Clinicopathological characteristics and prognostic significance of HDAC11 protein expression in non-small cell lung cancer: a retrospective study
Introduction
Lung cancer is the most common cause of cancer death worldwide (1). It is a molecularly heterogeneous disease, with over 85% of these patients affected by histological subtypes classified within non-small cell lung cancer (NSCLC) (2). With the advancements in of molecular biomarker research, significant progress has been made in utilizing targeted therapy to treat NSCLC. While these therapies are a key component in the fight against lung cancer, there remain a limited number of molecular therapeutic targets. Biomarkers represented by PD-L1 expression and tumor mutational burden (TMB) can be used to assess the prognosis of patients, but their predictive value is also controversial due to the need for sufficient tumor tissue. Clinical biomarkers should be considered not only in practicability, but also in operability and high cost. Therefore, it is urgent to explore suitable biomarkers that can be used in clinic to predict the prognosis of patients with NSCLC.
Histone or lysine deacetylase (HDAC/KDAC) regulates a variety of processes including transcription, cell death, and metabolism by targeting histones and non-histones to remove acetyl or acyl groups from their lysine residues (3-5). These enzymes control many growth & development processes and are key to the balance of gene expression within cells. As a result, prior work has been focused on the fundamental functions of HDACs in various organs and cellular processes in hopes to use isotype-specific or selective HDAC inhibition as a targeted strategy for treating specific diseases. As the sole class IV HDAC (6), HDAC11 is a functional enzyme with HDAC activity >10,000 times more efficient than deacetylase activity (3). While it is expressed in many organs, such as the brain and heart, its function currently is poorly understood (7,8). Previous findings have indicated that HDAC11 plays roles in immune response, neutrophil and T cell development, and tumor biology (9). According to one report, HDAC11 binds to the interleukin-10 (IL-10) promoter to promote histone deacetylation, thereby negatively regulating IL-10 of antigen-presenting cells (APCs), and subsequently affecting immune activation (10).
Notably, HDAC11, which is a key decision of great significance for autoimmunity, transplantation and cancer immunotherapy, represents a molecular target that affects immune activation and immune tolerance (10). Several interesting studies have uncovered that HDAC11 is associated with poor prognosis of neuroendocrine (10,11), gynecologic (12), and central nervous system tumors (13-16). However, the role of HDAC11 in NSCLC remains unclear to date. Hence, we aimed to study the role of HDAC11 in NSCLC to assess its relationship with clinical outcomes and potential as a new therapeutic target NSCLC. We present the following article in accordance with the REMARK reporting checklist (available at https://tlcr.amegroups.com/article/view/10.21037/tlcr-22-403/rc).
Methods
NSCLC tissue samples
Patients with NSCLC who underwent radical surgery between May 2009 and December 2013 with available paraffin-embedded tissue specimens at Tangdu Hospital (Xi’an, China) were retrospectively recruited to this study. The inclusion criteria were as follows: (I) NSCLC diagnosed by histology; (II) no radiotherapy or chemotherapy prior to surgery; (III) comprehensive staging imaging and exclusion of other organ tumors. Some clinical characteristics and results were collected from all patients, including age, gender, smoking history, histological type, clinical stage, T classification, lymph node metastases, M metastases, and histological differentiation. Postoperative survival was measured from the day of surgery, and the cut-off of follow-up was until death or December 2018. Patients were followed up every three months during this period. Primary endpoint was overall survival (OS). All tumors were staged according to the Union for International Cancer Control (UICC) Pathological Tumor-Node-Metastasis (pTNM) classification (7th edition) (17). The histological classification of the tumors was in line with World Health Organization (WHO) standards. The study was conducted in accordance with the Declaration of Helsinki (as revised in 2013). The study was approved by ethics committee of the Fourth Military Medical University (No. TDLL-202206-06) and informed consents were not requested for this retrospective study.
Tissue microarray immunohistochemistry
Paraffin-embedded tissue was cut into 5 µm slides coated with silane. They were dewaxed in xylene and hydrated by a series of anhydrous ethanol followed by antigenic microwave repair. After washing with phosphate-buffered saline (PBS) to reduce specific binding, the slides were sealed with goat serum blocking solution and excess liquid was dumped. A primary antibody (1:400; Proteintech, Rosemont, IL, USA) was added in proportion, and incubated overnight at 4 ℃. A negative control was obtained by replacing the primary antibody with PBS to maintain the specificity of staining. After the tissue sections had been washed with PBS, EnVision horseradish peroxidase (HRP; Kangwei, China) was used for secondary antibody incubation. A 3,3'-diaminobenzidine (DAB) Elite (Dako; Glostrup, Denmark) kit was used to display peroxidase activity, and sections were stained with hematoxylin. Immunohistochemistry (IHC) staining scores were based on two criteria: (I) staining intensity: (score 0, negative; score 1, yellow; score 2, brown; and score 3, tan); and (II) percentage of staining positive cells (score 0, ≤5%; score 1, 6–25%; score 2, 26–50%; score 3, 51–75%; and score 4, >75%). The 2 scores were multiplied to obtain the total score (0–12 max). We considered the mean as the dividing line between low expression and high expression in tissue specimens.
Weighted gene co-expression network analysis (WGCNA) and protein-protein interaction (PPI) screening of HDAC11 expression affecting the survival and prognosis of lung adenocarcinoma (LUAD)-related genes
We downloaded LUAD RNA-seq data from The Cancer Genome Atlas database (TCGA; https://portal.gdc.cancer.gov/). A total of 535 LUAD samples of tissues were presented. We built LUAD gene expression data by using a “WGCNA” package in the form of genetic co-expression modules. Soft power β=3 was selected to calculate topological overlap matrix (TOM) and corresponding dissimilarity degree (1-TOM). In the subsequent grouping, a hierarchical clustering tree was formed for the 1-TOM matrix. For the same gene expression, at least 50 genes were used to form the tree, which was divided into separate gene co-expression modules. A module-trait heat map was made and the relationship between the module-trait and HDAC11 expression was calculated. The module eigengene (ME) brown, with the largest correlation coefficient was selected, and the correlation coefficient was 0.21, with a total of 1,816 genes. Then, we used the limma package in R (R Foundation for Statistical Computing, Vienna, Austria) to screen differentially expressed genes (DEGs). The |log2FoldChange| ≥2 and adjust P≤0.05 were set to screen DEGs. The “ggplot. 2” in the edge R package was used to draw a volcano plot for the DEGs. In order to explore the function of LUAD, we used Gene Ontology (GO) and Kyoto Encyclopedia of Genes and Genomes (KEGG; http://www.kegg.jp/kegg/) pathway enrichment analysis according to “cluster Profile” package in R. Search Tool for the Retrieval of Interaction Genes/Proteins (STRING) database (http://string-db.org/) is a database that searches for known proteins and predicts interactions between proteins (18). PPI network analysis was performed in the STRING online database and the hub gene was identified by Cytoscape software. Finally, we used the Kaplan-Meier plotter database (https://kmplot.com/) to validate key genes.
Statistical analysis
We used χ2 test or Fisher’s exact test to evaluate the relationships between HDAC11 expression and the clinicopathologic parameters of the NSCLC patients. The Kaplan-Meier method and log-rank test were used to analyze survival data. Cox proportional hazards models were used to calculate hazard ratios (HRs) with 95% confidence intervals (CIs) in univariate and multivariate analysis Student’s t-test was used for comparison between groups. Data were expressed as mean ± standard deviation. Cases with incomplete patient data were eliminated. All statistical analyses were performed using SPSS 26.0 (IBM Corp., Armonk, NY, USA) software. All statistical tests were 2-sided, and a P value <0.05 was considered statistically significant.
Results
Patient characteristics
Tumor tissue and matched adjacent normal tissue from 339 patients were collected. We excluded 13 patients from the study due to loss of follow-up or incomplete data. A total of 326 patients had complete clinical data and were included in this retrospective study, for whom the median follow-up time was 27.0 (11.0 to 64.0) months. To determine the relationship between HDAC11 expression and clinicopathologic characteristics, we summarized details of baseline characteristics in Table 1. There were 146 patients aged <60 years and 180 patients ≥60 years. The majority were male (n=258). A total of 109 cases were diagnosed with LUAD; the other 217 were squamous cell lung carcinoma (LUSC). Clinical postoperative staging included stage I–II disease in 145 patients and stage III–IV disease in 181 patients. According to pTNM classification, patients had T1–T2 (n=129) or T3–T4 (n=197) disease, lymph node metastases were N0 (n=148) and N1–N2 (n=178), metastases were M0 (n=317) and M1 (n=9). Histopathologic diagnoses included well and moderately differentiated (n=144) and poorly or undifferentiated (n=182) tumors.
Table 1
Parameter | Number | HDAC11 expression | ||
---|---|---|---|---|
Low | High | P value | ||
Age (years) | 0.499 | |||
<60 | 146 | 83 | 63 | |
≥60 | 180 | 109 | 71 | |
Gender | 0.589 | |||
Male | 258 | 150 | 108 | |
Female | 68 | 42 | 26 | |
Smoking history | 0.159 | |||
Smoke | 221 | 136 | 85 | |
Never smoke | 105 | 56 | 49 | |
Histological type | 0.600 | |||
LUAD | 109 | 62 | 47 | |
LUSC | 217 | 130 | 87 | |
Clinical stage | 0.016 | |||
I–II | 145 | 96 | 49 | |
III–IV | 181 | 96 | 85 | |
T classification | <0.001 | |||
T1–T2 | 129 | 80 | 49 | |
T3–T4 | 197 | 105 | 92 | |
Lymph node metastases | 0.678 | |||
N0 | 148 | 89 | 59 | |
N1–N2 | 178 | 103 | 75 | |
Metastasis | 0.023 | |||
M0 | 317 | 190 | 127 | |
M1 | 9 | 2 | 7 | |
Differentiation | 0.021 | |||
Well and moderate | 144 | 95 | 49 | |
Poorly and not | 182 | 97 | 85 |
NSCLC, non-small cell lung cancer; LUAD, lung adenocarcinoma; LUSC, lung squamous cell carcinoma.
Association of HDAC11 expression in NSCLC and correlation with different clinical parameters
To investigate HDAC11 expression in NSCLC, we performed IHC analysis of tissue chips previously collected from the 326 patients (Figure 1A). We found that HDAC11 expression was significantly higher in cancer tissue than that in the adjacent tissue samples. From the 326 samples, there were 53 patients with a score of 0 and 7 patients with a score of 12 in the cancer tissue. In paracancerous tissue, 43 patients had a score of 0 and 1 patient had a score of 12. The mean value of cancer tissue was 3.1314, but that of adjacent tissue was only 1.7876 (Figure 1B). Existing data displayed that HDAC11 expression was closely related to clinical stage (P=0.016), T classification (P<0.001), M metastasis (P=0.023), and differentiation (P=0.021). There was no significant correlation with age, gender, smoking history, or lymph node metastases (Table 1).
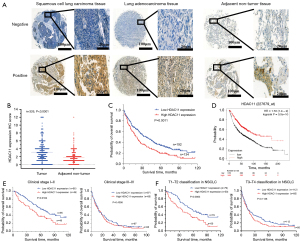
Relationship between HDAC11 expression and prognosis and survival in NSCLC
We performed correlation analyses to investigate the association of HDAC11 expression with different clinical outcomes. The results showed that the survival of patients with high HDAC11 expression (n=134) in NSCLC was significantly worse than that of those with low HDAC11 expression (n=192) (P=0.0011, Figure 1C). To further evaluate whether HDAC11 expression can be a prognostic factor, OS of NSCLC patients was analyzed by Cox proportional risk regression model. Univariate analyses showed that in our analysis of age, gender, smoking history, pTNM classification, and other factors, clinical stage (P<0.001, HR =3.164, 95% CI: 2.422 to 4.133), T classification (P<0.001, HR =2.141, 95% CI: 1.644 to 2.788), lymph node metastases (P<0.001, HR =1.821, 95% CI: 1.411 to 2.350), metastasis (P=0.025, HR =2.140, 95% CI: 1.098 to 4.169), differentiation (P<0.001, HR =5.274, 95% CI: 3.984 to 6.983), and HDAC11 expression (P=0.001, HR =1.503, 95% CI: 1.172 to 1.927) were significantly correlated with survival. Subsequent multivariate analysis showed that only clinical stage (P<0.001, 95% CI: 1.436 to 3.142), tumor differentiation (P<0.001, 95% CI: 3.358 to 6.021), and HDAC11 expression (P<0.001, 95% CI: 1.144 to 1.909) were associated with survival and prognosis of NSCLC patients (Table 2). Hence, we interpreted that HDAC11 expression may play an important role in influencing the prognosis of NSCLC. Considering the small actual sample size in this study, we verified the relationship between HDAC11 expression and survival in a larger cohort-Kaplan-Meier plotter database (n=1,925, Figure 1D). Consistent with the conclusions of this study, results in Kaplan-Meier plotter database showed that NSCLC patients with high HDAC11 expression had worse survival prognosis. Notably, we found when survival was analyzed based on stage at diagnosis, there was significant difference among patients in the clinical stage I–II (P=0.0104, Figure 1E), but not in the clinical stage III–IV (P=0.4356, Figure 1E). Furthermore, when analyzing according to T classification, it was revealed that patients with high HDAC11 expression had worse OS. This was evident for earlier (stage T1–T2) (P=0.0063, Figure 1F), but not more advanced (stage T3–T4) (P=0.1136, Figure 1F) T stages.
Table 2
Variable | Category | Univariate analysis | Multivariate analysis | |||
---|---|---|---|---|---|---|
HR (95% CI) | P value | HR (95% CI) | P value | |||
Age (years) | <60 vs. ≥60 | 1.182 (0.922–1.516) | 0.187 | – | – | |
Gender | Male vs. female | 1.071 (0.789–1.454) | 0.659 | – | – | |
Smoking history | Yes vs. no | 0.978 (0.751–1.273) | 0.868 | – | – | |
Histology | LUSC vs. LUAD | 0.822 (0.642–1.054) | 0.122 | – | – | |
Clinical stage | III–IV vs. I–II | 3.164 (2.422–4.133) | <0.001 | 2.124 (1.436–3.142) | <0.001 | |
T classification | T3−T4 vs. T1–T2 | 2.141 (1.644–2.788) | <0.001 | 1.145 (0.829–1.583) | 0.411 | |
Lymph node metastases | Yes vs. no | 1.821 (1.411–2.350) | <0.001 | 1.057 (0.772–1.449) | 0.729 | |
Metastasis | Yes vs. no | 2.140 (1.098–4.169) | 0.025 | 1.550 (0.765–3.141) | 0.223 | |
Differentiation | Poorly and not vs. well and moderate | 5.274 (3.984–6.983) | <0.001 | 4.497 (3.358–6.021) | <0.001 | |
HDAC11 expression | High vs. low | 1.503 (1.172–1.927) | 0.001 | 1.478 (1.144–1.909) | <0.001 |
NSCLC, non-small cell lung cancer; HR, hazard ratio; CI, confidence interval; LUAD, lung adenocarcinoma; LUSC, lung squamous cell carcinoma.
High HDAC11 expression level and poor prognosis in LUAD and LUSC
The 326 patients were divided into LUAD and LUSC according to pathological types, yielding 109 cases of LUAD and 217 cases of LUSC. We further investigated the effect of HDAC11 expression on prognosis in patients with LUAD and LUSC. Data in LUAD showed that high HDAC11 expression was significantly associated with poor prognosis (P=0.0004, Figure 2A), which was also confirmed in the Kaplan-Meier plotter database (HR =1.43, logrank P=0.0045, Figure 2B). Then, we performed survival analyses based on clinical stage and T classification in patients with LUAD. Clinical stage III–IV patients with high HDAC11 expression in LUAD had significantly poorer survival, (P=0.0276, Figure 2C). A similar trend was seen with T classification, as there was a significant difference in survival in T1-T2 classification (P=0.0088, Figure 2D). Notably, this difference was not present in LUSC, neither in the data of the patients in this study (P=0.1593, Figure 2E) nor in the Kaplan-Meier plotter database (HR =1.06, logrank P=0.72, Figure 2F). Interestingly, among patients with LUSC, only early clinical stages (I–II) (P=0.044, Figure 2G) were associated with survival, and there was no significant difference between advanced clinical stages (III/IV) and T classification (Figure 2H).
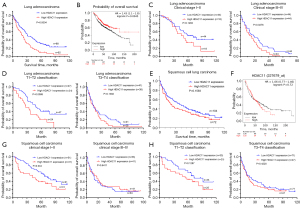
Structural network algorithms identified prognostic genes associated with HDAC11 expression in LUAD
Using edge R package chip reads to obtain 535 genes from TCGA, the mean expression level of HDAC11 gene was divided into high and low expression groups, including 209 NSCLC patients with high HDAC11 expression and 326 with low expression. Differences in LUAD samples to |log2FoldChange| ≥2 or more and adjust P≤0.05 or less for screening criteria yielded 1,173 DEGs (Figure 3A). The TMM standardization algorithm was used to standardize the data. There were 56 up-regulated genes and 1,117 down-regulated genes. In addition, a TOM was used to standardize the original matrix, which was also divided into 209 cases in the high expression group and 326 cases in the low expression group. We used to WGCNA analyze and select the module with the largest correlation coefficient, ME Brown, with a correlation coefficient of 0.21, and a total of 1,816 genes. Modules were divided into the sub-modules according to their gene expression levels (Figure 3B), and heat maps were used to show the significance and clinical features of modules. In the heat maps, red represents positive correlation and blue represents negative correlation (Figure 3C). Subsequently, 41 genes were obtained by intersection with DEGs (Figure S1A). The GO analysis only enriched to structural constituent of cytoskeleton (Figure S1B). The KEGG pathway enrichment analysis revealed relevance with staphylococcus aureus infection, NOD-like receptor signaling pathway, PPAR signaling pathway, apoptosis, and others (Figure 3D). PPI analysis screened 10 genes related to HDAC11 affecting the prognosis of LUAD (Figure 3E). The genes NKX2-5, SCG3, NOL4, OTX2, INSM1, LIN28A, FABP7, AFP, NEFM, and ACTL6B were selected as hub genes (Figure 3F). The Kaplan-Meier plotter database showed that NKX2-5 (HR =1.76, logrank P=1.6e-06, Figure 3G) and FABP7 (HR =1.54, logrank P=0.00026, Figure 3H) were associated with LUAD prognosis. Meanwhile, SCG3 (HR =1.26, logrank P=0.054, Figure S1C), NOL4 (HR =1.14, logrank P=0.29, Figure S1D), OTX2 (HR =1.2, logrank P=0.13, Figure S1E), INSM1 (HR =1.16, logrank P=0.2, Figure S1F), LIN28A (HR =1.08, logrank P=0.54, Figure S1G), AFP (HR =1.19, logrank P=0.13, Figure S1H), NEFM (HR =1.07, logrank P=0.56, Figure S1I) and ACTL6B (HR =1.06, logrank P=0.63, Figure S1J) were no correlation.
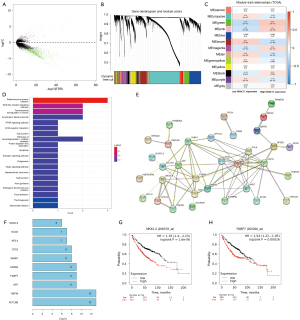
Discussion
As the predominant type of lung cancer, NSCLC accounts over 85% of all lung cancer cases and consists mainly of LUAD and LUSC (19). Targeted therapies have transformed cancer treatment with great success for patients with actionable NSCLC mutations; however, currently only a minority of patients can benefit from such treatment dependent on mutation status. Furthermore, resistance to chemotherapeutic agents and epithelial growth factor receptor (EGFR) inhibitors leads to recurrence of highly aggressive lung tumors in NSCLC, resulting in poor prognosis. Therefore, it is imperative to identify novel therapeutic strategies to combat NSCLC. At present, epigenetic therapy combined with immunotherapy has emerged as a promising approach to treating cancer The HDAC family is involved in a variety of physiological and pathological regulatory processes in humans (20), and investigation of this family comprises a new research field (21). As a new member of the HDACs family, HDAC11 is highly conserved (22), with molecular weight of only 39 KDa (8,23). Although most of the previous research on HDAC11 has focused on immunology (24), the clinical relevance of HDAC11 to NSCLC warrants attention. Previous studies have shown that HDAC11 plays a role in hematological system tumors (9,25-27), and other system tumors (28,29). For example, Bi et al. (30) reported that HDAC11 was highly expressed in hepatocellular carcinoma and closely related to disease prognosis, and the main mechanism was that HDAC11 regulated glycolysis according to the LKB1/AMPK signaling pathway. Another study demonstrated that plasma cell development and survival are dependent upon HDAC11, and knockdown or inhibition of HDAC11 induced cell death in multiple myeloma (MM) cell lines, suggesting this enzyme was a promising therapeutic target in MM (31). However, its carcinogenic effect and detailed molecular mechanism in NSCLC remain unclear.
In this study, the collected NSCLC samples were prepared for tissue microarray IHC analysis to study the expression of HDAC11 in clinical samples. Analysis of the results showed HDAC11 protein expression in tumor sections was significantly higher than that in adjacent non-cancerous tissues. However, the expression of HDAC11 in lung cancer has not been reported to date. Our data suggest that HDAC11 expression in NSCLC is significantly correlated with clinicopathological parameters such as clinical stage, T classification, metastasis, and tumor differentiation. Our results are consistent with a prior study demonstrating that HDAC11 expression affects the development and spread of lymph node metastasis in breast cancer (32). Hence, HDAC11 may be a new diagnostic tool or prognostic biomarker for NSCLC.
To our knowledge, this retrospective study is the first to suggest that HDAC11 may be an independent prognostic factor in patients with NSCLC. There is a significant correlation between high HDAC11 expression and poor prognosis in NSCLC patients. Univariate analyses showed that clinical stage, T classification, lymph node metastases, metastasis, differentiation, and HDAC11 expression were relevant to prognosis. Multivariate analysis further concluded that clinical stage, tumor differentiation, HDAC11 expression, and prognosis were related. In addition, HDAC11 expression in LUAD was significantly associated with OS, but not in patients with LUSC. We then verified the expression of HDAC11 in the Kaplan-Meier plotter database which confirmed these findings. Therefore, to determine whether HDAC11 expression can predict adverse outcomes in LUSC patients, further studies in a larger cohort of LUSC patients are needed to determine whether the lack of survival association was due to insufficient power or a difference in tumor biology.
With the development of gene chip and RNA sequencing, biological information analysis has been widely used to identify biomarkers related to cancer (33). Given the demonstrated significance of HDAC11 expression in LUAD, we further explored the mechanism of HDAC11 promoting LUAD by performing a functional enrichment analysis for the HDAC11 included GO and KEGG (http://www.kegg.jp/keg/) pathway. The GO analysis result showed that HDAC11 was only enriched to structural constituent of cytoskeleton; KEGG pathway enrichment analysis showed the relevant with staphylococcus aureus infection, NOD-like receptor signaling pathway, transcriptional misregulation in cancer, signaling pathway, and apoptosis. Most of the abovementioned pathways are inflammatory pathways, and it is generally believed that inflammation is closely related to cancer; in particular, chronic inflammation can regulate the activity of transcription silver, promote cell proliferation, inhibit cell apoptosis, and promote angiogenesis (34).
According to the mean expression of HDAC11, LUAD patients in the TCGA database were divided into a low expression group and high expression group, and 10 highly related genes were screened by WGCNA and PPI. The results showed that NKX2-5 and FABP7 were associated with LUAD prognosis. In recent years, abnormal expression of NKX2-5 has been linked to the occurrence and development of tumors (35). The FABP7 gene is thought to control the absorption and transport of fatty acids and plays an important role in differentiation of cells (36,37). Study has found that FABP7 is a prognostic marker in glioblastoma. Nuclear FABP7 immunoreactivity is preferentially expressed in infiltrative glioma and is associated with poor prognosis in EGFR-overexpressing glioblastoma (38). Therefore, we speculate that NKX2-5 and FABP7may be correlated with HDAC11 expression in LUAD, and thus affect the prognosis of patients.
A previous report has shown that HDAC11 inhibits BMP4 transcription in embryonic childhood tumors and promotes malignant development of tumors (39). Inhibition of HDAC11 led to p53-dependent apoptosis in hepatocellular carcinoma (29). Additionally, several cancer types have been shown to have HDAC overexpression (40).
Our study had limitations including a relatively small sample size, and inclusion of mostly non-metastatic NSCLC. Future studies involving larger samples are required to validate our findings. Second, we used the LUAD data from TCGA, which may have affected the reliability of our study. Finally, we showed that NKX2-5 and FABP7 are related to HDAC11 expression in LUAD, but the nature of this network relationship needs to be further elucidated as HDAC11 plays an important role in the development and progression of human cancers. This study has demonstrated for the first time that HDAC11 is a novel biomarker in NSCLC to predict patient outcomes. In NSCLC, HDAC11 expression is significantly correlated with patients’ OS, and patients with low HDAC11 expression level have higher survival prognosis and better prognosis overall. Notably, targeting HDAC11 may be a novel clinical strategy for LUAD.
Acknowledgments
The authors appreciate the academic support from the AME Lung Cancer Collaborative Group.
Funding: None.
Footnote
Reporting Checklist: The authors have completed the REMARK reporting checklist. Available at https://tlcr.amegroups.com/article/view/10.21037/tlcr-22-403/rc
Data Sharing Statement: Available at https://tlcr.amegroups.com/article/view/10.21037/tlcr-22-403/dss
Conflicts of Interest: All authors have completed the ICMJE uniform disclosure form (available at https://tlcr.amegroups.com/article/view/10.21037/tlcr-22-403/coif). JWN reports grants and personal fees from Takeda, during the conduct of the study; grants, personal fees, and non-financial support from Genentech/Roche, Exelixis; personal fees from AstraZeneca, Jounce Therapeutics, Eli Lilly and Company, Calithera Biosciences, Amgen, Iovance Biotherapeutics, Blueprint Pharmaceuticals, Regeneron Pharmaceuticals, Natera, Surface Oncology, D2G Oncology, Sanofi Genzyme, and Turning Point Therapeutics; grants and non-financial support from Merck, Novartis, Boehringer Ingelheim, Nektar Therapeutics, Adaptimmune, GSK, Janssen, and AbbVie, outside the submitted work. The other authors have no conflicts of interest to declare.
Ethical Statement: The authors are accountable for all aspects of the work in ensuring that questions related to the accuracy or integrity of any part of the work are appropriately investigated and resolved. The study was conducted in accordance with the Declaration of Helsinki (as revised in 2013). The study was approved by ethics committee of the Fourth Military Medical University (No. TDLL-202206-06) and informed consents were not requested for this retrospective study.
Open Access Statement: This is an Open Access article distributed in accordance with the Creative Commons Attribution-NonCommercial-NoDerivs 4.0 International License (CC BY-NC-ND 4.0), which permits the non-commercial replication and distribution of the article with the strict proviso that no changes or edits are made and the original work is properly cited (including links to both the formal publication through the relevant DOI and the license). See: https://creativecommons.org/licenses/by-nc-nd/4.0/.
References
- Bray F, Ferlay J, Soerjomataram I, et al. Global cancer statistics 2018: GLOBOCAN estimates of incidence and mortality worldwide for 36 cancers in 185 countries. CA Cancer J Clin 2018;68:394-424. Erratum in: CA Cancer J Clin 2020;70:313. [Crossref] [PubMed]
- Herbst RS, Morgensztern D, Boshoff C. The biology and management of non-small cell lung cancer. Nature 2018;553:446-54. [Crossref] [PubMed]
- Cao J, Sun L, Aramsangtienchai P, et al. HDAC11 regulates type I interferon signaling through defatty-acylation of SHMT2. Proc Natl Acad Sci U S A 2019;116:5487-92. [Crossref] [PubMed]
- Seto E, Yoshida M. Erasers of histone acetylation: the histone deacetylase enzymes. Cold Spring Harb Perspect Biol 2014;6:a018713. [Crossref] [PubMed]
- Bhaskara S. Histone deacetylase 11 as a key regulator of metabolism and obesity. EBioMedicine 2018;35:27-8. [Crossref] [PubMed]
- Haberland M, Montgomery RL, Olson EN. The many roles of histone deacetylases in development and physiology: implications for disease and therapy. Nat Rev Genet 2009;10:32-42. [Crossref] [PubMed]
- Liu H, Hu Q, Kaufman A, et al. Developmental expression of histone deacetylase 11 in the murine brain. J Neurosci Res 2008;86:537-43. [Crossref] [PubMed]
- Gao L, Cueto MA, Asselbergs F, et al. Cloning and functional characterization of HDAC11, a novel member of the human histone deacetylase family. J Biol Chem 2002;277:25748-55. [Crossref] [PubMed]
- Yanginlar C, Logie C. HDAC11 is a regulator of diverse immune functions. Biochim Biophys Acta Gene Regul Mech 2018;1861:54-9. [Crossref] [PubMed]
- Villagra A, Cheng F, Wang HW, et al. The histone deacetylase HDAC11 regulates the expression of interleukin 10 and immune tolerance. Nat Immunol 2009;10:92-100. [Crossref] [PubMed]
- Klieser E, Urbas R, Stättner S, et al. Comprehensive immunohistochemical analysis of histone deacetylases in pancreatic neuroendocrine tumors: HDAC5 as a predictor of poor clinical outcome. Hum Pathol 2017;65:41-52. [Crossref] [PubMed]
- Wang W, Fu L, Li S, et al. Histone deacetylase 11 suppresses p53 expression in pituitary tumor cells. Cell Biol Int 2017;41:1290-5. [Crossref] [PubMed]
- Dai W, Zeller C, Masrour N, et al. Promoter CpG island methylation of genes in key cancer pathways associates with clinical outcome in high-grade serous ovarian cancer. Clin Cancer Res 2013;19:5788-97. [Crossref] [PubMed]
- Thole TM, Lodrini M, Fabian J, et al. Neuroblastoma cells depend on HDAC11 for mitotic cell cycle progression and survival. Cell Death Dis 2017;8:e2635. [Crossref] [PubMed]
- Dali-Youcef N, Froelich S, Moussallieh FM, et al. Gene expression mapping of histone deacetylases and co-factors, and correlation with survival time and 1H-HRMAS metabolomic profile in human gliomas. Sci Rep 2015;5:9087. [Crossref] [PubMed]
- Lucio-Eterovic AK, Cortez MA, Valera ET, et al. Differential expression of 12 histone deacetylase (HDAC) genes in astrocytomas and normal brain tissue: class II and IV are hypoexpressed in glioblastomas. BMC Cancer 2008;8:243. [Crossref] [PubMed]
- Herlihy N, Dogrusöz M, van Essen TH, et al. Skewed expression of the genes encoding epigenetic modifiers in high-risk uveal melanoma. Invest Ophthalmol Vis Sci 2015;56:1447-58. [Crossref] [PubMed]
- Goldstraw P, Crowley J, Chansky K, et al. The IASLC Lung Cancer Staging Project: proposals for the revision of the TNM stage groupings in the forthcoming (seventh) edition of the TNM Classification of malignant tumours. J Thorac Oncol 2007;2:706-14. [Crossref] [PubMed]
- Szklarczyk D, Morris JH, Cook H, et al. The STRING database in 2017: quality-controlled protein-protein association networks, made broadly accessible. Nucleic Acids Res 2017;45:D362-8. [Crossref] [PubMed]
- Nixon NA, Blais N, Ernst S, et al. Current landscape of immunotherapy in the treatment of solid tumours, with future opportunities and challenges. Curr Oncol 2018;25:e373-e384. [Crossref] [PubMed]
- Topper MJ, Vaz M, Marrone KA, et al. The emerging role of epigenetic therapeutics in immuno-oncology. Nat Rev Clin Oncol 2020;17:75-90. [Crossref] [PubMed]
- Witt O, Deubzer HE, Milde T, et al. HDAC family: What are the cancer relevant targets? Cancer Lett 2009;277:8-21. [Crossref] [PubMed]
- Yang XJ, Seto E. The Rpd3/Hda1 family of lysine deacetylases: from bacteria and yeast to mice and men. Nat Rev Mol Cell Biol 2008;9:206-18. [Crossref] [PubMed]
- Thangapandian S, John S, Lee Y, et al. Molecular modeling study on tunnel behavior in different histone deacetylase isoforms. PLoS One 2012;7:e49327. [Crossref] [PubMed]
- Mithraprabhu S, Kalff A, Chow A, et al. Dysregulated Class I histone deacetylases are indicators of poor prognosis in multiple myeloma. Epigenetics 2014;9:1511-20. [Crossref] [PubMed]
- Buglio D, Khaskhely NM, Voo KS, et al. HDAC11 plays an essential role in regulating OX40 ligand expression in Hodgkin lymphoma. Blood 2011;117:2910-7. [Crossref] [PubMed]
- Sahakian E, Powers JJ, Chen J, et al. Histone deacetylase 11: A novel epigenetic regulator of myeloid derived suppressor cell expansion and function. Mol Immunol 2015;63:579-85. [Crossref] [PubMed]
- Shinohara H, Kuranaga Y, Kumazaki M, et al. Regulated Polarization of Tumor-Associated Macrophages by miR-145 via Colorectal Cancer-Derived Extracellular Vesicles. J Immunol 2017;199:1505-15. [Crossref] [PubMed]
- Gong D, Zeng Z, Yi F, et al. Inhibition of histone deacetylase 11 promotes human liver cancer cell apoptosis. Am J Transl Res 2019;11:983-90. [PubMed]
- Bi L, Ren Y, Feng M, et al. HDAC11 Regulates Glycolysis through the LKB1/AMPK Signaling Pathway to Maintain Hepatocellular Carcinoma Stemness. Cancer Res 2021;81:2015-28. [Crossref] [PubMed]
- Mostofa A, Distler A, Meads MB, et al. Plasma cell dependence on histone/protein deacetylase 11 reveals a therapeutic target in multiple myeloma. JCI Insight 2021;6:151713. [Crossref] [PubMed]
- Leslie PL, Chao YL, Tsai YH, et al. Histone deacetylase 11 inhibition promotes breast cancer metastasis from lymph nodes. Nat Commun 2019;10:4192. [Crossref] [PubMed]
- Wang S, Cheng Q. Microarray analysis in drug discovery and clinical applications. Methods Mol Biol 2006;316:49-65. [Crossref] [PubMed]
- Coussens LM, Werb Z. Inflammation and cancer. Nature 2002;420:860-7. [Crossref] [PubMed]
- Kwabi-Addo B, Wang S, Chung W, et al. Identification of differentially methylated genes in normal prostate tissues from African American and Caucasian men. Clin Cancer Res 2010;16:3539-47. [Crossref] [PubMed]
- Feng L, Hatten ME, Heintz N. Brain lipid-binding protein (BLBP): a novel signaling system in the developing mammalian CNS. Neuron 1994;12:895-908. [Crossref] [PubMed]
- Liu Y, Longo LD, De León M. In situ and immunocytochemical localization of E-FABP mRNA and protein during neuronal migration and differentiation in the rat brain. Brain Res 2000;852:16-27. [Crossref] [PubMed]
- Liang Y, Bollen AW, Aldape KD, et al. Nuclear FABP7 immunoreactivity is preferentially expressed in infiltrative glioma and is associated with poor prognosis in EGFR-overexpressing glioblastoma. BMC Cancer 2006;6:97. [Crossref] [PubMed]
- Mita R, Coles JE, Glubrecht DD, et al. B-FABP-expressing radial glial cells: the malignant glioma cell of origin? Neoplasia 2007;9:734-44. [Crossref] [PubMed]
- Li Y, Seto E. HDACs and HDAC Inhibitors in Cancer Development and Therapy. Cold Spring Harb Perspect Med 2016;6:a026831. [Crossref] [PubMed]