Identification and validation of a ferroptosis-related prognostic risk-scoring model and key genes in small cell lung cancer
Introduction
Small cell lung cancer (SCLC) is an aggressive neuroendocrine tumor with a poor prognosis, takes up 15% of total lung cancers approximately (1). The combination therapy composed of PD-L1 antibody, platinum-based chemotherapy has become the standard first-line therapy for extensive-stage small cell lung cancer (ES-SCLC), based on the results of the IMpower133 and CASPIAN trials (2,3). However, only a small of SCLC patients can benefit from immune checkpoint blockade. And no feasible genetic model associated with immune microenvironment to predict SCLC patients’ prognosis. There is an urgent need to explore the mechanisms influencing immunotherapy drugs resistance and immune infiltration in SCLC. The predictive ability of PD-L1 molecule is limited and affected by many factors, such as the spatio-temporal heterogeneity of tumors and the variety of diagnostic anti-PD-L1 antibodies. Although gene profiling studies have provided biological insight into the genomic, epi-genomic, and proteomic landscapes to indicate the prognosis and efficacy to immunotherapy in SCLC, many crucial gaps are still existing in selecting ideal biomarkers. Therefore, it is urgency to identify novel molecules associated with SCLC prognosis and immunotherapy drug resistance to guide clinical practice.
Ferroptosis, mainly characterized by ferroin and lipid peroxide accumulation on the membrane, is a novel mode of iron-dependent cell death (4,5). Recent studies have shown that ferroptosis has a key role on tumorigenesis and has become a new target for cancer therapies. Ferroptosis in cancer cells can induce an increase of immunosuppressive factors, such as PEG2, and suppress anti-tumor immunity, which may promote tumor growth (6,7). On the other hand, activation of ferroptosis inhibits tumor growth and contributes to chemotherapy resistance (8). Several small molecules have been found to be inducers of ferroptosis in tumor cells, such as erastin (5), sorafenib (9), and RAS-selective lethal (RLS3) (10). Some classical tumor-related transcription factors, such as tumor protein p53 (TP53) (11) and nuclear factor erythroid 2-related factor 2 (NRF2) (12), are also involved in the process of iron death. In lung cancer, research has found that erastin/sorafenib sensitizes tumor cells to cisplatin by inhibiting the NRF2/light chain of System xc− (xCT) pathway, which then inhibits tumor growth (13).
Most importantly, studies have reported that CD8+ T cells activated by PD-L1 inhibitors have the ability to facilitate lipid peroxidation and susceptibility to ferroptosis of tumor cells (14). The therapeutic alliance of ferroptosis and PD-L1 blockade efficiently inhibits the growth of melanoma and lung metastasis of breast cancer (15). While ferroptosis tolerance cancer cells were unresponsiveness to PD-L1 blockade, inhibition of ferroptosis resulted in resistance to immunotherapy treatment (16). However, the exact role of ferroptosis in SCLC is unknown, and it is unclear whether ferroptosis-related molecules can predict the prognosis, immune infiltration, and sensitivity to treatments of SCLC.
Here, we systematically studied RNA-sequencing data and clinical characteristics in patients with SCLC. We developed a risk-scoring model on the basis of 5-ferroptosis-related gene signatures, filtered out by univariate and multivariate Cox regression. In addition, we quested for the potential correlation between the ferroptosis-related genes, immune infiltration, and efficacy of immunotherapy in SCLC. We present the following article in accordance with the TRIPOD reporting checklist (available at https://tlcr.amegroups.com/article/view/10.21037/tlcr-22-408/rc).
Methods
Research design
First, we constructed and evaluated risk-scoring system based on 5 ferroptosis-related genes. Subsequently, we investigated the correlation of the 5 ferroptosis-related genes with the tumor immune microenvironment in SCLC, and identified a key gene TXNIP (thioredoxin-interacting protein) which most related to immune infiltration. Finally, we used Genomics of Drug Sensitivity in Cancer (GDSC) database to analyze the relationship between TXNIP and anti-tumor drug resistance, and preliminatively explore the relationship between TXNIP and immunotherapy response in SCLC patients receiving immunotherapy.
Patient data collection
RNA-sequencing data and clinical characteristics of SCLC patients were obtained from the cBioPortal (dataset: U Cologne, Nature 2015). Seventy-seven patients whose RNA-sequencing data and survival time were available in the dataset were enrolled in this study. All individuals’ clinical informations were summarized in Table 1. The relationship between gene expression and survival time was assessed.
Table 1
Variable | Number of samples |
---|---|
Gender (male/female) | 54/23 |
Age at diagnosis (≤65/>65) | 41/36 |
Stage (I-II/III-IV) | 47/30 |
T (T1/T2-4/NA) | 30/39/8 |
M (M0/M1/NA) | 57/8/12 |
N (N0/N1-3/NA) | 34/36/7 |
SCLC, small cell lung cancer.
We screened SCLC patients who were treated with first-line chemo-immunotherapy and evaluated their best clinical response. The stratification of their response to complete response (CR), partial response (PR), stable disease (SD) and progressive disease (PD), was performed by investigators according to the iRECIST (Response Evaluation Criteria in Solid Tumours for immunotherapy trials). Ten patients with good response (CR or PR) and 10 with a poor response (SD or PD) to chemo-immunotherapy were randomly selected and they were divided into the Response and Non-Response groups. The data of 20 SCLC patients treated with first-line chemo-immunotherapy was collected retrospectively from medical files.
Identification of ferroptosis-related genes in SCLC
FerrDb database provided the human ferroptosis-related genes (17). Ferroptosis-related genes in SCLC and their expression levels were obtained by integrating intersection genes from the SCLC transcriptome and human ferroptosis-related genes. Gene Ontology (GO) and Kyoto Encyclopedia of Genes and Genomes (KEGG) were used to evaluate the function of ferroptosis-related genes in SCLC.
Development of the risk-scoring system and nomogram
By univariate Cox regression analysis (P<0.05), prognostic genes were identified from these ferroptosis-related genes. And multivariate Cox regression analysis was used to establish an optimal prognostic risk-scoring model and predict the regression coefficients (α) of the model. Then, a prognostic risk-scoring system based on 5 genes was established, where risk-score = (α1 × expression of Gene1) + (α2 × expression of Gene2) + … + (αn × expression of Genen). Based on the median score of risk-scores, we divided SCLC patients into low- and high-risk groups. Participants with missing data on any predictors were excluded.
The assessment of the risk-scoring model
Kaplan-Meier (K-M) survival curves and receiver operating characteristics (ROC) curves were constructed to assess the sensitivity and specificity of the risk-scoring model. The independent prediction of the model was verified by Univariate and multivariate Cox analyses. The generality of the model was tested using stratified analysis of clinical variables. Then we constructed a nomogram prognostic map combined with clinical factors and prognostic ferroptosis-related genes to predict the 1-, 2-, and 3-year overall survival (OS) of SCLC patients.
Acquisition of SCLC immune infiltration
For investigating the role of ferroptosis-related genes in the local tumor microenvironment (TME) of SCLC, we obtained various immune cell infiltration data in SCLC patients using ImmuCellAI (http://bioinfo.life.hust.edu.cn/ImmuCellAI), which can estimate the abundance of immune cell infiltration based on RNA-seq or microarray data.
Drug resistance analysis
Cancer Cell Line Encyclopedia (CCLE) database provided gene expression profiles in SCLC cell lines (https://sites.broadinstitute.org/ccle/). And GDSC database provided 50% inhibition concentration (IC50) values of anti-cancer drugs (https://www.cancerrxgene.org/).
Immunohistochemistry (IHC) staining
Human SCLC tissue samples were collected from the patients hospitalized in the First Affiliated Hospital of Guangzhou Medical University. The expression of TXNIP and PD-L1 was determined by IHC staining. After deparaffinizing, hydrating, and being treated with 0.3% H2O2, the slides were incubated with goat serum for blocking the nonspecific binding. Next, the slides were incubated with the TXNIP antibody (1:200, Polyclonal, Boster Biological Technology, A01409-1) or PD-L1 antibody (1:250, E1L3N, Cell Signaling Technology, USA,18634T) overnight at 4 ℃, then incubating with secondary antibodies. The positive staining was visualized by staining with 3,3'-diaminobenzidine tetra-hydrochloride. After hematoxylin counter staining and hydrochloric acid alcohol differentiation, the images were captured. The study was conducted in accordance with the Declaration of Helsinki (as revised in 2013) and was approved by institutional ethics board of the First Affiliated Hospital of Guangzhou Medical University (No. 2021-K-24). Informed consent was taken from all the patients.
Statistical analysis
An independent-samples t-test was used to analyze continuous variables. Chi-square (χ2) or Fisher’s exact test was used to assess differences in categorical variables. R 4.1.0 was used for statistical analysis. P<0.05 (two-tailed) was considered statistically significant: *P<0.05, **P<0.01, ***P<0.001, and ****P<0.0001. The threshold value of the area under the curve (AUC) is 0.7.
Results
Enrichment analysis of ferroptosis-related genes in SCLC
Seventy-seven ferroptosis-related genes were selected from SCLC transcriptome data and human ferroptosis-related genes. GO (Figure 1A) and KEGG (Figure 1B) analysis of these genes were demonstrated. The GO analysis showed that the biological process (BP) was involved in the cellular response to oxidative stress, chemical stress, and the metabolism of metal ions. We found that cellular component (CC) mainly participated in the synthesis of the outer membrane of organelles and the synthesis of a variety of protease complexes, such as the protein kinase complex and the target of rapamycin complex 2 (TORC2). Furthermore, the GO analysis also showed that molecular function (MF) mainly regulated iron ion binding and antioxidant activity, specifically related to the activity of oxidoreductase, peroxidase, and hydro-lyase. The KEGG results manifested that the ferroptosis-related genes were mainly in relation to ferroptosis, PD-L1 expression and the programmed cell death receptor-1 (PD-1) checkpoint pathway in cancer, the tumor necrosis factor (TNF) signaling pathway, autophagy, the interleukin-17 (IL-17) signaling pathway, pathways of neurodegeneration, and the mammalian target of rapamycin (mTOR) signaling pathway.
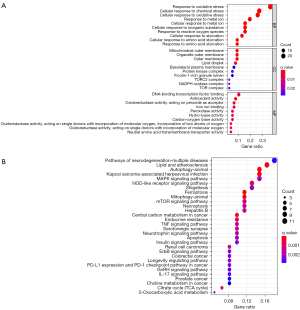
Construction of the risk-scoring evaluation system based on ferroptosis-associated genes
Univariate Cox analysis revealed that 8 ferroptosis-related genes were related to survival time (Table 2); Multivariate Cox analysis showed that 5 of these 8 ferroptosis-related genes were independent prognostic predictors of SCLC (Table 3). Therefore, risk-scores were calculated and the risk-signature based on the 5 ferroptosis-related genes were developed. Risk-score = (0.519 × expression of CISD1) + (0.350 × expression of TXNIP) + (−0.405 × expression of SLC7A5) + (−0.744 × expression of SLC2A8) + (0.555 × expression of HILPDA). We plotted the 5 identified ferroptosis-associated genes expression levels, risk-score distributions, and survival status profiles in high-risk and low-risk groups. The scatter diagrams (Figure 2A,2B) illustrated that the survival time and rate of SCLC patient exacerbated progressively as their risk-score increased. The heatmap showed the expression profiles of the 5 identified ferroptosis-associated genes (Figure 2C).
Table 2
Gene | HR | HR.95L | HR.95H | P value |
---|---|---|---|---|
CISD1 | 1.535 | 1.099 | 2.144 | 0.012 |
SESN2 | 0.623 | 0.451 | 0.860 | 0.004 |
TXNIP | 1.496 | 1.097 | 2.039 | 0.011 |
SLC7A5 | 0.597 | 0.426 | 0.838 | 0.003 |
SETD1B | 0.621 | 0.455 | 0.847 | 0.003 |
SLC2A8 | 0.545 | 0.381 | 0.779 | 0.001 |
HMGB1 | 1.331 | 1.017 | 1.742 | 0.037 |
HILPDA | 1.567 | 1.092 | 2.249 | 0.015 |
HR, hazard ratio; HR.95L, low 95% confidence interval of HR; HR.95H, high 95% confidence interval of HR.
Table 3
Gene | Coef (α) | HR | HR.95L | HR.95H | P value |
---|---|---|---|---|---|
CISD1 | 0.519 | 1.681 | 1.171 | 2.412 | 0.005 |
TXNIP | 0.350 | 1.420 | 1.025 | 1.966 | 0.035 |
SLC7A5 | –0.405 | 0.667 | 0.449 | 0.991 | 0.045 |
SLC2A8 | –0.744 | 0.475 | 0.325 | 0.695 | 0.000 |
HILPDA | 0.555 | 1.741 | 1.211 | 2.503 | 0.003 |
Coef (α) represents regression coefficient of each gene. HR, hazard ratio; HR.95L, low 95% confidence interval of HR; HR.95H, high 95% confidence interval of HR.
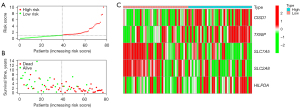
The independent predictability of the risk-scoring model
K-M survival analysis revealed that the OS in the high-risk group was shorter than that in the low-risk group (Figure 3A). The prediction efficiency of the risk-scoring model was tested by ROC curve. While the age curve (AUC =0.545), gender curve (AUC =0.593), and stage curve (AUC =0.566) were calculated, the AUC value of risk-score was 0.812, suggesting that the risk-score was better in predicting the survival time of patients (Figure 3B). In addition, the AUC values of the risk-scoring model based on 5 identified ferroptosis-associated genes were 0.812, 0.820, and 0.851 in predicting 1-, 2-, and 3-year OS, respectively (Figure 3C). The univariate and multivariate Cox analysis confirmed that the risk-score can be used as an independent predictor of SCLC patients’ prognosis (Figure 3D,3E). These findings validate the predictive power of the risk-scoring model and show that it is a promising model for predicting the prognosis of SCLC patients.
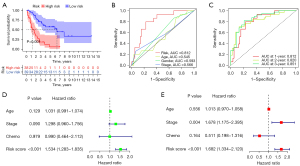
The generality of the risk-scoring model and the predictive nomogram
To verify the generality of the risk-scoring model, stratified analyses were performed in the basis of clinical variables. The risk-scoring model had prognostic significance in most subgroups (Figure 4A-4F), including age (≤65, >65), gender (female, male), T stage (T1, T2–4), N stage (N0, N1–3), clinical-stage (I–II, III–IV), and chemotherapy (chemo, no-chemo), except the OS between the M0 and M1 subgroups (Figure 4G) with the small size of the cohort. Patients in the high-risk group had a less favorable prognosis. To better combine our findings with clinical management and treatment, we present a hybrid nomogram showing the clinical characteristics and risk-score and deriving the predicted survival (Figure 5). The predictive nomogram combines risk-scores of 5 ferroptosis-associated genes and clinical characteristics, including age, tumor stage, and chemotherapy, to predict a patient’s 1-, 2-, and 3-year survival rate accurately and consistently.
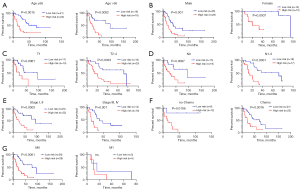
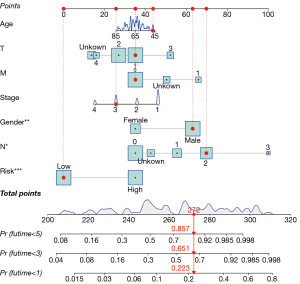
Ferroptosis-associated genes signatures were correlated with immune infiltration in SCLC
To explore the specific effect of ferroptosis-associated genes, we analyzed the immune infiltration in SCLC patients using infiltration scores from ImmuCellAI (Figure 6A). The results showed that the TXNIP gene was significantly related to a variety of immune cells infiltration (Figure 6B). TXNIP was negatively correlated with naive CD4+ T cells, naive CD8+ T cells, and NKT cells infiltration, and positively correlated with infiltration degree of cytotoxic T cells, type 1 regulatory T cells (Tr1), induced regulatory T cells (iTreg), central memory T cells, macrophages, CD4+ T cells, and infiltration score. In addition, 4 other genes were also associated with immune infiltration.
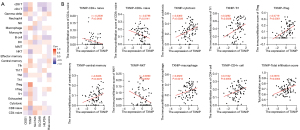
Expression of TXNIP is correlated with the prognosis and drug sensitivity of SCLC patients
Meanwhile, our study found differences in the SCLC patients’ prognosis between the TXNIP-high and TXNIP-low groups. Specifically, SCLC patients with TXNIP-high expression exhibited a better survival rate (Figure 7A,7B). To further explore the possible mechanism, we obtained the gene expression of the SCLC cell line and the anti-cancer drug IC50 from the CCLE database and the GDSC database, respectively. Then, we divided SCLC cell lines into the TXNIP-high group and the TXNIP-low group and compared the IC50 value of anti-cancer drugs. The high TXNIP group had a higher IC50 values of several anti-cancer drugs, such as Uprosertib (an AKT inhibitor) and dabrafenib (a Raf inhibitor) (Figure 7C). This indicated that SCLC patients with high TXNIP expression may be resistant to these anti-cancer drugs. And the IC50 values of AMG-319 (a phosphoinositide-3 kinase inhibitor) and Topotecan (topoisomerase I inhibitor) decreased in the high TXNIP group (Figure 7D,7E). The above results showed that patients with high TXNIP expression are relatively sensitive to AMG-319 and Topotecan treatment.
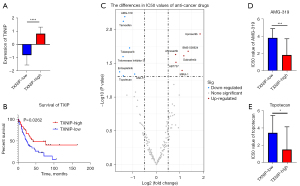
TXNIP may have the ability to predict the efficacy of immunotherapy
To further explore whether TXNIP is associated with the efficacy of immunotherapy, we collected pre-treatment baseline information and tumor specimens from 20 SCLC patients treated with first-line immunotherapy (Table 4). Then, we divided the patients into the Response and Non-Response groups (P<0.001) based on the best clinical response following first-line immunotherapy (Table 5). Analysis of the IHC score based on TXNIP and PD-L1 expression (Figure 8) showed that TXNIP scores were higher in the Response group, which indicated that patients with high TXNIP expression might respond better to immunotherapy (Figure 8A). There was no statistically significant even if the PD-L1 tended to be highly expressed in the Response group (Figure 8B), which suggested that TXNIP may have a better ability to predict the efficacy of immunotherapy than PD-L1.
Table 4
Characteristics | Response (%) (n=10) | Non-Response (%) (n=10) | P value |
---|---|---|---|
Age (y) | |||
Median [range] | 65.5 [50–72] | 65.5 [53–73] | 0.615 |
<60, n (%) | 3 (30.0) | 2 (20.0) | 1.000 |
Sex (male/female) | 10/0 | 9/1 | 1.000 |
Smoking status, n (%) | 0.474 | ||
Current/former | 10 (100.0) | 8 (80.0) | |
Never | 0 (0.0) | 2 (20.0) | |
ECOG PS, n (%) | 0.584 | ||
0 | 8 (25.0) | 5 (25.0) | |
1 | 2 (75.0) | 4 (75.0) | |
2 | 0 (0.0) | 1 (10.0) | |
Disease status, n (%) | 1.000 | ||
II/III | 3 (30.0) | 3 (30.0) | |
IV | 7 (70.0) | 7 (70.0) |
SCLC, small cell lung cancer; ECOG PS, Eastern Cooperative Oncology Group Performance Status.
Table 5
The best clinical benefit | Response (%) (n=10) | Non-Response (%) (n=10) | P value |
---|---|---|---|
CR | 1 (10.0) | 0 | <0.001 |
PR | 9 (90.0) | 0 | |
SD | 0 | 7 (70.0) | |
PD | 0 | 3 (30.0) |
SCLC, small cell lung cancer; CR, complete response; PR, partial response; SD, stable disease; PD, progressive disease.
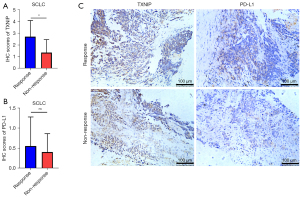
Discussion
Atezolizumab or durvalumab combined with chemotherapy represent a major step forward for ES-SCLC. However, no consistent predictive biomarkers can accurately guide the use of immune checkpoint inhibitors for SCLC patients (18). Recent evidence suggests that activation of ferroptosis can overcome tumor development and reduce the sensitivity of the tumor to chemotherapy (8). Ferroptosis has been reported to modulate the TME to inhibit tumor progression and improve prognosis (19,20). Our study first developed a novel risk-scoring model based on 5 ferroptosis-related prognostic genes from SCLC data from the cBioPortal dataset. Then, we examined the relationships between 5 ferroptosis-related prognostic genes and TME of SCLC. We have established an independent predictive model and identified potential biomarkers and therapeutic targets from the ferroptosis signaling pathway.
We built a prognostic risk-scoring model with 5 ferroptosis-related genes screened by univariate and multivariate COX analyses, including CISD1, TXNIP, HILPDA, SLC7A5 and SLC2A8. CISD1 is an iron-containing outer mitochondrial membrane protein required to regulate iron and reactive oxygen species (ROS) homeostasis in cells. CISD1 is a suppressor that prevents ferroptosis-induced cancer cell death (21). Previous studies have shown that CISD1 can inhibit lipid peroxidation by limiting mitochondrial iron uptake and suppressing ferroptosis with cysteine assistance (22). As a target gene of the protein disulfide isomerase (PDI) inhibitor, the TXNIP expression can be suppressed by a nanomolar PDI inhibitor (35G8), and 35G8-induced cell death proceeds via a mixture of autophagy and ferroptosis (23). In clear-cell carcinomas, HILPDA (hypoxia-inducible, lipid droplet-associated protein) was deemed to be a top re-sensitization factor, which could enrich polyunsaturated lipids to promotes glutathione peroxidase 4 (GPX4) inhibitor sensitivity and ferroptosis sensitivity downstream of hypoxia-inducible factor (HIF)-2α (24). It is noteworthy that HILPDA is also a target gene of HIF-1α, which has been found to re-sensitize HIF-2α-null cells to ferroptosis (24). Existing study also shows that the etoposide- and cisplatin-induced iron reduction and stemness of SCLC cells were consequent on HIF-1/ferritin axis which reduced the lysosome iron concentration (25). In our risk-assessment model, we found higher expression of HILPDA in the high-risk group, which included SCLC patients with a poorer prognosis than the low-risk group. Based on previous studies, we hypothesized that if HILPDA could also be a biomarker predicting sensitivity to GPX4-targeting agents for SCLC patients, then the patients in high-risk groups might have better prognosis following treatment with ferroptosis inducers. It may also be a potential target to benefit patients with recurrent SCLC. Moreover, solute carrier family 7 member 5 (SLC7A5) is an amino acid transporter, and SLC2A8 is a glucose transporter, both of which play a role in the biological process of ferroptosis (26,27).
Results also showed that the 5 ferroptosis-related genes correlated with immune infiltration in SCLC, suggesting that ferroptosis plays an important role in immune infiltration of SCLC. SCLC has recently been divided into neuroendocrine (NE) and non-neuroendocrine (non-NE) subtypes, showing decreased and increased immune cell infiltration, respectively (28,29). A study has found that non-NE SCLC is highly sensitive to ferroptosis induction therapy, while NE SCLC demonstrates selective addiction to the TRX redox pathway of ferroptosis (30). In our study, TXNIP was mostly positively correlated with immune cell infiltration in SCLC, indicating that ferroptosis inducers may have better efficacy in patients with high TXNIP expression.
The degree of immune invasion is related to the efficacy of chemotherapy and immunotherapy, so we investigated the role of the TXNIP gene in the therapeutic efficacy of SCLC patients. Through comparing the IC50 value of SCLC cell lines between the high and low TXNIP group, we found that TXNIP expression level was related to the sensitivity of anti-tumor drugs, including AMG-319 and Topotecan. In addition, TXNIP overexpression was observed in lung cancer patients who continued to respond to immunotherapy but was decreased in patients who did not respond to immunotherapy.
In conclusion, the risk-scoring model based on 5 ferroptosis-associated genes is a potentially powerful tool for predicting SCLC prognosis. TXNIP was found to be associated with the efficacy of immunotherapy and chemotherapy.
Acknowledgments
The authors appreciate the academic support from the AME Lung Cancer Collaborative Group.
Funding: This study was supported by the Natural Science Foundation of Guangdong Province of China (No. 2020A1515011384), the Science and Technology Program of Guangzhou, China (No. 202102010371), and the State Key Laboratory of Respiratory Disease - The open project (Nos. SKLRD-OP-202101; SKLRD-Z-202010).
Footnote
Reporting Checklist: The authors have completed the TRIPOD reporting checklist. Available at https://tlcr.amegroups.com/article/view/10.21037/tlcr-22-408/rc
Data Sharing Statement: Available at https://tlcr.amegroups.com/article/view/10.21037/tlcr-22-408/dss
Conflicts of Interest: All authors have completed the ICMJE uniform disclosure form (available at https://tlcr.amegroups.com/article/view/10.21037/tlcr-22-408/coif). LB received grants from Takeda, AstraZeneca, BMS and Roche; he also received payment for lectures and participated in advisory boards form Invitae, Eli-Lilly, AstraZeneca, Roche, MSD, Merck, BMS, Pfizer, Novartis, Takeda, Janssen; support for attending meeting from Pfizer. He is Int. Secretary-Austrian Society of Pathology; PPS Membership and Awards Committee; Member of the Mesothelioma Committee of IASLC. Mariano Provencio received consulting fees from BMS, MSD, Lilly, Takeda, Janssen; grants from BMS, Lilly, MSD and Takeda; support for attending meetings from MSD and AZ and received payment honoraria for lectures from BMS, MSD, AZ, Takeda. The other authors have no conflicts of interest to declare.
Ethical Statement: The authors are accountable for all aspects of the work in ensuring that questions related to the accuracy or integrity of any part of the work are appropriately investigated and resolved. The study was conducted in accordance with the Declaration of Helsinki (as revised in 2013). The study was approved by institutional ethics board of the First Affiliated Hospital of Guangzhou Medical University (No. 2021-K-24). Informed consent was taken from all the patients.
Open Access Statement: This is an Open Access article distributed in accordance with the Creative Commons Attribution-NonCommercial-NoDerivs 4.0 International License (CC BY-NC-ND 4.0), which permits the non-commercial replication and distribution of the article with the strict proviso that no changes or edits are made and the original work is properly cited (including links to both the formal publication through the relevant DOI and the license). See: https://creativecommons.org/licenses/by-nc-nd/4.0/.
References
- Rossi A, Tay R, Chiramel J, et al. Current and future therapeutic approaches for the treatment of small cell lung cancer. Expert Rev Anticancer Ther 2018;18:473-86. [Crossref] [PubMed]
- Horn L, Mansfield AS, Szczęsna A, et al. First-Line Atezolizumab plus Chemotherapy in Extensive-Stage Small-Cell Lung Cancer. N Engl J Med 2018;379:2220-9. [Crossref] [PubMed]
- Goldman JW, Dvorkin M, Chen Y, et al. Durvalumab, with or without tremelimumab, plus platinum-etoposide versus platinum-etoposide alone in first-line treatment of extensive-stage small-cell lung cancer (CASPIAN): updated results from a randomised, controlled, open-label, phase 3 trial. Lancet Oncol 2021;22:51-65. [Crossref] [PubMed]
- Stockwell BR, Friedmann Angeli JP, Bayir H, et al. Ferroptosis: A Regulated Cell Death Nexus Linking Metabolism, Redox Biology, and Disease. Cell 2017;171:273-85. [Crossref] [PubMed]
- Dixon SJ, Lemberg KM, Lamprecht MR, et al. Ferroptosis: an iron-dependent form of nonapoptotic cell death. Cell 2012;149:1060-72. [Crossref] [PubMed]
- Yang WS. Regulation of ferroptotic cancer cell death by GPX4. Cell 2014;156:317-31. [Crossref] [PubMed]
- Du H, Tang J, Li X, et al. Siglec-15 Is an Immune Suppressor and Potential Target for Immunotherapy in the Pre-Metastatic Lymph Node of Colorectal Cancer. Front Cell Dev Biol 2021;9:691937. [Crossref] [PubMed]
- Wang H, Lin D, Yu Q, et al. A Promising Future of Ferroptosis in Tumor Therapy. Front Cell Dev Biol 2021;9:629150. [Crossref] [PubMed]
- Sun X, Ou Z, Chen R, et al. Activation of the p62-Keap1-NRF2 pathway protects against ferroptosis in hepatocellular carcinoma cells. Hepatology 2016;63:173-84. [Crossref] [PubMed]
- Shin D, Kim EH, Lee J, et al. Nrf2 inhibition reverses resistance to GPX4 inhibitor-induced ferroptosis in head and neck cancer. Free Radic Biol Med 2018;129:454-62. [Crossref] [PubMed]
- Kang R, Kroemer G, Tang D. The tumor suppressor protein p53 and the ferroptosis network. Free Radic Biol Med 2019;133:162-8. [Crossref] [PubMed]
- Roh JL, Kim EH, Jang H, et al. Nrf2 inhibition reverses the resistance of cisplatin-resistant head and neck cancer cells to artesunate-induced ferroptosis. Redox Biol 2017;11:254-62. [Crossref] [PubMed]
- Li Y, Yan H, Xu X, et al. Erastin/sorafenib induces cisplatin-resistant non-small cell lung cancer cell ferroptosis through inhibition of the Nrf2/xCT pathway. Oncol Lett 2020;19:323-33. [PubMed]
- Wang W, Green M, Choi JE, et al. CD8+ T cells regulate tumour ferroptosis during cancer immunotherapy. Nature 2019;569:270-4. [Crossref] [PubMed]
- Song R, Li T, Ye J, et al. Acidity-Activatable Dynamic Nanoparticles Boosting Ferroptotic Cell Death for Immunotherapy of Cancer. Adv Mater 2021;33:e2101155. [Crossref] [PubMed]
- Stockwell BR, Jiang X. A Physiological Function for Ferroptosis in Tumor Suppression by the Immune System. Cell Metab 2019;30:14-5. [Crossref] [PubMed]
- Zhou N, Bao J. FerrDb: a manually curated resource for regulators and markers of ferroptosis and ferroptosis-disease associations. Database (Oxford) 2020;2020:baaa021.
- Buddharaju LNR, Ganti AK. Immunotherapy in lung cancer: the chemotherapy conundrum. Chin Clin Oncol 2020;9:59. [Crossref] [PubMed]
- Yu J, Wang Q, Zhang X, et al. Mechanisms of Neoantigen-Targeted Induction of Pyroptosis and Ferroptosis: From Basic Research to Clinical Applications. Front Oncol 2021;11:685377. [Crossref] [PubMed]
- Lu T, Zhang Z, Pan X, et al. Caveolin-1 promotes cancer progression via inhibiting ferroptosis in head and neck squamous cell carcinoma. J Oral Pathol Med 2022;51:52-62. [Crossref] [PubMed]
- Mittler R, Darash-Yahana M, Sohn YS, et al. NEET Proteins: A New Link Between Iron Metabolism, Reactive Oxygen Species, and Cancer. Antioxid Redox Signal 2019;30:1083-95. [Crossref] [PubMed]
- Homma T, Kobayashi S, Fujii J. Cysteine preservation confers resistance to glutathione-depleted cells against ferroptosis via CDGSH iron sulphur domain-containing proteins (CISDs). Free Radic Res 2020;54:397-407. [Crossref] [PubMed]
- Kyani A, Tamura S, Yang S, et al. Discovery and Mechanistic Elucidation of a Class of Protein Disulfide Isomerase Inhibitors for the Treatment of Glioblastoma. ChemMedChem 2018;13:164-77. [Crossref] [PubMed]
- Zou Y, Palte MJ, Deik AA, et al. A GPX4-dependent cancer cell state underlies the clear-cell morphology and confers sensitivity to ferroptosis. Nat Commun 2019;10:1617. [Crossref] [PubMed]
- Wang K, Chen X, Zuyi W, et al. Lysosome Fe2+ release is responsible for etoposide- and cisplatin-induced stemness of small cell lung cancer cells. Environ Toxicol 2021;36:1654-63. [Crossref] [PubMed]
- Alborzinia H, Ignashkova TI, Dejure FR, et al. Golgi stress mediates redox imbalance and ferroptosis in human cells. Commun Biol 2018;1:210. [Crossref] [PubMed]
- Alexander CM, Martin JA, Oxman E, et al. Alternative Splicing and Cleavage of GLUT8. Mol Cell Biol 2020;41:e00480-20. [Crossref] [PubMed]
- Dora D, Rivard C, Yu H, et al. Neuroendocrine subtypes of small cell lung cancer differ in terms of immune microenvironment and checkpoint molecule distribution. Mol Oncol 2020;14:1947-65. [Crossref] [PubMed]
- Rudin CM, Poirier JT, Byers LA, et al. Molecular subtypes of small cell lung cancer: a synthesis of human and mouse model data. Nat Rev Cancer 2019;19:289-97. [Crossref] [PubMed]
- Bebber CM, Thomas ES, Stroh J, et al. Ferroptosis response segregates small cell lung cancer (SCLC) neuroendocrine subtypes. Nat Commun 2021;12:2048. [Crossref] [PubMed]