Predictive performance of selected breath volatile organic carbon compounds in stage 1 lung cancer
Introduction
Lung cancer remains the leading cause of cancer deaths accounting for almost 25% of all cancer deaths (1). Regular screening in patients at risk has previously shown a mortality benefit and a patient’s best chance of survival remains early detection (2,3). The National Lung Cancer Screening Trial (NLST) demonstrated a 20% relative decrease in lung cancer mortality with low dose CT scans (LDCT) (4) with a sensitivity of 93.8%, specificity of 73.4%, and negative predictive value of 99.9% (4). Due to the results of this trial, LDCT scan has become the gold standard for early lung cancer detection. Despite these efforts, CT screening has suffered from slow adoption in part due to its 27% false positive rate (4) which has led to unnecessary procedures with associated morbidity and mortality. Only 15% of lung cancer patients are diagnosed at an early stage (5). If detected at stage 1, the 5-year survival can exceed 90%, thus additional early identification tests are needed (1,6,7).
Volatile organic compounds (VOCs) are carbon containing compounds which may be produced by the body, or be environmental contaminants (8). These compounds can be measured in breath, and there has been extensive research into their ability to be used for lung cancer diagnostics (8-22). The use of breath analytics in lung cancer has been previously attempted, each study with limitations. One of the first studies was in 1971 and identified 250 different VOCs in human breath samples (23). As technology advanced, this study was repeated in 1999 and over 3,000 breath VOCs were identified (18). Multiple studies have since followed and a re-emergence of a search for a VOC signature started in the 2010s. Fu et al. studied the differences in VOCs between lung cancer patients and healthy controls, with the goal to identify two or more VOCs as a “fingerprint” to identify lung cancer (12). They were able to identify a signature of 4 VOCs and reported a sensitivity and specificity of 89.9% and 81.3%, respectively (12). A second study was released a year later, which included an analysis of three groups of participants: benign nodules, healthy controls and lung cancer patients (21). This study reported a VOC signature with a sensitivity and specificity of 88.5% and 86.5%, respectively (21). Multiple other studies have been conducted, none of which identified a VOC signature (13,14,17,19,20,22). None of these studies provided a practical and reproducible VOC signature of cancer as: (I) the definitions of “signatures” are impractical as they only provide a list of compounds; (II) data that underlined the results are not publicly available; (III) code that was used for signatures is not available; (IV) VOCs are not expressed in units of concentration (e.g., µg/L); and (V) not enough practical details are provided about how to re-construct the “signature” in a new study. Moreover, many of these studies have been limited by small sample sizes, heterogeneous experimental conditions, lack of calibration of VOCs to the concentration scale, irreproducible analytic pipelines, and/or lack of built-in validation component. Despite being extensive, this existing literature does not provide a practical solution to detecting early-stage lung cancer using breath VOCs.
The current study was conducted to evaluate the reproducibility and validity of published associations between stage 1 lung cancer (S1LC) and 13 exhaled breath VOCs previously identified in the literature as promising lung cancer biomarkers. The study also explored whether a practical, clinically useful breath VOC signature can be constructed from these and other new VOCs to detect S1LC. We present the following article in accordance with the STARD reporting checklist (available at https://tlcr.amegroups.com/article/view/10.21037/tlcr-21-953/rc).
Methods
Study population
This study was conducted in accordance with the Declaration of Helsinki (as revised in 2013). Registered on ClinicalTrials.gov (identifier: NCT03275688), the study was conducted at the Johns Hopkins University Hospital (Baltimore, MD, USA) and approved by the Johns Hopkins institutional review board (No. IRB00121967). Informed consent was taken from all of the patients. Study data collection and analytic protocol for this prospective study was developed prior to data collection. Data was collected between February 2018 and July 2019. Adults over age 18 diagnosed S1LC, current smokers, greater than age 55, with at least a 30 pack-year smoking history and a negative Chest CT as well as non-smokers, greater than age 55, free of suspicion for lung cancer with a negative Chest CT were included in the study. Potential S1LC cases from the population of lung cancer patients seen by the senior author (LY) were enrolled in the study. This was a consecutive series, where patients were recruited until the target sample size was achieved. Data for potential S1LC cases who were not confirmed to have S1LC via biopsy were excluded from the subsequent analyses. Each biopsy-confirmed S1LC case was matched to one or two controls to reduce the potential for confounding and to ensure a good balance of covariates in the case and control groups. Two types of controls were used for each case: (I) the type 1 control was identified via the JHU electronic medical record system (Epic, Verona, WI) and was matched on smoking status, vaping, sex, family history of lung cancer, age, race, and alcohol use [see the supplementary materials (Appendix 1) for details on covariate matching]; and (II) the type 2 control was identified, when possible, during the preliminary patient visit as a cancer-free adult person who lives in the same household with the lung cancer case patient. Some patients did not have another adult living in the same household or if they have, they did not consent to participate in the study; in these cases, the type 2 control sample was not collected. All study participants were asked to abstain from smoking, vaping, or drinking for at least 30 min before conducting the test.
Collection of exhaled breath samples
Participants exhaled directly into two Tedlar® bags. Participants were instructed to take a deep inhalation and exhale ~150–300 mL of breath into the 0.5 L volume bag (Bag 1) to compensate for dead space. Immediately, the participant then inflated the 1 L volume bag (Bag 2) using the rest of the exhaled breath. All collected breath samples were delivered at room temperature to the Johns Hopkins Bloomberg School of Public Health (JHBSPH) research lab for VOC analysis within 2 h after collection (whenever possible). The balloon was measured within 24 h of breath by the lab in 166 (74%) patients. All but 3 balloons were read within 24 h of lab acquisition. Only data collected from Bag 2 were used for the analyses.
VOC analysis with thermal desorption gas chromatography-mass spectrometry (TD-GC-MS)
Thirteen previously reported VOCs, representing different chemical groups, were selected for quantitative analysis; details are provided in Table S1. For each selected VOC, a five-point calibration curve was generated by spiking reagent-grade standards into Tedlar® bags in concentrations ranging from 0.4 to 4,000 µg/mL using methanol as solvent. Five calibration curves for each VOC were generated, and their average slope and intercept were used to quantify concentrations from participant samples. Clean and humidified air was injected into a subset (10%) of bags to evaluate measurement background.
VOCs in the exhaled breath were analyzed using thermal desorption (TD) and gas chromatography–mass spectrometry (GC-MS). The laboratory did not receive information about participant case status. A multiple channel thermal desorption system (UNITY-xrTM) with an auto-sampler (CIA Advantage-xrTM both from Markes International, Inc., UK) was used to sample 100 mL of exhaled breath from each of the Tedlar bags at a flow rate of 50 mL/min and flow path temperature of 150 ℃. Helium was used as the carrier gas at a constant pressure of 5 Pounds per Square Inch (PSI); the sample was directly injected from the TD unit into the gas chromatograph for analysis. Chromatographic analysis was performed using a Trace GC-Ultra gas chromatograph attached to an ISQ Mass Spectrometer (GC-MS, Thermo Scientific). Additional details, including quality control, are provided in the supplementary materials (Appendix 1).
Calibration curves and quality control
Thirteen previously reported VOCs, representing different chemical groups, were selected for quantitative analysis (Table S1). The limit of detection (LOD) for each chemical was calculated according to the methods provided in the supplementary materials (Appendix 1).
Statistical analysis
Since many VOCs were below the LOD for a large percentage of observations, only four VOCs with less than 10% data below the LOD were used in analyses. Each concentration was log10-transformed. Additional models were fit with each individual VOC being above/below the LOD as a predictor and S1LC as an outcome using univariate logistic regression analysis. A total cohort of 300 individuals was planned: 240 individuals with lung nodules suspicious for possible lung cancer, 30 long-term smokers, and 30 non-smokers. With the overall prevalence of disease of 56.67%, the total sample size of 300 yielded at least 90% power to estimate sensitivity with a 95% confidence interval of ±0.09 at an expected sensitivity of 0.90 and at least 90% power to estimate specificity with a 95% confidence interval of ±0.11 at an expected specificity of 0.90 (24,25). Power analysis was conducted utilizing R (Viena, Austria) (26). Following the study analytic protocol, the first 30 groups of matched cases and controls, determined by case enrollment time, were used for training and the last 58 groups were used for testing. Larger proportion of the data was selected for testing to illustrate the higher robustness of the predictions. Analyses were conducted by combining the two types of controls, whenever they were both available. Additional details are provided in the supplementary materials (Appendix 1).
Each model was fit to the training data, and then applied to: (I) the testing; and (II) the combined testing and training data. All analyses were performed in the R statistical software (26). To detect statistically significant differences between VOC breath concentrations in S1LC and controls, two sample unpaired t-tests, which lose some power, but ensure that results are generalizable to the population, were performed using the R function t.test(). Classification tests using thresholds of the statistically significant VOC were developed based on the 10th, 25th and 50th percentiles of VOC concentrations in the training data of controls. Univariate and multivariate forward selection logistic regression models were fit using the glm() function in R. Forward selection was used to identify the combination of most predictive VOCs. Selection of VOCs were based on the improvement in the receiver operating characteristic area under the curve (AUC) in the training data, where at each stage the VOC with the highest AUC in the training data was incorporated into the model. For each selected model the AUC on the test data was computed. Missing observations were excluded in each candidate model when individual VOCs were below the LOD.
Results
Participant characteristics
A total of 330 participants, with 157 potential S1LC cases were considered in this study. Out of 157 potential cases, 65 were not biopsy-confirmed to have S1LC and were excluded from the study. Matching controls associated with these potential cases were also excluded (n=40). A total of 88 matched groups with biopsy-confirmed S1LC and at least a type 1 control available were recruited. Among these groups, 39 groups had only one type 1 control and no type 2 control and 49 had both type 1 and 2 controls. The total sample size was 225 (88 biopsy-confirmed cases, 88 type 1 controls, and 49 type 2 controls). No adverse events occurred as a result of breath collection for VOC measurement. Patient selection flowchart is shown in Figure 1. Table 1 provides the demographics for the study participants by group. The distributions of covariates for S1LC and type 1 controls are very similar for the covariates used for matching. The exceptions were history of family cancer and alcohol use. The latter variable was used for matching whenever possible but was not enforced; see the matching protocol details in the supplementary material (Appendix 1). The distributions of covariates among the type 2 controls are slightly different, as type 2 controls were only matched on being from the same household and not on covariates. Overall, the housemate controls were on average 4.7 years younger than the S1LC cases and matched controls. The proportion of participants who never smoked (63.3%) was higher among the housemate controls compared to the S1LC patients (18.2%) and their matched controls (18.2%). The proportion of participants with a history of family lung cancer was the highest among S1LC cases (36.4%), followed by type 2 controls (20.4%), and type 1 controls (3.4%). There was a significantly smaller percentage on S1LC individuals without kidney disease (81.8%) when compared to type 1 (90.9%) and type 2 (95.9%) controls.
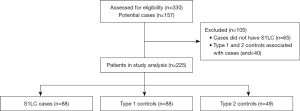
Table 1
Characteristics | Case (N=88) | Matched control (N=88, type 1) | Housemate Control (N=49, type 2) | P value |
---|---|---|---|---|
Age (years), mean (SD) | 67.85 (9.28) | 67.91 (9.81) | 63.13 (14.15) | 0.025 |
BMI (kg/m2), mean (SD) | 27.61 (6.11) | 28.68 (5.60) | 28.35 (4.81) | 0.446 |
Smoking history, n (%) | <0.001 | |||
Never | 16 (18.2) | 16 (18.2) | 31 (63.3) | |
Current | 14 (15.9) | 14 (15.9) | 3 (6.1) | |
Former | 58 (65.9) | 58 (65.9) | 15 (30.6) | |
Race, n (%) | 0.273 | |||
White | 63 (71.6) | 62 (70.5) | 38 (77.6) | |
Black | 17 (19.3) | 19 (21.6) | 3 (6.1) | |
Asian/Pacific Islander | 6 (6.8) | 6 (6.8) | 6 (12.2) | |
Other | 2 (2.3) | 1 (1.1) | 2 (4.1) | |
Female sex, n (%) | 52 (59.1) | 52 (59.1) | 24 (49.0) | 0.450 |
No history of family cancer, n (%) | 56 (63.6) | 85 (96.6) | 39 (79.6) | <0.001 |
No kidney disease, n (%) | 72 (81.8) | 80 (90.9) | 47 (95.9) | 0.030 |
No diabetes, n (%) | 70 (79.5) | 70 (79.5) | 41 (83.7) | 0.813 |
No liver disease, n (%) | 86 (97.7) | 82 (93.2) | 47 (95.9) | 0.340 |
No alcohol use, n (%) | 37 (42.0) | 29 (33.0) | 20 (40.8) | 0.423 |
P value column corresponds to the chi-squared test for the null hypothesis of equality of means in the three groups.
VOC concentration ranges and proportion below the LOD
Many VOCs in exhaled breath had low concentrations in the range of 0.0001 to 17.4973 µg/L for Acetoin and 0.00011 to 0.22125 µg/L for all other VOCs. Each VOC had a different LOD (second column in Table S2) and the percent of VOC measurements below the LOD for most VOCs was high for combined, training, and test data (see Table S2). Among the thirteen quantifiable VOCs considered in this analysis, only four VOC were below the LOD in less than 10% across all samples: 2-Pentanone (7.6%), Acetoin (3.1%), Heptanal (8.4%), Dodecane (1.8%). The proportion of VOCs below LOD among cases and controls in testing and training data was similar for all VOCs except p-Cymene. For p-Cymene the percentage of compounds below LOD was higher in S1LC cases. In the training data, 64% of the measurements were below LOD among cases and 38% among controls. In the test data 70% of the measurements were below LOD among cases and 54% among controls.
Figure 2 displays the distributions of VOC concentrations for training (Figure 2A) and test (Figure 2B) data separated by cases and control types. Acetoin concentrations tended to be lower both in training and test cases, while Heptanal and Dodecane concentrations tended to be lower in training and roughly similar in test samples. 2-Pentanone concentrations tended to be higher in both training and test cases than controls, though the difference was not significant (combined data t-test P value =0.699; see Table 2).
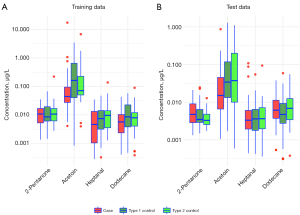
Table 2
VOC | Training data | Test data | Combined data (training +test) | ||||||||
---|---|---|---|---|---|---|---|---|---|---|---|
N cases | N controls | P value | N cases | N controls | P value | N cases | N controls | P value | |||
2-Pentanone | 28 | 48 | 0.568 | 55 | 77 | 0.105 | 83 | 125 | 0.699 | ||
Acetoin | 28 | 49 | 0.091 | 57 | 84 | 0.001 | 85 | 133 | <0.001 | ||
Dodecane | 29 | 51 | 0.268 | 56 | 85 | 0.462 | 85 | 136 | 0.762 | ||
Heptanal | 23 | 45 | 0.084 | 56 | 82 | 0.837 | 79 | 127 | 0.237 |
VOC, volatile organic compound.
VOC predictive performance to discriminate among S1LC and controls
Analysis of probability of concentrations to be below the LOD
As several VOCs had large proportions of observations that are below the LOD, the predictive performance was investigated for every VOC being above/below the LOD. Univariate analyses of the prediction performance of S1LC cases using the predictors “above or below the LOD” indicated that p-Cymene had the highest predictive accuracy (training AUC =0.630; testing AUC =0.580; see Table 3). The limit of detection for p-Cymene (see Table S2) was 0.00011 µg/L; thus, the model uses a decision rule of having a p-Cymene breath concentration below 0.00011 µg/L to predict S1LC cases. The test AUCs for the remaining 12 VOCs was close to 0.5 indicating that being above or below the LOD was not predictive of S1LC.
Table 3
VOC | Univariate AUC | |
---|---|---|
Training | Test | |
3-3-dimethyl-pentane | 0.504 | 0.494 |
2-Butanone | 0.504 | 0.503 |
2-Pentanone | 0.504 | 0.474 |
Toluene | 0.524 | 0.500 |
3-methyl-1-butanol | 0.561 | 0.518 |
Acetoin | 0.514 | 0.497 |
2-hexanol | 0.555 | 0.486 |
Hexanal | 0.528 | 0.500 |
Ethylbenzene | 0.504 | 0.511 |
Heptanal | 0.558 | 0.494 |
Cyclohexanone | 0.547 | 0.488 |
p-Cymene | 0.630 | 0.580 |
Dodecane | 0.517 | 0.511 |
VOCs are ordered by name, not by any measure. AUC, area under the curve; S1LC, stage 1 lung cancer; LOD, limit of detection; VOC, volatile organic compound.
Analysis of concentrations above the LOD
Table 2 presents the results of comparing the mean of the log10 concentration among cases and combined controls in the training, test, and combined test and training data using unpaired t-test. With the exception of Acetoin, the difference between cases and controls was not statistically significant for any of the group comparisons. For Acetoin the difference in the means was: (I) not significant in the training sample (P=0.091); (II) significant in the test sample (P=0.001); and (III) significant in the combined sample (P<0.001). These differences are likely due to the difference in sample size; for example, for Acetoin there are 28 cases and 49 controls in the training data, but there are 85 cases and 133 controls in the combined data.
Table 4 provides individual VOCs S1LC case prediction performance using univariate and multivariate forward selection logistic regression based on log10 concentrations above the LOD. In univariate models (one predictor at a time) Acetoin and Heptanal have training AUC greater than 0.6, while other compounds have AUCs close to 0.5. The AUC for Acetoin is 0.649 in the training data (N=77) and 0.650 in the test data (N=141), indicating that the predictive performance of Acetoin was preserved in the test data. In contrast, the AUC for Heptanal is 0.610 in the training data (N=68) and only 0.511 in the test data (N=138), indicating that Heptanal may not be a reliable predictor of S1LC cases. Dodecane has a consistent, low AUC for training (0.574) and test (0.541) data.
Table 4
VOC | Univariate model | Forward selection cumulative model | |||||||||
---|---|---|---|---|---|---|---|---|---|---|---|
Training | Test | Training | Test | ||||||||
Univariate AUC | N | Univariate AUC | N | Cumulative AUC | N | Cumulative AUC | N | ||||
Acetoin | 0.649 | 77 | 0.650 | 141 | 0.649 | 77 | 0.650 | 141 | |||
Heptanal | 0.610 | 68 | 0.511 | 138 | 0.669 | 64 | 0.559 | 137 | |||
2-Pentanone | 0.502 | 76 | 0.590 | 132 | 0.689 | 63 | 0.601 | 128 | |||
Dodecane | 0.574 | 80 | 0.541 | 141 | 0.686 | 63 | 0.592 | 127 |
Models are developed on the training data and evaluated on the test data set. The forward selection model is cumulative; for example, the row labeled 2-Pentanone indicates that 2-Pentanone was the third variable added to the model and the corresponding AUC refers to the model that includes Acetoin, Heptanal, and 2-Pentanone. VOC, volatile organic compound; AUC, area under the curve.
Cumulative AUCs for the multivariate forward selection logistic regression as additional VOCs are included into the model are provided in Table 4 for both the training and test data. Acetoin is the strongest predictor with a training AUC of 0.649 and a test AUC of 0.650. Adding Heptanal increases the training AUC to 0.669 and decreases the test AUC to 0.559. Adding 2-Pentanone to the model increases the training AUC (from 0.669 to 0.689) though the test AUC of 0.601 is lower than the test AUC of 0.65 for Acetoin alone. A two variable model adding either dodecane or 2-Pentanone could also be considered. However, more complex models are not considered at this time given the low individual AUC values for these VOCs and the high correlations among the other log concentrations of VOC (Table S3).
S1LC classification models based on Acetoin
Results based on VOC concentrations suggest that Acetoin: (I) has most concentrations above the limit of detection; (II) leads to the best predictive model in the test data; and (III) has a stable performance when transitioning from training to test data. Thus, we explore the specific Acetoin concentration thresholds expressed in mg/L and their associated S1LC case prediction performance. Because Acetoin concentrations were, on average, lower in S1LC patients compared to controls, the test follows the following rule:
if Acetointest <10threshold (from training data) | participant is classified as S1LC case; |
if Acetointest ≥10threshold (from training data) | participant is classified as control. |
The threshold (from training data), can be chosen in many different ways to balance sensitivity and specificity. Here we consider the following thresholds based on the percentiles of Acetoin concentrations in the training data of controls: (I) the 10th percentile (0.026 mg/L); (II) the 25th percentile (0.044 mg/L); and the 50th percentile (0.098 mg/L). Illustration of these thresholds classification accuracy of individual S1LC patients in the test data is illustrated in the Figure S1 and estimated sensitivity and specificity are reported in Table 5.
Table 5
Threshold | Test data | All data | |||||
---|---|---|---|---|---|---|---|
Sensitivity | Specificity | Accuracy | Sensitivity | Specificity | Accuracy | ||
10% (0.026 mg/L) | 0.649 | 0.583 | 0.610 | 0.518 | 0.699 | 0.628 | |
25% (0.044 mg/L) | 0.754 | 0.429 | 0.560 | 0.671 | 0.541 | 0.592 | |
50% (0.098 mg/L) | 0.930 | 0.286 | 0.546 | 0.871 | 0.368 | 0.564 |
S1LC, stage 1 lung cancer.
Discussion
This was the largest case-control VOC study to date with the inclusion of a healthy control and a housemate control to aid in the elimination of potential environmental confounders for VOCs that may indicate the presence of lung cancer. The control group (S1LC) cases were diverse in terms of covariates and analytic approach of combining type 1 and 2 cases ensures that study results are generalizable to the population. The novelty of the study consists of its focus on: (I) early lung cancer detection, specifically S1LC; (II) practical, translatable and reproducible signature of breath VOC for S1LC; (III) design of experiment targeted to elimination of potential confounders due to environment, technology, and breath analysis procedure; and (IV) definition of training and testing data sets before data were collected. Our study presents results that are contrary to the published literature indicating that: (I) most VOCs published in the literature have a weak or inexistent association with S1LC; (II) Acetoin, the only VOC that was associated with S1LC, has a much lower predictive performance than the performance of previously published VOC signatures (16), though none of these results specifically focused on S1LC; and (III) Acetoin concentrations were on average lower (not higher) in the breath of S1LC cases than in controls (27). Acetoin has an AUC of 0.65 with a sensitivity of 87.1% (specificity of 36.8%) when predicting that a person has S1LC if the Acetoin concentration is below 0.098 mg/L. This is a promising result that will need further investigation as this single VOC approaches the sensitivity of LDCT (4).
Acetoin has not been a VOC closely studied in its relationship to lung cancer, and in a recent review article on VOCs it was not a described candidate VOC for the detection of lung cancer (22) but is typically used in the flavorings of foods, as well as e-cigarettes (23). As we added additional VOCs to the model, the test AUC dropped. This is in contrast to multiple other studies. Indeed, in a small study of seventy patients, Gasparri et al. identified a signature, without providing the specific VOCs, with a sensitivity of 81% and specificity of 91% (13). A prior study of 229 participants reported an AUC of 0.81, though the VOCs used were not disclosed (28). Schumer et al. studied 2-butanone, 3-hydroxy-2-butanone, 2-hydroxyacetaldehyde and 4-hydroxyhexanal in a large study with 405 participants, and were able to show a sensitivity and specificity of 93.6% and 85.6%, respectively (21). Our paper raises serious concerns about these studies, especially because: (I) the data are not available; (II) methods used are only superficially described; (III) analytic methods used can be over-fit; (IV) VOC measurements are not expressed in concentration units, which implies that the measurement values may be indistinguishable from the experimental noise; and (V) there are many levels of data processing and cleaning that cannot be understood when data and code are not reproducible.
There are several limitations to our trial. First, the presence of dead space in the lung can dilute VOC’s in the same breath. To combat this, we used a separate Tedlar® bag for the first 150–200 cc of exhalation, followed by the rest of the breath into a 1 L Tedlar® bag. Second, the effect of condensation on VOCs is unknown and, unfortunately, we were unable to control for this effect in the Tedlar® bags. Third, it is not possible to control for all environmental exposures, so there may be confounders present that we did not consider- this includes the potential that participants did not abstain from smoking, vaping or drinking prior to breath collection. Fourth, although our protocol planned to analyze all breaths within a 24-h period, this was not always the case. It is possible that these delays could have led to changes in the VOC concentrations in the Tedlar® bags. Fifth, we focused on S1LC, which may not be associated with substantial changes in breath VOCs. This leaves the possibility that changes may occur in more advanced stages of lung cancer. Sixth, the time interval to abstain from smoking, vaping, or drinking for at least 30 min prior to collecting exhaled interval was chosen as a reasonable compromise for the participants and the study feasibility, however different interval lengths could affect the concentration of individual VOCs. Last, many of our demographic confounders were based on recall, such as a family history of cancer-selective memory may have played a part in answers when participants are being biopsied to assess whether they have cancer or not.
Lung cancer is the number one cause of cancer related deaths in the United States (1). The 5-year survival of patients identified to have lung cancer drastically decreases with each advancing stage. In the most recent American Cancer Society statistics, the 5-year survival for localized, regional and distant was 61%, 35%, 6%, respectively (1). Given the drastic decrease in survival for every increasing stage, a minimally invasive, accurate diagnostic test is needed. While we were unable to identify a signature with high diagnostic accuracy for lung cancer, we have identified a specific VOC that deserves further investigation: Acetoin. Further studies using this biomarker as, or part of, a screening test are warranted.
Acknowledgments
Funding: None.
Footnote
Reporting Checklist: The authors have completed the STARD reporting checklist. Available at https://tlcr.amegroups.com/article/view/10.21037/tlcr-21-953/rc
Data Sharing Statement: Available at https://tlcr.amegroups.com/article/view/10.21037/tlcr-21-953/dss
Conflicts of Interest: All authors have completed the ICMJE uniform disclosure form (available at https://tlcr.amegroups.com/article/view/10.21037/tlcr-21-953/coif). LY was a consultant for Nanobeak Biotech and formerly owned Nanobeak Biotech stock options. The study discussed in this publication was sponsored by Nanobeak Biotech. This arrangement has been reviewed and approved by the Johns Hopkins University in accordance with its conflict-of-interest policies. CC is a consultant with Bayer and Johnson and Johnson on methods development for wearable devices in clinical trials. The details of the contracts are disclosed through the Johns Hopkins University eDisclose system and have no direct or apparent relationship with the current paper. The other authors have no conflicts of interest to declare.
Ethical Statement: The authors are accountable for all aspects of the work in ensuring that questions related to the accuracy or integrity of any part of the work are appropriately investigated and resolved. This study was conducted in accordance with the Declaration of Helsinki (as revised in 2013). Registered on ClinicalTrials.gov (Identifier: NCT03275688), the study was conducted at the Johns Hopkins University Hospital (Baltimore, MD, USA) and approved by the Johns Hopkins Institutional Review Board (No. IRB00121967). Informed consent was taken from all of the patients.
Open Access Statement: This is an Open Access article distributed in accordance with the Creative Commons Attribution-NonCommercial-NoDerivs 4.0 International License (CC BY-NC-ND 4.0), which permits the non-commercial replication and distribution of the article with the strict proviso that no changes or edits are made and the original work is properly cited (including links to both the formal publication through the relevant DOI and the license). See: https://creativecommons.org/licenses/by-nc-nd/4.0/.
References
-
;Cancer Facts and Figures American Cancer Society 2021 . Available online: https://www.cancer.org/research/cancer-facts-statistics/all-cancer-facts-figures/cancer-facts-figures-2021.html - National Lung Screening Trial Research Team. Reduced lung-cancer mortality with low-dose computed tomographic screening. N Engl J Med 2011;365:395-409. [Crossref] [PubMed]
- Luo YH, Luo L, Wampfler JA, et al. 5-year overall survival in patients with lung cancer eligible or ineligible for screening according to US Preventive Services Task Force criteria: a prospective, observational cohort study. Lancet Oncol 2019;20:1098-108. [Crossref] [PubMed]
- National Lung Screening Trial Research Team. Results of initial low-dose computed tomographic screening for lung cancer. N Engl J Med 2013;368:1980-91. [Crossref] [PubMed]
- Ridge CA, McErlean AM, Ginsberg MS. Epidemiology of lung cancer. Semin Intervent Radiol 2013;30:93-8. [Crossref] [PubMed]
- Shah R, Sabanathan S, Richardson J, et al. Results of surgical treatment of stage I and II lung cancer. J Cardiovasc Surg (Torino) 1996;37:169-72. [PubMed]
- Goldstraw P, Chansky K, Crowley J, et al. The IASLC Lung Cancer Staging Project: Proposals for Revision of the TNM Stage Groupings in the Forthcoming (Eighth) Edition of the TNM Classification for Lung Cancer. J Thorac Oncol 2016;11:39-51. [Crossref] [PubMed]
- Miekisch W, Schubert JK, Noeldge-Schomburg GF. Diagnostic potential of breath analysis--focus on volatile organic compounds. Clin Chim Acta 2004;347:25-39. [Crossref] [PubMed]
- Buszewski B, Kesy M, Ligor T, et al. Human exhaled air analytics: biomarkers of diseases. Biomed Chromatogr 2007;21:553-66. [Crossref] [PubMed]
- Dent AG, Sutedja TG, Zimmerman PV. Exhaled breath analysis for lung cancer. J Thorac Dis 2013;5:S540-50. [PubMed]
- Di Francesco F, Fuoco R, Trivella MG, et al. Breath analysis: trends in techniques and clinical applications. Microchemical Journal 2005;79:405-10. [Crossref]
- Fu XA, Li M, Knipp RJ, et al. Noninvasive detection of lung cancer using exhaled breath. Cancer Med 2014;3:174-81. [Crossref] [PubMed]
- Gasparri R, Santonico M, Valentini C, et al. Volatile signature for the early diagnosis of lung cancer. J Breath Res 2016;10:016007. [Crossref] [PubMed]
- Handa H, Usuba A, Maddula S, et al. Exhaled breath analysis for lung cancer detection using ion mobility spectrometry. PLoS One 2014;9:e114555. [Crossref] [PubMed]
- Krilaviciute A, Heiss JA, Leja M, et al. Detection of cancer through exhaled breath: a systematic review. Oncotarget 2015;6:38643-57. [Crossref] [PubMed]
- Li M, Yang D, Brock G, et al. Breath carbonyl compounds as biomarkers of lung cancer. Lung Cancer 2015;90:92-7. [Crossref] [PubMed]
- Peng G, Tisch U, Adams O, et al. Diagnosing lung cancer in exhaled breath using gold nanoparticles. Nat Nanotechnol 2009;4:669-73. [Crossref] [PubMed]
- Phillips M, Gleeson K, Hughes JM, et al. Volatile organic compounds in breath as markers of lung cancer: a cross-sectional study. Lancet 1999;353:1930-3. [Crossref] [PubMed]
- Sakumura Y, Koyama Y, Tokutake H, et al. Diagnosis by Volatile Organic Compounds in Exhaled Breath from Lung Cancer Patients Using Support Vector Machine Algorithm. Sensors (Basel) 2017;17:287. [Crossref] [PubMed]
- Schallschmidt K, Becker R, Jung C, et al. Comparison of volatile organic compounds from lung cancer patients and healthy controls-challenges and limitations of an observational study. J Breath Res 2016;10:046007. [Crossref] [PubMed]
- Schumer EM, Trivedi JR, van Berkel V, et al. High sensitivity for lung cancer detection using analysis of exhaled carbonyl compounds. J Thorac Cardiovasc Surg 2015;150:1517-22; discussion 1522-4. [Crossref] [PubMed]
- Jia Z, Patra A, Kutty VK, et al. Critical Review of Volatile Organic Compound Analysis in Breath and In Vitro Cell Culture for Detection of Lung Cancer. Metabolites 2019;9:52. [Crossref] [PubMed]
- Pauling L, Robinson AB, Teranishi R, et al. Quantitative analysis of urine vapor and breath by gas-liquid partition chromatography. Proc Natl Acad Sci U S A 1971;68:2374-6. [Crossref] [PubMed]
- Ryan TP. Sample Size Determination and Power. New York: Wiley; 2013.
- Ye T, Yi Y. Sample size calculations in clinical research, third edition, by Shein-Chung Chow, Jun Shao, Hansheng Wang, and Yuliya Lokhnygina. Statistical Theory and Related Fields 2017;1:265-6.
- R Core Team (2014). R: A language and environment for statistical computing. R Foundation for StatisticalComputing, Vienna, Austria. Available online: http://www.R-project.org/
- Itoh T, Miwa T, Tsuruta A, et al. Development of an Exhaled Breath Monitoring System with Semiconductive Gas Sensors, a Gas Condenser Unit, and Gas Chromatograph Columns. Sensors (Basel) 2016;16:1891. [Crossref] [PubMed]
- Mazzone PJ, Wang XF, Xu Y, et al. Exhaled breath analysis with a colorimetric sensor array for the identification and characterization of lung cancer. J Thorac Oncol 2012;7:137-42. [Crossref] [PubMed]