Causal effects of genetically determined metabolites on cancers included lung, breast, ovarian cancer, and glioma: a Mendelian randomization study
Introduction
Previous researches have shown that metabolites are crucial factor affecting tumorigenesis. For example, liver kinase B1 (LKB1) mutant lung cancers have deficits in nucleotide metabolism that confer hypersensitivity to deoxythymidylate kinase inhibition (1). N-acetylaspartic acid plays a significant role in promoting tumor growth (2). 2-hydroxyglutarate is abnormally elevated in glioma (3). However, a definitive comprehensive summary of the causal effect between metabolites and tumors is scarce, which is the purpose of this research.
Metabolites are the substrates and products of biological metabolism that belong to host organisms and are also produced directly by microorganisms and xenobiotics (4). Metabolomics is the profiling of metabolites biofluids, cells, and tissues, which are regularly applied as a biomarker discovery tool (5). Biomarkers obtained from human studies can help find links between diseases and metabolic pathways (6). Owing to the sensitivity of metabolomics, subtle alterations in biological behavior can be detected to provide insight into the mechanisms that underlie various physiological status and aberrant processes, as well as incorporated disease (4). Though there is a long history of metabolite identification and validation, one of the biggest challenges in biomarker validation is to overcome inter-individual metabolite variation due to the divergence in genetic factors and environmental exposures (7,8). The identification of the metabolites and their biological roles is the essential step. Under these conditions, many studies have shown the tremendous potential of metabolomics in cancer research (9,10).
Cancer is a major public health problem worldwide, especially lung cancer and breast cancer. In 2021, the estimated death rate of lung cancer is still at the top of the list, though the morbidity of breast cancer exceeded lung cancer for the first time. High-mortality has become the label for ovarian cancer and glioma (included in the brain and other nervous systems) (11). Though numerous researches have been carried out to explore these tumors, involving the molecular mechanism, cytological behavior, and biological process, etc., our understandings of the above issues are still limited (12,13). Fortunately, genetics, especially genome-wide association studies (GWAS), emerged as a fundamental pillar in many research areas (14,15), providing more dimensions to explore the causes of cancer.
Mendelian randomization (MR) is a general method based on GWAS summary data to evaluate the causal effects between exposure and outcome using genetic variants as instrumental variables (IVs) (16). In other words, genetic variants from GWAS can be used to mimic a randomized controlled comparison to verify causality between various factors (9,10). Moreover, GWAS has been extended to metabolic profiles. Therefore, this study aimed to use two-sample MR approach to detect the causal effects of genetically determined metabotypes (GDMs) on lung cancer (LC), breast cancer (BC), ovarian cancer (OC), and glioma, due to the limitations of online data access, only four cancers were included in this research. We present the following article in accordance with the STREGA reporting checklist (available at https://tlcr.amegroups.com/article/view/10.21037/tlcr-22-34/rc).
Methods
Genetic variants from GWAS of metabolites
The entire methology implementation process is presented in Figure 1. GWAS summary datasets for 486 metabolites were detached from the study by Shin et al. (8), which has been the most comprehensive investigation of the genetic effects on human serum metabolism so far. Amount to 7,824 samples of two cohorts comprising of 1,768 participants from Germany KORA F4 and 6,056 participants from British Twins UK cohort. The KORA dataset and Twins UK dataset have been described in previous studies (8,16). The metabolites profiles of 486 fasting serum samples were analyzed by liquid-phase chromatography and gas chromatography (17). Sample preparation, mass spectrometry analysis, compound identification, quantification, and data curation were carried out for metabolic analysis by Metabolon, Inc. (https://www.metabolon.com/). After stringent quality control, 486 metabolites were used for genetic analysis, including 309 known and 177 unknown metabolites. The 309 known metabolites were further divided into 8 broad metabolic classes (amino acids, carbohydrates, cofactors and vitamins, energy, lipids, nucleotides, peptides, and xenobiotic metabolism) as described in the Kyoto Encyclopedia of Genes and Genomes (KEGG) database (18). The direct genotyping and imputation steps of the two cohorts were completed by HapMap 2-panel couple with passing stringent quality control metrics, and genome-wide discovery analysis was done based on approximately 2.1 million single nucleotide polymorphism (SNP) (19). Full GWAS summary datasets statistics were publicly available through the Metabolomics GWAS Server at http://metabolomics.helmholtz-muenchen.de/gwas/.
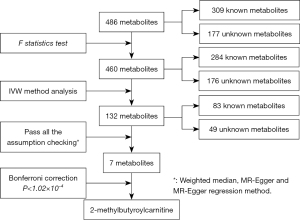
Selection criteria of instrumental variables for 486 metabolites
At the beginning, the unitary criteria were carried out for selecting SNPs from the 486 serum metabolites. we selected SNPs listed in the summary statistic, which have passed quality control with the P<1×10-5, this relaxation statistical threshold was commonly implemented in MR study to account for greater variation when few genome-wide significant SNPs were available for exposures (20). the clumping procedure was done by linkage disequilibrium (LD) analysis with lower P value as independent instruments while setting the LD threshold of r2<0.001 in a 10,000-kb window in the European 1000 Genomes reference panel (21). Furthermore, next steps to assess whether these instrumental variables were strong enough to predict the causal effect by two parameters: the explained genetic variation (R2) and F statistic. the former associated with the corresponding metabolite exposures by formula and the F statistic was calculated to avoid weak instrument bias based on the formula F=R2(n−k−1)/(1−R2)k, which n is the sample size and k represents the number if SNPs (Table S1) (22). Widely speaking, an F statistic of more than 10 was taken for a typical threshold for selecting strong instrumental variables. The F statistic of each metabolite less than 10 will be screened out (23).
Genetic variants from GWAS of cancer
To evaluate the potential causal relationship between metabolites with four types of cancers, four GWAS summary statistics for cancers form different consortias were included. The integration of different population according to cancer type originated from the consortia: International Lung Cancer Association Consortia (ILCCO) (24) for lung cancer [including 11,348 cases and 15,861 controls, histologically, it can be divided into lung adenocarcinoma (LAC) and squamous cell cancer], Breast Cancer Association Consortium (BCAC) (25) for breast cancer (including 122,977 cases and 105,974 controls, divided into ER-positive breast cancer and ER-negative breast cancer), Ovarian Cancer Association Consortium (OCAC) (26) for ovarian cancer (including 25,509 cases and 40,941 controls). The GWAS about glioma comprised 14 cohorts, 3 case-control studies, and 1 population-based case-only study (including 6,811 cases) (27) (see Table S2).
MR statistical analysis
The rationale of MR was shown in Figure S1. Inverse variance weighted (IVW) method was used to estimate the causal effects between metabolites and four primary cancers (28). And then we utilized a multiple-testing adjusted threshold of P<1.02×10−4 (0.05/486) using the Bonferroni correction to clarify the statistical significance (29). Moreover, results can be biased if instrument SNPs show horizontal pleiotropy and influence the outcome through causal pathways other than the exposure (30). Therefore, the IVs should follow three assumptions: (I) the IVs are strongly associated with the serum metabolites; (II) the IVs affect cancers only through their effect on the serum metabolites; and (III) the IVs are independent of any confounding factors (21). Accordingly, the weighted median, MR-Egger and leave-one-out methods were implemented for sensitivity analysis and to test the second assumption (20). The weighted median and MR-Egger method provided estimates when a subset <50% or up to 50% of the variants came from invalid instrumental variants separately (30,31), leave-one-out method is the most commonly used test for sensitivity. Circos plots were conducted to summarize and visually compare the IVW MR results, MR-Egger estimates and weighted median estimates. Moreover, we particularly detected the presentence of horizontal pleiotropy through MR-Egger regression and MR-PRESSO Global test (32). Finally, subgroup analyses were further explored using the available data.
MR analysis was conducted in R (version 3.6.2) using the package “TwoSampleMR” (version 0.5.5) (21), “MR-PRESSO” (33) and Circos plots were generated using EpiViz (version 0.1.0), a R package built under R (version 4.0.5), Epiviz was built under ComplexHeatmap (34) and Circlize (35) R packages to generate Circos plots to compare association analysis data. The study was conducted in accordance with the Declaration of Helsinki (as revised in 2013) (36).
Metabolic pathway analysis
After MR analysis, we next conducted a metabolic pathway analysis for the identified metabolites using web-based MetaboAnalyst 5.0 software (https://www.metaboanalyst.ca/MetaboAnalyst/faces/home.xhtml) (37), which All the metabolites was identified by IVW at PIVW <0.05. When used pathway analysis module to probe potential pathways that might be involved in the biological processes of the four main cancers, a total of 49 human serum metabolic pathways from two metabolite set libraries, including 44 metabolite sets from both The Small Molecule Pathway Database (SMPDB) and KEGG database, 4 metabolites sets from KEGG database solely.
Results
Study overview
We performed a two-sample MR analysis to assess the causal effects of human serum metabolites on four primary cancers using GWAS summary statistics. For assess the causality between each metabolites with the outcome, we extracted the genetic variants as instrumental variables. The entire filtrate flow was shown in Figure 1. The instrumental variants explained from 0.01% to 9.37% in their respective phenotypes. The minimum F statistic for validity tests of genetic predictors was 17.21, which illustrate all instrumental variables for the 486 metabotypes were sufficiently credible (F statistic >10) (detail see Tables S3-S10).
Causal effects of the 486 metabolites on cancer
By using the genetic variants as proxies, the IVW method was carried out, and 202 significant causative relationship features (corresponding to 132 unique metabolites) in total were identified at P<0.05, including 83 (62.8%) features for known metabolites and 49 features for unknown metabolites (Tables S10,S11). Specifically, 7-alpha-hydroxy-3-oxo-4-cholestenoate (7-Hoca) (ORIVW =1.45; 95% CI: 1.06–1.97; PIVW =0.018), gamma-glutamylisoleucine (ORIVW =1.40; 95% CI: 1.16–1.69; PIVW =0.0004), 1-oleoylglycerophosphocholine (ORIVW =1.22; 95% CI: 1.1–1.35; PIVW =0.0001), gamma-glutamylleucine (ORIVW =4.74; 95% CI: 1.18-18.93; PIVW =0.027) were the most significantly dangerous metabolites for lung cancer, ovarian cancer, breast cancer, and glioma, respectively. On the contrary, pseudouridine (ORIVW =0.50; 95% CI: 0.30–0.83; PIVW =0.007), 2-methylbutyroylcarnitine (ORIVW =0.77; 95% CI: 0.68–0.86; PIVW =3.0×10−6), 2-methylbutyroylcarnitine (ORIVW =0.77; 95% CI: 0.70–0.85; PIVW =3.4×10−7), glycylvaline (ORIVW =0.13; 95% CI: 0.02–0.75; PIVW =0.021) were factors with highest protective value for lung cancer, ovarian cancer, breast cancer, and glioma, respectively (see Table 1). Figure 2 showed all significant causative relationship features between known and unknown metabolites of the different types of cancer. Furthermore, causal relationship features following a multiple-testing-adjusted threshold under the Bonferroni correction (P<1.02×10−4) were used to obtain metabolites. Interestingly, we observed that 2-methylbutyroylcarnitine had a protective value on several types of cancers. Specifically, it lower the incidence of lung cancer (ORIVW =0.59; 95% CI: 0.50–0.70; PIVW =1.98×10−9), breast cancer (ORIVW =0.77; 95% CI: 0.70–0.85; PIVW =3.4×10−7), and ovarian cancer (ORIVW =0.77; 95% CI: 0.68–0.86; PIVW =3.0×10−6). In addition, it is notable that 2-methylbutyroylcarnitine was associated with higher mortality on glioma (ORIVW =2.19; 95% CI: 1.17–4.09; P=0.015), which potentially indicated the 2-methylbutyroylcarnitine shares similar biological mechanism among the three cancers except glioma (Table 2 and Figures S2-S5). These genetic variants explaining the association between 2-methylbutyroylcarnitine with four types of cancers were listed in Tables S12-S15 individually.
Table 1
Trait | Exposure | IVW | MR-Egger | Weighted median | |||||
---|---|---|---|---|---|---|---|---|---|
OR (95% CI) | P value | OR (95% CI) | P value | OR (95% CI) | P value | ||||
Lung cancer | 7-alpha-hydroxy-3-oxo-4-cholestenoate | 1.45 (1.06–1.97) | 0.0184 | 1.30 (0.74–2.26) | 0.3625 | 1.15 (1.01–1.36) | 0.0184 | ||
Lung cancer | Pseudouridine | 0.50 (0.30–0.83) | 0.0070 | 0.72 (0.17–3.06) | 0.6536 | 0.65 (0.31–1.34) | 0.2394 | ||
Ovarian cancer | Gamma-glutamylisoleucine | 1.40 (1.16–1.69) | 0.0004 | 1.18 (0.80–1.74) | 0.4098 | 1.33 (0.99–1.79) | 0.0570 | ||
Ovarian cancer | 2-methylbutyroylcarnitine | 0.77 (0.68–0.86) | 2.995E-06 | 1.17 (0.90–1.53) | 0.245 | 0.63 (0.52–0.0.75) | 0.000 | ||
Breast cancer | 1-oleoylglycerophosphocholine | 1.22 (1.1–1.35) | 0.0001 | 1.19 (0.97–1.45) | 0.0917 | 1.17 (1.02–1.34) | 0.0273 | ||
Breast cancer | 2-methylbutyroylcarnitine | 0.77 (0.70–0.85) | 3.418E-07 | 1.59 (1.27–1.99) | 6.233E-05 | 1.004 (0.91–1.11) | 0.935 | ||
Glioma | Gamma-glutamylleucine | 4.74 (1.18–18.93) | 0.0278 | 3.18 (0.03–296.7) | 0.6193 | 7.93 (1.03–61.02) | 0.0466 | ||
Glioma | Glycylvaline | 0.13 (0.02–0.75) | 0.0217 | 0.12 (0.0002–87.65) | 0.5347 | 0.09 (0.0071–1.20) | 0.0683 |
IVW, inverse variance weighted; MR, Mendelian randomization.
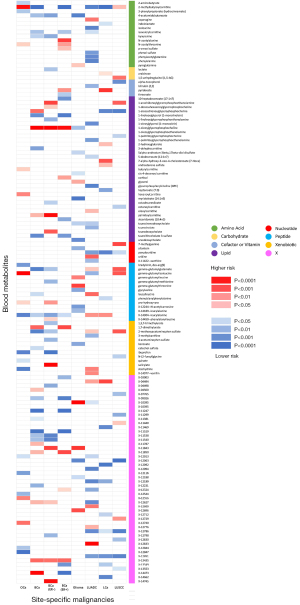
Table 2
Cancer type | IVW | MR-Egger | Weighted median | MR-PRESSO | MR-Egger regression | ||||||
---|---|---|---|---|---|---|---|---|---|---|---|
OR (95% CI) | P value | OR (95% CI) | P value | OR (95% CI) | P value | Egger intercept | Pleiotropy test | ||||
Breast cancer | 0.77 (0.70–0.85) | 3.42E-07 | 1.59 (1.27–1.99) | 6.23E-05 | 1.01 (0.91–1.11) | 9.35E-01 | 0.682 | −0.0045 | 0.0458 | ||
ER+ breast cancer | 0.72 (0.64–0.80) | 3.55E-09 | 1.58 (1.23–2.02) | 3.06E-04 | 1.00 (0.90–1.12) | 9.45E-01 | 0.792 | −0.0038 | 0.1506 | ||
Glioma | 2.19 (1.17–4.09) | 0.015 | 0.89 (0.16–4.96) | 8.95E-01 | 1.99 (0.75–5.31) | 1.67E-01 | 0.216 | −0.0054 | 0.8026 | ||
Lung cancer | 0.59 (0.50–0.70) | 1.98E-09 | 1.60 (1.08–2.36) | 1.91E-01 | 0.85 (0.66–1.09) | 2.03E-01 | <0.001 | −0.0025 | 0.6381 | ||
Lung adenocarcinoma | 0.60 (0.48–0.75) | 1.14E-05 | 1.72 (1.005–2.96) | 4.82E-02 | 0.59 (0.41–0.87) | 7.20E-03 | 0.123 | −0.0002 | 0.9826 | ||
Squamous cell lung cancer | 0.78 (0.63–0.98) | 3.3E-02 | 1.97 (1.17–3.32) | 1.12E-02 | 1.28 (0.89–1.84) | 1.79E-01 | 0.452 | −0.0079 | 0.3344 | ||
Ovarian cancer | 0.77 (0.68–0.86) | 3.00E-06 | 1.17 (0.90–1.53) | 2.45E-01 | 0.63 (0.52–0.75) | 4.77E-07 | 0.319 | −0.0040 | 0.3235 |
ER, estrogen receptor; IVW, inverse variance weighted; MR, Mendelian randomization.
Sensitivity and pleiotropy analysis
To avoid horizontal pleiotropy for MR research, sensitivity and pleiotropy analysis was implemented to evaluate the robustness of the estimates, all results were demonstrated in Tables S3-S10. Especially, Table 3 demonstrated the results of the sensitivity and pleiotropy analysis for 2-methylbutyroylcarnitine on the four primary cancers (see Figure S4). Besides, we found pleiotropy in 2-methylbutyroylcarnitine on breast cancer (Ppleiotropy =0.045) and lung cancer (PMR-PRESSO Global <0.0001). The result of IVW, MR-Egger and weighted-median of all known metabolites were integrated shown in Figure 3.
Table 3
Cancer type | Metabolite | Included SNP | IVW | MR-Egger | Weighted median | |||||
---|---|---|---|---|---|---|---|---|---|---|
OR (95% CI) | P value | OR (95% CI) | P value | OR (95% CI) | P value | |||||
Breast cancer | 3-dehydrocarnitine | 22 | 0.88 (0.78–0.98) | 0.0194 | 0.77 (0.60–0.995) | 0.047623 | 0.81 (0.70–0.95) | 0.00790012 | ||
ER− breast cancer | 1-oleoylglycerophosphocholine | 16 | 1.38 (1.17–1.62) | 0.0001 | 1.44 (1.05–1.99) | 0.026764 | 1.36 (1.07–1.73) | 0.01233648 | ||
Salicylate | 19 | 1.04 (1.01–1.06) | 0.0009 | 1.05 (1.02–1.08) | 0.002736 | 1.05 (1.02–1.09) | 0.00102991 | |||
Lung cancer | Leucylalanine | 19 | 1.16 (1.01–1.32) | 0.031 | 1.70 (1.11–2.61) | 0.017587 | 1.37 (1.12–1.66) | 0.0019708 | ||
Squamous cell lung cancer | Octanoylcarnitine | 17 | 0.74 (0.55–0.98) | 0.038 | 0.53 (0.29–0.95) | 0.036358 | 0.58 (0.36–0.92) | 0.0204581 | ||
Ovarian cancer | Ibuprofen | 101 | 0.96 (0.93–0.99) | 0.007 | 0.92 (0.85–0.99) | 0.033462 | 0.95 (0.91–0.995) | 0.03205872 | ||
Leucylalanine | 19 | 0.96 (0.93–0.99) | 0.007 | 0.92 (0.85–0.99) | 0.033462 | 0.95 (0.91–0.995) | 0.03205872 |
IVW, inverse variance weighted; MR, Mendelian randomization; ER, estrogen receptor; SNP, single nucleotide polymorphism.
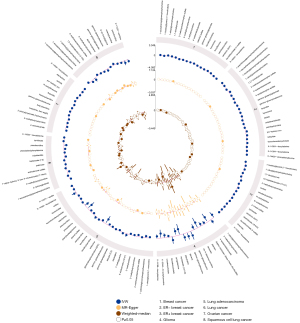
We further reported four suggestive association features that passed all sensitive analyses (P<0.05) (see Table 3 and Figures S6-S9), which respectively were leucylalanine on lung cancer (ORIVW =1.16; 95% CI: 1.01–1.32; PIVW =0.031), 3-dehydrocarnitine on breast cancer (ORIVW =0.88; 95% CI: 0.78–0.98; PIVW =0.019), ibuprofen on ovarian cancer (ORIVW =0.96; 95% CI: 0.93–0.99; PIVW =0.007), leucylalanine on ovarian cancer (ORIVW =0.96; 95% CI: 0.93–0.99; PIVW =0.007). These genetic variants explaining the association between the four metabolites with three types of cancers were listed in Tables S16-S19.
Subgroup analysis of lung cancer and breast cancer
In the exposure obtained from ILCCO and BCAC, lung cancer was divided into adenocarcinoma and squamous cell carcinoma; breast cancer was divided into ER-positive and ER-negative cancer. 2-methylbutyroylcarnitine was associated with protective effects on LAC (ORIVW =0.60; 95% CI: 0.48–0.70; PIVW =1.14×10−5) and ER-positive breast cancer (ORIVW =0.72; 95% CI: 0.64–0.80; PIVW =3.55×10−9) statistically under Bonferroni correction (P<1.02×10−4), but not in ER-negative breast cancer (ORIVW =1.06; 95% CI: 0.88–1.26; PIVW =0.55) and squamous cell lung cancer (SCLC) (ORIVW =0.78; 95% CI: 0.63–0.98; PIVW =0.03), these result might provide potential evidence for the biogenetic mechanisms of different tumors. It is worth noting that the causal association between 2-methylbutyroylcarnitine and LAC was robust when two additional MR tests were conducted (Pweighted-median =0.007, PMR-Egger =0.019). These genetic variants explaining the association between 2-methylbutyroylcarnitine with two types of cancers were listed in Tables S20,S21. Results were consistent in sensitivity analyses, which were listed in Tables S22-S24.
Metabolic pathway analysis
The metabolic pathway analysis identified four metabolic pathways among the four cancers at P<0.05. the results show that “Vitamin B6 metabolism (P=0.028) and Butanoate metabolism (P=0.047)” pathway might be involved in the genesis of lung cancer. “Aminoacyl-tRNA biosynthesis (P=0.006) and Phenylalanine, tyrosine and tryptophan biosynthesis (P=0.033)” pathway might be associated with LAC (Table S25).
Discussion
This MR study provided potential causal effects of human serum metabolites on four primary cancers using standard IVW and alternative weighted median, MR-Egger method. Using genetic variants as proxies, we observed 137 metabolites associated with the risk of cancers. Specifically, 2-methylbutyroylcarnitine, leucylalanine, 3-dehydrocarnitine, ibuprofen, salicylate, 1-oleoylglycerophosphocholine and octanoylcarnitine, were closely related to different cancers, which may play roles in oncogenesis.
In this study, 2-methylbutyroylcarnitine showed a low to moderate protective effect associated with different cancers. In previous research, serum 2-methylbutyroylcarnitine was lower in obese children than normal-weighted children (1.38 folds), but the exact mechanism was unclear (38). We speculate that this metabolite could be involved in tumor genesis through this protective aspect. It is interesting to note the causal relationship presented by different results in the subgroup analysis of lung cancer and breast cancer, which validated the different driving mechanisms (39-41). However, some unknown factors are still involved, leading to bias or the single instrumental variable significantly affects the outcome variable. Therefore, considering that few study has been published on this metabolite, more researches related to this metabolite are warranted to be conducted in the future to elaborate its influence on tumor pathophysiology.
As for the potential metabolites, we found more researches about them. One study about leucylalanine suggested this metabolite is more focused on anti-inflammatory and cardiovascular activities and toxicity (42). On the basis of speculation combined with the mendelian causal effect, leucylalanine may be a protective metabolite in ovarian cancer. But the possible mechanism related to lung cancer is unclear. 3-dehydrocarnitine, a member of the carnitine family, is an intermediate in carnitine degradation, which has long been associated with fatty acid metabolism, glucose tolerance, insulin function (43). Fatty acid metabolism has long been associated to cancer cell metabolism. Limited fatty acid available may control cancer cell proliferation. A research found that fatty acid metabolism contains unexplored plasticity in the cancer cell and tried to explain the metabolic plasticity in fatty acid desaturation (44,45). “Warburg effect” seems to be cancer’s favorite, i.e., the enhanced glycolysis or aerobic glycolysis, even when the ambient oxygen supply is sufficient (46-48). Moreover, 3-dehydrocarnitine is an early biomarker for predicting type 2 diabetes, with applications even prior to the development of insulin resistance (49). During the tumor promotional microenvironment in the mammary gland, Ibuprofen administration reduces overall tumor growth and enhances anti-tumor immune characteristics while avoiding adverse autoimmune reactions (50). But its real-world representation remains vague (51,52). One of the enabling characteristics of cancer development is tumor related inflammation and chronic inflammatory disease did promote the possibility of tumor occurrence (53). More notably, non-steroidal anti-inflammatory drugs (NSAIDs) combined with aromatase inhibitors reduce circulating E2, proinflammatory cytokines, and macrophage recruitment in the lung microenvironment after tobacco exposure, which were proved at preclinical studies as preventive agents of tobacco-induced lung cancer (54). The only one study could be retrieved referred to concentration of 1-oleoylglycerophosphocholine was higher when compared to the normal wild type rat using high mass accuracy electrospray ionization multistage tandem mass spectrometry (55). In a cohort study of metabolites involved in chronic disease by the effects of vegetarian dietary patterns, vegans showed lower abundance in acylcarnitine, and many subclasses of this metabolite may play a role in insulin dysregulation, inflammation, and so on (56) together with the effect on myocardium (57) and arterial stiffness (58)
There are some advantages to our study. Firstly, this research is the first MR study associating metabolomics with genomics to detect the causal relation of serum metabolites on cancers. Secondly, the SNPs from consortia we selected have been verified by peer review and have sufficient sample sizes. Thirdly, the methods chosen to evaluate the causal relationship between metabolites and cancers are valid, including IVW, weighted median and MR-Egger. Furthermore, rigorous Bonferroni correction was implemented, and we manually removed the non-significant genetic variants (P<1×10−5) in double verification.
A few drawbacks, however, exist in our study. Firstly, as the MR principle evolving these years, compared with adopting existed ones, refining the methodology and choosing better models is more meaningful. Secondly, some of the result of weighted median and MR-Egger between 2-methylbutyroylcarnitine with some types of cancers except LAC are not up to standard (P<0.05), which the weighted median and MR-Egger method needed and be marginalized by statistical calculation. Thirdly, due to the data availability, we could only access the four major cancers data included in the research , but those four tumors were typically representative.
Conclusions
To summarize, our MR study identified 132 metabolites that probably have causal effects on the progress of cancers. Interestingly, 38 metabolites have causal effects on more than one cancer, implying some overlapped metabolic pathways among four different cancers. Our study could also be used as the basis for other cancer research and combine with translational and clinical research to explain how the metabolites induce the development of cancers, Finally, although our study identified many metabolites associated to the incidence rate of cancers, further investigations are needed to reveal their functions in the pathogenesis of relevant diseases in the future.
Acknowledgments
We sincerely thank Qiwen Liu, Yining Wei, Na Jin, Ruiyu Hu, Zicong Deng, and Haotian Zhang for their efforts in many contributions in this study.
Funding: This study was supported by the China National Science Foundation (Grant Nos. 82022048 & 81871893), and the Key Project of Guangzhou Scientific Research Project (Grant No. 201804020030).
Footnote
Reporting Checklist: The authors have completed the STREGA reporting checklist. Available at https://tlcr.amegroups.com/article/view/10.21037/tlcr-22-34/rc
Conflicts of Interest: All authors have completed the ICMJE uniform disclosure form (available at https://tlcr.amegroups.com/article/view/10.21037/tlcr-22-34/coif). The authors have no conflicts of interest to declare.
Ethical Statement: The authors are accountable for all aspects of the work in ensuring that questions related to the accuracy or integrity of any part of the work are appropriately investigated and resolved. And the study was conducted in accordance with the Declaration of Helsinki (as revised in 2013).
Open Access Statement: This is an Open Access article distributed in accordance with the Creative Commons Attribution-NonCommercial-NoDerivs 4.0 International License (CC BY-NC-ND 4.0), which permits the non-commercial replication and distribution of the article with the strict proviso that no changes or edits are made and the original work is properly cited (including links to both the formal publication through the relevant DOI and the license). See: https://creativecommons.org/licenses/by-nc-nd/4.0/.
References
- Liu Y, Marks K, Cowley GS, et al. Metabolic and functional genomic studies identify deoxythymidylate kinase as a target in LKB1-mutant lung cancer. Cancer Discov 2013;3:870-9. [Crossref] [PubMed]
- Zand B, Previs RA, Zacharias NM, et al. Role of Increased n-acetylaspartate Levels in Cancer. J Natl Cancer Inst 2016;108:djv426. [Crossref] [PubMed]
- Elhammali A, Ippolito JE, Collins L, et al. A high-throughput fluorimetric assay for 2-hydroxyglutarate identifies Zaprinast as a glutaminase inhibitor. Cancer Discov 2014;4:828-39. [Crossref] [PubMed]
- Johnson CH, Patterson AD, Idle JR, et al. Xenobiotic metabolomics: major impact on the metabolome. Annu Rev Pharmacol Toxicol 2012;52:37-56. [Crossref] [PubMed]
- Koeth RA, Wang Z, Levison BS, et al. Intestinal microbiota metabolism of L-carnitine, a nutrient in red meat, promotes atherosclerosis. Nat Med 2013;19:576-85. [Crossref] [PubMed]
- Ridder L, van der Hooft JJ, Verhoeven S, et al. Automatic chemical structure annotation of an LC-MS(n) based metabolic profile from green tea. Anal Chem 2013;85:6033-40. [Crossref] [PubMed]
- Hamdalla MA, Ammar RA, Rajasekaran S. A molecular structure matching approach to efficient identification of endogenous mammalian biochemical structures. BMC Bioinformatics 2015;16:S11. [Crossref] [PubMed]
- Shin SY, Fauman EB, Petersen AK, et al. An atlas of genetic influences on human blood metabolites. Nat Genet 2014;46:543-50. [Crossref] [PubMed]
- Telomeres Mendelian Randomization Collaboration. Association Between Telomere Length and Risk of Cancer and Non-Neoplastic Diseases: A Mendelian Randomization Study. JAMA Oncol 2017;3:636-51. [Crossref] [PubMed]
- Day FR, Thompson DJ, Helgason H, et al. Genomic analyses identify hundreds of variants associated with age at menarche and support a role for puberty timing in cancer risk. Nat Genet 2017;49:834-41. [Crossref] [PubMed]
- CA: A Cancer Journal for Clinicians Statistics. 2021 [cited 2021 Jan 30]. Available online: https://acsjournals.onlinelibrary.wiley.com/doi/pdf/10.3322/caac.21654
- Wu Y, Williams EG, Dubuis S, et al. Multilayered genetic and omics dissection of mitochondrial activity in a mouse reference population. Cell 2014;158:1415-30. [Crossref] [PubMed]
- Johnson CH, Ivanisevic J, Benton HP, et al. Bioinformatics: the next frontier of metabolomics. Anal Chem 2015;87:147-56. [Crossref] [PubMed]
- Kunkle BW, Grenier-Boley B, Sims R, et al. Genetic meta-analysis of diagnosed Alzheimer's disease identifies new risk loci and implicates Aβ, tau, immunity and lipid processing. Nat Genet 2019;51:414-30. [Crossref] [PubMed]
- Wray NR, Ripke S, Mattheisen M, et al. Genome-wide association analyses identify 44 risk variants and refine the genetic architecture of major depression. Nat Genet 2018;50:668-81. [Crossref] [PubMed]
- Krumsiek J, Suhre K, Evans AM, et al. Mining the unknown: a systems approach to metabolite identification combining genetic and metabolic information. PLoS Genet 2012;8:e1003005. [Crossref] [PubMed]
- Jie K, Zhou Y, Li E, et al. Nonporous Adaptive Crystals of Pillararenes. Acc Chem Res 2018;51:2064-72. [Crossref] [PubMed]
- Kanehisa M, Goto S. KEGG: kyoto encyclopedia of genes and genomes. Nucleic Acids Res 2000;28:27-30. [Crossref] [PubMed]
- International HapMap Consortium. A second generation human haplotype map of over 3.1 million SNPs. Nature 2007;449:851-61. [Crossref] [PubMed]
- Choi KW, Chen CY, Stein MB, et al. Assessment of Bidirectional Relationships Between Physical Activity and Depression Among Adults: A 2-Sample Mendelian Randomization Study. JAMA Psychiatry 2019;76:399-408. [Crossref] [PubMed]
- Hemani G, Zheng J, Elsworth B, et al. The MR-Base platform supports systematic causal inference across the human phenome. Elife 2018;7:34408. [Crossref] [PubMed]
- Shim H, Chasman DI, Smith JD, et al. A multivariate genome-wide association analysis of 10 LDL subfractions, and their response to statin treatment, in 1868 Caucasians. PLoS One 2015;10:e0120758. [Crossref] [PubMed]
- Holsinger KE, Weir BS. Genetics in geographically structured populations: defining, estimating and interpreting F(ST). Nat Rev Genet 2009;10:639-50. [Crossref] [PubMed]
- Wang Y, McKay JD, Rafnar T, et al. Corrigendum: Rare variants of large effect in BRCA2 and CHEK2 affect risk of lung cancer. Nat Genet 2017;49:651. [Crossref] [PubMed]
- Michailidou K, Lindström S, Dennis J, et al. Association analysis identifies 65 new breast cancer risk loci. Nature 2017;551:92-4. [Crossref] [PubMed]
- Phelan CM, Kuchenbaecker KB, Tyrer JP, et al. Identification of 12 new susceptibility loci for different histotypes of epithelial ovarian cancer. Nat Genet 2017;49:680-91. [Crossref] [PubMed]
- Rajaraman P, Melin BS, Wang Z, et al. Genome-wide association study of glioma and meta-analysis. Hum Genet 2012;131:1877-88. [Crossref] [PubMed]
- Ettehad D, Emdin CA, Kiran A, et al. Blood pressure lowering for prevention of cardiovascular disease and death: a systematic review and meta-analysis. Lancet 2016;387:957-67. [Crossref] [PubMed]
- Sedgwick P. Multiple hypothesis testing and Bonferroni's correction. BMJ 2014;349:g6284. [Crossref] [PubMed]
- Bowden J, Davey Smith G, Burgess S. Mendelian randomization with invalid instruments: effect estimation and bias detection through Egger regression. Int J Epidemiol 2015;44:512-25. [Crossref] [PubMed]
- Bowden J, Davey Smith G, Haycock PC, et al. Consistent Estimation in Mendelian Randomization with Some Invalid Instruments Using a Weighted Median Estimator. Genet Epidemiol 2016;40:304-14. [Crossref] [PubMed]
- Hemani G, Bowden J, Davey Smith G. Evaluating the potential role of pleiotropy in Mendelian randomization studies. Hum Mol Genet 2018;27:R195-208. [Crossref] [PubMed]
- Verbanck M, Chen CY, Neale B, et al. Detection of widespread horizontal pleiotropy in causal relationships inferred from Mendelian randomization between complex traits and diseases. Nat Genet 2018;50:693-8. [Crossref] [PubMed]
- Gu Z, Eils R, Schlesner M. Complex heatmaps reveal patterns and correlations in multidimensional genomic data. Bioinformatics 2016;32:2847-9. [Crossref] [PubMed]
- Gu Z, Gu L, Eils R, et al. circlize Implements and enhances circular visualization in R. Bioinformatics 2014;30:2811-2. [Crossref] [PubMed]
- World Medical Association. World Medical Association Declaration of Helsinki: ethical principles for medical research involving human subjects. JAMA 2013;310:2191-4. [Crossref] [PubMed]
- Pang Z, Chong J, Zhou G, et al. MetaboAnalyst 5.0: narrowing the gap between raw spectra and functional insights. Nucleic Acids Res 2021;49:W388-96. [Crossref] [PubMed]
- Farook VS, Reddivari L, Chittoor G, et al. Metabolites as novel biomarkers for childhood obesity-related traits in Mexican-American children. Pediatr Obes 2015;10:320-7. [Crossref] [PubMed]
- Campbell JD, Alexandrov A, Kim J, et al. Distinct patterns of somatic genome alterations in lung adenocarcinomas and squamous cell carcinomas. Nat Genet 2016;48:607-16. [Crossref] [PubMed]
- Cancer Genome Atlas Research Network. Comprehensive molecular profiling of lung adenocarcinoma. Nature 2014;511:543-50. [Crossref] [PubMed]
- Cancer Genome Atlas Research Network. Comprehensive genomic characterization of squamous cell lung cancers. Nature 2012;489:519-25. [Crossref] [PubMed]
- Nath M, Pokharia S, Eng G, et al. New triorganotin (IV) derivatives of dipeptides as models for metal-protein interactions: synthesis, structural characterization and biological studies. Spectrochim Acta A Mol Biomol Spectrosc 2006;63:66-75. [Crossref] [PubMed]
- Samimi M, Jamilian M, Ebrahimi FA, et al. Oral carnitine supplementation reduces body weight and insulin resistance in women with polycystic ovary syndrome: a randomized, double-blind, placebo-controlled trial. Clin Endocrinol (Oxf) 2016;84:851-7. [Crossref] [PubMed]
- Currie E, Schulze A, Zechner R, et al. Cellular fatty acid metabolism and cancer. Cell Metab 2013;18:153-61. [Crossref] [PubMed]
- Vriens K, Christen S, Parik S, et al. Evidence for an alternative fatty acid desaturation pathway increasing cancer plasticity. Nature 2019;566:403-6. [Crossref] [PubMed]
- Li Z, Zhang H. Reprogramming of glucose, fatty acid and amino acid metabolism for cancer progression. Cell Mol Life Sci 2016;73:377-92. [Crossref] [PubMed]
- Carracedo A, Cantley LC, Pandolfi PP. Cancer metabolism: fatty acid oxidation in the limelight. Nat Rev Cancer 2013;13:227-32. [Crossref] [PubMed]
- Maan M, Peters JM, Dutta M, et al. Lipid metabolism and lipophagy in cancer. Biochem Biophys Res Commun 2018;504:582-9. [Crossref] [PubMed]
- Sun L, Liang L, Gao X, et al. Early Prediction of Developing Type 2 Diabetes by Plasma Acylcarnitines: A Population-Based Study. Diabetes Care 2016;39:1563-70. [Crossref] [PubMed]
- Pennock ND, Martinson HA, Guo Q, et al. Ibuprofen supports macrophage differentiation, T cell recruitment, and tumor suppression in a model of postpartum breast cancer. J Immunother Cancer 2018;6:98. [Crossref] [PubMed]
- Dierssen-Sotos T, Gómez-Acebo I, de Pedro M, et al. Use of non-steroidal anti-inflammatory drugs and risk of breast cancer: The Spanish Multi-Case-control (MCC) study. BMC Cancer 2016;16:660. [Crossref] [PubMed]
- Allott EH, Tse CK, Olshan AF, et al. Non-steroidal anti-inflammatory drug use, hormone receptor status, and breast cancer-specific mortality in the Carolina Breast Cancer Study. Breast Cancer Res Treat 2014;147:415-21. [Crossref] [PubMed]
- Crusz SM, Balkwill FR. Inflammation and cancer: advances and new agents. Nat Rev Clin Oncol 2015;12:584-96. [Crossref] [PubMed]
- Stabile LP, Farooqui M, Kanterewicz B, et al. Preclinical Evidence for Combined Use of Aromatase Inhibitors and NSAIDs as Preventive Agents of Tobacco-Induced Lung Cancer. J Thorac Oncol 2018;13:399-412. [Crossref] [PubMed]
- Loftus N, Miseki K, Iida J, et al. Profiling and biomarker identification in plasma from different Zucker rat strains via high mass accuracy multistage mass spectrometric analysis using liquid chromatography/mass spectrometry with a quadrupole ion trap-time of flight mass spectrometer. Rapid Commun Mass Spectrom 2008;22:2547-54. [Crossref] [PubMed]
- Miles FL, Orlich MJ, Mashchak A, et al. The Biology of Veganism: Plasma Metabolomics Analysis Reveals Distinct Profiles of Vegans and Non-Vegetarians in the Adventist Health Study-2 Cohort. Nutrients 2022;14:709. [Crossref] [PubMed]
- DeMaison L, Cohen LM, Liedtke AJ, et al. Effects of (+)-octanoylcarnitine in intact myocardium. Basic Res Cardiol 1988;83:77-86. [Crossref] [PubMed]
- Kim M, Jung S, Lee SH, et al. Association between arterial stiffness and serum L-octanoylcarnitine and lactosylceramide in overweight middle-aged subjects: 3-year follow-up study. PLoS One 2015;10:e0119519. [Crossref] [PubMed]