FDG PET and CT radiomics in diagnosis and prognosis of non-small-cell lung cancer
Introduction
Lung cancer accounts approximately for 20% of all cancer deaths, ranking as the leading cause of cancer related-death in Europe with more than 300,000 patients every year (1). Its prognosis is usually poor with a 5-year overall survival (OS) rate inferior to 20% in stage-IV patients. Non-small-cell lung cancer (NSCLC) accounts for approximately 80% of all lung cancers, including adenocarcinoma (ADC) and squamous cell carcinoma (SCC).
18F-FDG PET-CT is widely used in clinical practice, both as a qualitative tool for tumour staging and treatment response assessment but also as a quantitative tool. The 18F-FDG uptake measurement via standardized uptake value (SUV) quantifies tumour metabolism (2) and reflects the tumor activity.
Since 2015–2016 immune check-point inhibitors (ICIs) have transformed the landscape in thoracic oncology, with a significant benefit on OS and on progression free survival (PFS) as first and second line treatments in NSCLC patients.
Intra-tumour heterogeneity (ITH) (3) is due to several factors such as somatic mutations with different clones of cancer cells. Other explanations are related to hypoxia, apoptosis, cell density and vascularity. ITH also includes diversity between primary tumour and its metastases (4,5). All these phenomena trigger different responses to treatment and select cells contingents resistant to cancer therapies. ITH is considered as a poor prognosis factor and is present at a high level in NSCLC (6). The spatial relationship between PET voxels reflects metabolic heterogeneity (7,8) and that between CT voxels measures tissue density heterogeneity (9). In several cancers, including NSCLC, radiomics was proposed as a non-invasive assessment tool to investigate tumour heterogeneity. It provides parameters called radiomics features (RFs). It is now encompassed in the fields of artificial Intelligence and data mining (10-12).
PET study (13) has shown significant association between the driver epidermal growth factor receptor (EGFR) and some RFs. The relationship between PD-L1 expression and metabolic parameters (14) and with other RFs (15) have been studied. CT extracted features were also published (16,17). Despite numerous studies focusing on the use of radiomics in NSCLC, no radiomics-based model is clinically used or validated (18,19).
Until now, the lack of clinical use could be explained by the high variability in methodology approaches and the absence of internal or external validation in most studies. No consensus has thus been proposed nowadays for routine practice (20). The main goal of this paper is to investigate the potential of radiomics to predict OS and PFS. Besides we took advantage of our patient population to undertake an ancillary study that compares RFs and PD-L1 expression on one side and RFs and histo-clinical variables on the other. We present the following article in accordance with the TRIPOD reporting checklist (available at https://tlcr.amegroups.com/article/view/10.21037/tlcr-22-158/rc).
Methods
Patients
Patients with NSCLC treated in our institution from March 2017 to October 2019 were consecutively and retrospectively included. They all had an IHC for PD-L1 expression on biopsy sample or surgical resection specimen and an 18F-FDG PET-CT at the beginning of the disease. Patients were naive of previous treatment. Upon inclusion in the study, patients were anonymized.
PET/CT image acquisition
18FDG PET-CTs have been performed on two different devices: a Siemens Biograph device without time of flight (TOF) equipment and on a Siemens Horizon device with TOF equipment. Parameters of acquisition were the following for the Biograph device: CT slice thickness: 2.5 mm, matrix size: 512, PET slice thickness: 2 mm, matrix size: 180. They were the following for the Horizon device: CT slice thickness: 1.5 mm, matrix size: 512, PET slice thickness: 2 mm, matrix size: 180. The parameters of reconstruction on the Biograph device were: OSEM 2D, 4 iterations, 8 sub-sets and 5 mm gaussian filter. Using Horizon device they were: OSEM 3 D, 6 iterations, 10 sub-sets and 6.5 mm gaussian filter. No contrast enhancement has been done for CT.
IHC
PD-L1 expression was evaluated on tissue tumour with LDTs, established on a Benchmark Ultra system using a 22C3 antibody (DAKO monoclonal mouse anti human PD-L1). The results of PD-L1 expression have been returned as the percentage of PD-L1 positive cells (PPC), based on the total of viable tumour cells in the specimen. The following scores were used: PPC =0% (PPC0); PPC =1–49% (PPC1-49) and PPC ≥50% (PPC50).
RFs calculation
Forty-seven RFs were extracted for each patient and each modality, in compliance to the IBSI guidelines (21) using the LIFEx (22) dedicated software version 4.62. The segmentation PET procedure was based on an adaptive thresholding associated to a region growing approach proposed by the software. The PET contours were refined based on the CT images. The 47 PET RFs and the 47 CT RFs (Table 1) were then extracted using these contours. The first 7 PET and CT RFs were descriptive statistical parameters derived from SUV and from Hounsfield density unit (HU) respectively. To account for the possible variety between the two scanners, an a posteriori harmonization step was applied before selection of features and modelling. Only the RFs have been harmonized using the combat procedure (23).
Table 1
Category | Features |
---|---|
Metabolism density | SUV/HU min |
SUV/HU mean | |
SUV/HU σ2 | |
SUV/HU max | |
SUV/HU peak | |
TLG/TLU | |
SUV/HU coefficient of variation | |
Histogram | Skewness |
Kurtosis | |
Log Entropy 10 | |
Log Entropy 2 | |
Energy | |
Sphericity | Volume (mL) |
Volume (voxels) | |
Sphericity | |
Compacity | |
GLCM | Homogeneity |
Energy | |
Contrast | |
Correlation | |
Dissimilarity | |
Log Entropy 10 | |
Log Entropy 2 | |
GLRLM | SRE |
LRE | |
LGRE | |
HGRE | |
SRLGE | |
SRHGE | |
LRLGE | |
LRHGE | |
GLNU | |
RLNU | |
RP | |
NGLTDM | Coarseness |
Contrast | |
GLZLM | SZE |
LZE | |
LGZE | |
HGZE | |
SZLGE | |
SZHGE | |
LGLZE | |
HGLZE | |
GLNU | |
ZNU | |
ZP |
GLCM, gray level co-occurrence matrix; GLRLM, gray level run length matrix; NGLTDM, neighborhood grey level difference matrix; GLZLM, grey level zone length matrix; SUV, standard uptake value; HU, Hounsfield unit; σ2, standard deviation; TLG, total lesion glycolysis; TLU, total lesion HU; SRE, short run emphasis; LRE, long run emphasis; LGRE, long gray level run emphasis; HGRE, high gray level run emphasis; SRLGE, short run low gray level emphasis; SRHGE, short run high gray level emphasis; LRLGE, long run low gray level emphasis; LRHGE, long run high gray level emphasis; GLNU, gray level nonuniformity; RLNU, run length non uniformity; RP, run percentage; SZE, small zone emphasis; LZE, large zone emphasis; LGZE, low gray level zone emphasis; HGZE, high gray level zone emphasis; SZLGE, small zone low gray level emphasis; SZHGE, small zone high gray level emphasis; LGLZE, low gray level zone emphasis; HGLZE, high gray level zone emphasis; ZNU, zone size nonuniformity; ZP, zone percentage.
Statistical analysis
Prognosis analysis
Prediction of OS and PFS was chosen as the primary endpoint. Correlation between RFs and PDL1/histo-clinical variables was chosen as secondary and exploratory endpoints.
Regarding the prediction of OS and PFS, four models have been designed for each endpoint: (I) a conventional (clinical and histological) model, (II) a PET model, (III) a CT model and (IV) a (conventional + PET + CT) model, resulting in 8 models. The full dataset was then separated by random sampling in a training cohort and a testing cohort.
Firstly, significance of features was tested using a univariate Cox analysis on the training cohort. Five sub-groups of variables were defined: conventional and metabolic, first order PET, second-order PET, first order CT and second-order CT. Only significant variables were included in their respective sub-group. For each model and each sub-group a multivariate stepwise Cox analysis was performed to select the final variables. These variables were introduced one by one as long as the log-likelihood ratio test remained significant at 5% level. Then a final multivariate Cox analysis was performed. Prognostic scores were generated for each model by calculating, for each patient, the weighted sum of the products of each significant variable retained in the model with its corresponding Cox coefficient referred to as the radiomics signature. Patients have then been dichotomized into a low and a high risk groups by the median value of their score. The Kaplan-Meier curves of the 4 OS models and of the 4 PFS models were drawn. The log-likelihood of each training model has been calculated and compared using the log-likelihood ratio statistic. The difference between low and high risk groups have been tested using the Logrank test. Hazard ratio (HR) and the Harrell’s concordance index (C-index) (24) were calculated. For statistical tests the significance level of 5% has been considered, except when mentioned.
The radiomics signature was applied to patients of the testing cohort to calculate their prognostic score. Low and high risk groups were generated as previously described. The survival curves were calculated as well as all the statistical parameters above described.
Association between RFs and clinical variables
Links between histology and stage on one side and with the 3 expression of the PD-L1 on the other were tested using analysis of variance (ANOVA). In addition, the RFs of age, the gender sets (male versus female), the smoker sets (non-smokers versus smokers) were compared in the same way. The null hypothesis for each test is one-sided type. The P values derived from ANOVA have been corrected with the Bonferroni method. The P value is the probability that the test statistic can take a value greater than the value of the computed Fisher test statistic. In addition, the normalized difference delta between the second and the first modality and the area under curve (AUC) have been calculated.
All calculations were performed with Matlab statistical toolbox—r2018b (The MathWorks Inc.).
Ethical statement
The study was conducted in accordance with the Declaration of Helsinki (as revised in 2013). The study was approved by ethics board of Annecy Nuclear Medicine Center which is registered with “French Commission nationale de l’informatique et des libertés (CNIL)” under number DPO-34247 in terms of patient data protection. Informed consent was taken from all the patients. Only patients who have accepted their participation in the study were included. All data have been anonymized.
Results
Patients’ population
From the 212 original patients, 4 have been excluded due to an ambiguous histology status and eight more due to a too small tumour volume (inferior to 10 mm3). The final number of patients was 200. Patient characteristics are given in Table 2. Time between IHC and PET-CT was less than 2 weeks. The PET-CTs of the first 33 patients have been recorded on the Siemens Biograph device and the last 167 patients on the Siemens Horizon device.
Table 2
Characteristics | Whole cohort | Training cohort | Testing cohort |
---|---|---|---|
Gender | |||
Male | 139 (69%) | 69 | 70 |
Female | 61 (31%) | 33 | 28 |
Age | |||
Median (years) | 67 | 66 | 68 |
Range (years) | 35–91 | 37–90 | 35–91 |
PD-L1 | |||
0% | 63 (32%) | 35 | 28 |
1–50% | 88 (42%) | 46 | 42 |
>50% | 49 (26%) | 21 | 28 |
Histology | |||
ADC | 138 (68%) | 79 | 59 |
SCC | 62 (30%) | 23 | 39 |
Stage | |||
I | 24 (12%) | 9 | 15 |
II | 19 (9%) | 10 | 9 |
III | 48 (24%) | 19 | 29 |
IV | 109 (55%) | 64 | 45 |
Smoker | |||
0† | 57 (28%) | 31 | 26 |
0–20 | 52 (22%) | 28 | 24 |
>20 | 90 (50%) | 42 | 48 |
Unknown | 1 | 1 | |
Outcome | |||
Dead | 95 (47%) | 52 | 43 |
Alive | 105 (53%) | 50 | 55 |
Progression | 134 (67%) | 68 | 66 |
No progression | 66 (33%) | 34 | 32 |
†, pack-years.
Prognosis analysis
With a median follow-up of 834 days, 67% (134/200) and 47% (95/200) of the patients have experienced progression or death respectively. The values of the variables in the two cohorts, training (102/200) and testing (98/200) have been compared using the Chi-square test and the Wilcoxon test. Only two variables were significantly different between training and testing patients: histology and RF Peak-HU-CT, indicating a valid random sampling.
Significant features derived from the final multivariate stepwise analysis are indicated in Table 3. A signature has been built with these variables associated to their regression coefficient. Details about signatures are given in supplementary section (Appendix 1).
Table 3
Variable | Training | Testing | |||||
---|---|---|---|---|---|---|---|
P Logrank | HR | C-index | P Logrank | HR | C-index | ||
OS models | |||||||
Conventional: stage + gender | 2.22×10−8 | 5.29±1.44 | 0.80±0.086 | 0.009 | 2.27±1.44 | 0.61±0.11 | |
PET: coarseness-NGTDM + GLU-GLRLM | 0.04 | 1.96±0.84 | 0.63±0.11 | ns | 1.80±1.20 | 0.56±0.12 | |
CT: GLNU-GLZLM + ZP-GLZLM | ns | 1.49±0.63 | 0.62±0.11 | ns | 1.67±1.07 | 0.61±0.11 | |
Conventional + PET + CT: stage + gender + coarseness-NGTDM-PET | ns | 1.10±0.83 | 0.55±0.11 | ns | 1.72±1.09 | 0.57±0.11 | |
PFS models | |||||||
Conventional: stage + gender | 1.9×10−5 | 2.75±1.05 | 0.70±0.11 | 0.005 | 2.45±1.1 | 0.68±0.10 | |
PET: coarseness-NGTDM + SRLGE-GLRLM-PET | 0.004 | 2.48±1.36 | 0.64±1.62 | 0.01 | 1.80±0.83 | 0.56±1.32 | |
CT: SRE-GLRLM-CT | ns | 1.83±0.70 | 0.64±1.62 | ns | 1.67±0.76 | 0.61±0.11 | |
Conventional + PET + CT: stage + coarseness-NGTDM-PET | 1.9×10−5 | 2.75±1.62 | 0.73±0.11 | 0.02 | 2.21±1.32 | 0.62±0.11 |
OS, overall survival; PFS, progression-free survival; GLRLM, gray level run length matrix; GLNU, gray level nonuniformity; GLZLM, grey level zone length matrix; SRLGE, short run low gray level emphasis; SRE, short run emphasis; ns, not significant.
The Kaplan-Meier curves of the 4 OS models and of the 4 PFS models are shown in Figure 1A,1B respectively. Statistical survival data are given in Table 3. For OS results the logrank tests were not significant in models including CT RFs. The most efficient OS model is that which only includes Stage and Gender. Table 3 shows that performances of PFS models globally surpass those of OS ones even if the likelihood ratio tests between model-1 and model-4 is significant (P=0.014) for OS and not significant for RFS. For OS training the higher median survival time is superior to 1,176 days for low risk patients of conventional model and is lower with 281 days for high risk patients of the same model. The ratio is 4.18. For PFS, these values are respectively superior to 1,176 and 285 days with a ratio of 4.12.
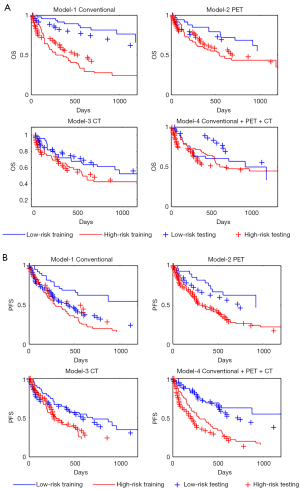
Testing cohort
The signatures from the training set were applied to patients included in the testing set. The corresponding survival curves are shown in Figure 1A,1B. The comparison between training and testing data indicated a large difference for low risk patients in OS model-4 and in PFS model-1. It must be noticed that the likelihoods could not be calculated in the testing cohort.
Association between RFs and clinical variables
Chi-square tests have been performed with contingency tables constructed from the 200 patients characteristics listed in Table 2. They were only significant for the comparison of gender status versus histology status: the proportion of SCC in male is double the proportion in female (40% vs. 20%). These findings are in accordance with current knowledge. All other comparisons were not significant.
Figure 2 sums up the links between histology status and stage status in one side and RFs in the other side. It gives for each RF, the significance level of the ANOVA test, the normalized difference delta between the second and the first modality and the corresponding AUC. These three parameters must be integrated for the clinical interpretation of the test. The differences delta of PET and CT should be interpreted independently of each other since they measure different kinds of biological and physical data.
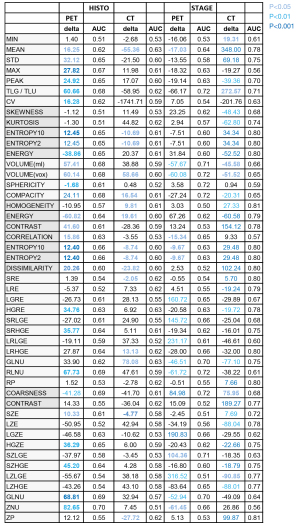
ANOVA found no significant difference of RFs between the PD-L1 expressions as well as between the smoker statuses. Then no PD-L1 and no smoker data was shown in Figure 2. Figure 3 showed a substantial overlap of the two metabolic RFs, SUVmax and TLG, and the PD-L1 expressions. In the same way, Figure 4A,4B showed an example of a high SUVmax of 43.16 on a tumour without PD-L1 expression versus a low SUVmax of 5.38 on a tumour with a high expression of PD-L1.
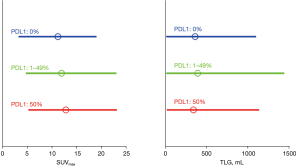
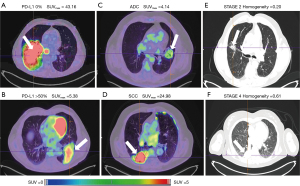
ANOVA for histology was significant at the 0.05 level for 28 PET RFs and for 15 CT RFs when ADC and SCC patients were compared. Most of metabolic RFs were significantly higher in the SCC patients than in the ADC patients as illustrated on Figure 4C,4D which showed a SCC patient with a clearly higher SUVmax than that of an ADC patient. The highest AUC concerned ZNU-GLZLM for PET and HUmean and HGRE-GLRLM for CT.
The comparison of stage 1 & 2 versus stage 3 & 4 is significant for 17 PET RFs and 33 CT RFs. Homogeneity-CT is higher in stage 3 & 4 patients than in the stage 1 & 2 ones (P<0.01 and AUC =0.81). As an example on Figure 4E,4F, the value of homogeneity-CT of the stage 4 para-pleural tumour is higher than that of the stage 1 & 2 tumour, with 0.61 and 0.20 values respectively.
Discussion
The reduced number of final significant RFs in multivariate survival analyses can be explained by a high redundancy of prognostic information between the included RFs. Better performances of PFS models could be explained by a higher number of patients with PFS events than the number of patients with OS events (Table 2). Models including CT RFs are less than optimal while those with PET RFs remain efficient. According to Logrank test, HR and C-index, conventional models only including Stage and Gender remain the most efficient to separate low risk and high risk patients.
A study (25) based only on a conventional model, without radiomics data, has shown good performance for PFS evaluation with a C-index of 0.75. A study (26) based on PET showed that a standardized, multi-centre dataset to predict PFS in locally advanced NSCLC was successful whereas prediction models with robust feature preselection were unsuccessful. In the same way a meta-analysis (20) found a poor prognostic value of radiomics. On the contrary, a study (27) has shown an improvement of PFS estimation by adding PET RFs to conventional model. Likewise, a study (28) found a good PFS predictive value for PET and CT RFs. It is similar for older studies (29,30). Finally, two studies (31,32) have concluded to an improvement of prognostic with radiomics compared to conventional data on special patients. Moreover, the high prognosis value of stage in our study, at least equal to that of some RFs, must be emphasized. We found some gaps, more or less pronounced, between training and testing results. This reflects a degree of instability between cohorts. In summary our results confirm the survival prediction potential of some RFs, alone or in association. Despite this, the addition of radiomics data to conventional data does not improve the prediction of survival in our patients.
Several conventional variables and a lot of RFs are significant to predict the prognosis in a univariate way in our cohort. Stage was the most significant variable in the models including conventional data. It can be noticed that the number of RFs CT is superior to that of PET ones. The number of significant variables according to univariate tests is clearly reduced in multivariate Cox model. The likelihood ratio test is only significant for the comparison between the conventional OS models and the (conventional + PET & CT) models and not significant for PFS models. That indicates an absence of additional prognosis information of radiomics compared to the conventional variables.
A limit of our prognostic study is the relative low ratios of events per variable included in the stepwise multivariable procedure, inferior to the advised value of 10. The retrospective nature of this study is also a disadvantage. Moreover, we have not considered the prediction of immunotherapy effects using radiomics as it was done in several papers. One of them (33) associated a biological inflammatory factor based on the ratio of neutrophils to leukocytes to metabolic PET RFs. It improves the selection of appropriate patients for immunotherapy. Response to immunotherapy can also be provided using a radiomics signature of CD8 cells (34). These applications in NSCLC are promising (35) but present some limitations (19) and are not yet widespread.
About association between RFs and clinical variables, our main findings are the followings. There was no association between PD-L1 expression and conventional and radiomics parameters, in discordance with several publications which indicate a higher FDG metabolism in patients with PD-L1 expression superior to 50% (14,15). However, the links between PD-L1 expression and tumour metabolism should be interpreted according to immune environment as proposed for prediction of immunotherapy response (33,34). We have observed a higher glycolysis metabolism in SCC tumours compared to that of ADC ones. Stage 3 & 4 tumours appear more homogeneous than stage 1 & 2 ones. Conventional variables are independent from each other apart from the impact of gender on the histology sub-type with a rate of SSC twice as high in men than in women.
Studies (16,17) have shown significant relations between PD-L1 expression and CT RFs while we did not find any. A correlation between the PD-L1 level of expression and medical imaging is of importance especially given the current development of in vivo imaging procedures with 89Zr labelled anti-CD8 minibody (36) and specific labelled 18F molecule such as adnectin (37) have been proposed. These immuno-imaging techniques, not yet used in clinical practice, are promising and could complete radiomics.
Regarding the correlation between RFs and the rest of clinico-histopathologic among SCC and especially ADC patients’ variables we confirmed the importance of the glycolysis level as a prognostic tool among SCC and especially ADC patients already described (38). Glycolysis was able to stratify ADC patients regarding their prognosis, a lower glucose metabolism being associated with a better prognosis. Results regarding the correlation between RFs and the tumor stage are relatively surprising since they would indicate that stage 3 & 4 tumors, including metastatic lesions are significantly more homogeneous on CT (but not in PET) than the stage 1 & 2 tumors. These results should be considered as exploratory and confirmed on external cohorts.
In summary, several conventional variables and a lot of RFs are significant to predict the prognosis in a univariate way in our cohort. Stage was the most significant variable in the models including conventional data. It can be noticed that the number of RFs CT is superior to that of PET ones. The number of significant variables according to univariate tests is clearly reduced in multivariate Cox model. The likelihood ratio test is only significant for OS models to compare the conventional models and the (conventional + PET & CT) models. That indicates a real but weak contribution to radiomics compared to the conventional variables. Moreover, the PD-L1 expression of tumours could not be differentiated by RFs.
In practice, for our population and in our institutions, we found a limited practical interest of radiomics in NSCLC patients. RFs provide prognostic information but don’t appear as superior to conventional data. Our data support the need of large scale, prospective trial to fully apprehend the complexity of patients’ responses to ICIs. Nevertheless we have respected the methodology described in the literature especially by using a well-established and widespread procedure (Lifex) for segmentation and to estimate the RFs as well as a suitable algorithm to harmonize them (Combat). As previously discussed, convolutional filters were not applied due to the lack of IBSI-recommendations regarding the use of LoG or wavelet features in PET and/or CT imagings. However, integration of such features could possibly enhance the models’ performances and should be further evaluated.
We have also compared the survival models using the log-likelihood ratio statistics and have performed a training/testing procedure. Whatever the practical impact of presenting negative results must be considered (39). The differences of findings between several institutions could be explained by specificities of populations: ethnics and social status of patients as well as kind and level of medical care, this confusion bias being rarely taken into account in available data. Technical development of PET-CT devices must also be taken into account as well as specificities of the radiomics software and the way to handle it. For information, LifeX (22) team has a project to develop an application that will enables the evaluation, in a multicenter way, of radiomics and\or AI models proposed for the management of lung cancer patients. Multicenter validation of models remains indeed essential to consider for their clinical use. Several studies focused on the lack of harmonization of radiomics (38,39) while others (10-12,40,41) underscore more sophisticated methodology such as principal components analysis (PCA), artificial intelligence (AI), multiblock discriminant analysis (42). However these techniques can be explored in research centers but are not suited for a clinical use which needs simple and robust procedures. Nowadays, one should be cautious in applying results obtained by others and the creation of one’s own survival analysis seems necessary, before calculating the optimal local suited radiomics signature.
Acknowledgments
Thanks to the physicians of the Annecy Center of Nuclear Medicine for acquisition of the PET-CT data and to the physicians of the Pneumology Department of the CHANGE Annecy Hospital for the clinical management of the patients.
Funding: None.
Footnote
Reporting Checklist: The authors have completed the TRIPOD reporting checklist. Available at https://tlcr.amegroups.com/article/view/10.21037/tlcr-22-158/rc
Data Sharing Statement: Available at https://tlcr.amegroups.com/article/view/10.21037/tlcr-22-158/dss
Conflicts of Interest: All authors have completed the ICMJE uniform disclosure form (available at https://tlcr.amegroups.com/article/view/10.21037/tlcr-22-158/coif). The authors have no conflicts of interest to declare.
Ethical Statement: The authors are accountable for all aspects of the work in ensuring that questions related to the accuracy or integrity of any part of the work are appropriately investigated and resolved. The study was approved by ethics board of Annecy Nuclear Medicine Center which is registered with “French Commission nationale de l’informatique et des libertés (CNIL)” under number DPO-34247 in terms of patient data protection. Informed consent was taken from all the patients. Only patients who have accepted their participation in the study were included. All data have been anonymized.
Open Access Statement: This is an Open Access article distributed in accordance with the Creative Commons Attribution-NonCommercial-NoDerivs 4.0 International License (CC BY-NC-ND 4.0), which permits the non-commercial replication and distribution of the article with the strict proviso that no changes or edits are made and the original work is properly cited (including links to both the formal publication through the relevant DOI and the license). See: https://creativecommons.org/licenses/by-nc-nd/4.0/.
References
- Barta JA, Powell CA, Wisnivesky JP. Global Epidemiology of Lung Cancer. Ann Glob Health 2019;85:8. [Crossref] [PubMed]
- Greenspan BS. Role of PET/CT for precision medicine in lung cancer: perspective of the Society of Nuclear Medicine and Molecular Imaging. Transl Lung Cancer Res 2017;6:617-20. [Crossref] [PubMed]
- Marusyk A, Polyak K. Tumor heterogeneity: causes and consequences. Biochim Biophys Acta 2010;1805:105-17. [PubMed]
- McGranahan N, Swanton C. Clonal Heterogeneity and Tumor Evolution: Past, Present, and the Future. Cell 2017;168:613-28. [Crossref] [PubMed]
- Zito Marino F, Bianco R, Accardo M, et al. Molecular heterogeneity in lung cancer: from mechanisms of origin to clinical implications. Int J Med Sci 2019;16:981-9. [Crossref] [PubMed]
- de Sousa VML, Carvalho L. Heterogeneity in Lung Cancer. Pathobiology 2018;85:96-107. [Crossref] [PubMed]
- Hensley CT, Faubert B, Yuan Q, et al. Metabolic Heterogeneity in Human Lung Tumors. Cell 2016;164:681-94. [Crossref] [PubMed]
- Orlhac F, Soussan M, Maisonobe JA, et al. Tumor texture analysis in 18F-FDG PET: Relationships between texture parameters, histogram indices, standardized uptake values, metabolic volumes and total lesion glycolysis. J Nucl Med 2014;55:414-22. [Crossref] [PubMed]
- Ganeshan B, Goh V, Mandeville HC, et al. Non-small cell lung cancer: Histopathological correlates for texture parameters at CT. Radiology 2013;266:326-36. [Crossref] [PubMed]
- Hatt M, Le Rest CC, Tixier F, et al. Radiomics: Data Are Also Images. J Nucl Med 2019;60:38S-44S. [Crossref] [PubMed]
- Sollini M, Antunovic L, Chiti A, et al. Towards clinical application of image mining: a systematic review on artificial intelligence and radiomics. Eur J Nucl Med Mol Imaging 2019;46:2656-72. [Crossref] [PubMed]
- Wolsztynski E, O'Sullivan J, Hughes NM, et al. Combining structural and textural assessments of volumetric FDG-PET uptake in NSCLC. IEEE Trans Radiat Plasma Med Sci 2019;3:421-33. [Crossref] [PubMed]
- Yip SS, Kim J, Coroller TP, et al. Associations Between Somatic Mutations and Metabolic Imaging Phenotypes in Non-Small Cell Lung Cancer. J Nucl Med 2017;58:569-76. [Crossref] [PubMed]
- Takada K, Toyokawa G, Okamonto T, et al. Metabolic characteristics of programmed cell death-ligand-1-expressing lung cancer on 18F-fluorodoxyglucose positron emission tomography/computed tomography. Cancer Med 2017;6:2552-61. [Crossref] [PubMed]
- Jiang M, Sun D, Guo Y, et al. Assessing PD-L1 expression level by radionomic features from PET/CT in nonsmall cell lung cancer patients: an initial result. Acad Radiol 2020;27:171-9. [Crossref] [PubMed]
- Yoon J, Suh YJ, Han K, et al. Utility of CT radiomics for prediction of PD-L1 expression in advanced lung adenocarcinomas. Thorac Cancer 2020;11:993-1004. [Crossref] [PubMed]
- Miles KA. How to use CT texture analysis for prognostication of non-small cell lung cancer. Cancer Imaging 2016;16:10. [Crossref] [PubMed]
- Pinto Dos Santos D, Dietzel M, Baessler B. A decade of radiomics research: are images really data or just patterns in the noise? Eur Radiol 2021;31:1-4. [Crossref] [PubMed]
- Chetan MR, Gleeson FV. Radiomics in predicting treatment response in non-small-cell lung cancer: current status, challenges and future perspectives. Eur Radiol 2021;31:1049-58. [Crossref] [PubMed]
- Rizzo S, Botta F, Raimondi S, et al. Radiomics: the facts and the challenges of image analysis. Eur Radiol Exp 2018;2:36. [Crossref] [PubMed]
- Zwanenburg A, Vallières M, Abdalah MA, et al. The Image Biomarker Standardization Initiative: Standardized Quantitative Radiomics for High-Throughput Image-based Phenotyping. Radiology 2020;295:328-38. [Crossref] [PubMed]
- Nioche C, Orlhac F, Boughdad S, et al. LIFEx: A Freeware for Radiomic Feature Calculation in Multimodality Imaging to Accelerate Advances in the Characterization of Tumor Heterogeneity. Cancer Res 2018;78:4786-9. [Crossref] [PubMed]
- Orlhac F, Boughdad S, Philippe C, et al. A post-reconstruction harmonization method for multicenter radiomic studies in PET. J Nucl Med 2018;59:1321-8. [Crossref] [PubMed]
- Harrell FE Jr, Califf RM, Pryor DB, et al. Evaluating the yield of medical tests. JAMA 1982;247:2543-6. [Crossref] [PubMed]
- Alexander M, Wolfe R, Ball D, et al. Lung cancer prognostic index: a risk score to predict overall survival after the diagnosis of non-small-cell lung cancer. Br J Cancer 2017;117:744-51. [Crossref] [PubMed]
- Oliveira C, Amstutz F, Vuong D, et al. Preselection of robust radiomics features does not improve outcome modelling in non-small cell lung cancer based on clinical routine FDG-PET imaging. EJNMMI Res 2021;11:79. [Crossref] [PubMed]
- Ahn HK, Lee H, Kim SG, et al. Pre-treatment 18F-FDG PET-based radiomics predict survival in resected non-small cell lung cancer. Clin Radiol 2019;74:467-73. [Crossref] [PubMed]
- Kirienko M, Cozzi L, Antunovic L, et al. Prediction of disease-free survival by the PET/CT radiomic signature in non-small cell lung cancer patients undergoing surgery. Eur J Nucl Med Mol Imaging 2018;45:207-17. [Crossref] [PubMed]
- Huang Y, Liu Z, He L, et al. Radiomics Signature: A Potential Biomarker for the Prediction of Disease-Free Survival in Early-Stage (I or II) Non-Small Cell Lung Cancer. Radiology 2016;281:947-57. [Crossref] [PubMed]
- Fried DV, Mawlawi O, Zhang L, et al. Stage III Non-Small Cell Lung Cancer: Prognostic Value of FDG PET Quantitative Imaging Features Combined with Clinical Prognostic Factors. Radiology 2016;278:214-22. [Crossref] [PubMed]
- Dissaux G, Visvikis D, Da-Ano R, et al. Pretreatment 18F-FDG PET/CT Radiomics Predict Local Recurrence in Patients Treated with Stereotactic Body Radiotherapy for Early-Stage Non-Small Cell Lung Cancer: A Multicentric Study. J Nucl Med 2020;61:814-20. [Crossref] [PubMed]
- Luna JM, Barsky AR, Shinohara RT, et al. Radiomic Phenotypes for Improving Early Prediction of Survival in Stage III Non-Small Cell Lung Cancer Adenocarcinoma after Chemoradiation. Cancers (Basel) 2022;14:700. [Crossref] [PubMed]
- Seban RD, Mezquita L, Berenbaum A, et al. Baseline metabolic tumor burden on FDG PET/CT scans predicts outcome in advanced NSCLC patients treated with immune checkpoint inhibitors. Eur J Nucl Med Mol Imaging 2020;47:1147-57. [Crossref] [PubMed]
- Sun R, Limkin EJ, Vakalopoulou M, et al. A radiomics approach to assess tumour-infiltrating CD8 cells and response to anti-PD-1 or anti-PD-L1 immunotherapy: an imaging biomarker, retrospective multicohort study. Lancet Oncol 2018;19:1180-91. [Crossref] [PubMed]
- Aide N, Hicks RJ, Le Tourneau C, et al. FDG PET/CT for assessing tumour response to immunotherapy. Report on the EANM symposium on immune modulation and recent review of the literature. Eur J Nucl Med Mol Imaging 2019;46:238-50. [Crossref] [PubMed]
- Pandit-Taskar N, Postow MA, Hellmann MD, et al. First-in-Humans Imaging with 89Zr-Df-IAB22M2C Anti-CD8 Minibody in Patients with Solid Malignancies: Preliminary Pharmacokinetics, Biodistribution, and Lesion Targeting. J Nucl Med 2020;61:512-9. [Crossref] [PubMed]
- Donnelly DJ, Smith RA, Morin P, et al. Synthesis and Biologic Evaluation of a Novel 18F-Labeled Adnectin as a PET Radioligand for Imaging PD-L1 Expression. J Nucl Med 2018;59:529-35. [Crossref] [PubMed]
- Schuurbiers OC, Meijer TW, Kaanders JH, et al. Glucose metabolism in NSCLC is histology-specific and diverges the prognostic potential of 18FDG-PET for adenocarcinoma and squamous cell carcinoma. J Thorac Oncol 2014;9:1485-93. [Crossref] [PubMed]
- Buvat I, Orlhac F. The Dark Side of Radiomics: On the Paramount Importance of Publishing Negative Results. J Nucl Med 2019;60:1543-4. [Crossref] [PubMed]
- Hatt M, Cheze Le Rest C, Antonorsi N, et al. Radiomics in PET/CT: Current Status and Future AI-Based Evolutions. Semin Nucl Med 2021;51:126-33. [Crossref] [PubMed]
- Yip SS, Aerts HJ. Applications and limitations of radiomics. Phys Med Biol 2016;61:R150-66. [Crossref] [PubMed]
- Lee SH, Kao GD, Feigenberg SJ, et al. Multiblock Discriminant Analysis of Integrative 18F-FDG-PET/CT Radiomics for Predicting Circulating Tumor Cells in Early-Stage Non-small Cell Lung Cancer Treated With Stereotactic Body Radiation Therapy. Int J Radiat Oncol Biol Phys 2021;110:1451-65. [Crossref] [PubMed]