Development and validation of a polygenic hazard score to predict prognosis and adjuvant chemotherapy benefit in early-stage non-small cell lung cancer
Introduction
Lung cancer is the leading cause of cancer-related deaths in China and around the world (1), and non-small cell lung cancer (NSCLC) accounts for about 85% of lung cancer (2). Surgical resection is considered the preferred treatment for stage I-II NSCLC patients but tumor recurrence and metastasis remain the main cause of treatment failure. Currently, adjuvant chemotherapy (ACT) has not been routinely recommended for stage I patients especially in stage IB, since its benefit remains undetermined (3). The National Comprehensive Cancer Network (NCCN) guidelines recommend that ACT is an appropriate option for stage IB patients with high-risk factors such as a poorly differentiated tumor, vascular invasion, wedge resection, tumor size greater than 4 cm, and pleural vascular invasion (4). However, the American Society of Clinical Oncology (ASCO) guidelines do not recommend ACT for stage IB patients (5). Moreover, although ACT is recommended universally without any risk stratification for stage II patients, the overall benefit of ACT is limited (6,7) and not all patients derive survival benefit from it (8-10). Therefore, the current tumor-node-metastasis (TNM) stage has limitations in predicting treatment response and guiding ACT application, and there is an urgent need to develop additional predictors to predict who would benefit from ACT.
Previous studies have found that genetic variation was associated with lung cancer predisposition, treatment response, and disease progression (11-13). Genetic variations involved in therapeutic drugs pharmacokinetics and pharmacodynamics, which may partially account for inter-individual differences in chemotherapy benefits (14). It is well known that the DNA repair pathway is an important signaling pathway in chemotherapy response (15-17). P53 and PI3K/PTEN/AKT pathways have also been reported to be associated with treatment response, survival, and toxicity in NSCLC patients treated with chemotherapy (18,19). In addition to candidate gene strategies, genome-wide association studies (GWAS) had also identified several single-nucleotide polymorphisms (SNPs) associated with the survival of NSCLC patients treated with chemotherapy (20-22). These researches indicated that the genetic variations may play an important role in the prognosis and suggested individual genetics as a potential biomarker for individualized therapeutic decision-making. However, the difficulty in applying genetic data to the clinical utility is that the effect of a single variant is relatively small, and is not informative enough for predicting prognosis. The polygenic risk score, combining the genotype dosage of multiple SNPs by their respective weight, has emerged as the main approach for predicting the genetic component of a specific outcome (23-27), and recent studies have developed GWAS-derived polygenic scores using SNPs to predict clinical survivals and treatment response (28-30). To the best of our knowledge, the association between polygenic score and prognosis of early-stage NSCLC patients has not been studied and their applications remain to be explored.
In this study, we developed and validated a polygenic hazard score (PHS) to estimate the clinical outcomes for patients with early-stage NSCLC. Further, we investigated whether the PHS could identify patients who would benefit most from ACT. We present the following article in accordance with the TRIPOD reporting Checklist (available at https://tlcr.amegroups.com/article/view/10.21037/tlcr-22-139/rc).
Methods
Patients and study design
We conducted a retrospective study of patients with stage I–II NSCLC. Participants were recruited from two populations described before: the Guangzhou GSA GWAS (11) and the Prostate, Lung, Colorectal, and Ovarian Cancer Screening (PLCO) trial (31). The patients in Guangzhou GSA GWAS were collected from Sun Yat-sen University Cancer Center (Guangzhou, China) between January 2006 and December 2017. The PLCO Trial was a randomized control study that included ~155,000 volunteers aged 55–74 at enrollment between 1993 and 2011 from 10 medical centers in the United States.
The flow chart of the patients is represented in Figure 1. Eligible patients were aged 18 years or older with histologically confirmed stage I or II NSCLC, radical surgery, and complete clinical and follow-up data. The exclusion criteria included previous or concurrent malignant disease and neoadjuvant treatments for NSCLC. Ultimately, 404 patients who treated with surgery plus ACT (SYSUCC ACT set), with 388/404 (96%) of these patients receiving platinum-based ACT, and 624 patients who treated with surgery alone (SYSUCC surgery set) were included in this study from Guangzhou GSA GWAS. The SYSUCC ACT set was randomly divided into a training set (n=202) and a test set (n=202). The training set was used to construct the PHS, and the test set and SYSUCC surgery set were used as validation cohort (SYSUCC validation cohort, n=826). Eligible patients from PLCO Trail were used as an external validation cohort (PLCO validation cohort, n=367). The study was conducted in accordance with the Declaration of Helsinki (as revised in 2013). The Institutional Review Board of Sun Yat-sen University Cancer Center approved this study (Approval No. B2022-131-01). Because of the retrospective nature of the study, the requirement for informed consent was waived.
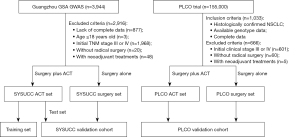
Clinical and follow-up information
For participants from the Guangzhou GSA GWAS, patients’ clinical information, including sex, age at diagnosis, smoking status, family history of cancer, histologic grade, EGFR mutation status, TNM stage, and treatments were reviewed from the medical records. These patients were followed up by outpatient visits or telephone contacts as the clinicians recommended (last follow-up in September 2020). The survival status of the patients was obtained from the follow-up department of the hospital and the death registration at the public security bureau. The PLCO Trial gathered clinical information from the first screening visit, such as sex, age, family history, and smoking history. The patients were followed up for more than 13 years after clinical enrollment (32). The endpoint of the study was overall survival (OS), which was defined as the date of surgery to the date of death or the last day of follow-up.
Genotyping, quality control, and imputation
For patients from the Guangzhou GSA GWAS, the methods of DNA extraction, genotyping, and imputation process have been detailed described (11). Briefly, DNA was extracted from peripheral blood and SNP genotyping was detected by Illumina Infinium® Global Screening Array (GSA) v1.0. Quality control at sample level and SNP level have been done according to the criteria described in the previous study (11). Qualified genotypes were imputed by a two-stage imputation approach, using SHAPEIT2 (33) for phasing and IMPUTE2 for imputation (34) and the Phase III 1000 Genomes Project was set as the reference. For patients from PLCO Trial, genomic DNA extracted from the blood samples was genotyped with Illumina HumanHap240Sv1.0, HumanHap300v1.1, HumanHap550v3.0 and Human610-Quadv1_B (dbGaP accession: phs000093.v2.p2 and phs000336.v1.p1) (35,36). We conducted similar quality control and imputation methods as we did in the Guangzhou GSA GWAS. In brief, we excluded samples with genotype completion rates <95%, gender discrepancy, familial relationships, extreme heterozygosity rates (>6 SD), or population outliers defined by principal component analysis (PCA) via EIGENSTRAT. Then, we excluded SNPs with call rates <95%, minor allele frequencies (MAFs) <0.01, or P<10-12 in testing of Hardy-Weinberg equilibrium (HWE). We performed quality control filtering by using PLINK. Qualified genotypes were imputed by a two-stage imputation approach, using SHAPEIT2 for phasing and IMPUTE2 for imputation and using the Phase III 1000 Genomes Project (Europeans) as the reference.
PHS construction
Analysis was restricted to the 2,943,474 SNPs shared between Guangzhou GSA GWAS and PLCO datasets. The PHS was created in three steps. Firstly, we conducted a GWAS to identify variants associated with OS in the SYSUCC ACT set. The GWAS was performed using the multivariate cox regression model with adjustment for sex (male or female), age (≤59 or >59 years, median age as the cutoff), smoking status (never smoking, quitting smoking, or current smoking), histology (lung adenocarcinoma, lung squamous cell carcinoma or other types), grade (G1 or G2 or G3), TNM stage (stage I or II), and the top three principal components. We performed LD pruning (r2>0.3) to keep the independent SNPs and selected candidate SNPs with P<1.0×10−3 from the GWAS results. Secondly, to avoid overfitting, we used the least absolute shrinkage and selection operator (LASSO) model to select the most important variants in the training set and the 1 standard error of the minimum criteria (the 1-SE) were used as criteria. This analysis was performed ten times using ten different random number seeds and only SNPs selected by all ten models were retained to calculate the PHS. Thirdly, the PHS was calculated by LASSO Cox model using the sum of values weighted by the coefficients of the most discriminative SNPs. We calculated PHS for each participant included in this study and the median PHS in the training set was used as the cut-off value to split patients into low PHS or high PHS groups.
Statistical analysis
The GWAS was performed with the “gwasurvivr” package of R software (37). The LASSO method was used to construct the PHS using the “glmnet” package (38). Survival analysis was conducted using the R package “survival” and “survminer”. Log-rank test was used to compare the survival time and survival curves were estimated using the Kaplan–Meier method. The association of PHS and other clinical factors with OS was assessed using univariate and multivariable Cox regression models. Hazard ratios (HR) and corresponding 95% confidence interval (CI) were evaluated according to Cox proportional hazard models. In propensity score matching analyses (39), patients in the surgery alone group were matched to the ACT plus surgery group with a 1:1 matching based on the sex, age, smoking status, histology, grade, and TNM stage. All statistical tests were two-sided and considered significant when the P was less than 0.05. All analyses were performed in R software, version 3.6.3.
Results
Patient clinical characteristics
One thousand and three hundred ninty-five eligible NSCLC patients were included in this study, including 202 patients in the training set, 826 patients in SYSUCC validation cohort, and 367 patients in PLCO validation cohort. The median follow-up time was 96.8 months [interquartile range (IQR), 78.5–113.1 months] for the training set, 96.4 months (IQR, 81.5–113.4 months) for SYSUCC validation cohort, and 171.0 months (IQR, 128.5–207.5 months) for PLCO validation cohort. For the above three populations, five-year survival (5-year OS) rates were 71.1%, 74.5%, and 74.1%, respectively. The baseline characteristics of the participants included in this study were summarized in Table 1. Most of the clinical characteristics were significantly different (P<0.05) between the study sets, which may partly be due to the different study designs of the original study cohorts (Table 1).
Table 1
Characteristics | All patients, n=1,395 | Training set (n=202) | SYSUCC validation cohort (n=826) | PLCO validation cohort (n=367) | P |
---|---|---|---|---|---|
Sex | 0.083 | ||||
Male | 809 (58.0%) | 123 (60.9%) | 491 59.4%) | 195 (53.1%) | |
Female | 586 (42.0%) | 79 (39.1%) | 335 (40.6%) | 172 (46.9%) | |
Age (year) | <0.001* | ||||
≤59 | 503 (36.1%) | 112 (55.4%) | 364 (44.1%) | 27 (7.4%) | |
>59 | 892 (63.9%) | 90 (44.6%) | 462 (55.9%) | 340 (92.6%) | |
Family cancer history | <0.001* | ||||
No | 954 (68.4%) | 160 (79.2%) | 650 (78.7%) | 144 (39.3%) | |
Yes | 440 (31.6%) | 42 (20.8%) | 176 (21.3%) | 222 (60.7%) | |
Smoking | <0.001* | ||||
Never | 571 (40.9%) | 102 (50.5%) | 428 (51.8%) | 41 (11.2%) | |
Ever | 301 (21.6%) | 26 (12.9%) | 145 (17.6%) | 130 (35.4%) | |
Current | 523 (37.5%) | 74 (36.6%) | 253 (30.6%) | 196 (53.4%) | |
Histology | <0.001* | ||||
AD | 891 (63.9%) | 138 (68.3%) | 546 (66.1%) | 207 (56.4%) | |
SCC | 378 (27.1%) | 41 (20.3%) | 228 (27.6%) | 109 (29.7%) | |
Other† | 126 (9.0%) | 23 (11.4%) | 52 (6.3%) | 51 (13.9%) | |
EGFR | <0.001* | ||||
Wild type | 397 (28.5%) | 82 (40.6%) | 315 (38.1%) | 0 (0.00%) | |
Mutation | 262 (18.8%) | 57 (28.2%) | 205 (24.8%) | 0 (0.00%) | |
Unknown | 736 (52.8%) | 63 (31.2%) | 306 (37.0%) | 367 (100%) | |
Grade | <0.001* | ||||
G1 | 634 (45.4%) | 111 (55.0%) | 380 (46.0%) | 143 (39.0%) | |
G2 | 524 (37.6%) | 81 (40.1%) | 312 (37.8%) | 131 (35.7%) | |
G3 | 187 (13.4%) | 10 (5.0%) | 114 (13.8%) | 63 (17.2%) | |
Unknown | 50 (3.6%) | 0 (0.0%) | 20 (2.4%) | 30 (8.17%) | |
TNM stage | <0.001* | ||||
I | 980 (70.3%) | 90 (44.6%) | 582 (70.5%) | 308 (83.9%) | |
II | 415 (29.7%) | 112 (55.4%) | 244 (29.5%) | 59 (16.1%) | |
ACT | <0.001* | ||||
Without | 935 (67.0%) | 0 (0.0%) | 624 (75.5%) | 311 (84.7%) | |
With | 460 (33.0%) | 202 (100%) | 202 (24.5%) | 56 (15.3%) | |
Radiotherapy | <0.001* | ||||
Without | 1,350 (96.8%) | 189 (93.6%) | 815 (98.7%) | 346 (94.3%) | |
With | 45 (3.23%) | 13 (6.44%) | 11 (1.33%) | 21 (5.72%) | |
Survival status | <0.001* | ||||
Alive | 784 (56.2%) | 115 (56.9%) | 527 (63.8%) | 142 (38.7%) | |
Dead | 611 (43.8%) | 87 (43.1%) | 299 (36.2%) | 225 (61.3%) |
†, other subtypes include large cell, adenosquamous, sarcomatoid, basaloid, and unclassifiable NSCLC. *, P<0.05. AD, lung adenocarcinoma; SCC, lung squamous cell carcinoma; EGFR, epidermal growth factor receptor; ACT, adjuvant chemotherapy.
We investigated which clinical factors were associated with OS using a Cox regression model. In univariate and multivariate analyses (Table S1), the independent prognostic factors were sex (adjusted HR =0.64, 95% CI: 0.51–0.79, P<0.001), age (adjusted HR =1.68, 95% CI: 1.35–2.08, P<0.001), tumor grade (adjusted HR =0.90, 95% CI: 0.74–1.10, P=0.299 for grade G2; adjusted HR =0.54, 95% CI: 0.40–0.74, P<0.001 for grade G3), and TNM stage (adjusted HR =1.76, 95% CI: 1.43–2.19, P<0.001), which were adjusted as covariates in following analyses.
PHS construction and validation
To build a PHS for predicting ACT treatment outcomes, we performed a GWAS among patients who received surgery plus ACT (SYSUCC ACT set, n=404) to identify variants associated with clinical outcomes following ACT (Figure 2A). GWAS identified 125 SNPs with P<1.0×10−3 and r2 threshold <0.3. The 37 most discriminative variants selected by LASSO analyses were used to derive a PHS in the training set (Figure 2B,2C; Table S2). The median PHS of 2.237 in the training set was used as the cutoff to divide each participant into high- and low-PHS subgroups. We then analyzed the correlation between PHS groups and clinical characteristics and found most of them were not associated with PHS, except for family cancer history (Table S3).
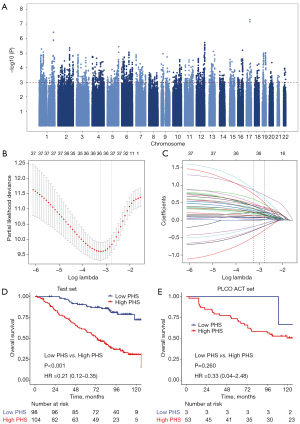
To test whether PHS can predict clinical outcomes following ACT, we investigated the impact of PHS groups on clinical outcomes among those who received ACT. Low-PHS was significantly associated with prolonged OS in test set (HR =0.21, 95% CI: 0.12–0.36, P<0.001; Figure 2D). The 5-year OS was 90.5% (95% CI: 84.7–96.6) for the low-PHS group and 60.3% (95% CI: 51.3–70.8) for the high-PHS group. We further validated the PHS in an independent population (PLCO ACT set, n=56) and a similar trend was observed (HR =0.33, 95% CI: 0.04–2.48, P=0.260; Figure 2E), although the results did not reach statistical significance likely due to the limited sample size. When including sex, age, grade, and TNM stage in a multivariate Cox regression analysis, the PHS still had an independent association with ACT outcomes (Table S4). These results suggested that PHS was associated with ACT outcomes and could affect patients’ survival possibly by modulating the ACT response.
Prognostic value for PHS
In addition to the significant associations between PHS and treatment outcomes following ACT as described above, we further assessed whether PHS was a stable prognostic biomarker independent of treatment strategies and other clinical factors. We firstly assessed whether PHS has a reliable prognostic value regardless of the treatment strategy (such as for patients treated with surgery alone). We observed similar results among the patients with surgery alone that the low PHS patients would have better OS than high PHS patients (SYSUCC surgery alone: HR =0.63, 95% CI: 0.48–0.84, P<0.001; PLCO surgery alone: HR =0.63, 95% CI: 0.38–1.03, P=0.063; Combined: HR =0.63, 95% CI: 0.50–0.80, P<0.001; Figure 3A). We also observed consistent results in the total population for patients with or without ACT (SYSUCC: HR =0.48, 95% CI: 0.38–0.62, P<0.001; PLCO: HR =0.60, 95% CI: 0.37–0.96, P=0.033; Combined: HR =0.52, 95% CI: 0.42–0.65, P<0.001; Figure 3B).
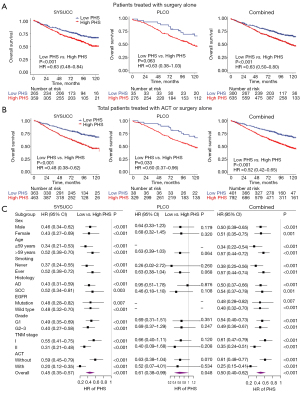
We further assessed the prognostic value of the PHS within each clinical subgroup of patients stratified by TNM stage, sex, age, smoking status, histology, EGFR mutation status, and grade in the validation cohorts. Kaplan-Meier survival analyses showed significant differences in OS between patients with different PHS subgroups in all subgroups (Figure S1). We next performed multivariate Cox regression analyses and still observed consistent results (Figure 3C). Compared with patients with high PHS, patients with low PHS had longer OS in all subgroup analyses (Figure 3C).
These results suggest that PHS shows potential as a stable prognostic biomarker independent of treatment strategies and clinical factors.
Stage II patients with low-PHS benefit most from ACT
Consistent with previous clinical studies, compared with patients treated with surgery alone, stage II patients could achieve additional benefit from ACT, while stage I patients did not (stage I: HR =1.06, 95% CI: 0.78–1.44, P=0.720; stage II: HR =0.66, 95% CI: 0.48–0.92, P=0.022; Figure 4A,4B). Of note, the survival benefit from ACT is moderate in stage II patients, with 12.3% 5-year OS rates improved (ACT: 68.3% vs. surgery alone: 56.0%).
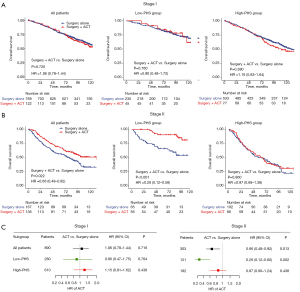
Since the low PHS was significantly associated with longer OS in patients treated with ACT, we hypothesized that the low PHS was associated with better ACT benefits. To verify this conjecture, we next used the PHS to evaluate the survival benefit of ACT. When stratified by PHS, for stage I NSCLC patients, ACT did not exhibit additional OS benefit, regardless of PHS subgroups (Figure 4A). Interestingly, for stage II patients with low PHS, those who received ACT experienced significantly longer OS (HR =0.26, 95% CI: 0.12–0.58, P<0.001; Figure 4B) than those who received surgery alone, with 24.7% 5-year OS rate improved (ACT: 90.4% vs. surgery: 65.7%). However, among patients with high PHS, patients who received ACT showed similar OS to those who underwent surgery alone (HR =0.97, 95% CI: 0.68–1.38, P=0.860; Figure 4B). Multivariate analyses showed similar results (Figure 4C). The HRs of ACT were lower (more protective) in all the low-PHS groups than in the corresponding high-PHS groups. These results indicated that the addition of ACT to surgery was more likely to provide a survival benefit in stage II patients with low PHS.
We used propensity score matching to match the patients with and without ACT. After matching, 516 patients were included (258 in each group) and no statistically significant difference was found in any characteristics (Table S5). Similar results were obtained in the matched cohort. Notably, ACT significantly improved OS in stage II patients with low PHS (HR =0.26, 95% CI: 0.11–0.58, P<0.001), while there was no ACT survival benefit among patients with high PHS (HR =0.84, 95% CI: 0.57–1.22, P=0.360; Figure S2). Therefore, the ACT decision should be carefully made based on the TNM stage and PHS as well.
Discussion
Accurate prediction of clinical outcome and treatment response is critical for prognostic stratification and treatment decision-making for NSCLC patients. In this study, we developed and validated a GWAS-based PHS to predict clinical outcomes and ACT benefit in early-stage NSCLC. The PHS was significantly associated with clinical outcomes and could be used to identify candidates for ACT.
The TNM stage has been widely used to predict prognosis and guide ACT in NSCLC patients (4). For patients with stage I NSCLC, the prognosis is generally favorable and they are likely to be cured by surgery alone (4). For stage II NSCLC, they were thought at a high risk of recurrence, and ACT was recommended by NCCN guidelines (4). However, the anatomically based TNM stage judge the risk of disease progression mainly according to tumor invasion without considering the other factors associated with individuals’ heterogeneity, such as the genetic background. Our study demonstrated that the PHS could complement the TNM stage by providing additional predictive information. The PHS could classify patients with different long-term clinical outcomes independently of the TNM stage and other clinical factors. More importantly, the PHS showed predictive value for ACT benefit and the addition of ACT to surgery was more likely to provide a survival benefit in stage II patients with low PHS. Hence, A key potential utility of the work is to use the PHS to guide individualized treatment plans. For instance, for stage II patients with low PHS, the ACT is strongly recommended because they can derive considerable survival benefits from the ACT. However, for stage II patients with high PHS, the ACT might not be the optimal adjuvant therapy, and alternative treatment should be received as soon as possible to catch the optimal treatment timing.
Several other predictive signatures for ACT benefit prediction have been developed in previous research, such as tumor tissue-based transcriptional signatures (40-42), blood-based circulating tumor DNA signatures (43), and radiomic features (44,45). However, few studies have been made on polygenic scores for prediction prognosis and treatment response in NSCLC. Investigation into the genetic features of the individuals could give additional insight into biological rationales for prognosis and drug response. Interestingly, several SNPs included in the PHS have been reported associated with disease progression, implying the potential role of the susceptibility genes involved in NSCLC development and survival. For example, rs17080884, located at chromosome 5q35.3 within the intergenic of TRIM7 and MIR463, is associated with the survival of NSCLC patients (for T allele: OR =2.18, P=8.75×10−6). The expression quantitative trait locus (eQTL) from GTEX.v8 data suggested that rs17080884-T allele is associated with higher expression of TRIM7 expression in several tissues, indicating the potential regulatory effect of rs17080884 on TRIM7. TRIM7 is a member of the tripartite motif family and its overexpression was associated with poor prognosis in osteosarcoma (46). Another study found that the high expression of TRIM7 increased lung tumor burden via increasing tumor growth in a Ras-driven cancer model (47). Another variant rs3857953 located at intronic region of MTSS1, was associated with OS (for C allele: OR =0.57, P=2.29×10−4). The eQTL analysis suggested that rs3857953-C allele is associated with higher expression of MTSS1 expression in the artery aorta. MTSS1 is a latent metastasis suppressor gene and has been reported to inhibit prostate cancer cell migration and proliferation (48), and also plays a role in the invasion and metastasis of lung cancer cells (49). The collective evidence suggested that these variants may be associated with the prognosis of NSCLC via the regulation effect on gene expression. We think this work provides more evidence to verify the concept that treatment recommendation strategy can be further optimized with genetic data. However, the biological mechanism of PHS affecting the treatment outcome has not been elucidated and further investigations into the SNPs functions might provide possible treatment targets.
The potential benefits of polygenic scores include predicting disease risk (11), stratifying patients (50), and delivering personalized treatment (29,30). Despite these potential benefits, there are potential risks that should be acknowledged. First of all, the construction of a standard and robust genetic score is an important premise of polygenic scores applications. In our study, The GWAS population had strict inclusion and exclusion criteria, as a subgroup from a larger study cohort (Guangzhou GSA GWAS). Patients in the GWAS cohort received the same treatment region (surgery plus ACT) and the homogenous population allowed us to focus on genetic variants with fewer biases. Second, it is very important that test the polygenic score performance in an independent dataset so that its reproducibility can be validated. In the present study, the performance of the PHS was firstly validated in an independent dataset of SYSUCC surgery set and subsequently in PLCO cohort, which proceeds from a population-based cohort better representing a real-world study cohort (31). Finally, genetics is merely one contributing factor to prognosis and additional predictive biomarkers (such as those mentioned above), as well as clinical factors, would further improve the ability of clinicians to optimize ACT strategies.
The limitations of our study are worthy of discussion. First, the SNPs used to construct the PHS did not exceed the genome-wide threshold for significance of 5×10−8 given the limited sample size of this GWAS. Nevertheless, it can be noted that despite the small sample sizes, our PHS was successfully validated associated with clinical outcomes in independent datasets. In the future, multi-center studies with larger sample sizes are still needed to optimize and validate the PHS. Second, the PHS showed a relatively higher prognostic value for Asian population (Guangzhou GSA GWAS cohort) than that for the European populations (PLCO cohort). Previous studies have shown that the prediction accuracy for polygenic scores would be decreased across different racial populations, especially when the discovery and target samples are from different races, with the population-specific allele frequencies and linkage disequilibrium (LD) patterns being two main reasons for this phenomenon (25,51-53). Therefore, in further research, it is needed to develop population-specific PHSs for other ancestral groups (e.g., European or African ancestry). Third, although the PLCO cohort was a subset of a prospective cohort, the other datasets were retrospective cohorts which could have introduced selection bias. While we have attempted to minimize the bias by adjusting for baseline clinical factors, other factors, that we failed to include in this study, could play a role, such as lymphovascular invasion (LVI), the spread of tumor through the airspaces (STAS), PD-L1 expression status and other driver gene’s expression. Considering the limitation mentioned, future studies with more rigorous design and comprehensive clinical data are needed to confirm the study findings.
In conclusion, we constructed a GWAS-derived PHS that effectively predicted clinical outcomes and ACT benefits in NSCLC patients. The PHS might help clinicians identify which patients are expected to benefit from ACT. Further research is also needed to optimize the PHS and ascertain how PHS can be effectively applied to clinical practice.
Acknowledgments
Funding: This study was funded by the National Key Research and Development Program of China (Nos. 2021YFC2500400, 2017YFC0907900), the Basic and Applied Basic Research Foundation of Guangdong Province, China (No. 2021B1515420007), the Special Support Program for High-level Professionals on Scientific and Technological Innovation of Guangdong Province, China (No. 2014TX01R201), National Natural Science Foundation of China (Nos. 81973131, 81903395, 82003520, 81803319, 81802708), and High Performance Computation Application Project, Sun Yat-sen University (No. 84000-31143413).
Footnote
Reporting Checklist: The authors have completed the TRIPOD reporting checklist. Available at https://tlcr.amegroups.com/article/view/10.21037/tlcr-22-139/rc
Data Sharing Statement: Available at https://tlcr.amegroups.com/article/view/10.21037/tlcr-22-139/dss
Conflicts of Interest: All authors have completed the ICMJE uniform disclosure form (available at https://tlcr.amegroups.com/article/view/10.21037/tlcr-22-139/coif). The authors have no conflicts of interest to declare.
Ethical Statement:
Open Access Statement: This is an Open Access article distributed in accordance with the Creative Commons Attribution-NonCommercial-NoDerivs 4.0 International License (CC BY-NC-ND 4.0), which permits the non-commercial replication and distribution of the article with the strict proviso that no changes or edits are made and the original work is properly cited (including links to both the formal publication through the relevant DOI and the license). See: https://creativecommons.org/licenses/by-nc-nd/4.0/.
References
- Siegel RL, Miller KD, Fuchs HE, et al. Cancer statistics, 2022. CA Cancer J Clin 2022;72:7-33. [Crossref] [PubMed]
- Gridelli C, Rossi A, Carbone DP, et al. Non-small-cell lung cancer. Nat Rev Dis Primers 2015;1:15009. [Crossref] [PubMed]
- Butts CA, Ding K, Seymour L, et al. Randomized phase III trial of vinorelbine plus cisplatin compared with observation in completely resected stage IB and II non-small-cell lung cancer: updated survival analysis of JBR-10. J Clin Oncol 2010;28:29-34. [Crossref] [PubMed]
- NCCN Clinical Practice Guidelines in Oncology: Non-Small Cell Lung Cancer. Version 3.2022. 2022.
- Kris MG, Gaspar LE, Chaft JE, et al. Adjuvant Systemic Therapy and Adjuvant Radiation Therapy for Stage I to IIIA Completely Resected Non-Small-Cell Lung Cancers: American Society of Clinical Oncology/Cancer Care Ontario Clinical Practice Guideline Update. J Clin Oncol 2017;35:2960-74. [Crossref] [PubMed]
- Pignon JP, Tribodet H, Scagliotti GV, et al. Lung adjuvant cisplatin evaluation: a pooled analysis by the LACE Collaborative Group. J Clin Oncol 2008;26:3552-9. [Crossref] [PubMed]
- NSCLC Meta-analyses Collaborative Group. Adjuvant chemotherapy, with or without postoperative radiotherapy, in operable non-small-cell lung cancer: two meta-analyses of individual patient data. Lancet 2010;375:1267-77. [Crossref] [PubMed]
- Felip E, Rosell R, Maestre JA, et al. Preoperative chemotherapy plus surgery versus surgery plus adjuvant chemotherapy versus surgery alone in early-stage non-small-cell lung cancer. J Clin Oncol 2010;28:3138-45. [Crossref] [PubMed]
- Waller D, Peake MD, Stephens RJ, et al. Chemotherapy for patients with non-small cell lung cancer: the surgical setting of the Big Lung Trial. Eur J Cardiothorac Surg 2004;26:173-82. [Crossref] [PubMed]
- Scagliotti GV, Fossati R, Torri V, et al. Randomized study of adjuvant chemotherapy for completely resected stage I, II, or IIIA non-small-cell Lung cancer. J Natl Cancer Inst 2003;95:1453-61. [Crossref] [PubMed]
- Dai J, Lv J, Zhu M, et al. Identification of risk loci and a polygenic risk score for lung cancer: a large-scale prospective cohort study in Chinese populations. Lancet Respir Med 2019;7:881-91. [Crossref] [PubMed]
- Chang IS, Jiang SS, Yang JC, et al. Genetic Modifiers of Progression-Free Survival in Never-Smoking Lung Adenocarcinoma Patients Treated with First-Line Tyrosine Kinase Inhibitors. Am J Respir Crit Care Med 2017;195:663-73. [Crossref] [PubMed]
- Huang YT, Heist RS, Chirieac LR, et al. Genome-wide analysis of survival in early-stage non-small-cell lung cancer. J Clin Oncol 2009;27:2660-7. [Crossref] [PubMed]
- Ma Q, Lu AY. Pharmacogenetics, pharmacogenomics, and individualized medicine. Pharmacol Rev 2011;63:437-59. [Crossref] [PubMed]
- Li XP, Yin JY, Wang Y, et al. The ATP7B genetic polymorphisms predict clinical outcome to platinum-based chemotherapy in lung cancer patients. Tumour Biol 2014;35:8259-65. [Crossref] [PubMed]
- Wang Y, Yin JY, Li XP, et al. The association of transporter genes polymorphisms and lung cancer chemotherapy response. PLoS One 2014;9:e91967. [Crossref] [PubMed]
- Feng J, Sun X, Sun N, et al. XPA A23G polymorphism is associated with the elevated response to platinum-based chemotherapy in advanced non-small cell lung cancer. Acta Biochim Biophys Sin (Shanghai) 2009;41:429-35. [Crossref] [PubMed]
- Yang Y, Gao W, Ding X, et al. Variations within 3'-UTR of MDM4 gene contribute to clinical outcomes of advanced non-small cell lung cancer patients following platinum-based chemotherapy. Oncotarget 2017;8:16313-24. [Crossref] [PubMed]
- Pu X, Hildebrandt MA, Lu C, et al. PI3K/PTEN/AKT/mTOR pathway genetic variation predicts toxicity and distant progression in lung cancer patients receiving platinum-based chemotherapy. Lung Cancer 2011;71:82-8. [Crossref] [PubMed]
- Hu L, Wu C, Zhao X, et al. Genome-wide association study of prognosis in advanced non-small cell lung cancer patients receiving platinum-based chemotherapy. Clin Cancer Res 2012;18:5507-14. [Crossref] [PubMed]
- Wu X, Ye Y, Rosell R, et al. Genome-wide association study of survival in non-small cell lung cancer patients receiving platinum-based chemotherapy. J Natl Cancer Inst 2011;103:817-25. [Crossref] [PubMed]
- Sato Y, Yamamoto N, Kunitoh H, et al. Genome-wide association study on overall survival of advanced non-small cell lung cancer patients treated with carboplatin and paclitaxel. J Thorac Oncol 2011;6:132-8. [Crossref] [PubMed]
- Ding Y, Hou K, Burch KS, et al. Large uncertainty in individual polygenic risk score estimation impacts PRS-based risk stratification. Nat Genet 2022;54:30-9. [Crossref] [PubMed]
- Polygenic Risk Score Task Force of the International Common Disease Alliance. Responsible use of polygenic risk scores in the clinic: potential benefits, risks and gaps. Nat Med 2021;27:1876-84. [Crossref] [PubMed]
- Martin AR, Kanai M, Kamatani Y, et al. Clinical use of current polygenic risk scores may exacerbate health disparities. Nat Genet 2019;51:584-91. [Crossref] [PubMed]
- Sugrue LP, Desikan RS. What Are Polygenic Scores and Why Are They Important? JAMA 2019;321:1820-1. [Crossref] [PubMed]
- Lewis CM, Vassos E. Polygenic risk scores: from research tools to clinical instruments. Genome Med 2020;12:44. [Crossref] [PubMed]
- Wei JH, Feng ZH, Cao Y, et al. Predictive value of single-nucleotide polymorphism signature for recurrence in localised renal cell carcinoma: a retrospective analysis and multicentre validation study. Lancet Oncol 2019;20:591-600. [Crossref] [PubMed]
- Tian XP, Ma SY, Young KH, et al. A composite single-nucleotide polymorphism prediction signature for extranodal natural killer/T-cell lymphoma. Blood 2021;138:452-63. [Crossref] [PubMed]
- Lanfear DE, Luzum JA, She R, et al. Polygenic Score for β-Blocker Survival Benefit in European Ancestry Patients With Reduced Ejection Fraction Heart Failure. Circ Heart Fail 2020;13:e007012. [Crossref] [PubMed]
- Hocking WG, Hu P, Oken MM, et al. Lung cancer screening in the randomized Prostate, Lung, Colorectal, and Ovarian (PLCO) Cancer Screening Trial. J Natl Cancer Inst 2010;102:722-31. [Crossref] [PubMed]
- Oken MM, Marcus PM, Hu P, et al. Baseline chest radiograph for lung cancer detection in the randomized Prostate, Lung, Colorectal and Ovarian Cancer Screening Trial. J Natl Cancer Inst 2005;97:1832-9. [Crossref] [PubMed]
- Delaneau O, Marchini J, Zagury JF. A linear complexity phasing method for thousands of genomes. Nat Methods 2011;9:179-81. [Crossref] [PubMed]
- Howie BN, Donnelly P, Marchini J. A flexible and accurate genotype imputation method for the next generation of genome-wide association studies. PLoS Genet 2009;5:e1000529. [Crossref] [PubMed]
- Mailman MD, Feolo M, Jin Y, et al. The NCBI dbGaP database of genotypes and phenotypes. Nat Genet 2007;39:1181-6. [Crossref] [PubMed]
- Tryka KA, Hao L, Sturcke A, et al. NCBI's Database of Genotypes and Phenotypes: dbGaP. Nucleic Acids Res 2014;42:D975-9. [Crossref] [PubMed]
- Rizvi AA, Karaesmen E, Morgan M, et al. gwasurvivr: an R package for genome-wide survival analysis. Bioinformatics 2019;35:1968-70. [Crossref] [PubMed]
- Goeman JJ. L1 penalized estimation in the Cox proportional hazards model. Biom J 2010;52:70-84. [PubMed]
- Austin PC. An Introduction to Propensity Score Methods for Reducing the Effects of Confounding in Observational Studies. Multivariate Behav Res 2011;46:399-424. [Crossref] [PubMed]
- Rosell R, Karachaliou N. Gene Expression Signatures Predicting Survival and Chemotherapy Benefit in Patients with Resected Non-small-Cell Lung Cancer. EBioMedicine 2018;33:16-7. [Crossref] [PubMed]
- Xie Y, Lu W, Wang S, et al. Validation of the 12-gene Predictive Signature for Adjuvant Chemotherapy Response in Lung Cancer. Clin Cancer Res 2019;25:150-7. [Crossref] [PubMed]
- Shukla S, Evans JR, Malik R, et al. Development of a RNA-Seq Based Prognostic Signature in Lung Adenocarcinoma. J Natl Cancer Inst 2016;109:djw200. [Crossref] [PubMed]
- Devarakonda S, Rotolo F, Tsao MS, et al. Tumor Mutation Burden as a Biomarker in Resected Non-Small-Cell Lung Cancer. J Clin Oncol 2018;36:2995-3006. [Crossref] [PubMed]
- Vaidya P, Bera K, Gupta A, et al. CT derived radiomic score for predicting the added benefit of adjuvant chemotherapy following surgery in Stage I, II resectable Non-Small Cell Lung Cancer: a retrospective multi-cohort study for outcome prediction. Lancet Digit Health 2020;2:e116-28. [Crossref] [PubMed]
- Xie D, Wang TT, Huang SJ, et al. Radiomics nomogram for prediction disease-free survival and adjuvant chemotherapy benefits in patients with resected stage I lung adenocarcinoma. Transl Lung Cancer Res 2020;9:1112-23. [Crossref] [PubMed]
- Zhou C, Zhang Z, Zhu X, et al. N6-Methyladenosine modification of the TRIM7 positively regulates tumorigenesis and chemoresistance in osteosarcoma through ubiquitination of BRMS1. EBioMedicine 2020;59:102955. [Crossref] [PubMed]
- Chakraborty A, Diefenbacher ME, Mylona A, et al. The E3 ubiquitin ligase Trim7 mediates c-Jun/AP-1 activation by Ras signalling. Nat Commun 2015;6:6782. [Crossref] [PubMed]
- Du P, Ye L, Ruge F, et al. Metastasis suppressor-1, MTSS1, acts as a putative tumour suppressor in human bladder cancer. Anticancer Res 2011;31:3205-12. [PubMed]
- Liu M, Zeng X, Lu YX, et al. Study on molecular mechanism of MiRNA-29a in promoting proliferation and invasion of non-small-cell lung cancer by inhibiting MTSS1. Eur Rev Med Pharmacol Sci 2018;22:5531-8. [PubMed]
- Seibert TM, Fan CC, Wang Y, et al. Polygenic hazard score to guide screening for aggressive prostate cancer: development and validation in large scale cohorts. BMJ 2018;360:j5757. [Crossref] [PubMed]
- Martin AR, Gignoux CR, Walters RK, et al. Human Demographic History Impacts Genetic Risk Prediction across Diverse Populations. Am J Hum Genet 2017;100:635-49. [Crossref] [PubMed]
- Duncan L, Shen H, Gelaye B, et al. Analysis of polygenic risk score usage and performance in diverse human populations. Nat Commun 2019;10:3328. [Crossref] [PubMed]
- Wang Y, Guo J, Ni G, et al. Theoretical and empirical quantification of the accuracy of polygenic scores in ancestry divergent populations. Nat Commun 2020;11:3865. [Crossref] [PubMed]