alternative approach to lung cancer treatment, which could improve the response to immunotherapy and subsequent prognosis. However, to date, there is no standardized quantification of prognostic biomarkers associated with lymphocyte activation. Therefore, it is necessary to explore the associations of biomarkers of lymphocyte activation with patient outcomes in lung cancer.
Genome-wide association studies (GWASs) are considered a powerful approach to genetic analysis of complex diseases and traits, including cancer (11). However, the functions of genetic variants in candidate genes affecting lymphocyte activation pathways in lung cancer tumor development and progression remain unknown. A promising hypothesis-driven approach has been utilized to reanalyze the reported GWAS datasets in the post-GWAS era, which could assess the associations between patient survival and functional SNPs across many genes of a given biomolecular pathway (12). Since there is no report that has explored the association between genetic variants in genes involved in the lymphocyte activation pathway and survival in NSCLC patients, it is important to detect biomarkers of lymphocyte activation to predict NSCLC survival and to provide a rationale for further investigation into the functions of survival-related SNPs in lymphocyte activation-related pathway genes in the progression of NSCLC. Therefore, we examined genotyping data from two known GWAS datasets and assessed associations between genetic variants in lymphocyte activation-related genes and survival in NSCLC patients. Here, we present the following article in accordance with the REMARK reporting checklist (available at https://tlcr.amegroups.com/article/view/10.21037/tlcr-22-104/rc).
Methods
Study population
In the current study, the discovery dataset included 1,185 eligible patients, which was from the GWAS dataset of the Prostate, Lung, Colorectal and Ovarian (PLCO) Cancer Screening Trial (https://www.ncbi.nlm.nih.gov/projects/gap/cgi-bin/study.cgi?study_id=phs001094.v1.p1). The PLCO was a randomized clinical trial funded by the National Cancer Institute (NCI), enrolling 77,500 males and 77,500 females with the age of 55–74 years, across 10 American medical facilities between 1993 and 2011. The included individuals were randomly assigned to the intervention (screening) and control (standard care) arms, and followed up for 13 years or more (13). Blood-extracted genomic DNA was utilized for genotyping using Illumina Human Hap550v3.0 and Human Hap240Sv1.0 (dbGaP accession: phs000093.v2.p2 and phs000336.v1.p1, respectively) (14,15). The PLCO trial was approved by the institutional review boards of each participating institution, with written informed consent obtained from each participant.
The validation dataset consisted of 984 Caucasian NSCLC cases from another NSCLC GWAS dataset, the Harvard Lung Cancer Susceptibility (HLCS) trial, where whole blood specimens were obtained from all patients, as well as personal data following diagnosis. DNA was extracted from blood specimens using the Auto Pure Large Sample Nucleic Acid Purification System (QIAGEN Company, Venlo, Limburg, Netherlands), followed by genotyping using Illumina Human hap610-Quad arrays. Genotyping data were imputed using MaCH3.0 software based on the 1000 Genomes Project (16).
The utilization of both GWAS cohorts in the current study was authorized by the National Center for Biological Information (NCBI; Project #6404) and the Internal Review Board of Duke University School of Medicine (Project #Pro00054575). The characteristics of the HLCS (n=984) and PLCO (n=1,185) trials have been previously described (17). The current study was conducted in accordance with the Declaration of Helsinki (as revised in 2013).
Gene selection and SNP imputation
In the Molecular Signatures Database (http://software.broadinstitute.org/gsea/msigdb/index.jsp), we selected the genes involved in lymphocyte activation-related pathway by the keyword “lymphocyte AND immune”. A total of 176 genes were available for further analysis as candidate genes (Table S1). Imputation with Minimac4 and r-square ≥0.3 was performed for these candidate genes. The totality of related SNPs and their ±2 kb flanking regions were obtained and submitted for quality assessment, as presented in Figure 1. Finally, 14,400 SNPs (1,599 genotyped and 12,801 imputed) from the PLCO trial underwent further analysis.
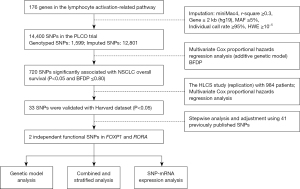
Statistical analyses
The follow-up time was defined as from the diagnosis of lung cancer to the last follow-up or death in both the PLCO and the HLCS datasets. Overall survival (OS) and disease-specific survival (DSS) were used as endpoints in the current study. In single-locus analysis, age, sex, smoking status, histology, tumor stage, chemotherapy, radiotherapy, surgery, and the top four principal components of the PLCO genotyping dataset described previously were adjusted for as previously reported (17) and then we used multivariable Cox proportional hazards regression analysis to evaluate associations between various SNPs in 176 lymphocyte activation related pathway genes and OS/DSS in the additive genetic model. For multiple testing corrections, the Bayesian false discovery probability (BFDP) was used with a cutoff of 0.80 (18). The upper bound of detectable relative risk was set as 3.0 with a prior probability of 0.10 in BFDP calculation. Afterwards, the selected SNPs of the PLCO trial were further validated using the HLCS dataset. A multivariable stepwise Cox regression model was utilized to identify independent SNPs after adjusting for patient characteristics and 41 previously reported survival-predictive SNPs from the PLCO trial. Finally, as to integrate the findings of the discovery and validation datasets, we performed inverse variance weighted meta-analysis, by using PLINK 1.90 with Cochran’s Q statistics and I2. The fixed-effects model was used with Cochran’s Q-test P>0.100 and heterogeneity statistic (I2)<50%; otherwise, the random-effects model was applied.
We also used Kaplan-Meier (KM) survival curve analysis for survival assessment by the combined genotype. For the stratified analysis in subgroups, we evaluated inter-study heterogeneity and potential interaction by the χ2-based Q-test. Subsequently, the receiver operating characteristic (ROC) curve and area under the ROC curve (AUC) were used to estimate the accuracy of the predictive model combining patient features and genetic factors in NSCLC survival using the timeROC package of R v3.6.3.
In expression quantitative trait loci (eQTL) analysis, we evaluated associations between SNPs and their respective mRNA expression levels by applying a linear regression model. Three sources provided the mRNA expression data for the genes of interest: the GWAS datasets of noncancerous lung tissue specimens from 383 individuals and whole blood cell specimens from 369 patients included in the genotype-tissue expression (GTEx) project (19); 373 European patients in the 1000 Genomes Project (20); and lung cancer tissue samples from The Cancer Genome Atlas (TCGA) (dbGaP Study Accession: phs000178.v10.p8) (21). A paired t-test was also conducted to examine differences in mRNA expression between paired cancer and adjacent noncancerous tissue specimens in the TCGA database. Finally, the KM method was used to assess the association of mRNA expression and survival (http://kmplot.com/analysis/index.php?p=service&cancer=lung).
Bioinformatics-based functional prediction was made for the validated SNPs to predict their bioinformatics function using SNPinfo (22) (https://snpinfo.niehs.nih.gov), RegulomeDB (23) (http://www.regulomedb.org), and HaploReg (24) (http://archive.broadinstitute.org/mammals/haploreg/haploreg.php). The cBioPortal for Cancer Genomics (http://www.cbioportal.org) database was used to examine mutation data for selected genes in lung cancer samples. Unless specified, SAS v9.4 (SAS Institute, Cary, NC, USA) was used for analysis of data.
Results
The association of SNPs in lymphocyte activation-related pathway genes with OS in NSCLC patients in the PLCO and HLCS datasets
Figure 1 depicts the study design. The baseline features of 1,185 and 984 NSCLC patients in the PLCO and HLCS studies have been reported previously (17). In the discovery phase using the PLCO dataset, 14,400 SNPs (including 1,599 genotyped and 12,801 imputed SNPs) were identified in 176 lymphocyte activation-related pathway genes, of which 720 SNPs were found to be significantly associated with NSCLC OS (P<0.05) after multiple test corrections (BFDP ≤0.8). A total of 33 SNPs remained significant with a P value less than 0.05 after further validation with the HLCS dataset.
Independent SNPs related to OS in NSCLC in the PLCO dataset
As the HLCS dataset not having individual genotyping and detailed patient covariates, we used stepwise multivariable Cox regression analyses to evaluate independent effects of the 33 SNPs in the PLCO dataset. Subsequent multivariate stepwise Cox analyses were adjusted for available patient-related variables, the first four principal components, and 41 previously reported SNPs from the PLCO GWAS dataset. Two SNPs in two distinct genes, namely, forkhead box P1 (FOXP1) rs2568847 G>C and RAR-related orphan receptor A (RORA) rs922782 T>G, remained significantly associated with OS of patients with NSCLC (P=0.0013 and P=0.0057, respectively; Table 1). Further combined-analysis of these two SNPs showed Cochran’s Q-test P<0.100 and I2>50% for rs922782, and thus, the random effects model was employed (Table 2).
Table 1
Variables | Category | Frequency | HR (95% CI)a | Pa | HR (95% CI)b | Pb |
---|---|---|---|---|---|---|
Age | Continuous | 1,185 | 1.03 (1.02–1.05) | <0.0001 | 1.04 (1.03–1.06) | <0.0001 |
Sex | Male | 698 | 1.00 | 1.00 | ||
Female | 487 | 0.76 (0.66–0.89) | 0.005 | 0.70 (0.59–0.82) | <0.0001 | |
Smoking status | Never | 115 | 1.00 | 1.00 | ||
Current | 423 | 1.69 (1.26–2.27) | 0.0004 | 2.10 (1.55–2.85) | <0.0001 | |
Former | 647 | 1.67 (1.27–2.20) | 0.0003 | 2.03 (1.52–2.70) | <0.0001 | |
Histology | Adenocarcinoma | 577 | 1.00 | 1.00 | ||
Squamous cell | 285 | 1.20 (0.99–1.44) | 0.059 | 1.20 (0.99–1.46) | 0.071 | |
Others | 323 | 1.32 (1.12–1.57) | 0.001 | 1.41 (1.18–1.69) | 0.0002 | |
Tumor stage | I–IIIA | 655 | 1.00 | 1.00 | ||
IIIB–IV | 528 | 2.84 (2.34–3.45) | <0.0001 | 3.40 (2.78–4.16) | <0.0001 | |
Chemotherapy | No | 639 | 1.00 | 1.00 | ||
Yes | 538 | 0.57 (0.48–0.68) | <0.0001 | 0.54 (0.45–0.65) | <0.0001 | |
Radiotherapy | No | 762 | 1.00 | 1.00 | ||
Yes | 415 | 0.93 (0.79–1.10) | 0.415 | 0.98 (0.83–1.16) | 0.821 | |
Surgery | No | 637 | 1.00 | 1.00 | ||
Yes | 540 | 0.20 (0.15–0.26) | <0.0001 | 0.18 (0.14–0.23) | <0.0001 | |
FOXP1 rs2568847 G>C | GG/GC/CC | 602/496/87 | 1.17 (1.03–1.32) | 0.0157 | 1.22 (1.08–1.38) | 0.0013 |
RORA rs922782 T>G | TT/TG/GG | 333/602/250 | 0.74 (0.67–0.82) | <0.0001 | 0.86 (0.77–0.96) | 0.0057 |
a, adjusted for age, sex, tumor stage, histology, smoking status, chemotherapy, radiotherapy, surgery, identified SNPs, and PC1, PC2, PC3, PC4; b, other 41 published SNPs were included for further adjustment: rs779901, rs3806116, rs199731120, rs10794069, rs1732793, rs225390, rs3788142, rs73049469, rs35970494, rs225388, rs7553295, rs1279590, rs73534533, rs677844, rs4978754, rs1555195, rs11660748, rs73440898, rs13040574, rs469783, rs36071574, rs7242481, rs1049493, rs1801701, rs35859010, rs1833970, rs254315, rs425904, rs35385129, rs4487030, rs60571065, rs13213007, rs115613985, rs9673682, rs2011404, rs7867814, rs2547235, rs4733124, rs11225211, rs11787670, rs67715745. SNPs, single-nucleotide polymorphisms; NSCLC, non-small cell lung cancer; PLCO, the Prostate, Lung, Colorectal and Ovarian cancer screening trial; HR, hazards ratio; CI, confidence interval.
Table 2
SNPs | Allelea | Gene | PLCO (n=1,185) | HLCS (n=984) | Combined-analysis | |||||||||
---|---|---|---|---|---|---|---|---|---|---|---|---|---|---|
EAF | HR (95% CI)b | Pb | EAF | HR (95% CI)c | Pc | Phetd | I 2 | HR (95% CI)e | Pe | |||||
rs2568847 | G>C | FOXP1 | 0.28 | 1.23 (1.10–1.38) | 2×10–4 | 0.25 | 1.19 (1.06–1.35) | 0.0046 | 0.718 | 0 | 1.21 (1.11–1.32) | 5.38×10−6 | ||
rs922782 | T>G | RORA | 0.47 | 0.75 (0.68–0.83) | 1.90×10–8 | 0.43 | 0.90 (0.81–0.99) | 0.0383 | 0.014 | 83.44 | 0.82 (0.76–0.88) | 2.68×10−2 |
a, effect/reference allele; b, adjusted for age, sex, stage, histology, smoking status, chemotherapy, radiotherapy, surgery, PC1, PC2, PC3 and PC4; c, adjusted for age, sex, stage, histology, smoking status, chemotherapy, radiotherapy, surgery, PC1, PC2 and PC3; d, Phet, P value for heterogeneity by Cochrane’s Q test; e, meta-analysis in the random-effects model. SNPs, single-nucleotide polymorphisms; NSCLC, non-small cell lung cancer; GWAS, genome-wide association study; PLCO, the Prostate, Lung, Colorectal and Ovarian cancer screening trial; HLCS, Harvard Lung Cancer Susceptibility Study; EAF, effect allele frequency; HR, hazards ratio; CI, confidence interval.
As shown in Table 3, in the dominant genetic model comparing with reference genotypes, FOXP1 rs2568847 GC + CC and RORA rs922782 TT were both associated with a significantly increased death risk (rs2568847 GC + CC, Ptrend=0.0001 for OS and Ptrend=0.0002 for DSS; rs922782 TT, Ptrend<0.0001 for both OS and DSS). The gene locations of all significant SNPs in the genome were visualized with Manhattan plots in Figure S1 and the independent SNPs were shown with regional association plots in Figure S2.
Table 3
Alleles | Frequencya | OSb | DSSb | |||||
---|---|---|---|---|---|---|---|---|
Death (%) | HR (95% CI) | P | Death (%) | HR (95% CI) | P | |||
FOXP1 rs2568847 G>C | ||||||||
GG | 598 | 392 (65.55) | 1.00 | 349 (58.36) | 1.00 | |||
GC | 490 | 332 (67.76) | 1.31 (1.13–1.52) | 0.0004 | 303 (61.84) | 1.32 (1.13–1.54) | 0.0006 | |
CC | 87 | 65 (74.71) | 1.40 (1.07–1.83) | 0.0148 | 57 (65.52) | 1.38 (1.04–1.84) | 0.0261 | |
Trend test | 0.0002 | 0.0004 | ||||||
Dominant | ||||||||
GG | 598 | 392 (65.55) | 1.00 | 349 (58.36) | 1.00 | |||
GC + CC | 577 | 397 (68.80) | 1.32 (1.15–1.52) | 0.0001 | 360 (62.39) | 1.33 (1.14–1.54) | 0.0002 | |
RORA rs922782 T>G | ||||||||
TT | 329 | 241 (73.25) | 1.00 | 224 (68.09) | 1.00 | |||
TG | 598 | 405 (67.73) | 0.83 (0.71–0.98) | 0.0233 | 362 (60.54) | 0.81 (0.69–0.96) | 0.0153 | |
GG | 248 | 143 (57.66) | 0.54 (0.44–0.67) | <0.0001 | 123 (49.60) | 0.52 (0.41–0.65) | <0.0001 | |
Trend test | <0.0001 | <0.0001 | ||||||
Dominant | ||||||||
TT | 329 | 241 (73.25) | 1.00 | 224 (68.09) | 1.00 | |||
TG + GG | 846 | 548 (64.78) | 0.73 (0.63–0.86) | <0.0001 | 485 (57.33) | 0.71 (0.61–0.84) | <0.0001 | |
Reversed | ||||||||
TG + GG | 846 | 548 (64.78) | 1.00 | 485 (57.33) | 1.00 | |||
TT | 329 | 241 (73.25) | 1.36 (1.17−1.59) | <0.0001 | 224 (68.09) | 1.41 (1.20−1.65) | <0.0001 | |
NUGc | ||||||||
0 | 436 | 277 (63.53) | 1.00 | 240 (55.05) | 1.00 | |||
1 | 572 | 386 (67.48) | 1.41 (1.20–1.65) | <0.0001 | 354 (61.89) | 1.48 (1.25–1.75) | <0.0001 | |
2 | 167 | 126 (75.45) | 1.70 (1.37–2.11) | <0.0001 | 115 (68.86) | 1.73 (1.38–2.17) | <0.0001 | |
Trend test | <0.0001 | <0.0001 | ||||||
Dichotomized NUG | ||||||||
0 | 436 | 277 (63.53) | 1.00 | 115 (68.86) | 1.00 | |||
1–2 | 739 | 512 (69.28) | 1.47 (1.27–1.71) | <0.0001 | 594 (58.93) | 1.53 (1.31–1.80) | <0.0001 |
a, 10 missing date were excluded; b, adjusted for age, sex, smoking status, histology, tumor stage, chemotherapy, surgery, radiotherapy and principal components; c, unfavorable genotypes were FOXP1 rs2568847 GC + CC and RORA rs922782 TT. SNP, single nucleotide polymorphism; OS, overall survival; DSS, disease-specific survival; NSCLC, non-small cell lung cancer; PLCO, Prostate, Lung, Colorectal and Ovarian cancer screening trial; HR, hazard ratio; CI, confidence interval; NUG, number of unfavorable genotypes.
Analyses of independent SNPs related to NSCLC survival in the PLCO dataset
For analyzing the accumulative impact of significant SNPs on survival of NSCLC patients, their unfavorable genotypes (FOXP1 rs2568847 GC + CC and RORA rs922782 TT) into a genetic score to classify all NSCLC cases into three groups according to the number of unfavorable genotypes (NUG). Multivariate analysis showed that a high genetic score was significantly associated with an increased death risk (Ptrend<0.0001 for both OS and DSS). Based on NUG, all cases were divided into the low-risk group (0 NUG) and high-risk group (1–2 NUGs). Compared with the 0-NUG group, the 1–2 NUGs group showed markedly elevated risk of death both in terms of OS (HR =1.47; 95% CI, 1.27 to 1.71; P<0.0001) and DSS (HR =1.53; 95% CI, 1.31 to 1.80; P<0.0001). KM curves were constructed to represent the association between unfavorable genotypes and OS and DSS in NSCLC (Figure 2A-2D).
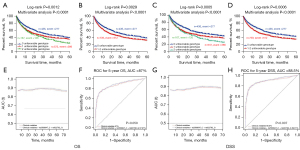
In further stratification analysis of any effects from combined deleterious genotypes on NSCLC survival to be altered by age, sex, smoking status, histology, tumor stage, chemotherapy, radiotherapy, and/or surgery in the PLCO dataset. We did not find any remarkable associations between unfavorable genotypes and each of these covariates on NSCLC survival, except for age and chemotherapy treatment (age, P=0.0432 and P=0.0196 for OS and DSS, respectively; chemotherapy, P=0.0383 and P=0.0301 for OS and DSS, respectively) (Table S2).
Time dependent AUC and ROC curve for both independent SNPs for the prediction of NSCLC survival
The ability of NUG to predict 5-year survival of NSCLC patients was assessed by comparing the AUC of the model including only clinical variables that integrate both patient indexes and NUGs. Addition of risk genotypes to the 5-year OS prediction model resulted in an increase in the AUC from 87.0% to 87.97% (P=0.059; Figure 2E,2F), while the AUC of the prediction model for 5-year DSS changed noticeably from 88.50% to 89.61% (P=0.007; Figure 2G,2H).
eQTL analysis
To explore the association between these SNPs and the respective mRNA expression, we performed eQTL analysis. In the GTEx Project dataset, the FOXP1 rs2568847 C allele was significantly correlated with higher FOXP1 mRNA expression in 369 whole blood specimens (P=0.004; Figure 3A) but not in 383 normal lung tissue samples (Figure S3A). In contrast, the RORA rs922782 G allele was not significantly associated with the corresponding mRNA expression in noncancerous lung tissues (n=515) nor whole blood specimens (n=515) (P=0.720; Figure S3B, P=0.069; Figure S3C). In lymphoblastoid cells RNA-Seq data from the 1000 Genomes Project, the FOXP1 rs2568847 C allele and mRNA expression were not significantly correlated in any of the three genetic models (Figure S3D-S3F). Interestingly, the RORA rs922782 G allele was significantly associated with higher expression of RORA in the recessive model (P=0.014; Figure 3B), but not in the other two genetic models (Figure S3G,S3H).
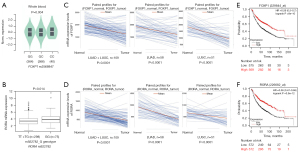
Differential mRNA expression analysis in target tissues
The mRNA expression level of these genes was assessed in paired NSCLC and adjacent noncancerous tissue specimens in the TCGA database to further evaluate the expression of these two genes in NSCLC. As shown in Figure 3C, FOXP1 mRNA expression level was markedly lower in lung adenocarcinoma (LUAD), lung squamous cell carcinoma (LUSC), and combined LUAD + LUSC specimens compared to the paired adjacent noncancerous tissue specimens (P<0.0001 for all). Similarly, RORA had lower expression levels in LUAD, LUSC, and LUAD + LUSC combined specimens, compared with the adjacent paired noncancerous lung tissues (P<0.0001 for all, Figure 3D). Additionally, high mRNA expression levels of both FOXP1 and RORA were correlated with a better survival as shown by the KM survival curves of lung cancer (Figure 3E,3F) (http://kmplot.com/analysis/index.php?p=service&cancer=lung).
Bioinformatics functional prediction analysis
We further performed functional prediction analysis on these two significant SNPs by using three online bioinformatics tools, including SNPinfo, RegulomeDB, and HaploReg. The results demonstrated that both FOXP1 rs2568847 G>C and the RORA rs922782 T>G changes may potentially affect enhancer histone marks and cause motif changes (Table S3). In addition, based on experimental data collected by the Encyclopedia of DNA Elements (ENCODE) project, RORA rs922782 is likely located on the H3K4Me1 motif, and the minor allele may impact its binding activity to regulate transcription factors (Figure S4).
Mutation analysis
Finally, we evaluated mutation status of FOXP1 and RORA in lung cancer tissues in the cBioPortal for Cancer Genomics database. As depicted in Figure S5, FOXP1 displayed relatively higher somatic mutation rates in the NSCLC database (4%, in the MSKCC 2018; 2.5% in the MSK PD1; and 2.19% in the TCGA 2016). In contrast, RORA showed starkly lower mutation rates (0.89% in the TRACERx 2017 and 0.79% in the TCGA 2016). Considering the lower mutation rate in RORA, our findings suggest that functional SNPs in RORA may have an important function in controlling mRNA expression in NSCLC.
Discussion
In the current study, we used two previously published GWASs to have assessed the associations between SNPs in the lymphocyte activation-related pathway genes and survival of NSCLC patients. We identified and validated two novel SNPs, namely, FOXP1 rs2568847 G>C and RORA rs922782 T>G, which were both shown to have a significant association with both NSCLC OS and DSS in European individuals after adjustment for available covariates and the 41 previously-reported significant SNPs in the PLCO trial. Additionally, the combined risk genotypes of these two SNPs significantly predicted a reduced NSCLC OS and DSS. Further eQTL analysis for examining the correlation of functional genotype and mRNA expression levels revealed that in 369 whole blood samples, the FOXP1 rs2568847 C allele significantly predict an elevated FOXP1 mRNA transcript levels while the RORA rs922782 G allele was significantly associated with higher expression levels of RORA in lymphoblastoid cell lines from 373 European individuals in the 1000 Genomes Project. Meanwhile, FOXP1 and RORA mRNA expression levels in lung tumor tissue specimens were significantly lower than that of adjacent noncancerous lung tissue samples in the TCGA database. Both these genes appear to have tumor suppressive effects, because an elevated mRNA transcript level predicted an improved survival of NSCLC patients. Jointly, these findings revealed a biologically plausible association between genetic variations in lymphocyte activation-related genes and survival of NSCLC patients.
FOXP1, located on chromosome 3p14.1, is a member of the widely expressed FOXP subfamily of “forkhead” transcription factors that have broad functions in cell cycle progression, proliferation, differentiation, and apoptosis (25). FOXP proteins, encoded by FOXP1, play critical roles in immune responses, lymphocyte development, and cancer pathogenesis (26-28). Previous studies have suggested that FOXP1 may function as an oncogene or a tumor suppressor depending on the cancer type (29-31). FOXP1 is reportedly able to mediate the inductive effects of select factors affecting NSCLC progression in vitro. For instance, a study has shown that lymphoid enhancer-binding factor 1 antisense RNA 1 (LEF1-AS1) promotes the malignant behavior of lung cancer cells via FOXP1 upregulation (32). Another study has demonstrated that long intergenic non-protein coding RNA 1614 (LINC01614) is involved in LUAD cell progression through FOXP1 upregulation (33). In contrast, one study has reported that high FOXP1 expression level is correlated with an increased survival of NSCLC patients (34) and that FOXP1 knockdown promotes the progression of LUAD (35). Thus, the functions of FOXP1 in lung cancer progression are still controversial. Here, our data showed that FOXP1 mRNA expression was significantly reduced in lung cancer compared with noncancerous tissue specimens and that high FOXP1 expression was correlated with an improved survival. These results suggest that FOXP1 is more likely to play a suppressor role in lung cancer. Meanwhile, the FOXP1 rs2568847 C allele significantly predicted a higher FOXP1 transcript levels in unaffected cells, while the rs2568847 C allele was correlated with a reduced survival of NSCLC patients in the PLCO dataset. Therefore, additional molecular mechanisms might alter FOXP1 expression in cancer, which warrants further investigation.
RAR-RORA, located at 15q22.2, encodes a nuclear receptor that belongs to the ROR subfamily (36). RORA contributes to anti-apoptotic and anti-inflammatory responses, repressing cell proliferation in multiple malignancies such as breast, prostate, and ovarian cancers (37). Furthermore, RORA is frequently inactivated in cancers, which makes it an attractive target for cancer therapy (38). These data suggested that RORA might function as a cancer suppressor. This concurs with our data showing an elevated RORA expression level in noncancerous tissues, compared with lung cancer tissue specimens in the TCGA dataset. Another report demonstrated that a genetic variant of RORA was associated with cutaneous melanoma survival and might be a promising biomarker (39). Our results revealed that the RORA rs922782 G allele was associated with an increased mRNA expression level of RORA in lung cancer, which was in turn associated with an improved survival of NSCLC patients. According to the ENCODE database, the RORA rs922782 G allele is located in a substantial region of the H3K4Me1 motif, and the minor allele may alter its binding to affect transcription factors associated with RORA.
However, there were some limitations in the current study. First, the above-mentioned findings may not be applicable to other ethnicities, since only Caucasians were examined in both GWAS datasets studied. Second, despite a relatively large sample size in the PLCO trial, the subgroups had relatively small case numbers that would likely reduce the statistical power. Third, there are a number of distinct lymphocyte populations that have different effects, which requires further exploration. Finally, the exact molecular mechanisms and specific lymphocyte populations responsible for the determined associations of the identified independent SNPs with NSCLC patient survival remain unclear.
In conclusion, this report identified two independent functional SNPs, namely, FOXP1 rs2568847 G>C and RORA rs922782 T>G, that showed significant associations with survival of NSCLC patients in the discovery and validation GWAS datasets. It is likely that the RORA rs922782 G allele may affect survival of NSCLC patients, possibly by modifying targeted gene expression. These data provide a rationale for further investigation into the functions of survival-related SNPs in lymphocyte activation-related pathway genes in the progression of NSCLC.
Acknowledgments
The authors thank all the participants of the PLCO Cancer Screening Trial and the National Cancer Institute for providing access to the data collected by the PLCO trial. The statements contained herein are solely those of the authors and do not represent or imply concurrence or endorsement by the National Cancer Institute. The authors would also like to acknowledge the dbGaP repository for providing cancer genotyping datasets. The accession numbers for the datasets for lung cancer are phs000336.v1.p1 and phs000093.v2.p2. A list of contributing investigators and funding agencies for these studies can be found in https://cdn.amegroups.cn/static/public/10.21037tlcr-22-104-1.docx.
Funding: This work was supported by the National Institute of Health (Nos. CA090578, CA074386, CA092824, U01CA209414); the Duke Cancer Institute as part of the P30 Cancer Center Support Grant (No. NIH/NCI CA014236); and the V Foundation for Cancer Research (No. D2017-19).
Footnote
Reporting Checklist: The authors have completed the REMARK reporting checklist. Available at https://tlcr.amegroups.com/article/view/10.21037/tlcr-22-104/rc
Conflicts of Interest: All authors have completed the ICMJE uniform disclosure form (available at https://tlcr.amegroups.com/article/view/10.21037/tlcr-22-104/coif). The authors have no conflicts of interest to declare.
Ethical Statement: The authors are accountable for all aspects of the work in ensuring that questions related to the accuracy or integrity of any part of the work are appropriately investigated and resolved. The study was conducted in accordance with the Declaration of Helsinki (as revised in 2013).
Open Access Statement: This is an Open Access article distributed in accordance with the Creative Commons Attribution-NonCommercial-NoDerivs 4.0 International License (CC BY-NC-ND 4.0), which permits the non-commercial replication and distribution of the article with the strict proviso that no changes or edits are made and the original work is properly cited (including links to both the formal publication through the relevant DOI and the license). See: https://creativecommons.org/licenses/by-nc-nd/4.0/.
References
- Oudkerk M, Liu S, Heuvelmans MA, et al. Lung cancer LDCT screening and mortality reduction - evidence, pitfalls and future perspectives. Nat Rev Clin Oncol 2021;18:135-51. [Crossref] [PubMed]
- Siegel RL, Miller KD, Fuchs HE, et al. Cancer Statistics, 2021. CA Cancer J Clin 2021;71:7-33. [Crossref] [PubMed]
- Rodak O, Peris-Díaz MD, Olbromski M, et al. Current Landscape of Non-Small Cell Lung Cancer: Epidemiology, Histological Classification, Targeted Therapies, and Immunotherapy. Cancers (Basel) 2021;13:4705. [Crossref] [PubMed]
- Vrankar M, Stanic K. Long-term survival of locally advanced stage III non-small cell lung cancer patients treated with chemoradiotherapy and perspectives for the treatment with immunotherapy. Radiol Oncol 2018;52:281-8. [Crossref] [PubMed]
- Wu F, Fan J, He Y, et al. Single-cell profiling of tumor heterogeneity and the microenvironment in advanced non-small cell lung cancer. Nat Commun 2021;12:2540. [Crossref] [PubMed]
- Zhao J, Lin G, Zhuo M, et al. Next-generation sequencing based mutation profiling reveals heterogeneity of clinical response and resistance to osimertinib. Lung Cancer 2020;141:114-8. [Crossref] [PubMed]
- Ren X, Zhang L, Zhang Y, et al. Insights Gained from Single-Cell Analysis of Immune Cells in the Tumor Microenvironment. Annu Rev Immunol 2021;39:583-609. [Crossref] [PubMed]
- Stanton SE, Disis ML. Clinical significance of tumor-infiltrating lymphocytes in breast cancer. J Immunother Cancer 2016;4:59. [Crossref] [PubMed]
- Paijens ST, Vledder A, de Bruyn M, et al. Tumor-infiltrating lymphocytes in the immunotherapy era. Cell Mol Immunol 2021;18:842-59. [Crossref] [PubMed]
- Gueguen P, Metoikidou C, Dupic T, et al. Contribution of resident and circulating precursors to tumor-infiltrating CD8+ T cell populations in lung cancer. Sci Immunol 2021;6:eabd5778. [Crossref] [PubMed]
- Bowden SJ, Bodinier B, Kalliala I, et al. Genetic variation in cervical preinvasive and invasive disease: a genome-wide association study. Lancet Oncol 2021;22:548-57. [Crossref] [PubMed]
- Gallagher MD, Chen-Plotkin AS. The Post-GWAS Era: From Association to Function. Am J Hum Genet 2018;102:717-30. [Crossref] [PubMed]
- Weissfeld JL, Schoen RE, Pinsky PF, et al. Flexible sigmoidoscopy in the randomized prostate, lung, colorectal, and ovarian (PLCO) cancer screening trial: added yield from a second screening examination. J Natl Cancer Inst 2012;104:280-9. [Crossref] [PubMed]
- Tryka KA, Hao L, Sturcke A, et al. NCBI's Database of Genotypes and Phenotypes: dbGaP. Nucleic Acids Res 2014;42:D975-9. [Crossref] [PubMed]
- Mailman MD, Feolo M, Jin Y, et al. The NCBI dbGaP database of genotypes and phenotypes. Nat Genet 2007;39:1181-6. [Crossref] [PubMed]
- Zhai R, Yu X, Wei Y, et al. Smoking and smoking cessation in relation to the development of co-existing non-small cell lung cancer with chronic obstructive pulmonary disease. Int J Cancer 2014;134:961-70. [Crossref] [PubMed]
- Wang Y, Liu H, Ready NE, et al. Genetic variants in ABCG1 are associated with survival of nonsmall-cell lung cancer patients. Int J Cancer 2016;138:2592-601. [Crossref] [PubMed]
- Wakefield J. A Bayesian measure of the probability of false discovery in genetic epidemiology studies. Am J Hum Genet 2007;81:208-27. [Crossref] [PubMed]
- GTEx Consortium. Human genomics. The Genotype-Tissue Expression (GTEx) pilot analysis: multitissue gene regulation in humans. Science 2015;348:648-60. [Crossref] [PubMed]
- Lappalainen T, Sammeth M, Friedländer MR, et al. Transcriptome and genome sequencing uncovers functional variation in humans. Nature 2013;501:506-11. [Crossref] [PubMed]
- Cancer Genome Atlas Research Network. Comprehensive molecular profiling of lung adenocarcinoma. Nature 2014;511:543-50. [Crossref] [PubMed]
- Xu Z, Taylor JA. SNPinfo: integrating GWAS and candidate gene information into functional SNP selection for genetic association studies. Nucleic Acids Res 2009;37:W600-5. [Crossref] [PubMed]
- Boyle AP, Hong EL, Hariharan M, et al. Annotation of functional variation in personal genomes using RegulomeDB. Genome Res 2012;22:1790-7. [Crossref] [PubMed]
- Ward LD, Kellis M. HaploReg v4: systematic mining of putative causal variants, cell types, regulators and target genes for human complex traits and disease. Nucleic Acids Res 2016;44:D877-81. [Crossref] [PubMed]
- Calissi G, Lam EW, Link W. Therapeutic strategies targeting FOXO transcription factors. Nat Rev Drug Discov 2021;20:21-38. [Crossref] [PubMed]
- Ren J, Han L, Tang J, et al. Foxp1 is critical for the maintenance of regulatory T-cell homeostasis and suppressive function. PLoS Biol 2019;17:e3000270. [Crossref] [PubMed]
- Gao J, Geng R, Deng H, et al. A Novel Forkhead Box Protein P (FoxP) From Litopenaeus vannamei Plays a Positive Role in Immune Response. Front Immunol 2020;11:593987. [Crossref] [PubMed]
- Kim JH, Hwang J, Jung JH, et al. Molecular networks of FOXP family: dual biologic functions, interplay with other molecules and clinical implications in cancer progression. Mol Cancer 2019;18:180. [Crossref] [PubMed]
- Li H, Han X, Yang S, et al. FOXP1 drives osteosarcoma development by repressing P21 and RB transcription downstream of P53. Oncogene 2021;40:2785-802. [Crossref] [PubMed]
- Wang W, Li Y, Li X, et al. Circular RNA circ-FOXP1 induced by SOX9 promotes hepatocellular carcinoma progression via sponging miR-875-3p and miR-421. Biomed Pharmacother 2020;121:109517. [Crossref] [PubMed]
- O'Sullivan AG, Eivers SB, Mulvaney EP, et al. Regulated expression of the TPβ isoform of the human T prostanoid receptor by the tumour suppressors FOXP1 and NKX3.1: Implications for the role of thromboxane in prostate cancer. Biochim Biophys Acta Mol Basis Dis 2017;1863:3153-69. [Crossref] [PubMed]
- Wang A, Zhao C, Gao Y, et al. LEF1-AS1 contributes to proliferation and invasion through regulating miR-544a/ FOXP1 axis in lung cancer. Invest New Drugs 2019;37:1127-34. [Crossref] [PubMed]
- Liu AN, Qu HJ, Yu CY, et al. Knockdown of LINC01614 inhibits lung adenocarcinoma cell progression by up-regulating miR-217 and down-regulating FOXP1. J Cell Mol Med 2018;22:4034-44. [Crossref] [PubMed]
- Feng J, Zhang X, Zhu H, et al. High expression of FoxP1 is associated with improved survival in patients with non-small cell lung cancer. Am J Clin Pathol 2012;138:230-5. [Crossref] [PubMed]
- Sheng H, Li X, Xu Y. Knockdown of FOXP1 promotes the development of lung adenocarcinoma. Cancer Biol Ther 2019;20:537-45. [Crossref] [PubMed]
- Solt LA, Burris TP. Action of RORs and their ligands in (patho)physiology. Trends Endocrinol Metab 2012;23:619-27. [Crossref] [PubMed]
- Morales-Santana S, Morell S, Leon J, et al. An Overview of the Polymorphisms of Circadian Genes Associated With Endocrine Cancer. Front Endocrinol (Lausanne) 2019;10:104. [Crossref] [PubMed]
- Ma X, Chen H, Li L, et al. CircGSK3B promotes RORA expression and suppresses gastric cancer progression through the prevention of EZH2 trans-inhibition. J Exp Clin Cancer Res 2021;40:330. [Crossref] [PubMed]
- Li B, Wang Y, Xu Y, et al. Genetic variants in RORA and DNMT1 associated with cutaneous melanoma survival. Int J Cancer 2018;142:2303-12. [Crossref] [PubMed]