A narrative review of deep learning applications in lung cancer research: from screening to prognostication
Introduction
Lung cancer is the leading cause of cancer mortality and the second most common type of newly diagnosed cancer worldwide, with 2.2 and 1.8 million patients being diagnosed and dying in 2020, respectively (1-3). In the past decades, lung cancer research has led to the development of various diagnostic and therapeutic options and methods capable of accurately predicting patients’ prognoses and treatment outcomes (4,5). Thanks to progress in lung cancer research, patients with lung cancer have a longer life expectancy and higher quality of life than was previously the case, without significant physical health consequences (6). The number of newly diagnosed lung cancers has continued to decline, as has mortality due to lung cancer; as a result, the 5-year relative survival rate has improved to 21.7% (2,7).
In recent years, deep learning (DL) techniques have staked out a place in various fields of medicine, and lung cancer research is no exception (8-12). Specifically, DL techniques have been actively applied to detect lung nodules in chest radiographs or computed tomography (CT) examinations, optimize candidate selection for lung cancer screening (LCS), predict the malignancy of lung nodules, stage lung cancer, and predict treatment response, patients’ prognoses, and genetic mutations in lung cancers (13,14). DL algorithms have often shown comparable or higher performance than human experts in the aforementioned clinical settings (13,14).
This narrative review presents an overview of the DL algorithms that have been applied in lung cancer research according to their clinical use cases. In addition, we discuss their strengths and limitations, and highlight future directions of lung cancer research using DL techniques. We present the article in accordance with the Narrative Review reporting checklist (available at https://tlcr.amegroups.com/article/view/10.21037/tlcr-21-1012/rc).
Methods
Search strategy and terminology
We searched the Embase and OVID-MEDLINE databases to identify relevant publications with combinations of the following search terms: deep learning, machine learning, artificial intelligence, lung cancer, lung malignancy, image, CT, computed tomography, and chest radiographs. The timeframe for the search was from October 2016 until September, 2021. An additional review of the bibliographies of key references was performed to ensure that all important literature was included. We only reviewed articles published in English without any limits on the publication year (Table 1).
Table 1
Items | Specification |
---|---|
Date of search | September 29, 2021 |
Databases and other sources searched | Embase and OVID-MEDLINE databases |
Search terms used | Search terms: deep learning, machine learning, artificial intelligence, lung cancer, lung malignancy, image, CT, computed tomography, and chest radiographs |
Search strategy of Embase and OVID-MEDLINE database: (deep learning OR machine learning OR artificial intelligence) AND (lung cancer OR lung malignancy) AND (image OR CT OR computed tomography OR chest radiographs) | |
Timeframe | From October, 2016 until September, 2021 |
Inclusion and exclusion criteria | • Inclusion criteria: |
(I) English-language article; | |
(II) Article types were randomized controlled trials, prospective or retrospective cohort studies, and case-control studies | |
• Exclusion criteria: | |
(I) Article not published in English | |
(II) Article types were editorial comments, abstracts, conference materials, case reports or series, review articles, guidelines, consensus statements, or study protocol | |
Selection process | Study selection and full-text articles were assessed by two authors in consensus (Jong Hyuk Lee and Chang Min Park) |
Any additional considerations, if applicable | None |
The terminology of the datasets discussed in this review article is defined as follows: a development dataset consists of training, validation, and internal test sets (15,16). We defined training and validation datasets as data used to train and optimize the parameters for a model (training dataset) and to monitor and search for the best performance (validation dataset) (15,16). An internal test dataset was defined as data used to evaluate the performance of a DL model by using previous questions in the training and validation dataset (15,16). Therefore, the internal test may substantially overestimate the performance of a DL algorithm (15,16). In contrast, an external test dataset is defined as separate data not used for model development, and it is of vital importance to demonstrate a model’s robustness and generalizability (15-18).
DL applications in lung cancer research
The following paragraphs deal with the details of DL algorithms applied to lung cancer research: lung nodule detection on chest radiographs, lung cancer screening with low-dose CT, malignancy prediction for indeterminate lung nodules, lung cancer staging, prognostication of patients with lung cancer, prediction of treatment response, and prediction of genetic mutations in lung cancer (Figure 1).
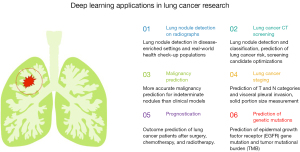
Lung nodule detection on chest radiographs
LCS should be implemented using a screening tool with a high detection performance for pulmonary nodules or masses in high-risk individuals (19-22). In this regard, although chest radiography is one of the most common diagnostic imaging examinations (23), it has not been considered the optimal tool for lung cancer screening (20,24). The sensitivity of chest radiography for detecting lung cancer has been reported to be highly variable, ranging from 20% to 92%, with radiologists’ perceptual errors being the most common cause of failure to diagnose lung cancer on chest radiographs (25-28). The application of a computer-assisted detection (CADe) system for lung nodules on chest radiographs has been reported to improve sensitivity (29,30), which may be a game-changer for lung nodule detection tasks.
Nam et al. developed a DL-based automatic detection algorithm with 43,292 chest radiographs and externally tested it for malignant nodule detection (31). They reported that the DL algorithm achieved a sensitivity of 71–91%, a specificity of 93–100%, and an area under the receiver operating characteristic (ROC) curve (AUC) of 0.92–0.99 in their external test datasets, which were superior to those of most physicians in the reader study (31). Furthermore, all physicians showed improvement in lung nodule detection performance with assistance of the DL algorithm, as demonstrated by a 0.043 mean enhancement of the Jackknife alternative free-response ROC figure of merit (31). Sim et al. performed a similar task of detecting malignant lung nodules on chest radiographs with another commercially available DL software (Auto Lung Nodule Detection, version 1.00, Samsung Electronics) (32). Using the DL algorithm improved radiologists’ sensitivity from 65.1% to 70.3% and decreased the false-positive (FP) findings per image from 0.2 to 0.18 for detecting lung cancer (32). These studies suggested the potential of DL technology for lung cancer detection on chest radiographs. However, they had clear limitations in that their test datasets were composed of disease-enriched datasets, not reflecting real-world clinical practice (with lung cancer prevalence of 60–68% and 75% in the studies of Nam et al. and Sim et al., respectively) (31,32).
Thereafter, Lee et al. deployed a commercially available DL algorithm (Lunit Insight, version 4.7.2., Lunit) for lung nodule detection in Korea’s real-world health check-up population with a lung cancer prevalence of 0.2% among 50,098 individuals (33). The model showed comparable sensitivity and negative predictive value to that of board-certified radiologists, with only 3% of chest radiographs having potentially positive results (33). These results suggested the possibility of using the DL algorithm as a stand-alone or first screening tool in resource-constrained environments where there is often a lack of trained radiologists (34).
Lung cancer screening with low-dose CT
The National Lung Screening Trial (NLST) revealed that low-dose chest CT (LDCT) LCS could reduce lung cancer mortality in high-risk populations by 20%, leading to the recommendation of LCS with LDCT for high-risk populations (19). Since then, nationwide screening programs have been implemented in multiple countries worldwide (19,21,35,36). However, the large volume of LDCT scans in LCS imposes a substantial burden on radiologists, and the high FP rate is another problem, leading to unnecessary diagnostic tests and invasive diagnostic procedures (19,22,35,37-41). To address this issue, a CADe system with LDCT has been introduced. However, before applying the DL technique, the CADe system showed unsatisfactory detection performance (sensitivity of 70% or lower) for lung nodules and a substantially high FP rate, which was insufficient for clinical implementation (42-44). In addition, CADe continues to serve only as the second reader, it is uncertain whether CADe will eventually improve clinical care efficiency (45,46).
Performance improvements have recently been achieved with the application of convolutional neural network (CNN)-based DL models (47-49). In 2016, the LUng Nodule Analysis (LUNA) challenge was held for the purpose of nodule detection and FP reduction based on 888 annotated images (49). The best model that participated in this challenge achieved a sensitivity of 93%, and the combined models had a sensitivity of 95% at an FP rate of lower than 1 per scan (49). Since the introduction of the DL technique, ongoing efforts have been made to work towards the clinical application of this state-of-the-art system d (46). Li et al. tested a commercially available DL-based CADe tool (DL-CAD) in 346 LCS participants (50). The DL-CAD had a significantly higher detection rate of lung nodules than that of double reading by two thoracic radiologists (86.2% vs. 79.2%) with an FP rate of 1.53 per CT examination. However, although this FP rate was significantly lower than that of CADe without the DL technique (e.g., 17 per CT examination) (51), it was still higher compared to that of the radiologists (0.13 per CT scan) (50); thus, further research to reduce the FP rate is warranted.
Ciompi et al. trained a DL algorithm to classify pulmonary nodules into solid, calcified, part-solid, non-solid, perifissural, and spiculated nodules, using a dataset of 943 subjects with 1,805 nodules from the Multicentric Italian Lung Detection (MILD) trial (52). Subsequently, they tested this DL algorithm using 468 subjects with 639 nodules from the Danish Lung Cancer Screening Trial (DLCST), and 162 nodules of those nodules were included in the reader performance test. For the task of classifying nodules into the six categories listed above, the DL system had comparable agreement (Cohen’s kappa value, 0.54–0.67 for the DL algorithm vs. 0.59–0.75 for the human readers) and classification performance (69.6% for the algorithm vs. 72.9% for the human readers) to the four human readers. For all 639 nodules in the external test set, the algorithm showed a positive predictive value and sensitivity of 89.2% and 82.2%, 88.9% and 82.8%, 43.6% and 64.9%, 87.4% and 87.4%, 78.4% and 60.4%, and 32.7% and 54.3% for solid, calcified, part-solid, non-solid, perifissural, and spiculated nodules, respectively. Despite the relatively low detection performance of part-solid nodules, this study suggested that a DL system can help consistently classify nodule type, which determines the management strategy for LCS participants (53).
Ardila et al. constructed a three-dimensional CNN model that performed end-to-end analyses of whole LDCT volumes for lung cancer detection (54). In the internal test set composed of NLST data, the model had AUCs of 0.944 and 0.873 for predicting the risk of lung cancer in 1 year and 2 years, respectively (54). In the reader study with the external dataset, the model had higher sensitivity and specificity for lung cancers than radiologists when only a single LDCT was available and comparable diagnostic performance when serial LDCTs (prior and current LDCTs) were available (54).
Meanwhile, Huang et al. proposed a DL algorithm (DeepLR) for predicting the 3-year lung cancer risk after two screening CT examinations, using the NLST datasets as a training cohort and the Pan-Canadian Early Detection of Lung Cancer (PanCan) study as an external test cohort (55). It demonstrated good discrimination, with 1-year, 2-year, and 3-year time-dependent AUC values for cancer diagnosis of 0.968, 0.946, and 0.899, respectively (55). Furthermore, individuals categorized as high risk by the DeepLR had a higher risk of lung cancer diagnosis and mortality (55). Based on these results, the authors asserted the potential that a DL algorithm could be used for accurate guidance of clinical management after two consecutive screening CT scans (55).
Other than lung nodule detection or classification, a DL algorithm (CXR-LC) to identify high-risk candidates for LCS was recently developed and tested by Lu et al., for which the authors used the NLST and Prostate, Lung, Colorectal, and Ovarian Cancer Screening (PLCO) Trial datasets (56). Interestingly, the model used only easily obtainable inputs (e.g., age, sex, smoking status, and a chest radiograph image) and showed a significantly higher AUC value (0.755 vs. 0.634) and sensitivity (74.9% vs. 63.8%) than the Centers for Medicare & Medicaid Services (CMS) eligibility criteria, while missing 30.7% fewer incident lung cancers than the CMS eligibility criteria (56).
Indeed, sufficient test steps with external datasets, which are independent of the model development and reflect daily clinical practice, are indispensable to guarantee the generalizability and applicability of models in clinical practice (57,58). In this regard, a limitation of these previous studies is that they were based on publicly available datasets (e.g., the NLST or PLCO datasets) (54-56). Future research should include external validation studies applying these DL models in a cohort including heterogeneous races from a variety of countries.
Malignancy prediction for indeterminate lung nodules
Early diagnosis for lung cancer can indubitably improve patients’ outcomes and reduce lung cancer mortality (59). However, since most detected pulmonary nodules are benign regardless of the source of the detection (e.g., LCS CT or an incidental finding in an unrelated examination) (19,21), most of these indeterminate lung nodules without a malignancy risk-based optimization approach receive unnecessary diagnostic work-ups involving invasive procedures (e.g., needle biopsy or surgery), leading to increased medical expenditures (19,22,35,60). Conversely, however, an overly conservative approach may delay or miss the diagnosis, leading to upstaged cancers (36-38,61). The current management options for indeterminate pulmonary nodules are based on qualitative or quantitative estimates of the malignancy risk of those nodules (53,62-64). The prime examples are Lung-RADS by the American College of Radiology, guidelines by the Fleischner Society and British Thoracic Society, and logistic regression-based models (e.g., the Brock or Mayo risk models) (53,62-64).
Massion et al. addressed this issue using a DL model (LCP-CNN) that was developed to classify benign and malignant nodules from indeterminate pulmonary nodules (65). In an internal test with the NLST dataset, the model had a significantly higher AUC (0.921) than those of the Brock model (0.856) and Mayo model (0.852). In an external test with two independent cohorts, the model showed comparable or higher AUCs than those of the Mayo model (0.919 vs. 0.819 and 0.835 vs. 0.781, respectively). The authors additionally reported the results of the two-way reclassification analysis by calculating the fraction of cancers classified by the LCP-CNN model and the Mayo model. As a result, the overall net reclassifications for cancer and benign nodules in the two external test cohorts were as high as 0.34 and 0.58 compared to the Mayo model. That is, nodules that were malignant but classified as benign, and those that were benign but classified as malignant by the Mayo model were more accurately reclassified by the LCP-CNN model as malignant and benign, respectively. Finally, the LCP-CNN model showed a sensitivity of 96.8–98.4% and specificity of 44.2–64.3% with a model threshold of 5%, indicating higher specificity and comparable sensitivity to those of the Mayo model (sensitivity, 98.4–100%; specificity, 3–11.5%), and sensitivity of 36.5–70.3% and specificity of 78.8–97.5% with a model threshold of 65%, showing higher sensitivity and comparable specificity to those of the Mayo model (sensitivity, 4.8–25%; specificity, 90.4–99.8%). It is noteworthy that the LCP-CNN model was externally validated with three hospitals from the UK in another study (66). For 1,187 patients with indeterminate pulmonary nodules with a lung cancer prevalence of 19.3%, the LCP-CNN showed a significantly higher AUC (0.896) than that of the Brock model (0.868). The model also had higher discrimination performance for malignancy and lower false-negative (FN) rates than the Brock model.
Ohno et al. approached this issue by calculating the volume change and volume doubling time of pulmonary nodules, assisted by the DL technique applied to computer-aided detection of volume (CADv) measurements (67). They reported that the AUC and accuracy of total volume change per day calculated by the CADv with the DL method (AUC, 0.94; accuracy, 90%) were significantly higher than those of the volume doubling times with CADv using the DL method (AUC, 0.67; accuracy, 83%), and CADv not using the DL method (total volume change per day: AUC, 0.69 and accuracy, 67%; volume doubling time: AUC, 0.58, and accuracy, 65%) (67).
Lung cancer staging
As with other types of cancer, lung cancer staging is essential for planning the treatment strategy, predicting the prognosis, and evaluating treatment results (5). Accurate staging leads to survival benefits by multidisciplinary treatment combining surgery and chemoradiation therapy (68).
In most cases, subsolid lung nodules that persistently present in chest CT examinations pathologically represent preinvasive lesions, such as atypical adenomatous hyperplasia (AAH) or adenocarcinoma in situ (AIS), or lung cancers such as minimally invasive adenocarcinoma (MIA) or invasive adenocarcinoma (53,69). Among these categories, AIS and MIA are staged as T categories of Tis and Tmi according to the eighth-edition staging system (70,71). Prior studies have investigated the use of DL algorithms to discriminate among these entities and identify early-stage invasive adenocarcinoma (72,73). Zhao et al. developed their DL algorithm model (DenseSharp Network) to differentiate the AAH-AIS group, MIA group, and invasive adenocarcinoma group with 651 nodules ≤10 mm in size (72). They conducted an external test with 128 pathologically proven nodules and compared the model’s diagnostic performance with that of four radiologists. The model showed a higher F1 score [defined as the harmonic mean of the precision and recall; F1 score = 2 · precision · recall/(precision + recall)] than that of four radiologists for the task of three-group classification (AAH-AIS vs. MIA vs. invasive adenocarcinoma groups). The DL algorithm achieved AUCs of 0.788 and 0.880 for the subtasks of classification of the invasive adenocarcinoma-MIA group from the AAH-AIS group and the invasive adenocarcinoma group from the AAH-AIS-MIA group, respectively (72).
The eighth-edition staging system by the American Joint Commission on Cancer adopted the solid portion size on CT and the invasive component size on pathology to determine the clinical and pathologic T category, respectively, because the solid portion size or invasive component size is a better prognostic predictor than the total tumor size (70,71,74). Ahn et al. externally tested a commercially available DL algorithm (MedLungCT AI, version 1.0.0; VUNO) to segment the entire nodule and solid portion of subsolid nodules using 448 patients with surgically resected lung adenocarcinomas (75). They found that the inter-reader agreement between the radiologists and the MedLung CT AI was good [intraclass correlation coefficient (ICC) range, 0.82–0.89] and was par on with the agreement level between the radiologists (76). Both the algorithm and radiologists commonly had a tendency to underestimate the invasive portion size relative to the pathologically proven invasive component size (75).
Visceral pleural invasion (VPI) by lung cancer is an isolated T2 descriptor due to its adverse prognostic implication after adjustment for the pathologic T category (77). Choi et al. developed an in-house DL algorithm to predict VPI, using 676 patients with clinical stage 1A lung adenocarcinoma (78). In an external test consisting of 141 patients, the model had an AUC of 0.75 for VPI, comparable to the three thoracic radiologists’ evaluations (AUC range, 0.73–0.79). At the cutoffs that showed 90% sensitivity and specificity in the internal test set, the algorithm had comparable to higher sensitivity and higher specificity than the radiologists (78). Finally, the model’s output was an independent predictor for VPI in multivariate logistic regression in conjunction with the clinical stage and nodule type (77,78).
The clinical significance of lung cancer with a nodal category of N2 or higher is that multidisciplinary management is offered due to its survival benefits (68). However, despite routine imaging workups including CT, positron emission tomography (PET), and endobronchial ultrasonography to correctly diagnose N2 disease before treatment, the sensitivity is still limited, and up to 8.5% of clinical N0 lung cancers were found to have N2 metastasis at pathologic examination (5,79,80). Recently, Zhong et al. developed a DL signature to predict N2 disease in clinical stage I non-small cell lung cancers (NSCLCs) using chest CT images of 2,663 patients (81). The authors performed rigorous external tests using open-source data (n=133) and CT datasets prospectively collected from four institutions (n=300). Notably, the prevalence of N2 disease in these external datasets, ranging from 10% to 10.7%, was similar to the real-world prevalence of N2 disease (80). The model had a significantly higher AUC (0.81) than those obtained using the three currently used clinical models (the Veterans Affairs model, Fudan model, and Beijing model; the range of AUCs, 0.61–0.68) and the maximum standardized uptake value on PET (AUC, 0.57). In addition, the authors suggested the biological basis for this DL signature by verifying the association of the model’s risk score with gene expression patterns [e.g., epidermal growth factor receptor (EGFR) or anaplastic lymphoma kinase mutations]. The fact that the authors investigated the association between the model findings and radio-genomics through a gene alteration analysis and gene set enrichment analysis helps readers and researchers understand the biological basis of the model and alleviate its black-box characteristics, which is one of the well-known drawbacks of DL techniques (82).
Prognostication of patients with lung cancer and prediction of treatment response
An accurate prediction of the prognosis of patients with lung cancer allows clinicians to evaluate tumor progression, facilitates communication between physicians and patients, and helps establish appropriate treatment strategies (74,83,84). Prognostic stratification and the consequent treatment strategy have been primarily determined based on cancer staging (85). However, even in patients with the same cancer stage, the prognosis varies due to heterogeneous treatment responses (86). To solve this issue, DL techniques have been investigated for predicting the prognosis of patients with lung cancer and their response to treatment.
Kim et al. developed a DL algorithm to extract prognostic information from preoperative CT examinations, using 800 patients with surgically resected T1-4N0M0 lung adenocarcinoma (87). In an external test with 108 patients with clinical stage I adenocarcinomas, the model’s probability had comparable prognostic performance to the clinical T category for disease-free survival. In addition, the output of the DL model was an independent prognostic factor for disease-free survival in conjunction with other clinical factors, including clinical T category and smoking status. Meanwhile, the DL signature developed by Zhong et al., which proposed risk scores for N2 disease, significantly stratified overall and recurrence-free survival in patients with clinical stage I NSCLCs (81). In the Cox regression analyses, the signature’s risk score turned out to be a significant prognostic factor for both overall survival and recurrence-free survival with other factors including age, sex, nodule type (subsolid nodule), and pathologic nodal stage. Their DL model also predicted the benefits of adjuvant chemotherapy in patients with moderate-to-high risk scores (81).
As for radiotherapy, Lou et al. developed a multi-task DL network (Deep Profiler) for predicting time-to-event treatment outcomes, using CT images of 849 patients who received stereotactic body radiotherapy for stage IA to IV lung cancers (88). In an external test with 95 patients, a high Deep Profiler score was a significant predictor of 3-year cumulative local treatment failure. In addition, a model combining the Deep Profiler score with clinical variables had better prediction performance than classical radiomics or clinical variable-based models alone. Notably, radiation dose reduction could be achieved in 23.3% of the patients with this combined model. Considering the current clinical practice in which radiotherapy continues to be delivered regardless of individual tumor characteristics, this study suggested that the DL algorithms could guide the individualization of radiotherapy (88). Another study proposed using DL algorithms, developed using CT images, to predict overall survival for patients with stage I to IIIb NSCLC who had radiotherapy or surgery (89). In external tests, the algorithms showed AUCs of 0.70 and 0.71 for the 2-year overall survival after each treatment, respectively, and significantly stratified patients’ survival probabilities according to the models’ output. The algorithms significantly outperformed a clinical model (using age, sex, and TNM stage) and a random forest model based on engineered features (using tumor shape, voxel intensity information, and patterns) and imaging parameters. Interestingly, the area that contributed most to survival prediction by the model’s activation mapping was the interface between the tumor and stoma, especially uninterrupted areas of higher CT density. The phenotypes captured by the algorithm were also correlated with the cell cycle and transcriptional processes (89).
Advanced lung cancers treated with nonsurgical modalities such as radiotherapy or chemotherapy require monitoring of the treatment response using follow-up imaging studies over time (70,90,91). This clinical response has been assessed using tumor size measurements, such as in the RECIST criteria (91). Regarding this point, using pre- and post-treatment CT images at 1, 3, and 6 months of follow-up in 179 patients with stage III NSCLC treated with chemoradiation, Xu et al. developed and tested a CNN model to predict various survival outcomes and cancer-specific events (i.e., 1-year, 2-year, and overall survival; progression; distant metastasis; and logo-regional recurrence) (90). The performance of the model for predicting these outcomes was continuously enhanced with additional CT images. For example, with pre-treatment CT alone, the model only showed an AUC of 0.58 for 2-year overall survival, and by adding post-treatment CT after 1, 3, and 6 months, the value was significantly increased to 0.64, 0.69, and 0.74, respectively. In addition, the model predicted the pathologic response after treatment and had added value to the simple pathologic volume change after radiation treatment.
Prediction of genetic mutations in lung cancer
EGFR genotyping is critical for determining the treatment strategy for patients with lung adenocarcinoma because EGFR tyrosine kinase inhibitors that target specific EGFR mutations have resulted in survival benefits (92-95). Although mutational sequencing of biopsy specimens is the gold standard for confirming EGFR mutation, tissue sampling is not always possible, and there may be potential risks of biopsy-related complications or irrelevant results from tissue sampling errors (96,97). To address this issue, Wang et al. developed and tested a DL algorithm to predict EGFR-mutant lung adenocarcinoma in 844 patients (development dataset, n=603; external test dataset, n=241) whose lung cancers’ EGFR mutation status had been proven (98). In the external test, the algorithm had an AUC of 0.81, which was significantly higher than those of clinical, CT semantic, and radiomics models (0.61, 0.64, and 0.64, respectively). Interestingly, the authors suggested that visualizing tumor areas that were identified as suspicious for EGFR mutation in the model might enable more accurate biopsy targeting, thereby avoiding FN results caused by intra-tumor heterogeneity (98).
The tumor mutational burden (TMB), which emerged through next-generation gene sequencing, is a predictor of NSCLC patients’ response to immune checkpoint inhibitors (99-101). However, as with other biomarkers, it requires invasive biopsy procedures and labor-intensive laboratory tests. To address this issue, He et al. developed a TMB radiomic biomarker (TMBRB) using the DL technique to distinguish high and low-TMB, using CT images of 327 patients with NSCLC (102). In an external test dataset composed of 123 NSCLC patients, the TMBRB discriminated high- and low-TMB with higher accuracy (AUC, 0.81) than the histologic subtype (AUC, 0.71) and radiomic model alone (AUC, 0.74). In addition, the discrimination of high- and low-TMB NSCLC by the TMBRB was a significant predictor of overall survival and progression-free survival. Based on these findings, the authors proposed a noninvasive biomarker for TMB based only upon CT images, which will help physicians decide whether to use immune checkpoint inhibitors.
Summary
In this review, we briefly summarized the various DL algorithms applied to lung cancer research to date, covering the detection of lung nodules on chest radiographs and LCS CT, malignancy prediction in indeterminate pulmonary nodules, lung cancer staging, treatment response prediction, prognostication, and prediction of genetic mutations in lung cancers. Numerous papers have reported promising and exciting results regarding the performance of DL algorithms. However, the following two issues should be addressed in the future. First, evidence is lacking on the eventual outcomes of applying the DL algorithms to real-world clinical practice. To confirm these outcomes, more rigorous real-world testing will be needed in settings covering various countries, races, and medical environments, and then, there must be a careful consideration of the advantages and disadvantages of applying DL models. Second, it is necessary to clearly define how to utilize DL models in heterogeneous clinical scenarios. Although it is commonly accepted that DL algorithms bring an added value thanks to their higher diagnostic or predictive performance (103,104), human-DL collaboration to achieve certain goals remains poorly understood (105,106), and further research is warranted to investigate this interaction to maximize the usefulness of the DL algorithms.
In conclusion, DL algorithms have demonstrated potential value for various tasks from lung cancer screening to prognostication of lung cancer patients. Future research is warranted to clarify the clinical application of these models in daily clinical practice and to verify their real-world clinical usefulness.
Acknowledgments
The authors would like to acknowledge Andrew Dombrowski, Ph.D. (Compecs, Inc.) for his assistance in improving the use of English in this manuscript.
Funding: This study was supported by the Seoul National University Hospital Research Fund (grant number: 06-2018-4520). However, the funder had no role in the study design; in the collection, analysis, and interpretation of the data; in the writing of the report; and in the decision to submit the article for publication.
Footnote
Reporting Checklist: The authors have completed the Narrative Review reporting checklist. Available at https://tlcr.amegroups.com/article/view/10.21037/tlcr-21-1012/rc
Conflicts of Interest: All authors have completed the ICMJE uniform disclosure form (available at https://tlcr.amegroups.com/article/view/10.21037/tlcr-21-1012/coif). EJH received research grants from Lunit, Coreline soft, and Monitor corportation. HK received a research grant from Lunit, and holds stock and stock options in Medical IP. CMP received research grants from Lunit and Coreline Soft, and holds stock in Promedius and stock options in Lunit and Coreline Soft. CMP serves as a current editorial board member of Translational Lung Cancer Research from September 2021 to August 2023. CMP is now a board member of the Big Data Review Board of Seoul National University Hospital and has leadership role in Korean Society of Radiology, Korean Society of Thoracic Radiology, Korean Society of Artificial Intelligence in Medicine. The other author has no conflicts of interest to declare.
Ethical Statement:
Open Access Statement: This is an Open Access article distributed in accordance with the Creative Commons Attribution-NonCommercial-NoDerivs 4.0 International License (CC BY-NC-ND 4.0), which permits the non-commercial replication and distribution of the article with the strict proviso that no changes or edits are made and the original work is properly cited (including links to both the formal publication through the relevant DOI and the license). See: https://creativecommons.org/licenses/by-nc-nd/4.0/.
References
- Sung H, Ferlay J, Siegel RL, et al. Global Cancer Statistics 2020: GLOBOCAN Estimates of Incidence and Mortality Worldwide for 36 Cancers in 185 Countries. CA Cancer J Clin 2021;71:209-49. [Crossref] [PubMed]
- National Cancer Institute. Surveillance, Epidemiology, and End Results Program, Cancer Stat Facts: Lung and Bronchus Cancer [cited 2021 Dec 19]. Available online: https://seer.cancer.gov/statfacts/html/lungb.html
- Ferlay J, Colombet M, Soerjomataram I, et al. Cancer statistics for the year 2020: An overview. Int J Cancer 2021; [Epub ahead of print]. [Crossref] [PubMed]
- Worley S. Lung cancer research is taking on new challenges: knowledge of tumors’ molecular diversity is opening new pathways to treatment. P T 2014;39:698-714. [PubMed]
- Rami-Porta R, Call S, Dooms C, et al. Lung cancer staging: a concise update. Eur Respir J 2018;51:1800190. [Crossref] [PubMed]
- American Lung Association. Lung Cancer Research [cited 2021 Dec 19]. Available online: https://www.lung.org/lung-health-diseases/lung-disease-lookup/lung-cancer/basics/lung-cancer-research
- American Lung Association. State of lung cancer 2020 [cited 2021 Dec 19]. Available online: https://www.lung.org/research/state-of-lung-cancer
- Prevedello LM, Halabi SS, Shih G, et al. Challenges Related to Artificial Intelligence Research in Medical Imaging and the Importance of Image Analysis Competitions. Radiol Artif Intell 2019;1:e180031. [Crossref] [PubMed]
- Kahn CE Jr. Artificial Intelligence, Real Radiology. Radiol Artif Intell 2019;1:e184001. [Crossref] [PubMed]
- Lincoln CM, Chatterjee R, Willis MH. Augmented Radiology: Looking Over the Horizon. Radiol Artif Intell 2019;1:e180039. [Crossref] [PubMed]
- Kadir T, Gleeson F. Lung cancer prediction using machine learning and advanced imaging techniques. Transl Lung Cancer Res 2018;7:304-12. [Crossref] [PubMed]
- Wang X, Li Q, Cai J, et al. Predicting the invasiveness of lung adenocarcinomas appearing as ground-glass nodule on CT scan using multi-task learning and deep radiomics. Transl Lung Cancer Res 2020;9:1397-406. [Crossref] [PubMed]
- Lee JG, Jun S, Cho YW, et al. Deep Learning in Medical Imaging: General Overview. Korean J Radiol 2017;18:570-84. [Crossref] [PubMed]
- Hwang EJ, Park CM. Clinical Implementation of Deep Learning in Thoracic Radiology: Potential Applications and Challenges. Korean J Radiol 2020;21:511-25. [Crossref] [PubMed]
- Park SH, Han K. Methodologic Guide for Evaluating Clinical Performance and Effect of Artificial Intelligence Technology for Medical Diagnosis and Prediction. Radiology 2018;286:800-9. [Crossref] [PubMed]
- Do S, Song KD, Chung JW. Basics of Deep Learning: A Radiologist’s Guide to Understanding Published Radiology Articles on Deep Learning. Korean J Radiol 2020;21:33-41. [Crossref] [PubMed]
- Kohli M, Prevedello LM, Filice RW, et al. Implementing Machine Learning in Radiology Practice and Research. AJR Am J Roentgenol 2017;208:754-60. [Crossref] [PubMed]
- Aerts HJ. The Potential of Radiomic-Based Phenotyping in Precision Medicine: A Review. JAMA Oncol 2016;2:1636-42. [Crossref] [PubMed]
- National Lung Screening Trial Research Team. Reduced lung-cancer mortality with low-dose computed tomographic screening. N Engl J Med 2011;365:395-409. [Crossref] [PubMed]
- Oken MM, Hocking WG, Kvale PA, et al. Screening by chest radiograph and lung cancer mortality: the Prostate, Lung, Colorectal, and Ovarian (PLCO) randomized trial. JAMA 2011;306:1865-73. [Crossref] [PubMed]
- van Klaveren RJ, Oudkerk M, Prokop M, et al. Management of lung nodules detected by volume CT scanning. N Engl J Med 2009;361:2221-9. [Crossref] [PubMed]
- de Koning HJ, van der Aalst CM, de Jong PA, et al. Reduced Lung-Cancer Mortality with Volume CT Screening in a Randomized Trial. N Engl J Med 2020;382:503-13. [Crossref] [PubMed]
- Ron E. Cancer risks from medical radiation. Health Phys 2003;85:47-59. [Crossref] [PubMed]
- van Beek EJ, Mirsadraee S, Murchison JT. Lung cancer screening: Computed tomography or chest radiographs? World J Radiol 2015;7:189-93. [Crossref] [PubMed]
- Quekel LG, Goei R, Kessels AG, et al. Detection of lung cancer on the chest radiograph: impact of previous films, clinical information, double reading, and dual reading. J Clin Epidemiol 2001;54:1146-50. [Crossref] [PubMed]
- Berlin NI, Buncher CR, Fontana RS, et al. The National Cancer Institute Cooperative Early Lung Cancer Detection Program: Results of the Initial Screen (Prevalence) Early Lung Cancer Detection: Introduction. Am Rev Respir Dis 1984;130:545-9. [PubMed]
- Gavelli G, Giampalma E. Sensitivity and specificity of chest x-ray screening for lung cancer. Cancer 2000;89:2453-6. [Crossref] [PubMed]
- Muhm JR, Miller WE, Fontana RS, et al. Lung cancer detected during a screening program using four-month chest radiographs. Radiology 1983;148:609-15. [Crossref] [PubMed]
- van Beek EJ, Mullan B, Thompson B. Evaluation of a real-time interactive pulmonary nodule analysis system on chest digital radiographic images: a prospective study. Acad Radiol 2008;15:571-5. [Crossref] [PubMed]
- White CS, Flukinger T, Jeudy J, et al. Use of a computer-aided detection system to detect missed lung cancer at chest radiography. Radiology 2009;252:273-81. [Crossref] [PubMed]
- Nam JG, Park S, Hwang EJ, et al. Development and Validation of Deep Learning-based Automatic Detection Algorithm for Malignant Pulmonary Nodules on Chest Radiographs. Radiology 2019;290:218-28. [Crossref] [PubMed]
- Sim Y, Chung MJ, Kotter E, et al. Deep Convolutional Neural Network-based Software Improves Radiologist Detection of Malignant Lung Nodules on Chest Radiographs. Radiology 2020;294:199-209. [Crossref] [PubMed]
- Lee JH, Sun HY, Park S, et al. Performance of a Deep Learning Algorithm Compared with Radiologic Interpretation for Lung Cancer Detection on Chest Radiographs in a Health Screening Population. Radiology 2020;297:687-96. [Crossref] [PubMed]
- Lee JH, Park S, Hwang EJ, et al. Deep learning-based automated detection algorithm for active pulmonary tuberculosis on chest radiographs: diagnostic performance in systematic screening of asymptomatic individuals. Eur Radiol 2021;31:1069-80. [Crossref] [PubMed]
- Jonas DE, Reuland DS, Reddy SM, et al. Screening for Lung Cancer With Low-Dose Computed Tomography: Updated Evidence Report and Systematic Review for the US Preventive Services Task Force. JAMA 2021;325:971-87. [Crossref] [PubMed]
- Pinsky PF. Lung cancer screening with low-dose CT: a world-wide view. Transl Lung Cancer Res 2018;7:234-42. [Crossref] [PubMed]
- Henschke CI, Yip R, Yankelevitz DF, et al. Definition of a positive test result in computed tomography screening for lung cancer: a cohort study. Ann Intern Med 2013;158:246-52. [Crossref] [PubMed]
- Yip R, Henschke CI, Yankelevitz DF, et al. CT screening for lung cancer: alternative definitions of positive test result based on the national lung screening trial and international early lung cancer action program databases. Radiology 2014;273:591-6. [Crossref] [PubMed]
- Rampinelli C, De Marco P, Origgi D, et al. Exposure to low dose computed tomography for lung cancer screening and risk of cancer: secondary analysis of trial data and risk-benefit analysis. BMJ 2017;356:j347. [Crossref] [PubMed]
- Pinsky PF. Assessing the benefits and harms of low-dose computed tomography screening for lung cancer. Lung Cancer Manag 2014;3:491-8. [Crossref] [PubMed]
- Gareen IF, Duan F, Greco EM, et al. Impact of lung cancer screening results on participant health-related quality of life and state anxiety in the National Lung Screening Trial. Cancer 2014;120:3401-9. [Crossref] [PubMed]
- Armato SG 3rd, Altman MB, La Rivière PJ. Automated detection of lung nodules in CT scans: effect of image reconstruction algorithm. Med Phys 2003;30:461-72. [Crossref] [PubMed]
- Suzuki K, Armato SG III, Li F, et al. Massive training artificial neural network (MTANN) for reduction of false positives in computerized detection of lung nodules in low-dose computed tomography. Med Phys 2003;30:1602-17. [Crossref] [PubMed]
- Roos JE, Paik D, Olsen D, et al. Computer-aided detection (CAD) of lung nodules in CT scans: radiologist performance and reading time with incremental CAD assistance. Eur Radiol 2010;20:549-57. [Crossref] [PubMed]
- Liang M, Tang W, Xu DM, et al. Low-Dose CT Screening for Lung Cancer: Computer-aided Detection of Missed Lung Cancers. Radiology 2016;281:279-88. [Crossref] [PubMed]
- Lee SM, Park CM. Application of Artificial Intelligence in Lung Cancer Screening. J Koerean Soc Radiol 2019;80:872-9. [Crossref]
- Huang X, Shan J, Vaidya V, et al. Lung nodule detection in CT using 3D convolutional neural networks. 2017 IEEE 14th International Symposium on Biomedical Imaging (ISBI 2017) 2017;379-83.
- Schultheiss M, Schober SA, Lodde M, et al. A robust convolutional neural network for lung nodule detection in the presence of foreign bodies. Sci Rep 2020;10:12987. [Crossref] [PubMed]
- Setio AAA, Traverso A, de Bel T, et al. Validation, comparison, and combination of algorithms for automatic detection of pulmonary nodules in computed tomography images: The LUNA16 challenge. Med Image Anal 2017;42:1-13. [Crossref] [PubMed]
- Li L, Liu Z, Huang H, et al. Evaluating the performance of a deep learning-based computer-aided diagnosis (DL-CAD) system for detecting and characterizing lung nodules: Comparison with the performance of double reading by radiologists. Thorac Cancer 2019;10:183-92. [Crossref] [PubMed]
- Al Mohammad B, Brennan PC, Mello-Thoms C. A review of lung cancer screening and the role of computer-aided detection. Clin Radiol 2017;72:433-42. [Crossref] [PubMed]
- Ciompi F, Chung K, van Riel SJ, et al. Towards automatic pulmonary nodule management in lung cancer screening with deep learning. Sci Rep 2017;7:46479. [Crossref] [PubMed]
- MacMahon H, Naidich DP, Goo JM, et al. Guidelines for Management of Incidental Pulmonary Nodules Detected on CT Images: From the Fleischner Society 2017. Radiology 2017;284:228-43. [Crossref] [PubMed]
- Ardila D, Kiraly AP, Bharadwaj S, et al. End-to-end lung cancer screening with three-dimensional deep learning on low-dose chest computed tomography. Nat Med 2019;25:954-61. [Crossref] [PubMed]
- Huang P, Lin CT, Li Y, et al. Prediction of lung cancer risk at follow-up screening with low-dose CT: a training and validation study of a deep learning method. Lancet Digit Health 2019;1:e353-62. [Crossref] [PubMed]
- Lu MT, Raghu VK, Mayrhofer T, et al. Deep Learning Using Chest Radiographs to Identify High-Risk Smokers for Lung Cancer Screening Computed Tomography: Development and Validation of a Prediction Model. Ann Intern Med 2020;173:704-13. [Crossref] [PubMed]
- Park CM, Lee JH. Deep Learning for Lung Cancer Nodal Staging and Real-World Clinical Practice. Radiology 2022;302:212-3. [Crossref] [PubMed]
- Park SH. Diagnostic Case-Control versus Diagnostic Cohort Studies for Clinical Validation of Artificial Intelligence Algorithm Performance. Radiology 2019;290:272-3. [Crossref] [PubMed]
- American Cancer Society. Cancer Facts & Figures 2021 [cited 2021 Dec 19]. Available online: https://www.cancer.org/content/dam/cancer-org/research/cancer-facts-and-statistics/annual-cancer-facts-and-figures/2021/cancer-facts-and-figures-2021.pdf
- Lim KP, Marshall H, Tammemägi M, et al. Protocol and Rationale for the International Lung Screening Trial. Ann Am Thorac Soc 2020;17:503-12. [Crossref] [PubMed]
- Gierada DS, Pinsky P, Nath H, et al. Projected outcomes using different nodule sizes to define a positive CT lung cancer screening examination. J Natl Cancer Inst 2014;106:dju284. [Crossref] [PubMed]
- American College of Radiology. Lung CT Screening Reporting & Data System (Lung-RADS v1.1 [cited 2021 Dec 19]. Available online: https://www.acr.org/Clinical-Resources/Reporting-and-Data-Systems/Lung-Rads
- McWilliams A, Tammemagi MC, Mayo JR, et al. Probability of cancer in pulmonary nodules detected on first screening CT. N Engl J Med 2013;369:910-9. [Crossref] [PubMed]
- Swensen SJ, Silverstein MD, Ilstrup DM, et al. The probability of malignancy in solitary pulmonary nodules: application to small radiologically indeterminate nodules. Arch Intern Med 1997;157:849-55. [Crossref] [PubMed]
- Massion PP, Antic S, Ather S, et al. Assessing the Accuracy of a Deep Learning Method to Risk Stratify Indeterminate Pulmonary Nodules. Am J Respir Crit Care Med 2020;202:241-9. [Crossref] [PubMed]
- Baldwin DR, Gustafson J, Pickup L, et al. External validation of a convolutional neural network artificial intelligence tool to predict malignancy in pulmonary nodules. Thorax 2020;75:306-12. [Crossref] [PubMed]
- Ohno Y, Aoyagi K, Yaguchi A, et al. Differentiation of Benign from Malignant Pulmonary Nodules by Using a Convolutional Neural Network to Determine Volume Change at Chest CT. Radiology 2020;296:432-43. [Crossref] [PubMed]
- Scagliotti GV, Pastorino U, Vansteenkiste JF, et al. Randomized phase III study of surgery alone or surgery plus preoperative cisplatin and gemcitabine in stages IB to IIIA non-small-cell lung cancer. J Clin Oncol 2012;30:172-8. [Crossref] [PubMed]
- Lee JH, Lim WH, Hong JH, et al. Growth and Clinical Impact of 6-mm or Larger Subsolid Nodules after 5 Years of Stability at Chest CT. Radiology 2020;295:448-55. [Crossref] [PubMed]
- Goldstraw P, Chansky K, Crowley J, et al. The IASLC Lung Cancer Staging Project: Proposals for Revision of the TNM Stage Groupings in the Forthcoming (Eighth) Edition of the TNM Classification for Lung Cancer. J Thorac Oncol 2016;11:39-51. [Crossref] [PubMed]
- Travis WD, Asamura H, Bankier AA, et al. The IASLC Lung Cancer Staging Project: Proposals for Coding T Categories for Subsolid Nodules and Assessment of Tumor Size in Part-Solid Tumors in the Forthcoming Eighth Edition of the TNM Classification of Lung Cancer. J Thorac Oncol 2016;11:1204-23.
- Zhao W, Yang J, Sun Y, et al. 3D deep learning from CT scans predicts tumor invasiveness of subcentimeter pulmonary adenocarcinomas. Cancer Res 2018;78:6881-9. [Crossref] [PubMed]
- Kim H, Lee D, Cho WS, et al. CT-based deep learning model to differentiate invasive pulmonary adenocarcinomas appearing as subsolid nodules among surgical candidates: comparison of the diagnostic performance with a size-based logistic model and radiologists. Eur Radiol 2020;30:3295-305. [Crossref] [PubMed]
- Amin MB, Gress DM, Vega LRM, AJCC Cancer Staging Manual. 8th ed. Chicago, IL: Springer International Publishing; 2017.
- Ahn Y, Lee SM, Noh HN, et al. Use of a Commercially Available Deep Learning Algorithm to Measure the Solid Portions of Lung Cancer Manifesting as Subsolid Lesions at CT: Comparisons with Radiologists and Invasive Component Size at Pathologic Examination. Radiology 2021;299:202-10. [Crossref] [PubMed]
- Koo TK, Li MY. A Guideline of Selecting and Reporting Intraclass Correlation Coefficients for Reliability Research. J Chiropr Med 2016;15:155-63. [Crossref] [PubMed]
- Rami-Porta R, Bolejack V, Crowley J, et al. The IASLC Lung Cancer Staging Project: Proposals for the Revisions of the T Descriptors in the Forthcoming Eighth Edition of the TNM Classification for Lung Cancer. J Thorac Oncol 2015;10:990-1003.
- Choi H, Kim H, Hong W, et al. Prediction of visceral pleural invasion in lung cancer on CT: deep learning model achieves a radiologist-level performance with adaptive sensitivity and specificity to clinical needs. Eur Radiol 2021;31:2866-76. [Crossref] [PubMed]
- Vial MR, O’Connell OJ, Grosu HB, et al. Diagnostic performance of endobronchial ultrasound-guided mediastinal lymph node sampling in early stage non-small cell lung cancer: A prospective study. Respirology 2018;23:76-81. [Crossref] [PubMed]
- Lin JT, Yang XN, Zhong WZ, et al. Association of maximum standardized uptake value with occult mediastinal lymph node metastases in cN0 non-small cell lung cancer. Eur J Cardiothorac Surg 2016;50:914-9. [Crossref] [PubMed]
- Zhong Y, She Y, Deng J, et al. Deep Learning for Prediction of N2 Metastasis and Survival for Clinical Stage I Non-Small Cell Lung Cancer. Radiology 2022;302:200-11. [Crossref] [PubMed]
- Castelvecchi D. Can we open the black box of AI? Nature 2016;538:20-3. [Crossref] [PubMed]
- Weeks JC, Cook EF, O’Day SJ, et al. Relationship between cancer patients’ predictions of prognosis and their treatment preferences. JAMA 1998;279:1709-14. [Crossref] [PubMed]
- Kaplowitz SA, Campo S, Chiu WT. Cancer patients’ desires for communication of prognosis information. Health Commun 2002;14:221-41. [Crossref] [PubMed]
- American Cancer Society, Cancer Staging [cited 2021 Dec 19]. Available online: https://www.cancer.org/treatment/understanding-your-diagnosis/staging.html
- Uramoto H, Tanaka F. Recurrence after surgery in patients with NSCLC. Transl Lung Cancer Res 2014;3:242-9. [PubMed]
- Kim H, Goo JM, Lee KH, et al. Preoperative CT-based Deep Learning Model for Predicting Disease-Free Survival in Patients with Lung Adenocarcinomas. Radiology 2020;296:216-24. [Crossref] [PubMed]
- Lou B, Doken S, Zhuang T, et al. An image-based deep learning framework for individualising radiotherapy dose: a retrospective analysis of outcome prediction. Lancet Digit Health 2019;1:e136-47. [Crossref] [PubMed]
- Hosny A, Parmar C, Coroller TP, et al. Deep learning for lung cancer prognostication: A retrospective multi-cohort radiomics study. PLoS Med 2018;15:e1002711. [Crossref] [PubMed]
- Xu Y, Hosny A, Zeleznik R, et al. Deep Learning Predicts Lung Cancer Treatment Response from Serial Medical Imaging. Clin Cancer Res 2019;25:3266-75. [Crossref] [PubMed]
- RECIST Working Group. RECIST 1.1 [cited 2021 Dec 19]. Available online: https://recist.eortc.org/recist-1-1-2/
- Sequist LV, Yang JCH, Yamamoto N, et al. Phase III study of afatinib or cisplatin plus pemetrexed in patients with metastatic lung adenocarcinoma with EGFR mutations. J Clin Oncol 2013;31:3327-34. [Crossref] [PubMed]
- Maemondo M, Inoue A, Kobayashi K, et al. Gefitinib or chemotherapy for non-small-cell lung cancer with mutated EGFR. N Engl J Med 2010;362:2380-8. [Crossref] [PubMed]
- Li T, Kung HJ, Mack PC, et al. Genotyping and genomic profiling of non-small-cell lung cancer: implications for current and future therapies. J Clin Oncol 2013;31:1039-49. [Crossref] [PubMed]
- Zhou C, Wu YL, Chen G, et al. Erlotinib versus chemotherapy as first-line treatment for patients with advanced EGFR mutation-positive non-small-cell lung cancer (OPTIMAL, CTONG-0802): a multicentre, open-label, randomised, phase 3 study. Lancet Oncol 2011;12:735-42. [Crossref] [PubMed]
- Sacher AG, Dahlberg SE, Heng J, et al. Association Between Younger Age and Targetable Genomic Alterations and Prognosis in Non-Small-Cell Lung Cancer. JAMA Oncol 2016;2:313-20. [Crossref] [PubMed]
- Hong H, Hahn S, Matsuguma H, et al. Pleural recurrence after transthoracic needle lung biopsy in stage I lung cancer: a systematic review and individual patient-level meta-analysis. Thorax 2021;76:582-90. [Crossref] [PubMed]
- Wang S, Shi J, Ye Z, et al. Predicting EGFR mutation status in lung adenocarcinoma on computed tomography image using deep learning. Eur Respir J 2019;53:1800986. [Crossref] [PubMed]
- Rizvi H, Sanchez-Vega F, La K, et al. Molecular determinants of response to anti-programmed cell death (PD)-1 and anti-programmed death-ligand 1 (PD-L1) blockade in patients with non-small-cell lung cancer profiled with targeted next-generation sequencing. J Clin Oncol 2018;36:633-41. [Crossref] [PubMed]
- Goodman AM, Piccioni D, Kato S, et al. Prevalence of PDL1 Amplification and Preliminary Response to Immune Checkpoint Blockade in Solid Tumors. JAMA Oncol 2018;4:1237-44. [Crossref] [PubMed]
- Offin M, Rizvi H, Tenet M, et al. Tumor Mutation Burden and Efficacy of EGFR-Tyrosine Kinase Inhibitors in Patients with EGFR-Mutant Lung Cancers. Clin Cancer Res 2019;25:1063-9. [Crossref] [PubMed]
- He B, Dong D, She Y, et al. Predicting response to immunotherapy in advanced non-small-cell lung cancer using tumor mutational burden radiomic biomarker. J Immunother Cancer 2020;8:e000550. [Crossref] [PubMed]
- Geijer H, Geijer M. Added value of double reading in diagnostic radiology,a systematic review. Insights Imaging 2018;9:287-301. [Crossref] [PubMed]
- Gonem S, Janssens W, Das N, et al. Applications of artificial intelligence and machine learning in respiratory medicine. Thorax 2020;75:695-701. [Crossref] [PubMed]
- Tschandl P, Rinner C, Apalla Z, et al. Human-computer collaboration for skin cancer recognition. Nat Med 2020;26:1229-34. [Crossref] [PubMed]
- Gaube S, Suresh H, Raue M, et al. Do as AI say: susceptibility in deployment of clinical decision-aids. NPJ Digit Med 2021;4:31. [Crossref] [PubMed]