Plasma RNA profiling unveils transcriptional signatures associated with resistance to osimertinib in EGFR T790M positive non-small cell lung cancer patients
Introduction
Lung cancer is the most common cancer worldwide resulting in nearly 20% of all cancer deaths (1). The most common subgroup of lung cancer is non-small cell lung cancer (NSCLC) constituting around 85% of lung cancer cases. Activating mutations in the gene encoding epidermal growth factor receptor (EGFR) occur in approximately 15% of all NSCLC adenocarcinomas in Western patients with the prevalence being 3–4 times higher in Asians (2). These mutations result in a constitutively active EGFR receptor promoting uncontrolled proliferation, invasion and metastasis (3). The vast majority of NSCLCs harboring activating mutations in EGFR respond favorably to first-generation ATP-competitive tyrosine kinase inhibitors (TKIs) erlotinib and gefitinib (4-6), or to second-generation EGFR TKIs afatinib and dacomitinib that irreversibly bind to the kinase domain. Although first- and second-generation TKIs are clinically favorable compared to platinum-based therapy, inevitably all such tumors develop resistance to these TKIs, which is partly a consequence of the emergence of a secondary mutation, T790M (7). This mutation results in an increased affinity for ATP, negating the efficacy of ATP-competitive TKIs (8). Osimertinib is a covalent irreversible third-generation TKI, which targets NSCLCs with activating mutations in EGFR regardless of presence of the T790M mutation (9). Osimertinib received U.S. Food and Drug Administration (FDA)-approval in 2017 as second-line therapy, followed by approval as first-line therapy in 2018 by both the FDA and the European Medicines Agency (EMA) (10-14). Despite the impressive effects of osimertinib in the clinical setting, patients receiving osimertinib eventually develop resistance. Known resistance mechanisms involve acquired mutation of the drug-binding cysteine, C797S, as well as amplifications of MET, HER2 and PIK3CA, together accounting for up to 50% of resistant cases. Furthermore, a large fraction of EGFR T790M NSCLCs progressing on osimertinib exhibit lost T790M-status (15-17). Several of the reported resistance mechanisms impact cell signaling pathways, likely altering gene transcription programs. Therefore, investigation of transcriptional changes is imperative for uncovering RNA biomarkers as well as understanding potential mechanisms and biological outcomes of acquired resistance to osimertinib. This requires repeated biopsies to capture the progression of the disease. Repeated solid tissue biopsy sampling presents an invasive clinical procedure that only captures the molecular nature of the cells at the sampling site. In contrast, blood liquid biopsies will potentially capture all RNAs shed into the bloodstream in extracellular vesicles, including exosomes, from any tumor cell, potentially minimizing tumor heterogeneity. The drawback of liquid biopsies is that the subsequent profiling cannot distinguish tumor-derived vesicle-bound RNA from vesicle-bound RNA shed from healthy tissues. However, studying datasets of tens or even hundreds of solid tissue biopsy samples does not guarantee the identification of a common denominator for a specific phenotype. This demands rigorous cross-validation approaches, involving independently collected datasets. Furthermore, analysis would gain power by summarizing sparse individual gene events to the pathway level. The method of network enrichment analysis applied here allowed accounting for any altered transcripts regardless of expression of pathway genes (18,19). Using this approach, in an aim to identify potential new biomarkers of resistance, we have studied longitudinal changes in the transcriptional landscape of plasma extracellular vesicle bound RNAs from a cohort of EGFR T790M NSCLC patients receiving osimertinib as second-line treatment, and demonstrate that blood plasma serves as a comprehensive RNA source and that our major biological findings from plasma extracellular vesicle bound RNAs are corroborated using public and newly generated cell model data. We present the following article in accordance with the MDAR reporting checklist (available at https://tlcr.amegroups.com/article/view/10.21037/tlcr-22-236/rc).
Methods
Patient cohort and sample preparation
Twenty patients were included in the study. Five patients were males (38, 68, 43, 65 and 78 years old) and fifteen patients were females (62, 69, 53, 73, 56, 60, 75, 79, 59, 75, 76, 66, 69, 61 and 64 years old). All patients were enrolled in the Northern European multicenter phase II TREM study (EudraCT No. 2015-000307-10) and diagnosed with EGFR T790M-mutant NSCLC with a treatment history involving disease progression on minimum one first and/or second-generation EGFR TKI (20). The patients were treated with osimertinib 80 mg daily until radiologic progression. In this osimertinib-treated cohort, samples of twelve patients were collected via the Oslo University Hospital and eight patients at the Karolinska University Hospital. Whole blood was drawn just before treatment start (baseline) and at radiological disease progression on osimertinib treatment while patients were still on osimertinib and just before change of therapy. Plasma was separated through centrifugal isolation, 2,000 g for 15 min, and aliquoted to fresh 1ml tubes and stored at -80C. The regional ethical committees at respective hospitals approved sampling for this study.
EGFR mutant parental cell lines NCI-H1975 and HCC827 and TKI-refractory cell lines (erlotinib-resistant HCC827, gefitinib-resistant HCC827, osimertinib-resistant HCC827 and osimertinib-resistant NCI-H1975) (21) were cultured in RPMI-1640 medium with 10% supplemented Fetal Bovine Serum at 5% CO2, 37 ℃, and passaged when reaching sub-confluent conditions.
RNA extraction
Exosomal RNA was isolated at Karolinska Institutet. 1 mL plasma/sample point was centrifuged at 16,000 g for 10 minutes followed by processing using the ExoRNeasy serum plasma midi kit (Qiagen), as previously described (22), and the RNA was eluted in 14 µL RNase free water. RNA quantity and quality were assessed through the documentation of RNA integrity number (RIN) curves. All samples selected for analysis displayed similar RIN curves with a range from 1.50–2.90. Cell line total RNA was extracted from EGFR mutant TKI-refractory NSCLC cell lines (erlotinib-resistant HCC827, gefitinib-resistant HCC827, osimertinib-resistant HCC827 and osimertinib-resistant NCI-H1975) and EGFR mutant parental NSCLC cell lines (HCC827 and NCI-H1975) (21) using mirVana miRNA isolation kit (ThermoFisher Scientific Cat #AM1560).
Transcriptome analysis
3 µL of eluted exosomal total RNA, or cell line total RNA, was pre-amplified for 6 cycles before loaded onto Clariom D Pico Assay, human transcriptome arrays (ThermoFisher Scientific #902925). Cell line total RNA was loaded onto Clariom D Pico Assay in biological duplicates. Transcript expression values were normalized using Signal Space Transformation (SST-RMA) method.
Exploratory and statistical analyses
The SST-RMA values were bell-shape distributed, although the right tail was too extended. Therefore, the values were further log-transformed in order to render distribution closer to Gaussian and ensure homoscedasticity and usage of parametric statistics. However, the fold change values and boxplots visualization were based on the original SST-RMA values. Principal component analysis, Volcano plot analysis, RNA-class distribution analysis and differential gene expression analysis was performed for 81042 transcripts with Clariome annotation in R environment using functions from package base. The removal of batch effects, generalized least squared models and network enrichment analysis were implemented with R packages limma, nlme and NEArender, respectively (https://cran.r-project.org/web/packages/).
Network enrichment analysis
The network enrichment analysis (NEA) employs the global network, which combines all major types of molecular interactions in an unbiased way. By utilizing this topological information, NEA can render experimentally observed molecular alterations into a space of pathways and processes. The pathway view enables lower dimensionality, is more transparent for biological interpretation compared to other multivariate methods, and is also more efficient in absence of replicates—which is a typical situation in patient sample collections.
Similarly to the well-known over-representation analysis (ORA), NEA can analyze experimental altered gene sets (AGS), such as top N differentially expressed genes (DEG). AGSs are then tested for enrichment, i.e., significant “relatedness” with regard to (usually a large collection of) functional gene sets (FGS), such as pathways or other custom sets of biological importance. In ORA, enrichment of FGS versus AGS is determined by the fraction of genes shared by the two sets. NEA instead counts network edges that connect genes of AGS with any genes of FGS, which number is compared to a number expected by chance. The latter is influenced by variability in network edge numbers of involved genes and is therefore normalized by topological properties (node degrees) of gene nodes in the global network.
NEA assigns profiles of FGS enrichment scores to each submitted AGS, which then could be used as either descriptive or predictive variables in the same way as gene expression profiles. NEA possesses higher statistical power to detect enrichment compared to ORA (18) and better reproducibility compared to both using raw gene profiles and diverse enrichment methods, which were tested in a systematic benchmark (19). Furthermore, NEA can identify network enrichment against e.g., signaling pathways, members of which not necessarily changed their own expression.
The integrated framework of NEA consists of three components: AGSs for each clinical sample, a sufficiently large collection of FGSs, and a version of global interaction network. The analysis was run in R environment using package NEArender of version 1.4. NEArender produced network enrichment scores for each AGS-FGS pair.
Global network version
The global network for NEA was a set of functional links from curated databases collected in the Pathway Commons project (version 9) (23) with 846,631 unique edges between 20,063 unique human gene nodes.
Functional gene sets (FGS)
The collection of pathways and gene sets included all entries from BioCarta, KEGG, Reactome, WikiPathways, MetaCyc, PID databases as well as 50 hallmarks from MSigDB. In addition, we used immunologic MSigDB collection C7 of 4872 signatures.
Altered gene sets (AGS) from cells and patients
For each sample, a specific AGS was compiled as a list of 25 genes most deviating by expression values from the rest of samples in the respective cohort (either cell lines or patients).
Network visualization
The sub-networks for illustrations were generated at the public NEA resource https://www.evinet.org/ (24).
Least squares models for patients and cells
R packages base, car, nlme were employed, so that the models are presented using R code and functions of these packages.
Model PA-1 (repeated measures ANOVA)
gls( model = |
Expression ~ Origin + Concentration + Type + Concentration * Type, |
correlation = corAR1( |
form = ~ Type | Patients, |
value = ACF( |
gls(model = Expression ~ Origin + Concentration + Type + Concentration * Type), |
form = ~ Levels | Patients |
)[2,2] |
), method=”REML”); |
Expression: gene expression; |
Concentration: RNA concentration in the samples; |
Origin: Oslo or Stockholm site; |
Type: baseline or progression. |
Model CL-1 (2-way ANOVA with interaction term)
aov(Expression ~ Line + Type + Line * Type) |
with variables |
Expression: gene expression; |
Line: parental cell line, HCC827 or NCIH1975; |
Type: original or resistant. |
Model CCLE-1 (3-way ANOVA with one interaction term)
anova(lm(Sensitivity ~ Tissue + EGFR + Expression + EGFR * Expression)), |
with factors |
Sensitivity: to one of [ER, GE, OS]; |
Tissue: tissue or organ of the original tumor; |
EGFR: mutation status; |
Expression: gene expression. |
Model PCA-1 (3-way ANOVA without interaction terms)
anova(lm(PC ~ Origin + Concentration + Type)), |
with variables |
PC: sample-specific principal component value; |
Concentration: RNA concentration in the samples; |
Origin: Oslo or Stockholm; |
Type: baseline or progression. |
Ethical statement
The study was conducted in accordance with the Declaration of Helsinki (as revised in 2013) and the ICH-Guidelines of Good Clinical Practice and according to regulatory requirements. This study received ethical approval by the institutional review board at Karolinska University Hospital (registration No. 2016/944-31/1) and Oslo North Regional Ethics Board (No. 2015/181). Additional approvals by Stockholm Medical Biobank (No. Bbk-01605) were received. All patients provided written informed consent.
Data availability statement
The data generated in this study are available within the article and its supplementary data files. Raw data for this study were generated at the Karolinska Institutet BEA core facility and is available from the corresponding author upon request.
Sample definition and in-laboratory replication
All experiments were conducted using biological replicates. Visualized data reflects either all biological replicates, or representative biological replicates, as stated. If visualized with error bars, each data point represents all biological replicates of a specific analysis group.
Results
Transcript coverage of plasma-derived exosomal RNA
To demonstrate that blood plasma serves as a comprehensive RNA source, we analyzed exosomal RNA from plasma sampled at baseline and progression of disease from twenty EGFR-mutant NSCLC patients receiving osimertinib in a multicenter phase II study (20) (Table 1, Table S1) as well as RNA derived from six EGFR-mutant NSCLC cell lines (two parental and four TKI-refractory) (21). First, we compared the level of per chromosome representation of mRNA transcripts detected in our blood plasma and cell line samples to RNA-seq transcriptomics data from traditionally used cancer samples: either in vitro Cancer Cell Line Encyclopedia (CCLE) cell cultures or 545 primary, fresh-frozen NSCLC tumors (TCGA) (21,25-27) (Figure 1). Using as reference a collection of 386 cancer-related genes, we found that detectable gene expression per chromosome was more variable in the blood plasma samples. However, the overall representation of the mRNA-landscape was found fairly similar between the plasma samples (Clariom D platform), our cell line samples (Clariom D platform), and RNA-seq CCLE and The Cancer Genome Atlas (TCGA) samples, with a maximum of 81% detected genes per sample per chromosome (Table S2).
Table 1
Assessment | Value |
---|---|
Gender (%) | Male: 5/20 (25%), female: 15/20 (75%) |
Median age, years (range) | 64 (38–79) |
Smoking status (%) | Current: 2/20 (10%), ex-smoker: 9/20 (45%), never smoker: 9/20 (45%) |
Performance status (%) | 0: 7/20 (35%), 1: 12/20 (60%), 2: 1/20 (5%) |
Histology (%) | Adenocarcinoma: 20/20 (100%) |
Stage at diagnosis (%) | IV: 20/20 (100%) |
EGFR mutation subtype (%) | Exon 19: 15/20 (75%), L858R: 4/20 (20%), Exon 18: 1/20 (5%), T790M: 20/20 (100%) |
Median PFS osimertinib (months) | 10.6 |
EGFR, epidermal growth factor receptor; PFS, progression-free survival.
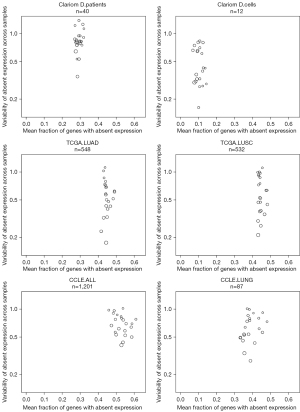
Next, we explored possible variability of transcriptomics data due to known factors in a principal component analysis (PCA) of all the 40 samples (Figures S1,S2, Table S3). In order to detect potential influence of sample RNA concentration, site of delivery (“origin”, i.e., Stockholm or Oslo) as well as sample type (“baseline vs. progression”) on specific principal components (PC), we subjected each PC to linear model analysis (model PCA-1, see Methods). While most components were not associated with any changes between baseline and progression, PC32 clearly separated baseline from progression samples (Figures S1,S2). This PCA investigation confirmed that influence of the three factors on variability in RNA expression should be accounted for. Therefore, the subsequent analysis of differential expression between baseline and progression included necessary covariates and an interaction term “Concentration * Type” (model PA-1). As an example, we included paired sample information in a repeated measures model on individual genes (model PA-1) and found that most informative genes from PC32 significantly overlapped with genes detected by this model. Differential RNA expression was most pronounced for PYY3, ABCA2, MT1L, PRODH2, HMGB1P19, MIR892B and OR56B2P (FDR <0.0001) (Table S4).
Dynamics of plasma-derived exosomal RNA
Based on the conclusions above, we evaluated differential RNA abundance in plasma derived exosomes between baseline and progression samples using a repeated measures model PA-1, which accounted for patients’ identities and detected changes due to tumor progression while subtracting influence of total RNA concentration and batch effect of delivery site. We required the interaction term “concentration * progression/baseline” to be insignificant in order to exclude less stable findings. In total, 128 transcripts displayed significant differential expression [log2(fold-change) >0.25, FDR P<0.15, value for interaction term “concentration X progression/baseline” >0.05] (Figure 2A, Table S5). Among these, expression of 41 and 87 transcripts decreased and increased toward progression, respectively. Importantly, the most pronounced genes from PC32 (Table S4) were among the top differentially expressed genes. In addition, we detected multiple genes in cell signaling pathway, immune system pathway and transcription, including MKNK1, RASA1, RGS18, IL17RA, ZNF17 and LIN9 (Figure 2B-2I, Table S5).
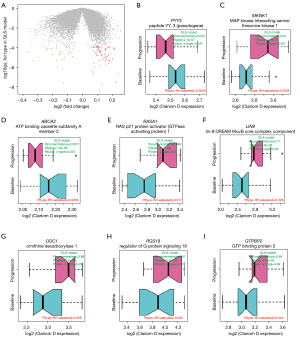
Pathway enrichment of differentially expressed genes
The differential expression analysis presented above produced a list of genes altered during the treatment. In order to characterize the list at a more general level we subjected the DEG list as an altered gene set (AGS) to network enrichment analysis (NEA) against a collection of 6,529 functional gene sets (FGS). The NEA approach (18) is similar to over-representation analysis of DEG but considers the network context of each gene (Figure 3). This overall NEA exposed a number of highly enriched pathways (FDR <3×10-10), including pathways related to ERBB and PI3K signaling, as well as syndecan and glypican pathways. The glypican pathway produced the highest number of AGS to FGS links. Interestingly, we also observed several immune-related pathways, including IFN-gamma and IL-6 signaling pathways (Figure 4A,4B, Table S6). The network analysis considers transcripts present in the global interaction network, i.e., nearly all protein coding genes, most miRNAs, but neither long intergenic non-coding RNAs nor pseudogenes. A minor fraction of the pathway genes were identified as DEGs, which emphasizes that enrichment methods not using network analysis would be unlikely to detect these relations.
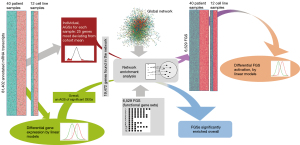
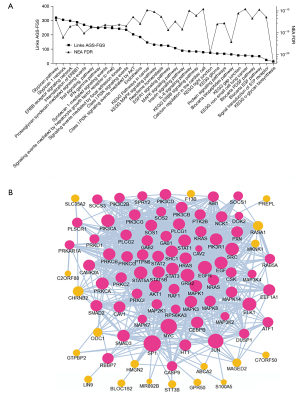
Differential pathway activation in plasma-derived exosomal RNA
The overall approach above detected pathways that characterized the DEG list as an integral, coherent gene group. Furthermore, we also created individual, patient-specific altered gene sets (AGS) by gathering genes that differed in each given sample from the cohort gene means (Figure 3). We compiled 40 sample-specific AGSs and subjected them to NEA. This produced a matrix of 40× 6,529 enrichment values and enabled using NEA scores in the same way as the original mRNA expression values, with the difference that 6,529 NEA profiles were used instead of RNAs. Namely, we detected differential pathway activation (DPA) for 16 out of 6,529 FGSs [FDR<0.25 and P(interaction) >0.05], also requiring that NEA z-score should exceed 3 in at least one of the 40 samples (Figure 5, Figure S3, Table S7). One of the 16 differentially activated pathways, gene set GSE35825, displayed biological overlap with FGSs detected in the overall NEA approach (Figures 4A,5A, Tables S6,S7). The original publication by Liu et al. presented a transcriptomics dataset comparing IFN-alpha versus IFN-gamma stimulated macrophages derived from mouse bone marrow (28). The data was further processed to present the 200 most differentially expressed genes. In our patient data, the sample-specific gene sets were often linked in the global network to the GSE35825-based set of 200 genes and this linkage, quantified as NEA Z-scores, manifested values in the baseline samples systematically higher than the matching progression samples (Figure 5A). Interestingly, one gene of the GSE35825-based set [Endothelin 1 (EDN1) (29)] was consistently upregulated in the progression samples (Figure 5B,5C). In general, it appeared that immunity-related pathways dominated the differences between baseline and progression phenotypes (Table S7).
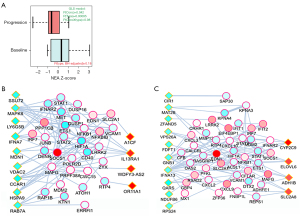
Reciprocal validation using the three data sources
In order to demonstrate that our findings reflect a potential biological context of acquired resistance upon progression, we used the web resource www.evicor.org (30) in order to match the results obtained in the patient cohort to sensitivity correlates from our TKI-refractory cell line panel and CCLE dataset. We could estimate overlaps between sets of lower p-value correlates between different analyses. This approach, by calculating Fisher’s exact statistics of the overlap and controlling error rates via appropriate adjustment for multiple testing, demonstrated that there was a statistically significant match between the findings in all pairwise comparisons and at both gene and pathway levels (Table S8). In total, our cell line panel resulted in 64 enriched pathways related to TKI-resistance versus 33 enriched pathways in the patient cohort (Table S8). Since the experimental setups behind the three data sources were entirely independent, the overlaps indicate a biological and clinical relevance of the patient blood plasma sampling.
Discussion
In this study, we investigated the transcriptome from longitudinally sampled liquid biopsies to assess potential RNA biomarkers with a possible association to the development of resistance to osimertinib in the clinic. While there have been a number of proposed resistance mechanisms to osimertinib, some reports rely solely on analysis of cell-free DNA (31-35), which may not provide the full biological picture of how a tumor can circumvent osimertinib therapy. Our investigation focused on comparing exosomal RNA at treatment baseline to exosomal RNA harvested at disease progression in twenty patients receiving osimertinib as second-line treatment. All patients enrolled in this study had prior treatment with a first-generation EGFR TKI and tested positive for the T790M mutation before starting osimertinib treatment. Therefore, it is possible that the T790M mutation could have arisen as a consequence of prior TKI-treatment and that patients without the T790M mutation would have a distinct RNA landscape in the baseline setting. Moreover, the methodology used in this study (22) is likely to extract RNAs originating from various extracellular vesicles below 200 nm in diameter, and not solely RNAs derived from exosomes. There are so far no studies investigating the exosomal transcriptome from plasma, following osimertinib resistance. Analysis of our non-sequencing transcriptomics platform revealed presence of all major RNA categories, which provided a robust base for direct interpretation of results in DEG analysis and for enrichment analyses. The ability to derive biologically sensible results, validated with external experimental and model datasets, provided a proof of potential usability of blood plasma sampling. When comparing the full cohort of baseline versus progression samples, we were intrigued by the relatively low amount of differentially expressed transcripts. We observed significant differential expression of protein coding mRNA transcripts MKNK1, ABCA2, PRODH2, RASA1, IL17RA, ZNF17, LIN9, RGS18, APOBEC3D, GTPBP2, WDR89, ODC1, ERICH6 and GSG2. However, despite the abundant RNA coverage, there was a systematic lack of previously reported aberrations, including MET, HER2 and PIK3CA, which might be explained by tumor heterogeneity. This created the incentive of analyzing tumor progression at the pathway level. Interestingly, we observed changes in ERBB, PI3K and ECM (syndecan and glypican) pathways when using the overall NEA approach, while the individual analysis proved to be highly informative on presence and involvement of immune cell transcriptomes. Both syndecans and glypicans are cell surface bound heparan sulfate proteoglycans (HSPGs). HSPGs are implicated in regulation of cell proliferation, migration, and differentiation and are therefore considered key players in cancer initiation and progression. However, there is very limited data on the potential role of HSPG in resistance to EGFR TKIs. Nishio et al. reported that high concentrations of heparan sulfate in serum were strongly related to poor treatment outcome of EGFR TKIs (36). Heparin-binding epidermal growth factor-like growth factor (HB-EGF) is a ligand for EGFR and has the ability to bind HSPGs, which facilitates EGFR activation. Another study demonstrated that the expression of HB-EGF was clearly increased in lung cancer cell lines with EGFR mutation compared to those without EGFR mutation and implicated HB-EGF as a target in resistance to EGFR TKIs due to EGFR downstream aberrations (37). The role of overexpression of HSPGs in relation to HB-EGF-mediated EGFR activation in TKI resistance remains to be shown. Our study suggests a possible usability of HSPGs as biomarkers in patients with disease progression on osimertinib treatment.
The individual NEA exposed potential roles of immunity-related FGS in the course of progression. This result is in line with a study by Isomoto et al. (38), showing that the densities of CD8+ and FOXP3+ lymphocytes as well as the expression of CD73 in tumor cells increased after the development of EGFR TKI resistance, suggestive of possible immunosuppressive effects of regulatory T (Treg) cells and CD73 expression, the latter via induction of adenosine that interacts with the A2A receptor. Notably, most of our findings (either DEGs or differentially activated pathways and gene sets) were significant correlates, i.e., differential values were observed in subsets of samples, which emphasized the complexity of the alterations and the necessity to consider subset and multivariate approaches when developing potential biomarkers.
Profiling liquid biopsies at the RNA level instead of at the DNA level raises some concerns, which need careful consideration. Exosomes are shed into the bloodstream by virtually all cells in the body (39). Therefore, the profiled RNA-landscape will be a mixture of tumor-derived exosomes and exosomes of various sources of non-malignant cells. Although longitudinal profiling will likely reduce the influence from non-malignant cell derived exosomes, the ultimate impact of such influence may look very different from patient to patient, and hence contribute to the observed heterogeneity. On the other hand, the heterogeneity and robustness of mRNA detection in this study was comparable or better than in the public RNA-seq based datasets. In order to truly decipher the impact on, and possibly contribution from, the RNA-landscape in acquired resistance to osimertinib, it may be crucial to extend the analysis to include both liquid biopsies and solid biopsies, as well as to include analysis of DNA and circulating DNA. Such future extension of the analysis, where the RNA-landscape of solid biopsies is compared with the RNA-landscape of liquid biopsies at baseline and progression of disease should determine the ultimate usability of liquid biopsy RNA-profiles in resistance to targeted therapies. Finally, the transcripts and profiles unveiled in this study do not present a causal relationship to osimertinib resistance. Future studies, in vitro and in vivo, are warranted to validate whether any of the uncovered differentially expressed transcripts play a mechanistic role in circumventing sensitivity to the EGFR TKI osimertinib.
Conclusions
In conclusion, we demonstrate a potential usability of conducting exosomal RNA profiling from plasma to define patients with resistance signatures to the third-generation EGFR TKI osimertinib. Our study highlights the abundance of RNAs in blood plasma, relevance of network-based analysis, and the involvement of multiple RNA species in dictating the transcriptional landscape of osimertinib-refractory NSCLC patients, including mechanisms related to ERBB, ECM and immune-related pathways.
Acknowledgments
We are grateful to the staff at the BEA core facility at Karolinska Institutet for assistance with generation of transcriptomics raw data using the Clariom D platform.
Funding: This study was supported with funding from the Swedish Research Council #2019-01711 (to PH), the Stockholm Cancer Society (to PH, SE), the Swedish Cancer Society (to SE) and the Sjöberg Foundation (to SE).
Footnote
Reporting Checklist: The authors have completed the MDAR reporting checklist. Available at https://tlcr.amegroups.com/article/view/10.21037/tlcr-22-236/rc
Data Sharing Statement: Available at https://tlcr.amegroups.com/article/view/10.21037/tlcr-22-236/dss
Conflicts of Interest: All authors have completed the ICMJE uniform disclosure form (available at https://tlcr.amegroups.com/article/view/10.21037/tlcr-22-236/coif). The authors have no conflicts of interest to declare.
Ethical Statement: The authors are accountable for all aspects of the work in ensuring that questions related to the accuracy or integrity of any part of the work are appropriately investigated and resolved. The study was conducted in accordance with the Declaration of Helsinki (as revised in 2013) and the ICH-Guidelines of Good Clinical Practice and according to regulatory requirements. This study received ethical approval by the institutional review board at Karolinska University Hospital (registration No. 2016/944-31/1) and Oslo North Regional Ethics Board (No. 2015/181). Additional approvals by Stockholm Medical Biobank (No. Bbk-01605) were received. All patients provided written informed consent.
Open Access Statement: This is an Open Access article distributed in accordance with the Creative Commons Attribution-NonCommercial-NoDerivs 4.0 International License (CC BY-NC-ND 4.0), which permits the non-commercial replication and distribution of the article with the strict proviso that no changes or edits are made and the original work is properly cited (including links to both the formal publication through the relevant DOI and the license). See: https://creativecommons.org/licenses/by-nc-nd/4.0/.
References
- Bray F, Ferlay J, Soerjomataram I, et al. Global cancer statistics 2018: GLOBOCAN estimates of incidence and mortality worldwide for 36 cancers in 185 countries. CA Cancer J Clin 2018;68:394-424. [Crossref] [PubMed]
- Paez JG, Jänne PA, Lee JC, et al. EGFR mutations in lung cancer: correlation with clinical response to gefitinib therapy. Science 2004;304:1497-500. [Crossref] [PubMed]
- Sharma SV, Bell DW, Settleman J, et al. Epidermal growth factor receptor mutations in lung cancer. Nat Rev Cancer 2007;7:169-81. [Crossref] [PubMed]
- Jackman DM, Yeap BY, Sequist LV, et al. Exon 19 deletion mutations of epidermal growth factor receptor are associated with prolonged survival in non-small cell lung cancer patients treated with gefitinib or erlotinib. Clin Cancer Res 2006;12:3908-14. [Crossref] [PubMed]
- Riely GJ, Pao W, Pham D, et al. Clinical course of patients with non-small cell lung cancer and epidermal growth factor receptor exon 19 and exon 21 mutations treated with gefitinib or erlotinib. Clin Cancer Res 2006;12:839-44. [Crossref] [PubMed]
- Carey KD, Garton AJ, Romero MS, et al. Kinetic analysis of epidermal growth factor receptor somatic mutant proteins shows increased sensitivity to the epidermal growth factor receptor tyrosine kinase inhibitor, erlotinib. Cancer Res 2006;66:8163-71. [Crossref] [PubMed]
- Pao W, Miller VA, Politi KA, et al. Acquired resistance of lung adenocarcinomas to gefitinib or erlotinib is associated with a second mutation in the EGFR kinase domain. PLoS Med 2005;2:e73. [Crossref] [PubMed]
- Yun CH, Mengwasser KE, Toms AV, et al. The T790M mutation in EGFR kinase causes drug resistance by increasing the affinity for ATP. Proc Natl Acad Sci U S A 2008;105:2070-5. [Crossref] [PubMed]
- Cross DA, Ashton SE, Ghiorghiu S, et al. AZD9291, an irreversible EGFR TKI, overcomes T790M-mediated resistance to EGFR inhibitors in lung cancer. Cancer Discov 2014;4:1046-61. [Crossref] [PubMed]
- Jänne PA, Yang JC, Kim DW, et al. AZD9291 in EGFR inhibitor-resistant non-small-cell lung cancer. N Engl J Med 2015;372:1689-99. [Crossref] [PubMed]
- Yang JC, Ahn MJ, Kim DW, et al. Osimertinib in Pretreated T790M-Positive Advanced Non-Small-Cell Lung Cancer: AURA Study Phase II Extension Component. J Clin Oncol 2017;35:1288-96. [Crossref] [PubMed]
- Goss G, Tsai CM, Shepherd FA, et al. Osimertinib for pretreated EGFR Thr790Met-positive advanced non-small-cell lung cancer (AURA2): a multicentre, open-label, single-arm, phase 2 study. Lancet Oncol 2016;17:1643-52. [Crossref] [PubMed]
- Mok TS, Wu Y-L, Ahn M-J, et al. Osimertinib or Platinum-Pemetrexed in EGFR T790M-Positive Lung Cancer. N Engl J Med 2017;376:629-40. [Crossref] [PubMed]
- Ramalingam SS, Vansteenkiste J, Planchard D, et al. Overall Survival with Osimertinib in Untreated, EGFR-Mutated Advanced NSCLC. N Engl J Med 2020;382:41-50. [Crossref] [PubMed]
- Papadimitrakopoulou VA, Wu YL, Han JY, et al. Analysis of resistance mechanisms to osimertinib in patients with EGFR T790M advanced NSCLC from the AURA3 study. Ann Oncol 2018;29:741. [Crossref]
- Leonetti A, Sharma S, Minari R, et al. Resistance mechanisms to osimertinib in EGFR-mutated non-small cell lung cancer. Br J Cancer 2019;121:725-37. [Crossref] [PubMed]
- Mehlman C, Cadranel J, Rousseau-Bussac G, et al. Resistance mechanisms to osimertinib in EGFR-mutated advanced non-small-cell lung cancer: A multicentric retrospective French study. Lung Cancer 2019;137:149-56. [Crossref] [PubMed]
- Alexeyenko A, Lee W, Pernemalm M, et al. Network enrichment analysis: extension of gene-set enrichment analysis to gene networks. BMC Bioinformatics 2012;13:226. [Crossref] [PubMed]
- Franco M, Jeggari A, Peuget S, et al. Prediction of response to anti-cancer drugs becomes robust via network integration of molecular data. Sci Rep 2019;9:2379. [Crossref] [PubMed]
- Eide IJZ, Helland Å, Ekman S, et al. Osimertinib in T790M-positive and -negative patients with EGFR-mutated advanced non-small cell lung cancer (the TREM-study). Lung Cancer 2020;143:27-35. [Crossref] [PubMed]
- McGowan M, Kleinberg L, Halvorsen AR, et al. NSCLC depend upon YAP expression and nuclear localization after acquiring resistance to EGFR inhibitors. Genes Cancer 2017;8:497-504. [Crossref] [PubMed]
- Enderle D, Spiel A, Coticchia CM, et al. Characterization of RNA from Exosomes and Other Extracellular Vesicles Isolated by a Novel Spin Column-Based Method. PLoS One 2015;10:e0136133. [Crossref] [PubMed]
- Cerami EG, Gross BE, Demir E, et al. Pathway Commons, a web resource for biological pathway data. Nucleic Acids Res 2011;39:D685-90. [Crossref] [PubMed]
- Jeggari A, Alekseenko Z, Petrov I, et al. EviNet: a web platform for network enrichment analysis with flexible definition of gene sets. Nucleic Acids Res 2018;46:W163-70. [Crossref] [PubMed]
- Barretina J, Caponigro G, Stransky N, et al. The Cancer Cell Line Encyclopedia enables predictive modelling of anticancer drug sensitivity. Nature 2012;483:603-7. [Crossref] [PubMed]
- Cancer Genome Atlas Research Network. Comprehensive genomic characterization of squamous cell lung cancers. Nature 2012;489:519-25. [Crossref] [PubMed]
- Cancer Genome Atlas Research Network. Comprehensive molecular profiling of lung adenocarcinoma. Nature 2014;511:543-50. [Crossref] [PubMed]
- Liu SY, Sanchez DJ, Aliyari R, et al. Systematic identification of type I and type II interferon-induced antiviral factors. Proc Natl Acad Sci U S A 2012;109:4239-44. [Crossref] [PubMed]
- Rosanò L, Spinella F, Bagnato A. Endothelin 1 in cancer: biological implications and therapeutic opportunities. Nat Rev Cancer 2013;13:637-51. [Crossref] [PubMed]
- Petrov I, Alexeyenko A. EviCor: Interactive Web Platform for Exploration of Molecular Features and Response to Anti-cancer Drugs. J Mol Biol 2022;434:167528. [Crossref] [PubMed]
- Piotrowska Z, Isozaki H, Lennerz JK, et al. Landscape of Acquired Resistance to Osimertinib in EGFR-Mutant NSCLC and Clinical Validation of Combined EGFR and RET Inhibition with Osimertinib and BLU-667 for Acquired RET Fusion. Cancer Discov 2018;8:1529-39. [Crossref] [PubMed]
- Shi P, Oh YT, Deng L, et al. Overcoming Acquired Resistance to AZD9291, A Third-Generation EGFR Inhibitor, through Modulation of MEK/ERK-Dependent Bim and Mcl-1 Degradation. Clin Cancer Res 2017;23:6567-79. [Crossref] [PubMed]
- Yang Z, Yang N, Ou Q, et al. Investigating Novel Resistance Mechanisms to Third-Generation EGFR Tyrosine Kinase Inhibitor Osimertinib in Non-Small Cell Lung Cancer Patients. Clin Cancer Res 2018;24:3097-107. [Crossref] [PubMed]
- Le X, Puri S, Negrao MV, et al. Landscape of EGFR-Dependent and -Independent Resistance Mechanisms to Osimertinib and Continuation Therapy Beyond Progression in EGFR-Mutant NSCLC. Clin Cancer Res 2018;24:6195-203. [Crossref] [PubMed]
- Oxnard GR, Hu Y, Mileham KF, et al. Assessment of Resistance Mechanisms and Clinical Implications in Patients With EGFR T790M-Positive Lung Cancer and Acquired Resistance to Osimertinib. JAMA Oncol 2018;4:1527-34. [Crossref] [PubMed]
- Nishio M, Yamanaka T, Matsumoto K, et al. Serum heparan sulfate concentration is correlated with the failure of epidermal growth factor receptor tyrosine kinase inhibitor treatment in patients with lung adenocarcinoma. J Thorac Oncol 2011;6:1889-94. [Crossref] [PubMed]
- Yotsumoto F, Fukagawa S, Miyata K, et al. HB-EGF Is a Promising Therapeutic Target for Lung Cancer with Secondary Mutation of EGFRT790M. Anticancer Res 2017;37:3825-31. [PubMed]
- Isomoto K, Haratani K, Hayashi H, et al. Impact of EGFR-TKI Treatment on the Tumor Immune Microenvironment in EGFR Mutation-Positive Non-Small Cell Lung Cancer. Clin Cancer Res 2020;26:2037-46. [Crossref] [PubMed]
- Cui S, Cheng Z, Qin W, et al. Exosomes as a liquid biopsy for lung cancer. Lung Cancer 2018;116:46-54. [Crossref] [PubMed]