Association between changes in thioredoxin reductase and other peripheral blood biomarkers with response to PD-1 inhibitor-based combination immunotherapy in non-small cell lung cancer: a retrospective study
Introduction
Lung cancer was the second most frequently diagnosed cancer and the leading cause of cancer-related death worldwide in 2020 (1). Approximately two-thirds of lung cancer deaths worldwide are attributed to smoking (large variation according to the countries/continent) (1,2). Non-small cell lung cancer (NSCLC) represents is the most common histological subtype of lung cancer (3). Immunotherapy alone or in combination with chemotherapy have showed to significantly increase survival as compared to chemotherapy in patients with advanced NSCLC. Programmed death-ligand 1 (PD-L1), a ligand of programmed cell death receptor protein 1 (PD-1), has been shown to be upregulated in cancer cells and immune cells to inhibit the effector T cells (4,5). Correspondingly, antibodies that inhibit the interaction between PD-1 and PD-L1 have been developed as a therapeutic approach to enhance patients’ immune response against tumor cells. However, most of the patients benefit from immunotherapy but not all, some of them can experience significant immunotoxicities, moreover this kind of treatment is expensive and is not always widely available (6,7). By identifying predictive biomarkers effectiveness of PD-1 inhibitor-based therapies could be maximize, leading to healthcare resources optimization.
Currently, the solely biomarker approved to drive the immunotherapy is the PD-L1 expression that has demonstrated a correlation with overall response rate but has strong limitations (8). Several tumor tissue biomarkers (tumor mutational burden, gene expression signatures, presence or absence of oncogenic driver mutation, the density of tumor-infiltrating lymphocytes) and blood biomarkers have proved to affect the clinical outcome of patients when treated with immunotherapy but must be considered still investigational (9). In recent years, several biomarkers, including different types of blood cell counts, circulating tumor markers [interleukin (IL)-8, IL-6, blood tumor mutation burden, etc.], peripheral blood inflammation parameters, and thioredoxin reductase (TrxR), have been identified as potential prognostic factors for immunotherapies in patients with NSCLC (10,11). TrxR is an enzyme and part of the antioxidant reduction (redox) system which plays a crucial role in tumor development. High reactive oxygen species (ROS) level leads to oxidative stress and eventually increases serum TrxR (12). Several animal model studies have demonstrated a link between TrxR levels, cancer progression, and chemotherapy resistance in cancer cells (13-16). There was a tight relationship between TrxR and chemotherapy, which influences the redox process, and most of the PD-1-based immunotherapy performed for patients with advanced NSCLC was based on combination therapy. Therefore, it is important to monitor and analyze TrxR values in the treatment of advanced NSCLC patients. A retrospective population-based study showed that TrxR correlated with the efficacy of chemotherapy for gastric cancer (17). Other cell studies have shown that TrxR can modify immunomodulatory activities while increasing the effectiveness of various growth factors leading to cancer cell growth (18,19). Redox was also found to improve the immune microenvironment of tumors by blocking the thioredoxin (Trx)/TrxR system (20). In the context of immunotherapy, TrxR plays a crucial role in immunoregulation, and the complex mechanisms of action between the tumour microenvironment and immunotherapy drugs remain unclear, and it remains to be determined whether changes in TrxR will affect the efficacy of immunotherapy. Despite that there is evidence that TrxR could predict the response to chemotherapy in patients with gastric (17), hepatocellular (21) and breast cancer (22), the relationship between PD-1-based immunotherapy and TrxR in NSCLC remains unclear, highlighting the need for further research.
In this study, we aimed to retrospectively evaluate the prognostic value of biomarkers such as TrxR, clinicopathological parameters, and peripheral blood inflammatory factors in patients with advanced NSCLC receiving PD-1-based immunotherapy, association between TrxR and clinical outcomes in particular. We also aimed to develop predictive survival models that may guide clinicians in the use of PD-1-based immunotherapy in NSCLC patients. We present the following article in accordance with the REMARK reporting checklist (available at https://tlcr.amegroups.com/article/view/10.21037/tlcr-22-300/rc).
Methods
Study design and participants
We retrospectively enrolled patients diagnosed with advanced NSCLC who underwent PD-1-based immunotherapy as monotherapy or in combination with chemotherapy and bevacizumab at the Jiangsu Cancer Hospital, China from August 2018 to January 2021. Patients were considered eligible for the clinical study if we have stored peripheral serum samples at baseline, at 6 and 12 weeks after treatment onset. The following patients were excluded: (I) those with comorbidity including severe diabetes mellitus, heart failure, liver, and/or kidney failure; (II) those with major psychiatric disorders; (III) those with a history of other malignancies; and (IV) special populations, such as pregnant and lactating women. The principal endpoints of this study were the definition of the association between serum biomarkers and TrxR with overall survival (OS) and progression-free survival (PFS) assessed by univariate and multivariate Cox proportional hazard regression. Patients’ tumor characteristics, including gender, age, smoking status, types of pathology, number of metastatic lesions, epidermal growth factor receptor (EGFR) and Kirsten rat sarcoma viral oncogene homologue (KRAS) mutational status, stage, Eastern Cooperative Oncology Group performance status (ECOG PS), and other treatment received (i.e., radiotherapy), were extracted from the electronic medical records. The study was conducted in accordance with the Declaration of Helsinki (as revised in 2013). The study was approved by institutional Ethics Committee of Jiangsu Cancer hospital. Individual consent for this retrospective analysis was waived.
Assessment of hematological parameters
Hematological parameters, including white blood cell (WBC) count, absolute neutrophil count (ANC), absolute monocyte count (AMC), absolute lymphocyte count (ALC), platelet (PLT) count, lactate dehydrogenase (LDH), hemoglobin (Hb), albumin (Alb), carcinoembryonic antigen (CEA), neutrophil-to-lymphocyte ratio (NLR; ANC/ALC), lymphocyte-to-monocyte ratio (LMR), platelet-to-lymphocyte ratio (PLR; absolute platelet count/ALC), platelet-to-albumin ratio (PAR; absolute platelet count/Alb), and derived NLR (dNLR), were collected at baseline (before first round of treatment), 6 weeks after 2 cycles of treatment, and 12 weeks after 4 cycles of treatment. The differences in the hematological parameters at weeks 6 and 12 from the baseline values were calculated.
Assay for TrxR activity
Peripheral blood samples were collected in ethylenediaminetetraacetic acid (EDTA) and then centrifuged at 3,500 rpm for 5 minutes at room temperature within 2 hours of collection. The supernatant was harvested at 4 ℃ and tested immediately. Storage temperature was 4 ℃ while incubation temperature was 37 ℃. We measured TrxR activity with a commercially available TrxR activity colorimetric assay kit (Clairvoyance Health Technology Co., Ltd., Wuhan, China) using a 5,5'-dithiobis (2-nitrobenzoic) acid reduction, as recommended by the manufacturer. Positive and negative controls for the kit were performed for each reaction to monitor the performance of the assay.
Assessment of treatment response and survival
Whole-body computed tomography (CT) scans were performed every 6–8 weeks during treatment and response was assessed by investigators according to investigator asses the Response Evaluation Criteria in Solid Tumors (RECIST) version 1.1 (23). Follow-up was concluded by on January 1st 2021. Objective response rate (ORR) and disease control rate (DCR) were calculated for each patient. The ORR was defined as the sum of complete response (CR) and partial response (PR). The DCR was defined as the sum of CR, PR, and stable disease (SD). PFS and OS status were also assessed. Progression was defined as clinical or radiological progression or death after the initial treatment, whichever occurred first. The patients who, according to their medical records, were still alive at the time of data collection were followed up remotely by phone or email to assess survival.
Statistical analysis
We first evaluated the biomarker change among patients with advanced NSCLC. The patients’ baseline treatment blood parameters were considered as continuous variables. The post-treatment parameters were categorized into two groups based on whether they increased (Up group) or decreased (Down group) compared to the baseline treatment parameter. Continuous variables were first checked for normality by Shapiro-Wilk test, were all found to be not normally distributed, and were therefore expressed as medians and interquartile ranges (IQRs). Categorical variables were expressed as frequencies and percentages. Chi-square test or Fisher’s exact test was used to determine the univariate associations between categorical variables with treatment responses. Fisher’s test was used if the expected frequency of any categorical variable was less than 5.
Survival analysis was carried out using the Kaplan-Meier method and the log-rank test. Survival status and survival time factors were considered in the survival analysis. Cox proportional hazards regression analysis was conducted to identify potential indicators associated with PFS and/or OS. Factors with a P value less than 0.05 in the univariate Cox regression analysis were further included in a multivariate analysis to identify the factors independently associated with survival. Nomograms to predict the survival probability at 6, 12, and 18 months after treatment were constructed for each identified predictive factor. Each nomogram was also validated internally using a bootstrap method with 1,000 resamples. The predictive ability of each factor was assessed by calculating the concordance index (C-index), with a C-index of 0.5 indicating a completely random prediction and a C-index of 1.0 indicating a perfect prediction. Calibration curves and area under the curve (AUC) were used to assess the correlation between actual outcomes and predicted probabilities. Participants were categorized into high- or low-risk groups based on their predictive risk scores (higher or lower than the median score). The Kaplan-Meier method was used to compare the survival between the high- and low-risk groups for all constructed models.
The patient risk score and clinical characteristics were then tested for normality and homogeneity using the Shapiro-Wilk test and Levene’s test, respectively, whereby a P value above 0.05 indicated that the data were normally distributed with equal variance. For patient risk score and clinical characteristics that were normally distributed, analysis of variance (ANOVA) was used to compare the correlation between categorical variables. Conversely, if the distribution was not normal, or the variance was not equal, the non-parametric Kruskal-Wallis H-test was used to compare categorical variables.
We calculated the sample size of the multivariable Cox regression model for OS using the previously reported method (24). Given the widely accepted rule of thumb of 10 events per variable and given there were 3 variables in the final Cox model (25,26), the total number of events expected was 30. Taking into account an approximate 41% 1-year event rate and a 20% lost-to-review rate among the participants, we required a total sample size of at least 91 patients.
Statistical analyses were performed using R software (version 4.0.5; The R Foundation for Statistical Computing, Vienna, Austria) and SPSS 26.0 (IBM Corp., Armonk, NY, USA). A two-sided P value less than 0.05 was considered statistically significant.
Results
Participant characteristics
We retrospectively enrolled a total of 90 patients with advanced NSCLC. The patients’ baseline characteristics are presented in Table 1. The median age was 63 years, and only 3 cases had an ECOG PS of 2. Three-quarters of them were males and about two-thirds were ever smokers. About 60% of them had adenocarcinoma. All patients received PD-1 inhibitor-based combinations, less than 20% were treated with PD-1 inhibitor given as monotherapy. About two-thirds received immunotherapy in the second-line or third-line setting. After 6 weeks of treatment, TrxR was elevated in 31 patients and had decreased in 59 patients. The median age was 63 years, and only 3 cases had an ECOG PS of 2. The ORR and DCR were 26.7% and 94.4%, respectively. The median follow-up time was 19.7 (range, 13.6 to 25.8) months. The median PFS and OS were 8.5 months (range, 7.1 to 10.0 months; Figure 1A) and 15.5 months (range, 13.8 to 17.2 months; Figure 1B), respectively.
Table 1
Characteristics | TrxR at 6-week decreased (n=59) | TrxR at 6-week increased (n=31) | Total (n=90) |
---|---|---|---|
Gender | |||
Male | 43 (72.9) | 26 (83.9) | 69 (76.7) |
Female | 16 (27.1) | 5 (16.1) | 21 (23.3) |
Age (years) | |||
≥63 | 30 (50.8) | 15 (48.4) | 45 (50.0) |
63 | 29 (49.2) | 16 (51.6) | 45 (50.0) |
ECOG PS | |||
0 | 11 (18.6) | 3 (9.7) | 14 (15.6) |
1 | 45 (76.3) | 28 (90.3) | 73 (81.1) |
2 | 3 (5.1) | 0 (0.0) | 3 (3.3) |
PD-1 inhibitor | |||
Pembrolizumab | 26 (44.1) | 7 (22.6) | 33 (36.7) |
Sintilimab | 27 (45.8) | 20 (64.5) | 47 (52.2) |
Toripalimab | 6 (10.2) | 4 (12.9) | 10 (11.1) |
Stage | |||
Recurrence | 11 (18.6) | 6 (19.4) | 17 (18.9) |
IIIB | 8 (13.6) | 6 (19.4) | 14 (15.6) |
IV | 40 (67.8) | 19 (61.3) | 59 (65.6) |
Types of pathology | |||
Adenocarcinoma | 37 (62.7) | 20 (64.5) | 57 (63.3) |
Squamous | 22 (37.3) | 11 (35.5) | 33 (36.7) |
Smoking | |||
No | 20 (33.9) | 11 (35.5) | 31 (34.4) |
Yes | 39 (66.1) | 20 (64.5) | 59 (65.6) |
Mutation type | |||
EGFR | 8 (13.6) | 8 (25.8) | 16 (17.8) |
KRAS | 3 (5.1) | 2 (6.5) | 5 (5.6) |
Wild-type | 48 (81.4) | 20 (64.5) | 68 (75.6) |
Unknown | 0 (0.0) | 1 (3.2) | 1 (1.1) |
Degree of differentiation | |||
Low | 49 (83.1) | 24 (77.4) | 73 (81.1) |
Moderate/high | 10 (16.9) | 7 (22.6) | 17 (18.9) |
Lines of therapy | |||
1 | 22 (37.3) | 9 (29.0) | 31 (34.4) |
2 | 23 (39.0) | 13 (41.9) | 36 (40.0) |
3 | 14 (23.7) | 9 (29.0) | 23 (25.6) |
Treatment options | |||
PD-1 alone | 11 (18.6) | 5 (16.1) | 16 (17.8) |
Combination chemotherapy | 36 (61.0) | 17 (54.8) | 53 (58.9) |
Combination anti-angiogenic therapy | 3 (5.1) | 5 (16.1) | 8 (8.9) |
Both | 9 (15.3) | 4 (12.9) | 13 (14.4) |
Radiotherapy | |||
No | 30 (50.8) | 15 (48.4) | 45 (50.0) |
Yes | 29 (49.2) | 16 (51.6) | 45 (50.0) |
Data are expressed as n (%). NSCLC, non-small cell lung cancer; ECOG PS, Eastern Cooperative Oncology Group performance status; PD-1, programmed cell death receptor protein 1; EGFR, epidermal growth factor receptor; KRAS, Kirsten rat sarcoma viral oncogene homologue; TrxR, thioredoxin reductase.

Relationship between immunotherapy response and peripheral blood parameters of patients with advanced NSCLC
Table 2 summarizes the association between the peripheral blood indicators and treatment response. The variations of these indicators from baseline were calculated, and those that conformed to a skewed distribution were expressed as a median and IQR, as shown in Tables S1,S2.
Table 2
Variables | DCR | ORR | |||||
---|---|---|---|---|---|---|---|
With DCR/total, n/N (%) | χ2 | P value | With ORR/total, n/N (%) | χ2 | P value | ||
Age (years) | 5.294 | 0.021 | 0.227 | 0.634 | |||
≥63 | 45/45 (100.0) | 13/45 (28.9) | |||||
<63 | 40/45 (88.9) | 11/45 (24.4) | |||||
WBC6W | 2.532 | 0.112 | 11.012 | 0.001 | |||
Up | 37/41 (90.2) | 4/41 (9.8) | |||||
Down | 48/49 (98.0) | 20/49 (40.8) | |||||
ANC6W | 0.756 | 0.385 | 12.007 | 0.001 | |||
Up | 42/46 (91.3) | 5/46 (10.9) | |||||
Down | 43/44 (97.7) | 19/44 (43.2) | |||||
AMC6W | 0.000 | 1.000 | 0.909 | 0.340 | |||
Up | 42/45 (93.3) | 14/45 (31.1) | |||||
Down | 43/45 (95.6) | 10/45 (22.2) | |||||
ALC6W | 0.024 | 0.878 | 0.009 | 0.924 | |||
Up | 39/42 (92.9) | 11/42 (26.2) | |||||
Down | 46/48 (95.8) | 13/48 (27.1) | |||||
EON6W | 2.275 | 0.319 | 2.656 | 0.102 | |||
Up | 32/35 (91.4) | 6/35 (17.1) | |||||
Down | 53/55 (96.4) | 18/55 (32.7) | |||||
PLT6W | 0.003 | 0.959 | 0.818 | 0.366 | |||
Up | 35/37 (94.6) | 8/37 (21.6) | |||||
Down | 50/53 (94.3) | 16/53 (30.2) | |||||
LDH6W | 0.447 | 0.504 | 0.026 | 0.873 | |||
Up | 46/50 (92.0) | 13/50 (26.0) | |||||
Down | 39/40 (97.5) | 11/40 (27.5) | |||||
Hb6W | 0.101 | 0.750 | 0.010 | 0.921 | |||
Up | 32/33 (97.0) | 9/33 (27.3) | |||||
Down | 53/57 (93.0) | 15/57 (26.3) | |||||
Alb6W | 0.756 | 0.385 | 0.016 | 0.899 | |||
Up | 43/44 (97.7) | 12/44 (27.3) | |||||
Down | 42/46 (91.3) | 12/46 (26.1) | |||||
CEA6W | 4.695 | 0.030 | 2.675 | 0.102 | |||
Up | 34/39 (87.2) | 7/39 (17.9) | |||||
Down | 51/51 (100.0) | 17/51 (33.3) | |||||
TrxR6W | 1.401 | 0.237 | 0.018 | 0.894 | |||
Up | 31/31 (100.0) | 8/31 (25.8) | |||||
Down | 54/59 (91.5) | 16/59 (27.1) | |||||
NLR6W | 0.591 | 0.442 | 5.260 | 0.022 | |||
Up | 44/48 (91.7) | 8/48 (16.7) | |||||
Down | 41/42 (97.6) | 16/42 (38.1) | |||||
LMR6W | 0.000 | 1.000 | 0.227 | 0.634 | |||
Up | 42/45 (93.3) | 11/45 (24.4) | |||||
Down | 43/45 (95.6) | 13/45 (28.9) | |||||
PLR6W | 0.000 | 1.000 | 0.000 | 1.000 | |||
Up | 43/45 (95.6) | 12/45 (26.7) | |||||
Down | 42/45 (93.3) | 12/45 (26.7) | |||||
PAR6W | 0.000 | 1.000 | 2.675 | 0.102 | |||
Up | 37/39 (94.9) | 7/39 (17.9) | |||||
Down | 48/51 (94.1) | 17/51 (33.3) | |||||
dNLR6W | 0.000 | 1.000 | 4.139 | 0.042 | |||
Up | 43/46 (93.5) | 8/46 (17.4) | |||||
Down | 42/44 (95.5) | 16/44 (36.4) | |||||
PNI6W | 0.000 | 1.000 | 0.909 | 0.340 | |||
Up | 42/45 (93.3) | 14/45 (31.1) | |||||
Down | 43/45 (95.6) | 10/45 (22.2) | |||||
WBC12W | 0.000 | 1.000 | 0.227 | 0.634 | |||
Up | 42/45 (93.3) | 11/45 (24.4) | |||||
Down | 43/45 (95.6) | 13/45 (28.9) | |||||
ANC12W | 0.000 | 1.000 | 0.365 | 0.546 | |||
Up | 43/46 (93.5) | 11/46 (23.9) | |||||
Down | 42/44 (95.5) | 13/44 (29.5) | |||||
AMC12W | 1.048 | 0.306 | 2.737 | 0.98 | |||
Up | 39/43 (90.7) | 8/43 (18.6) | |||||
Down | 46/47 (97.9) | 16/47 (34.0) | |||||
ALC12W | 0.221 | 0.639 | 0.038 | 0.846 | |||
Up | 35/36 (97.2) | 10/36 (27.8) | |||||
Down | 50/54 (92.6) | 14/54 (25.9) | |||||
EON12W | 0.000 | 1.000 | 1.068 | 0.301 | |||
Up | 35/37 (94.6) | 12/37 (32.4) | |||||
Down | 50/53 (94.3) | 12/53 (22.6) | |||||
PLT12W | 0.131 | 0.717 | 1.060 | 0.303 | |||
Up | 35/38 (92.1) | 8/38 (21.1) | |||||
Down | 50/52 (96.2) | 16/52 (30.8) | |||||
LDH12W | 0.000 | 1.000 | 0.050 | 0.824 | |||
Up | 41/43 (95.3) | 11/43 (25.6) | |||||
Down | 44/47 (93.6) | 13/47 (27.7) | |||||
Hb12W | 0.000 | 1.000 | 0.000 | 1.000 | |||
Up | 43/45 (95.6) | 12/45 (26.7) | |||||
Down | 42/45 (93.3) | 12/45 (26.7) | |||||
Alb12W | 3.994 | 0.046 | 5.260 | 0.022 | |||
Up | 48/48 (100.0) | 8/48 (16.7) | |||||
Down | 37/42 (88.1) | 16/42 (38.1) | |||||
CEA12W | 0.000 | 1.000 | 5.682 | 0.017 | |||
Up | 42/45 (93.3) | 7/45 (15.6) | |||||
Down | 43/45 (95.6) | 17/45 (37.8) | |||||
TrxR12W | 3.696 | 0.049 | 0.299 | 0.584 | |||
Up | 48/53 (90.6) | 14/53 (26.4) | |||||
Down | 37/37 (100.0) | 10/37 (27.0) | |||||
NLR12W | 0.000 | 1.000 | 0.365 | 0.546 | |||
Up | 43/46 (93.5) | 11/46 (23.9) | |||||
Down | 42/44 (95.5) | 13/44 (29.5) | |||||
LMR12W | 1.158 | 0.282 | 6.173 | 0.013 | |||
Up | 47/48 (97.9) | 18/48 (37.5) | |||||
Down | 38/42 (90.5) | 6/42 (14.3) | |||||
PLR12W | 0.000 | 1.000 | 0.065 | 0.799 | |||
Up | 44/47 (93.6) | 12/47 (25.5) | |||||
Down | 43/41 (95.3) | 12/43 (27.9) | |||||
PAR12W | 1.533 | 0.216 | 0.454 | 0.501 | |||
Up | 35/39 (89.7) | 9/39 (23.1) | |||||
Down | 50/51 (98.0) | 15/51 (29.4) | |||||
dNLR12W | 0.000 | 1.000 | 0.490 | 0.484 | |||
Up | 44/47 (93.6) | 14/47 (29.8) | |||||
Down | 41/43 (95.3) | 10/43 (23.3) | |||||
PNI12W | 0.591 | 0.442 | 1.105 | 0.293 | |||
Up | 41/42 (97.6) | 9/42 (21.4) | |||||
Down | 44/48 (91.7) | 15/48 (31.3) |
WBC, white blood cell; ANC, absolute neutrophil count; AMC, absolute monocyte count; ALC, absolute lymphocyte count; EON, eosinophil number; PLT, platelet; LDH, lactate dehydrogenase; Hb, hemoglobin; Alb, albumin; CEA, carcinoembryonic antigen; TrxR, thioredoxin reductase; NLR, neutrophil-to-lymphocyte ratio; LMR, lymphocyte-to-monocyte ratio; PLR, platelet-to-lymphocyte ratio; PAR, platelet-to-albumin ratio; dNLR, derived NLR; PNI, prognostic nutritional index; DCR, disease control rate; ORR, objective response rate.
The chi-squared test showed that DCR was associated with decreased TrxR12w (χ2=3.696; P=0.049), decreased Alb12w (χ2=3.994; P=0.046), and decreased CEA6w (χ2=4.695; P=0.030), (Table 2), respectively. ORR was associated with WBC6w (χ2=11.012; P=0.001), ANC6w (χ2=12.007; P=0.001), NLR6w (χ2=5.260; P=0.022), dNLR6w (χ2=4.139; P=0.042), Alb12w (χ2=5.260; P=0.022), CEA12w (χ2=5.682; P=0.017), and LMR12w (χ2=6.173; P=0.013), respectively.
Clinical prediction model for PFS in patients with advanced NSCLC
The univariate and multivariate Cox regression analyses showed that types of pathology [hazard ratio (HR) =2.10; 95% confidence interval (CI): 1.237–3.576; P=0.006], ECOG PS of 1 (HR =0.10; 95% CI: 0.024–0.411; P=0.001), ECOG PS of 0 (HR =0.22; 95% CI: 0.062–0.794; P=0.021), CEA6w (HR =1.81; 95% CI: 1.091–3.003; P=0.022) were significantly associated with a prolonged PFS (Table 3). The univariate log-rank tests showed that patients with adenocarcinoma (HR =2.00; 95% CI: 1.200–3.200; P=0.008; Figure 2A), ECOG PS of 0 (HR =2.40; 95% CI: 1.200–5.000; P=0.001; Figure 2B) and CEA6w decrease (HR =1.80; 95% CI: 1.100–2.900; P=0.025; Figure 2C) had a longer PFS (Figure 2), respectively. Other variable values that were not statistically significant in association with PFS were listed in Table S3.
Table 3
Variables | Progression | ||||||
---|---|---|---|---|---|---|---|
Univariate | Multivariate | ||||||
HR | 95% CI | P value | HR | 95% CI | P value | ||
Types of pathology | 1.953 | 1.180–3.231 | 0.009* | 2.103 | 1.237–3.576 | 0.006* | |
ECOG PS | |||||||
2 | 1 | 1 | |||||
1 | 0.096 | 0.024–0.388 | 0.001* | 0.099 | 0.024–0.411 | 0.001* | |
0 | 0.177 | 0.052–0.601 | 0.006* | 0.221 | 0.062–0.794 | 0.021* | |
CEA6W | 1.758 | 1.066–2.900 | 0.027* | 1.810 | 1.091–3.003 | 0.022* | |
PLT12W | 1.754 | 1.044–2.918 | 0.034* | 1.607 | 0.995–2.703 | 0.074 |
*, P<0.05. PFS, progression-free survival; ECOG PS, Eastern Cooperative Oncology Group performance status; CEA, carcinoembryonic antigen; PLT, platelet; HR, hazard ratio; CI, confidence interval.

The PFS prediction model was validated using types of pathology, ECOG PS and CEA6w population (Figure 3A), and showed a C-index of 0.715 (95% CI: 0.685–0.745) and an AUC of 0.694 (95% CI: 0.667–0.704; Figure 3B). The 6- and 12-month calibration curves showed a good correlation between the actual and predicted outcomes (Figure 3C,3D). Patients with lower risk scores (50%) had a longer PFS (HR =0.33; 95% CI: 0.20–0.55; P<0.0001; Figure 3E). The relationship between risk scores and clinical characteristics were assessed, ECOG PS and best response to treatment were found to be correlated with the model’s risk score (Figure 4).
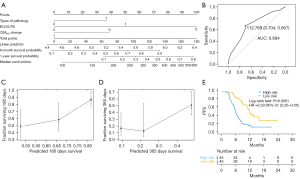
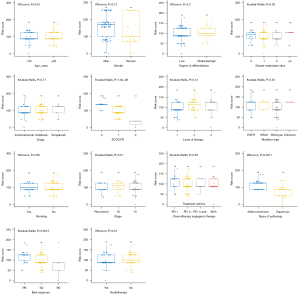
Clinical prediction model for OS in patients with advanced NSCLC
Table 4 summarizes the results of the univariate and multivariate Cox regression analyses for OS. The univariate and multivariate Cox regression analyses showed that types of pathology, ALC6w decreased, CEA6w decreased, PLT6w increased, TrxR6w decreased, PLR6w increased, PAR6w increased, WBC12w decreased, ANC12w decreased, PLT12w decreased, PLR12w decreased, and dNLR12w decreased were significantly associated with a prolonged OS. The univariate log-rank tests showed that patients with TrxR6w decreased (HR =3.402; 95% CI: 1.701–6.806; P=0.001; Figure 5A), ALC6w decreased (HR =2.182; 95% CI: 1.013–4.700; P=0.046; Figure 5B), and adenocarcinoma (HR =1.950; 95% CI: 1.044–3.642; P=0.0036; Figure 5C) had a longer OS (Figure 5). Other variable values that were not statistically significant in association with OS were listed in Table S3.
Table 4
Variables | Mortality | ||||||
---|---|---|---|---|---|---|---|
Univariate | Multivariate | ||||||
HR | 95% CI | P value | HR | 95% CI | P value | ||
Types of pathology | 1.824 | 1.017–3.274 | 0.044* | 1.950 | 1.044–3.642 | 0.036* | |
ALC6W | 1.950 | 1.132–3.360 | 0.016* | 2.182 | 1.013–4.700 | 0.046* | |
CEA6W | 1.936 | 1.121–3.343 | 0.018* | 1.634 | 0.793–3.369 | 0.183 | |
PLT6W | 0.527 | 0.299–0.930 | 0.027* | 0.387 | 0.111–1.351 | 0.137 | |
TrxR6W | 2.550 | 1.409–4.615 | 0.002* | 3.402 | 1.701–6.806 | 0.001* | |
PLR6W | 0.437 | 0.250–0.763 | 0.004* | 0.921 | 0.322–2.634 | 0.879 | |
PAR6W | 0.571 | 0.327–0.997 | 0.049* | 2.094 | 0.552–7.942 | 0.277 | |
WBC12W | 1.733 | 1.004–2.993 | 0.049* | 1.458 | 0.444–4.788 | 0.535 | |
ANC12W | 1.864 | 1.079–3.218 | 0.025* | 0.723 | 0.186–2.818 | 0.641 | |
PLT12W | 2.528 | 1.409–4.535 | 0.002* | 2.213 | 0.921–5.318 | 0.076 | |
PLR12W | 2.289 | 1.263–4.148 | 0.006* | 1.029 | 0.439–2.415 | 0.947 | |
dNLR12W | 1.801 | 1.040–3.118 | 0.036* | 1.786 | 0.693–4.602 | 0.230 |
*, P<0.05. OS, overall survival; ALC, absolute lymphocyte count; CEA, carcinoembryonic antigen; PLT, platelet; TrxR, thioredoxin reductase; PLR, platelet-to-lymphocyte ratio; PAR, platelet-to-albumin ratio; WBC, white blood cell; ANC, absolute neutrophil count; dNLR, derived NLR; HR, hazard ratio; CI, confidence interval.
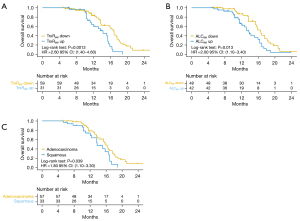
The OS prediction model resulted in a C-index of 0.671 (95% CI: 0.626–0.716; Figure 6A) and an AUC of 0.561 (95% CI: 0.556–0.574; Figure 6B). The 12- and 18-month calibration curves showed a good correlation between the actual and predicted outcomes (Figure 6C,6D). After calculating the risk score, 45 cases were classified as having a higher risk score and 45 cases were classified as having a lower risk score. Cases with lower risk scores had a longer OS (HR =0.49; 95% CI: 0.28–0.84; P=0.0089; Figure 6E). However, no correlation was found between any of the clinical characteristics and the model’s risk score (Figure 7).
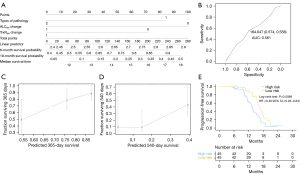
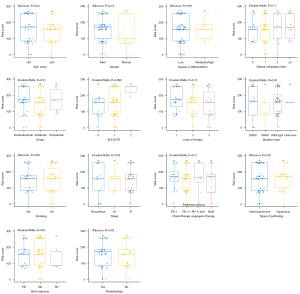
Discussion
Anti-PD-1-based immunotherapy plays an important role in the treatment of NSCLC (27,28). However, the lack of strong predictive markers of immune checkpoint inhibitor efficacy is still a major issue, with patients exposed to the risk of immune-related adverse events not getting any survival benefit from these agents, without considering the high costs for healthcare systems. This emphasizes the need to identify appropriate immune checkpoint inhibitor biomarkers. The TrxR is a redox system link that is closely associated with tumor development and alteration of the tumor microenvironment leading to disease progression (29,30). Previous studies have identified that CEA, Alb, WBC, ANC, NLR, dNLR, and LMR are associated with immunotherapy response in patients with advanced NSCLC (31-35). However, to our knowledge, this was the first study to evaluate the association between TrxR and the efficacy of PD-1-based immunotherapy in the management of advanced NSCLC.
Our findings revealed that a decrease in TrxR post-treatment was associated with better treatment outcome and prognosis in NSCLC patients after PD-1 inhibitor-based immunotherapy. Age, change in CEA6w, Alb12w, and TrxR12w were shown to be associated with DCR, while change in WBC6w, ANC6w, NLR6w, dNLR6w, Alb12w, CEA12w, and LMR12w were associated with ORR. The cut-off for TrxR were determined by ROC, which were relied on the data we collected and lacked uniform criteria for grouping. In addition, limited by sample size, we did not perform external validation of the validation cohort, only internal validation of the model.
The TrxR is a reduced coenzyme II (NADPH)-dependent dimeric selenase containing the flavin adenine dinucleotide (FAD) structural domains TrxR1, TrxR2, and TrxR3, whose main function is to catalyze NADPH and maintain reduction of the small molecule Trx (18). TrxR, together with Trx and NADPH, forms a potent protein reduction system called the Trx system, which plays an important role in the body’s redox regulation and antioxidant defense, cell proliferation, and signal transduction (36,37). Both Trx and TrxR have been found to be overexpressed in a variety of tumor tissues and oversecreted by tumor cells, which in turn stimulates tumor cell growth and prevent apoptosis (38). Given that TrxR can be inhibited by specific drugs, it is considered a promising target for cancer research. Furthermore, previous studies have reported that TrxR expression in tumor tissues is higher when compared with normal tissues (39,40). TrxR could be used to monitor abnormal cell proliferation or tumorigenesis in humans, and hence could potentially be used to identify tumors at an early stage (41,42). In lung cancer, TrxR is more frequently expressed in patients with poorly differentiated and larger tumors, and in those with lymph node metastases (43-45). In our study, we explored the association between TrxR, survival outcome and response to immunotherapy among patients with advanced NSCLC. Patients with decreased TrxR after treatment had a more favorable clinical outcome, which is consistent with previous research in gastric cancer patients (17). Unfortunately, we were not able to assess the relationship between TrxR and diagnosis of advanced NSCLC, as the TrxR levels are not routinely measured at the time of diagnosis in the participating oncology hospitals. Further research on TrxR and NSCLC diagnosis is warranted.
This study also investigated the association between peripheral blood parameters and prognosis treated with PD-1 inhibitor-based immunotherapy in advanced NSCLC patients. The blood parameters associated with PFS or OS in the multivariate Cox regression were used to build clinical prediction models, respectively. We also compared the risk scores with patient prognosis (Figures 3E,6E) to provide a more intuitive and effective view of the validity of the model scores. The model scores showed moderate prediction performance for both PFS and OS.
Our study had several limitations. The retrospective study design as well as the small number of cases included in the study limited the statistical power of analyses. In addition, the collection and analysis of TrxR and blood samples may vary between patient area, leading to batch effects that might compromise the validity of our findings. Furthermore, due to the retrospective nature of our study, we could not compare our findings with a control group of patients treated with chemotherapy alone. Another mayor issue is the lack of PD-L1 expression score of included patients. Indeed, although suboptimal, PD-L1 expression showed to some predictive role in NSCLC treated with immune checkpoint inhibitors (46). Moreover, our population was tested for EGFR and KRAS mutations only and we could not exclude that some included patients harbor other oncogenic alterations (i.e., anaplastic lymphoma kinase or ROS1 rearrangements) that may be negatively related to immunotherapy efficacy in NSCLC, especially when used as single-agents (47). Although we analyzed the ORR and DCR, the small number of events did not allow us to perform a multivariate regression analysis on treatment responses, which highlights the need for extended monitoring. Our study had a limited sample size, so we could not externally validate the model, and as a result, we will continue to accumulate and build a better clinical prediction model in subsequent studies. Finally, the 6- and 12-week check points for hematological parameters were arbitrarily chosen, and thus may not be optimal.
Conclusions
In summary, several peripheral blood markers, including TrxR, can be used to evaluate the response to immunotherapy in patients with advanced NSCLC. Therefore, repeated measurements of peripheral blood markers during treatment may help physicians to response to immunotherapy and clinical outcome.
Acknowledgments
We are grateful to all participants and their families. The authors appreciate the academic support from the AME Lung Cancer Collaborative Group.
Funding: This work was supported by the National Science Foundation of China (Nos. 81972313 and 81972822), Bethune Charitable Foundation (No. G-X-2019-0101-12), and Hui Lan Public Foundation (No. HL-HS-2020102).
Footnote
Reporting Checklist: The authors have completed the REMARK reporting checklist. Available at https://tlcr.amegroups.com/article/view/10.21037/tlcr-22-300/rc
Data Sharing Statement: Available at https://tlcr.amegroups.com/article/view/10.21037/tlcr-22-300/dss
Conflicts of Interest: All authors have completed the ICMJE uniform disclosure form (available at https://tlcr.amegroups.com/article/view/10.21037/tlcr-22-300/coif). GS received payment from Takeda Pharmaceutical Co. Advisory board. PB received honoraria from AstraZeneca, BeiGene, Bristol Meyers Squibb, Roche, Takeda; he received support for virtual meeting registration from Amgen, Daiichi Sankyo; he received institutional research grants from Roche, Pfizer. He did not receive support for the present work. The other authors have no conflicts of interest to declare.
Ethical Statement: The authors are accountable for all aspects of the work in ensuring that questions related to the accuracy or integrity of any part of the work are appropriately investigated and resolved. The study was conducted in accordance with the Declaration of Helsinki (as revised in 2013). The study was approved by institutional Ethics Committee of Jiangsu Cancer hospital. Individual consent for this retrospective analysis was waived.
Open Access Statement: This is an Open Access article distributed in accordance with the Creative Commons Attribution-NonCommercial-NoDerivs 4.0 International License (CC BY-NC-ND 4.0), which permits the non-commercial replication and distribution of the article with the strict proviso that no changes or edits are made and the original work is properly cited (including links to both the formal publication through the relevant DOI and the license). See: https://creativecommons.org/licenses/by-nc-nd/4.0/.
References
- Bray F, Laversanne M, Weiderpass E, et al. The ever-increasing importance of cancer as a leading cause of premature death worldwide. Cancer 2021;127:3029-30. [Crossref] [PubMed]
- Sung H, Ferlay J, Siegel RL, et al. Global Cancer Statistics 2020: GLOBOCAN Estimates of Incidence and Mortality Worldwide for 36 Cancers in 185 Countries. CA Cancer J Clin 2021;71:209-49. [Crossref] [PubMed]
- Planchard D, Popat S, Kerr K, et al. Metastatic non-small cell lung cancer: ESMO Clinical Practice Guidelines for diagnosis, treatment and follow-up. Ann Oncol 2018;29:iv192-237. [Crossref]
- Zou W, Wolchok JD, Chen L. PD-L1 (B7-H1) and PD-1 pathway blockade for cancer therapy: Mechanisms, response biomarkers, and combinations. Sci Transl Med 2016;8:328rv4. [Crossref] [PubMed]
- Ansell SM, Lesokhin AM, Borrello I, et al. PD-1 blockade with nivolumab in relapsed or refractory Hodgkin's lymphoma. N Engl J Med 2015;372:311-9. [Crossref] [PubMed]
- Duma N, Santana-Davila R, Molina JR. Non-Small Cell Lung Cancer: Epidemiology, Screening, Diagnosis, and Treatment. Mayo Clin Proc 2019;94:1623-40. [Crossref] [PubMed]
- Camidge DR, Doebele RC, Kerr KM. Comparing and contrasting predictive biomarkers for immunotherapy and targeted therapy of NSCLC. Nat Rev Clin Oncol 2019;16:341-55. [Crossref] [PubMed]
- Hofman P, Heeke S, Alix-Panabières C, et al. Liquid biopsy in the era of immuno-oncology: is it ready for prime-time use for cancer patients? Ann Oncol 2019;30:1448-59. [Crossref] [PubMed]
- Bodor JN, Boumber Y, Borghaei H. Biomarkers for immune checkpoint inhibition in non-small cell lung cancer (NSCLC). Cancer 2020;126:260-70. [Crossref] [PubMed]
- Ottonello S, Genova C, Cossu I, et al. Association Between Response to Nivolumab Treatment and Peripheral Blood Lymphocyte Subsets in Patients With Non-small Cell Lung Cancer. Front Immunol 2020;11:125. [Crossref] [PubMed]
- Seban RD, Assié JB, Giroux-Leprieur E, et al. Prognostic value of inflammatory response biomarkers using peripheral blood and 18F-FDG PET/CT in advanced NSCLC patients treated with first-line chemo- or immunotherapy. Lung Cancer 2021;159:45-55. [Crossref] [PubMed]
- Lim JY, Yoon SO, Hong SW, et al. Thioredoxin and thioredoxin-interacting protein as prognostic markers for gastric cancer recurrence. World J Gastroenterol 2012;18:5581-8. [Crossref] [PubMed]
- Zheng X, Ma W, Sun R, et al. Butaselen prevents hepatocarcinogenesis and progression through inhibiting thioredoxin reductase activity. Redox Biol 2018;14:237-49. [Crossref] [PubMed]
- Mohammadi F, Soltani A, Ghahremanloo A, et al. The thioredoxin system and cancer therapy: a review. Cancer Chemother Pharmacol 2019;84:925-35. [Crossref] [PubMed]
- Zhu B, Ren C, Du K, et al. Olean-28,13b-olide 2 plays a role in cisplatin-mediated apoptosis and reverses cisplatin resistance in human lung cancer through multiple signaling pathways. Biochem Pharmacol 2019;170:113642. [Crossref] [PubMed]
- Cattaruzza L, Fregona D, Mongiat M, et al. Antitumor activity of gold(III)-dithiocarbamato derivatives on prostate cancer cells and xenografts. Int J Cancer 2011;128:206-15. [Crossref] [PubMed]
- Peng W, Zhou Z, Zhong Y, et al. Plasma activity of Thioredoxin Reductase as a Novel Biomarker in Gastric Cancer. Sci Rep 2019;9:19084. [Crossref] [PubMed]
- Powis G, Kirkpatrick DL. Thioredoxin signaling as a target for cancer therapy. Curr Opin Pharmacol 2007;7:392-7. [Crossref] [PubMed]
- Pekkari K, Goodarzi MT, Scheynius A, et al. Truncated thioredoxin (Trx80) induces differentiation of human CD14+ monocytes into a novel cell type (TAMs) via activation of the MAP kinases p38, ERK, and JNK. Blood 2005;105:1598-605. [Crossref] [PubMed]
- Lei H, Wang G, Zhang J, et al. Inhibiting TrxR suppresses liver cancer by inducing apoptosis and eliciting potent antitumor immunity. Oncol Rep 2018;40:3447-57. [Crossref] [PubMed]
- Li C, Peng Y, Mao B, et al. Thioredoxin reductase: a novel, independent prognostic marker in patients with hepatocellular carcinoma. Oncotarget 2015;6:17792-804. [Crossref] [PubMed]
- Dong C, Zhang L, Sun R, et al. Role of thioredoxin reductase 1 in dysplastic transformation of human breast epithelial cells triggered by chronic oxidative stress. Sci Rep 2016;6:36860. [Crossref] [PubMed]
- Lathrop K, Kaklamani V. The Response Evaluation Criteria in Solid Tumors (RECIST). In: Badve S, Kumar G. editors. Predictive Biomarkers in Oncology. Cham: Springer, 2019.
- Riley RD, Ensor J, Snell KIE, et al. Calculating the sample size required for developing a clinical prediction model. BMJ 2020;368:m441. [Crossref] [PubMed]
- Concato J, Peduzzi P, Holford TR, et al. Importance of events per independent variable in proportional hazards analysis. I. Background, goals, and general strategy. J Clin Epidemiol 1995;48:1495-501. [Crossref] [PubMed]
- Peduzzi P, Concato J, Kemper E, et al. A simulation study of the number of events per variable in logistic regression analysis. J Clin Epidemiol 1996;49:1373-9. [Crossref] [PubMed]
- Dantoing E, Piton N, Salaün M, et al. Anti-PD1/PD-L1 Immunotherapy for Non-Small Cell Lung Cancer with Actionable Oncogenic Driver Mutations. Int J Mol Sci 2021;22:6288. [Crossref] [PubMed]
- Ren S, Xiong X, You H, et al. The Combination of Immune Checkpoint Blockade and Angiogenesis Inhibitors in the Treatment of Advanced Non-Small Cell Lung Cancer. Front Immunol 2021;12:689132. [Crossref] [PubMed]
- Wu W, Yang Z, Xiao X, et al. A thioredoxin reductase inhibitor ethaselen induces growth inhibition and apoptosis in gastric cancer. J Cancer 2020;11:3013-9. [Crossref] [PubMed]
- Liu L, Zhou X, Zheng R, et al. Self-delivery nanomedicine for chemotherapy sensitized photodynamic therapy. Chem Commun (Camb) 2021;57:7296-9. [Crossref] [PubMed]
- Dal Bello MG, Filiberti RA, Alama A, et al. The role of CEA, CYFRA21-1 and NSE in monitoring tumor response to Nivolumab in advanced non-small cell lung cancer (NSCLC) patients. J Transl Med 2019;17:74. [Crossref] [PubMed]
- Takada K, Takamori S, Yoneshima Y, et al. Serum markers associated with treatment response and survival in non-small cell lung cancer patients treated with anti-PD-1 therapy. Lung Cancer 2020;145:18-26. [Crossref] [PubMed]
- Sumiyoshi I, Okabe T, Togo S, et al. High lymphocyte population-related predictive factors for a long-term response in non-small cell lung cancer patients treated with pemetrexed: a retrospective observational study. J Transl Med 2021;19:92. [Crossref] [PubMed]
- Soda H, Ogawara D, Fukuda Y, et al. Dynamics of blood neutrophil-related indices during nivolumab treatment may be associated with response to salvage chemotherapy for non-small cell lung cancer: A hypothesis-generating study. Thorac Cancer 2019;10:341-6. [Crossref] [PubMed]
- Thor M, Montovano M, Hotca A, et al. Are unsatisfactory outcomes after concurrent chemoradiotherapy for locally advanced non-small cell lung cancer due to treatment-related immunosuppression? Radiother Oncol 2020;143:51-7. [Crossref] [PubMed]
- Nakamura H, Bai J, Nishinaka Y, et al. Expression of thioredoxin and glutaredoxin, redox-regulating proteins, in pancreatic cancer. Cancer Detect Prev 2000;24:53-60. [PubMed]
- Selenius M, Rundlöf AK, Olm E, et al. Selenium and the selenoprotein thioredoxin reductase in the prevention, treatment and diagnostics of cancer. Antioxid Redox Signal 2010;12:867-80. [Crossref] [PubMed]
- Tonissen KF, Di Trapani G. Thioredoxin system inhibitors as mediators of apoptosis for cancer therapy. Mol Nutr Food Res 2009;53:87-103. [Crossref] [PubMed]
- Ye SF, Li J, Ji SM, et al. Dose-biomarker-response modeling of the anticancer effect of ethaselen in a human non-small cell lung cancer xenograft mouse model. Acta Pharmacol Sin 2017;38:223-32. [Crossref] [PubMed]
- Zhu X, Huang C, Peng B. Overexpression of thioredoxin system proteins predicts poor prognosis in patients with squamous cell carcinoma of the tongue. Oral Oncol 2011;47:609-14. [Crossref] [PubMed]
- Baker A, Payne CM, Briehl MM, et al. Thioredoxin, a gene found overexpressed in human cancer, inhibits apoptosis in vitro and in vivo. Cancer Res 1997;57:5162-7. [PubMed]
- Becker K, Gromer S, Schirmer RH, et al. Thioredoxin reductase as a pathophysiological factor and drug target. Eur J Biochem 2000;267:6118-25. [Crossref] [PubMed]
- Soini Y, Kahlos K, Näpänkangas U, et al. Widespread expression of thioredoxin and thioredoxin reductase in non-small cell lung carcinoma. Clin Cancer Res 2001;7:1750-7. [PubMed]
- Skogastierna C, Johansson M, Parini P, et al. Statins inhibit expression of thioredoxin reductase 1 in rat and human liver and reduce tumour development. Biochem Biophys Res Commun 2012;417:1046-51. [Crossref] [PubMed]
- Zhang W, Zheng X, Wang X. Oxidative stress measured by thioredoxin reductase level as potential biomarker for prostate cancer. Am J Cancer Res 2015;5:2788-98. [PubMed]
- Bie F, Tian H, Sun N, et al. Research Progress of Anti-PD-1/PD-L1 Immunotherapy Related Mechanisms and Predictive Biomarkers in NSCLC. Front Oncol 2022;12:769124. [Crossref] [PubMed]
- Mazieres J, Drilon A, Lusque A, et al. Immune checkpoint inhibitors for patients with advanced lung cancer and oncogenic driver alterations: results from the IMMUNOTARGET registry. Ann Oncol 2019;30:1321-8. [Crossref] [PubMed]