A novel approach for the non-invasive diagnosis of pulmonary nodules using low-depth whole-genome sequencing of cell-free DNA
Introduction
The introduction of low-dose computed tomography (CT) for lung cancer screening, as well as CT imaging for other indications, has significantly increased the detection rate of pulmonary nodules in recent years, with approximately 1.5 million cases estimated per year in the United States (1,2). Despite reducing lung cancer mortality through the detection of pulmonary nodules, low-dose CT has an extremely high false-positive rate (96.4%), which leads to unnecessary workups of benign nodules (3-5). The detective methods to make a difference between benign and malignant pulmonary nodules mainly include CT/positron emission tomography (PET)-CT, long-term follow-up CT scans or even invasive procedures like bronchoscopy, transthoracic fine-needle aspiration biopsy, and surgery. Size and growth rate of pulmonary nodules are the main indicators to assess probability of nodule malignancy. Usually, lung nodules measured more than 30 mm in diameter on CT imaging are more likely to be cancerous than smaller nodules. It is still challenging to evaluate the malignancy risk for lung nodules with diameters of less than 30 mm only depending on CT scan. Long-term follow-up CT scans and invasive procedures result in a significant burden as most small lung nodules are benign (6-9). Thus, non-invasive methods are urgently required to aid in the differentiation of benign from malignant pulmonary nodules.
Plasma cell-free DNA (cfDNA) are short DNA fragments of double-stranded DNA ranging around a modal size of ~166 bp circulating in the blood that are released by apoptotic or necrotic cells but also by active secretion of non-damaged cells. The half-life of cfDNA is relatively short with 16 min to 2.5 h in the blood. The level of cfDNA is much lower in plasma from healthy individuals than cancer patients. Plasma cfDNA have been successfully utilized in the detection and diagnosis of various cancer types, including hematological malignancies, breast cancer, osteosarcoma, and ovarian cancer (10,11). Circulating tumor DNA (ctDNA) accounts for a variable proportion of the total cfDNA (12-14). CtDNA is believed to contain the same genetic aberrations as the corresponding tumor. The potential utility of ctDNA for early diagnosis, detection of minimal residual disease and molecular genotyping has been explored in lung cancer (15,16). However, it is difficult to reliably detect mutated ctDNA in early-stage non-small cell lung cancer (NSCLC) due to the low abundance of ctDNA in cfDNA, which is estimated to be less than 0.01% in 50% of patients with stage I NSCLC (17,18). Mutation-based cfDNA tests, which included the whole-exome sequencing (WES) and targeted multiple gene sequencing (Panel-seq) in early-stage NSCLC require ultra-deep coverage that are costly and technically challenging due to sequencing artifacts, vast mutational heterogeneity between patients, and the frequent occurrence of non-malignant somatic mutations in cfDNA, e.g., those that drive clonal hematopoiesis (12,19-22). Therefore, new non-invasive approaches other than mutation-based cfDNA tests should be explored.
Whole-genome sequencing (WGS) of cfDNA can identify chromosomal abnormalities such as copy number alterations (CNAs) in cancer (23,24). CNAs usually range from 1 Mb to 100+ Mb, spanning large genomic regions. Some studies have reported that, compared with point mutations, the detection of cancer-derived CNA events in cfDNA is likely to be a superior approach for ctDNA-based early cancer detection because ctDNA CNAs contribute a much larger number of ctDNA fragments to the total cfDNA per CNA event (24,25). CNAs have been previously identified to be specific biomarkers of lung malignancy (26,27). CfDNA fragmentomics, other than CNA events in cfDNA, also provides a way for non-invasive detection of lung cancer. It was reported that genome-wide cfDNA fragmentation analysis such as DNA evaluation of fragments for early interception (DELFI) approach can discriminate lung cancer patients from non-cancer individuals (28-30). Non-invasive prenatal testing (NIPT) utilizes low genomic depth (<1×) to detect large chromosomal aberrations. With the widespread use of NIPT, some women have been found to have CNAs in their plasma originating from undiagnosed maternal cancer (31,32). These findings highlight the potential of detecting cfDNA CNAs in early-stage cancer.
The feasibility of discriminating between benign and malignant pulmonary nodules according to the WGS-derived characteristics of cfDNA has not been adequately investigated (33). Compared to WES of plasma cfDNA, low-depth WGS (LD-WGS) was more reliable, more efficient, less expensive for the detection of CNAs. In this study, we developed a novel genome-wide approach to distinguish benign from malignant pulmonary nodules using LD-WGS of cfDNA. This method has the potential to provide a cost-effective, non-invasive diagnostic approach for the accurate diagnosis of pulmonary nodules. We present the following article in accordance with the TRIPOD reporting checklist (available at https://tlcr.amegroups.com/article/view/10.21037/tlcr-22-647/rc).
Methods
Study population
Patients undergoing treatment for suspected lung cancer at Tianjin Medical University Cancer Institute and Hospital between June 2015 and August 2017 were considered for this study. All of the following inclusion criteria had to be fulfilled: (I) lung nodules were no more than 30 mm in diameter as determined by CT scan; (II) lung nodules were evaluated as malignant by radiologists and physicians, were undiagnosed, or were evaluated as benign but the patients preferred surgery; and (III) patients underwent surgical excision, bronchoscopic biopsy, or transthoracic biopsy. The exclusion criteria were as follows: (I) patients with other malignant tumors; (II) those with no pathological diagnosis; and (III) subtypes of lung malignancy were small cell lung cancer or pulmonary large cell neuroendocrine carcinoma. A total of 420 cases, including 131 patients with benign pulmonary nodules and 289 patients with NSCLC, were analyzed.
The initial diagnoses were obtained by CT. Plasma samples were collected 1–5 days before treatment and within 1 month after nodule detection. Pathological diagnosis was made on the basis of biopsy or surgical samples. The staging was according to the 8th Edition of the International Staging of Thoracic Malignancies and the histological subtype was according to the 2015 World Health Organization (WHO) classification of lung tumors (34,35). We collected the following clinicopathological data: age, sex, smoking status, histological subtype, stage, and CT results reported by the radiologist. The study was conducted in accordance with the Declaration of Helsinki (as revised in 2013). This study was approved by the ethical committee of Tianjin Medical University Cancer Institute and Hospital (approval Nos. bc2016014, bc2018009, and bc2019091), and all participants provided written informed consent.
External independent dataset from The Chinese University of Hong Kong (CUHK) including 38 healthy controls and 10 lung cancer patients was used for validation (36).
Sample preparation, library construction, and sequencing
A 5-mL sample of whole blood was collected from each patient in an ethylenediaminetetraacetic acid (EDTA) tube and processed immediately. Plasma and cellular components were separated by centrifugation at 1,600 ×g for 10 min at 4 ℃. Plasma was further centrifuged for 10 min at 16,000 ×g at 4 ℃ to remove any remaining cellular debris and then stored at −80 ℃. CfDNA was extracted using a MagPure Circulating DNA KingFisher (KF) Kit (Magen, Guangzhou, China). The concentration of the extracted cfDNA was quantified by a Qubit 3.0 fluorometer (Life Technologies, Paisley, UK), and the size distribution was detected using an Agilent DNA High Sensitivity Kit on an Agilent 2100 Bioanalyzer (Agilent Technologies, Santa Clara, CA, USA). The total cfDNA of each plasma sample was input for library preparation using the MGIEasy cfDNA Library Prep Set (MGI-Tech, Shenzhen, China).
CfDNA isolation and WGS library construction were both performed using a MGISP-960 High-Throughput Automated Sample Preparation System (MGI-Tech) according to the manufacturer’s protocol. Briefly, purified cfDNA was subjected to end repair, A-tailing, ligation modules, polymerase chain reaction (PCR) amplification, and single-strand circularization. All single-strand circular DNA libraries were sequenced on the MGISEQ-2000 platform (MGI-Tech) with paired-end reads to generate approximately 1.5–3 Gb of whole-genome data for each sample with a coverage of 37% at 1× depth (MGI-Tech). The quantity of cfDNA is shown in (available online: https://cdn.amegroups.cn/static/public/tlcr-22-647-1.xlsx).
Calculation of weighted reads distribution difference (WRDD)
Following WGS, the sequencing reads were aligned to the hg38 reference genome. All autosomes were joined together and divided into a series of fixed-length windows (30 kb/window) along the DNA. We then counted the read number of each sample within each window of DNA. Differences in the read counts along a series of regions comprised the read distribution pattern, which reflected the bias of the read distribution related to the sample type.
For each sample, we combined the varying number of read windows to create a region and observed the features of this region. To identify the critical distribution characteristics of the reads across the genome, we defined the WRDD, which emphasizes CNAs in regions of the whole genome and provides additional genomic information. If a region consisted of n windows, the read number matrix x of a sample set with m samples within the region could be described as:
where xi,j is the read number of sample i in window j. The weight value w of these n windows could be calculated as:
where the function variance calculates the variance value of the read numbers of m samples in each window. The average read number of each window was used as the benchmark. The read numbers of benchmark b of these n windows could be calculated as:
where the function mean calculates the mean value of the read number of m samples in each window. The weighted sum value Si of sample i could be calculated as:
where the function scale calculates the scaled values of a given number list. Read numbers were scaled by sample. If the sum of the weighted differences in the read number of benign values in a given region had a higher variance than that of the malignant samples, that region was referred to as a benign variable (BV) region; otherwise, it was referred as a malignant variable (MV) region. The WRDD value was calculated differently for BV regions than for MV regions, as follows:
Calculation of t-values
For a given region, a t-value was calculated to measure the ability of the region to differentiate sample types. For a specific region, the t-value was calculated according to the WRDD values of the two types of samples using the following formula:
where and are the means of the two WRDD value lists that originate from the two sample sets, while n1 and n2 are the element numbers of the two sample sets, and S1 and S2 are their standard deviation (SD) values, respectively. A higher t-value was indicative of a better ability of a particular region to differentiate between the sample types.
Design of the genetic algorithm
To explore regions with high t-values, we developed a modified genetic algorithm. First, a simple strategy was adopted to generate a series of original regions. The genetic algorithm was then used to perform continuous combination and separation operations on these regions to obtain new regions with higher t-values.
- Generation of the original regions. A continuous series of n windows at location i was combined with another series of n windows located 2jn windows downstream of the location to obtain an original region consisting of 2n windows. The original region that began at the window i was defined as:
- where n is the number of a series of continuous windows (n=5) and N is the total number of windows (N=95,833). A certain distance between the two series of windows was specified to ensure that the regions possessed discriminatory abilities across long distances.
- Combination and separation of regions. The genetic algorithm involved the combination of two parental regions for information exchange and the generation of offspring regions. All original regions were put in a regional pool for the random selection of parental regions to generate offspring regions. The probability that region i was selected as one of the parental regions was as follows:
- where N is the total number of all existing regions and ti is the t-value of the i-th region. Given that region x had been selected as the first parental region, the probability that another region i was selected as the second parental region was as follows:
- where N is the total number of all existing regions, mi is the mean of the positions of all windows contained by the region i, and ti is the t-value of the i-th region. The inclusion of the term |mx−mi| was set for the preferential selection of regions with a shorter inter-regional distance as the parental regions. Following the selection of both parental regions, 20% of the unified windows included in the two parental regions were randomly selected by sampling with the replacement and deleting it to form the offspring region. The obtained offspring region was then added to the regional pool for the next round of selection. The parental regions were not deleted during the entire process. The offspring region F generated by two parental regions P1 and P2 and probability p were defined as:
- where S(p,s) is the subset of p% elements sampled randomly from set s with replacement (in this work, p=20%). The offspring generation was analyzed 300,000 times to generate the same number of regions, which balanced the costs and potential effects.
- where N is the total number of all existing regions and ti is the t-value of the i-th region. Given that region x had been selected as the first parental region, the probability that another region i was selected as the second parental region was as follows:
Benign and malignant diagnostic (BEMAD) model construction and prediction
BV and MV regions with the highest t-values were selected to construct the BEMAD model. When using the model with N regions to determine a sample’s type, the sum of the WRDDs of the sample in the N responding regions was calculated and used as the score of the sample for prediction.
To eliminate the influence of the imbalance in t-values between regions of different types, we separately calculated the sample scores for the MV and BV regions. The sums of the scores in BV and MV regions were called the BV and MV region scores, respectively. We then scaled the BV and MV region scores of a sample and combined them to obtain its final score:
where scorebenign is the BV region score of a sample, scoremalignant is the MV region score, is the mean BV score of the corresponding training cohort, is its SD value, is the mean of the MV region score of the corresponding training cohort, and is its SD value. We determined whether a sample was benign or malignant based on its score.
The process of developing the BEMAD model according to the WRDD was as follows (Figure 1):
- A region was generated by combining windows in the genome (Figure 1A). The whole genome was divided into a series of 30-kilo-base pair fixed-length and non-overlapping windows, which resulted in 95,833 windows including trans-chromosomal windows. The average read number in a series of benign and malignant samples was calculated as the benchmark for each window. Regions were generated by combining windows that were selected with a modified genetic algorithm.
- The WRDD value of a sample in a region was calculated by converting the sum of the weighted differences of read numbers between the sample and the benchmark of the windows contained within a region (Figure 1B). To eliminate differences in the read number of the sample and its benchmark in that region, we scaled the read numbers of the sample in all windows within a region to ensure that the total read numbers were the same at the regional level. The read numbers of the benchmarks were also scaled in the same way. Given that the sum values of benign samples were further away from 0 (above or under) in the BV regions, while those of malignant samples were closer to 0, we converted the sum values to their absolute values to clearly separate them, which resulted in higher absolute values for the benign samples. The sum values in MV regions were converted using the same method, resulting in higher absolute values for the malignant samples. To ensure that the absolute values of the malignant samples were higher than those of the benign samples in both the BV and MV regions, we further converted the absolute values in the BV regions to their inverse values. To summarize, the WRDD of BV regions = −|sum|, while the WRDD for MV regions = |sum|. A t-value was used to evaluate the discrimination capacity of the regions in the benign and malignant groups. The two WRDD value sets obtained from the two sample groups were then used to calculate the t-value of a region; a higher t-value was indicative of a greater ability of a region to distinguish the respective sample group.
- Regions with the top x of t-values were selected for the model construction (Figure 1C). The sum of the WRDDs within the regions generated the score of a sample, which was used to predict whether the sample was benign or malignant.
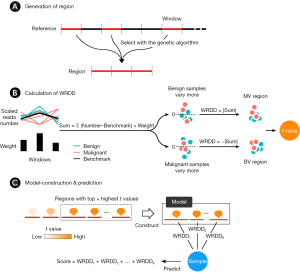
Statistical analyses
All statistical analyses were performed using R version 3.6.3. The area under the receiver operating characteristic (ROC) curve (AUC) values, 95% confidence intervals (CIs), cut-off value related to the maximum AUC, and the corresponding specificity and sensitivity from the model output were obtained with the pROC (v.1.16.2) R package (37). The Mann-Whitney U test was performed to determine the differences in score distributions between two groups. The Student’s t-test was used to measure the differences in clinicopathological factors with continuous variables (e.g., age) between patients with benign and malignant pulmonary nodules, and Fisher’s exact test was performed to measure the differences in clinicopathological features with discrete variables (e.g., sex) using the built-in functions, “t.test” and “fisher.test”, respectively, of the current version of R. The counting of read numbers was performed using “readcounter”, a tool in HMMcopy (Bioconductor R package version 1.32.0.). The investigators were not blinded to the groups during experiments and outcome assessments. A P value of <0.05 indicated statistical significance.
Results
Patients’ features
We retrospectively analyzed plasma samples from 420 individuals with lung nodules of ≤30 mm diameter according to CT images (Table 1). Of these, 289 (69%) were NSCLC patients [adenocarcinoma, n=240 (83%); and squamous cell carcinoma, n=49 (17%)], which were referred to as malignant samples. The remaining 131 (31%) patients had benign pulmonary nodules [cyst, n=4 (3%); fibrosis, n=7 (5%); granuloma, n=24 (18%); hamartoma, n=34 (26%); inflammation, n=35 (27%); tuberculosis, n=17 (13%); other cases, n=10 (8%)], which were referred to as benign samples. Of the 289 NSCLC patients, 247 (85%) were stage I (229 of whom were stage IA and 18 were stage IB).
Table 1
Characteristics | Total | Training cohort | Validation cohort | ||||||||
---|---|---|---|---|---|---|---|---|---|---|---|
Benign | Malignant | P value | Benign | Malignant | P value | Benign | Malignant | P value | |||
Count, n [%] | 131 [31] | 289 [69] | – | 101 [28] | 259 [72] | – | 30 [50] | 30 [50] | – | ||
Age, mean ± SD | 53±10 | 59±8.4 | 7.70E-07 | 54±10 | 59±8.3 | 2.40E-05 | 52±12 | 57±9.3 | 0.037 | ||
Sex, n [%] | 0.074 | 0.24 | 0.29 | ||||||||
Female | 55 [42] | 149 [52] | 45 [45] | 134 [52] | 10 [33] | 15 [50] | |||||
Male | 76 [58] | 140 [48] | 56 [55] | 125 [48] | 20 [67] | 15 [50] | |||||
Smoking, n [%] | 0.83 | 0.81 | 1 | ||||||||
No | 67 [51] | 153 [53] | 51 [50] | 135 [52] | 16 [53] | 17 [57] | |||||
Yes | 64 [49] | 136 [47] | 50 [50] | 124 [48] | 14 [47] | 13 [43] | |||||
Smoking-years (for smokers only), mean ± SD | 29±12 | 32±13 | 0.088 | 29±12 | 32±13 | 0.14 | 29±13 | 34±13 | 0.35 | ||
Pack-years (for smokers only), mean ± SD | 26±17 | 22±14 | 0.18 | 25±16 | 22±14 | 0.26 | 28±23 | 24±13 | 0.59 | ||
Nodule size (mm), mean ± SD | 19±8.1 | 21±7.4 | 0.042 | 19±8.2 | 21±7.5 | 0.029 | 21±8 | 21±7.1 | 0.72 | ||
Pathological stage, n [%] | – | – | – | ||||||||
I | – | 247 [85] | – | 224 [86] | – | 23 [77] | |||||
II | – | 15 [5] | – | 12 [5] | – | 3 [10] | |||||
III | – | 27 [9] | – | 23 [9] | – | 4 [13] | |||||
Subtype, n [%] | – | – | – | ||||||||
AD | – | 240 [83] | – | 216 [83] | – | 24 [80] | |||||
SC | – | 49 [17] | – | 43 [17] | – | 6 [20] | |||||
Cyst | 4 [3] | – | 3 [3] | – | 1 [3] | – | |||||
Fibrosis | 7 [5] | – | 4 [4] | – | 3 [10] | – | |||||
Granuloma | 24 [18] | – | 20 [20] | – | 4 [13] | – | |||||
Hamartoma | 34 [26] | – | 29 [29] | – | 5 [17] | – | |||||
Inflammation | 35 [27] | – | 29 [29] | – | 6 [20] | – | |||||
Tuberculosis | 17 [13] | – | 11 [11] | – | 6 [20] | – | |||||
Others | 10 [8] | – | 5 [5] | – | 5 [17] | – | |||||
Procedure type, n [%] | – | – | – | ||||||||
Surgical excision | 119 [91] | 284 [98] | – | – | – | – | |||||
Bronchoscopic biopsy | 0 [0] | 3 [1] | – | – | – | – | |||||
transthoracic biopsy | 12 [9] | 2 [1] | – | – | – | – |
When comparing age/smoking-years/pack-years/nodule size, Student’s t-test was used to calculate the P value; when comparing sex/smoking, Fisher’s exact test was used to calculate the P value. SD, standard deviation; AD, adenocarcinoma; SC, squamous cell carcinoma.
The malignant and benign samples were matched for sex, smoking status, and pack-years of smoking (P>0.05). NSCLC patients were older compared to individuals with benign pulmonary nodules (P=7.70e–7; Student’s t-test). The mean nodule size of the malignant samples was larger than that of the benign samples (P=0.042; Student’s t-test). The samples were randomly divided into a training cohort (n=360, including 101 benign samples and 259 malignant samples) and an independent validation cohort (n=60, including 30 benign samples and 30 malignant samples) at a ratio of 6:1. For the independent validation cohort, we limited the ratio of benign to malignant samples to 1:1. A workflow of the study is shown in Figure S1.
Development of the BEMAD model
We designed a procedure with 10-fold cross-validation in the training cohort (Figure 2). Higher t-values were identified in the BV regions compared with the MV regions. Approximately 99% of regions with the top 1,000 t-values were BV regions. Owing to the large differences in t-values between the BV and MV regions, the two types of regions were analyzed separately. BV regions with the 10 highest t-values were chosen first for the model construction (Figure 2A, available online: https://cdn.amegroups.cn/static/public/tlcr-22-647-2.xlsx). The mean AUC in 10 test sets of the BV region-based model was 0.8 (95% CI: 0.73–0.86). To explore the distribution of sample scores in different histological subtypes, we compared them in 10 test sets. We first normalized the scores from the same dataset to eliminate the differences in scores among the different test sets. We found that the average score of the malignant samples was higher than that of the benign samples (P=2.6e–20; Mann-Whitney U test; Figure 2A). There was no difference between the average scores of adenocarcinomas and squamous cell carcinomas.
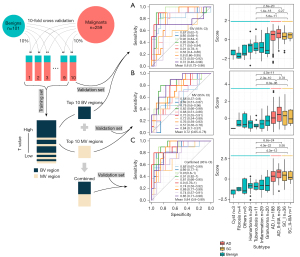
Subsequently, MV regions with the 10 highest t-values were selected to run the same procedure as the construction of the BV-based model (Figure 2B, available online: https://cdn.amegroups.cn/static/public/tlcr-22-647-2.xlsx). The MV region-based model obtained a mean AUC value of 0.72 (95% CI: 0.65–0.78). The score distributions also revealed that malignant samples had a higher average score (P=4.0e–11; Mann-Whitney U test; Figure 2B), and there was no difference between the average scores of adenocarcinomas and squamous cell carcinomas.
Considering the significant differences between the performances of the two models constructed using the top 10 BV regions and the top 10 MV regions, we combined these 20 regions for the construction of the BEMAD model (Figure 2C). Since the t-values of the top 10 BV regions were higher than those of the top 10 MV regions, the direct combination of the regions would have weakened the contribution of the MV regions toward the prediction results. To resolve this issue, we separately normalized the scores of the MV and BV regions of each sample and summed the two normalized scores to obtain the final score. The average AUC increased to 0.84 (95% CI: 0.80–0.89), with a sensitivity of 80% and a specificity of 83%.
We compared our method to other cfDNA genome-wide cancer detection approaches, including ichorCNA and DELFI using the LUCAS cohort (29,38). The DELFI approach achieved an AUC of 0.94 (95% CI: 0.91–0.98). Our BEMAD model provided a similar performance, with an average AUC of 0.93 (95% CI: 0.91–0.95). Meanwhile, the ichorCNA approach obtained an AUC of 0.80 (95% CI: 0.73–0.87) (Figure S2). These results suggested that our method was comparable to the DELFI approach and superior to the ichorCNA approach.
BEMAD algorithm model validation
The BEMAD algorithm model was validated in an independent validation cohort containing 30 benign samples and 30 malignant samples. The model obtained an AUC value of 0.87 (95% CI: 0.82–0.97) (Figure 3A), and the sensitivity and specificity were 74% and 86%, respectively. The distributions of the benign and malignant sample scores were similar to those observed in the training cohort, with a higher average score obtained in the malignant samples (Figure 3B). Additionally, we used another external independent dataset from The CUHK including 38 healthy control and 10 lung cancer patients for validation (36). Our model’s performance achieved 0.84 (95% CI: 0.70–0.97) of AUC, with a sensitivity of 0.8 and a specificity of 0.82 (Figure S3).
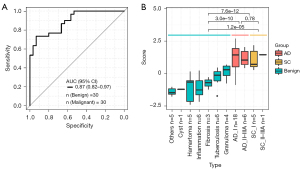
A retrospective review of the patients’ CT reports revealed that there were 47 undiagnosed lung nodules in the training cohort and nine undiagnosed lung nodules in the validation cohort. According to the pathological diagnoses, the BEMAD model correctly identified 25 of 28 (89%) benign lung nodules and 15 of 19 (79%) malignant lung nodules in the training cohort, and 7 (100%) benign and 2 (100%) malignant lung nodules in the validation cohort. This indicated that our cfDNA-based method could further stratify undiagnosed lung nodules, aiding in CT to improve the differential diagnosis of lung nodules and reducing unnecessary invasive procedures.
Influence of clinicopathological parameters on the performance of the BEMAD algorithm model
To determine whether clinicopathological factors affected the performance of the BEMAD model, we analyzed the effects of sex, age, smoking status, and nodule size on the score distribution of the samples. The differences in the score distributions of benign and malignant samples were compared according to sex (female vs. male), smoking status (smoking vs. non-smoking), age (<60 vs. ≥60 years), and nodule size (≤10 vs. >10 mm) (Figure 4A-4D). All differences in score distributions in the benign and malignant samples were non-significant (P>0.05; Mann-Whitney U test), indicating that the BEMAD model was robust and unlikely to be influenced by clinicopathological factors.
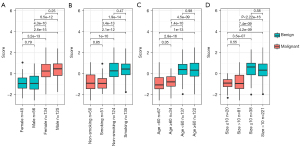
Characteristics of critical windows in the BEMAD model
To better understand the characteristics of selected genomic regions in the BEMAD model and their potential correlation with tumorigenesis, we analyzed the features of windows within each genomic region that was used for the construction of the BEMAD model. We found that some windows were critical in distinguishing malignant from benign samples, as demonstrated by the highest t-value among the windows in that region. We also observed that the critical window for 50% of the MV regions was window #57113 (chr10:38503000–38532999 in the hg38 reference genome), while the critical window for the remaining MV regions was window #92938 (chr21:10657000–10686999), and that for all BV regions was #92863 (chr21:8407000–8436999).
Interestingly, we found that many repeated motifs within these three windows could be classified into one of three types: “AATGG” for #57113, “TCCAT” for #92938, and “TCTC” for #92863. The first two repeated motifs were associated with microsatellite repeats, influencing microsatellite instability, which is a critical marker of tumorigenesis (39,40). The third repeated motif was novel and should be studied in the future.
Indeed, we found that in the region with numerous “AATGG” and “TCCAT” motifs, the differences in read numbers between benign and malignant samples were more significant compared with their upstream regions (Figure 5A,5B). However, this was not observed for the “TCTC” motif. These findings indicated that microsatellite instability is a feature of critical windows in the BEMAD model.
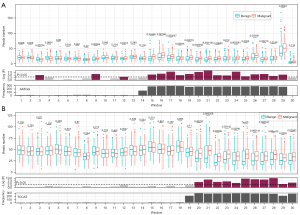
Discussion
The precise diagnosis of lung nodules is challenging. In clinical practice, 10–30% of resected lung nodules are found to be benign, which is a result of the limitations of radiological detection and the inability of CT to accurately distinguish benign from malignant nodules (41). For these patients, surgery represents overtreatment. Therefore, the development of economical non-invasive approaches that can discriminate benign from malignant pulmonary nodules is extremely valuable to aid diagnosis and reduce the number of unnecessary surgeries performed in patients with benign tumors. In this study, we developed an algorithm that utilized cfDNA LD-WGS data to differentiate between benign and malignant pulmonary nodules.
Cancer-associated CNAs have been detected in the cfDNA of patients with cancer, underscoring their potential clinical applications for the screening, early detection, and monitoring of human cancer (23,24,42-44). However, CNAs only reflect copy number variation relative to normal controls in some specific locations in the genome. Our approach introduces a new bioinformatics analysis to reflect genomic changes, namely WRDD. WRDD identifies differences in read numbers at multiple locations within a genomic region and amplifies local differences through weighting. In addition, it offers more information than CNAs, which is critical for capturing the extremely weak signals of cfDNA in early-stage NSCLC. Furthermore, WRDD allows for discontinuity within a region, which enables the elimination of unimportant windows when a region spans a large area. Although both CNAs and WRDD reflect abnormal gains or losses in the genome, WRDD serves as an extension of CNAs that can provide additional signal details and can also reflect the early characteristics of genomic instability in NSCLC.
To confirm the suitable performance of our method, we compared it to the other approaches including ichorCNA and DELFI using their cohort (29,30). Our BEMAD model achieved an average AUC of 0.93, which was almost identical to that obtained by DELFI (AUC of 0.94) and superior to that of ichorCNA (AUC of 0.80). Notably, the percentage of stage I patients in their cohort (training cohort 15/129, validation cohort 28/46) in which detection is more challenging than the later stages was far smaller than ours (training cohort 224/259, validation cohort 23/30). Therefore, the superior approach still needs to be validated with cohorts that include a large number of early-stage patients.
In recent years, cfDNA methylation patterns have demonstrated their potential utility in the early detection of several cancer types including lung cancer. However, various methods have been applied in different studies, including cfDNA methylation and bioinformatics analyses. Moreover, most of these studies focused on distinguishing cancer patients from healthy individuals, and few studies have explored the differentiation of benign from malignant lesions (33,45-49). Liang et al. attempted to discriminate between benign and malignant pulmonary nodule samples using cfDNA whole-genome bisulfite sequencing data; their model produced an AUC value of 0.839 in a training cohort containing 40 malignant and 26 benign samples, and an AUC of 0.816 in an independent validation cohort containing 39 malignant and 27 benign samples. In comparison, this study obtained AUC values of 0.84 and 0.87, respectively (33). Therefore, the performance of our WRDD-based cfDNA detection method for the discrimination of benign and malignant pulmonary nodules is comparable to that of the methylation signal-based cfDNA diagnostic method. Notably, inflammation-related benign diseases (inflammation and inflammatory granuloma), which are often erroneously classified as malignant in clinical practice, accounted for a smaller proportion of the benign samples in the study by Liang et al. (11/53=20.7%, as compared with 59/131=45.0% in the present study) (33). In addition, our LD-WGS approach is easier to implement in molecular testing laboratories. DNA methylation analysis requires a higher cfDNA amount, at 10 ng, which is 4–5 times greater than that required by our model. DNA methylation analysis also requires the genome coverage to be greater than 30×, which limits the use of this technique in clinical practice.
There were reports about the potential application of cfDNA from other liquid biopsy sources like bronchoalveolar lavage fluid (BALF) for identifying lung cancer (50,51). However, the performance of BALF cfDNA was not superior to that of plasma cfDNA to differentiate malignant from benign pulmonary nodules by analyzing the methylation and mutation profiling of cfDNA (50). It will be interesting to compare CNAs signatures from BALF cfDNA to our approach from plasma cfDNA for identifying lung cancer. Considering that obtaining BALF is invasive compared with collecting plasma, plasma cfDNA could be more suitable for early detection of lung cancer.
The differential diagnosis of pulmonary nodules of 6–20 mm is challenging for radiologists and thoracic surgeons, and usually requires long-term CT surveillance and even invasive procedures (8). In our cohort, the AUC value of pulmonary nodules of 10–20 mm was 0.83, while that of nodules ≤10 mm was 0.86 (Figure S4). The performance of the BEMAD model in differentiating benign from malignant lung nodules was not influenced by nodule size. For the undiagnosed lung nodules in our training cohort, the BEMAD model correctly identified 89% of benign nodules and 79% of malignant lung nodules. The real-world impact of this model is that approximately 50% of patients with benign lung nodules would avoid longitudinal radiographical follow-up, as recommended by the Fleischner Society guidelines (8), or invasive procedures. These results suggest that our model has the potential to be used as an adjuvant with CT in most patients with benign nodules to avoid unnecessary surgery.
To explore the underlying biological explanations of differentiation between benign and malignant samples based on the critical window for BV and MV regions used for the construction of the BEMAD model, we annotated the regions. These regions are mostly intergenic regions, which could act to control the expression of nearby genes. We noticed that windows #57113 and #92863 included a significant number of non-coding RNAs, while window #92938 nearby included immune-related genes (available online: https://cdn.amegroups.cn/static/public/tlcr-22-647-3.xlsx). It is known that non-coding RNAs and immune regulation play important roles in carcinogenesis (52,53), which could be involved in the differentiation of benign and malignant samples.
The present study has some limitations that should be noted. Firstly, the study lacks completely independent validation cohorts from another center. Thus, it would be useful to validate the model at other centers to assess the scalability of our results in other populations. Secondly, radiomic nodule characteristics such as nodule quality (solid, part-solid, or non-solid) and spiculation were not available in our cohort; therefore, it may be useful to assess how radiomic characteristics affect the performance of our model, which would in turn enable a direct comparison with the Veterans Affairs SNAP Cooperative Study Group and Mayo Clinic models (54,55). Thirdly, all samples included in this study were retrospective; thus, a prospective study to assess the performance of the BEMAD model is required for clinical application. It is worth noting that NIPT has been widely used in reproductive medicine for robust clinical evaluation due to the relatively low amount of cfDNA required, the use of genomic sequencing data, and the convenient experimental process with high sensitivity and specificity. Our experimental process could be easily plugged into the current NIPT clinical workflow for whole-genome library construction and data generation using cfDNA. When the BEMAD model was deployed in the diagnostic computational system, it could efficiently provide an accurate evaluation of benign and malignant pulmonary nodules. Hence, our approach could guide subsequent clinical decisions to improve patient care.
The advantages of our approach are that LD-WGS is inexpensive, the method referring to the workflow of NIPT is feasible, and there are no training issues that arise when introducing this approach to clinical practice. So it is worth wide application in diagnosis of pulmonary nodules in clinical. As an auxiliary screening technology for lung cancer, the input data (LD-WGS cfDNA) can be generated by existing conventional non-invasive prenatal screening and diagnostic technologies (such as NIPT), with lower sampling requirements and easier experimental accessibility than methylation sequencing technology. Furthermore, the cost of our approach is one-tenth that of conventional WGS (3 Gb base pair/sample vs. 30 Gb base pair/sample), and much lower than mutation-based methods, such as WES and Panel-seq (a minimum raw data of 100G base pair/sample and 20G base pair/sample). It will be important to explore combining the optimized BEMAD algorithm model with multi-omics biomarkers like the critical features from the miRNA expression and certain methylation variable positions of cfDNA used for early pan-cancer screening to further improve the sensitivity and specificity of benign and malignant diagnoses of pulmonary nodules before its clinical application (56).
Conclusions
In conclusion, we developed a novel non-invasive approach for discriminating between benign and malignant pulmonary nodules. This approach does not necessitate of prior knowledge of the tumor mutation profile, only requires a small amount of plasma and less than 3 Gb genomic data for analysis. In addition, the BEMAD algorithm model is highly robust and is not affected by the most common clinicopathological risk factors. CfDNA LD-WGS could serve as a parallel technique alongside CT imaging to further stratify undiagnosed lung nodules, reducing unnecessary invasive procedures in patients with benign lung nodules.
Acknowledgments
The authors appreciate the academic support from the AME Lung Cancer Collaborative Group. We thank Giuseppe Giaccone and Olivier Elemento from Weill-Cornell Medicine for helpful comments. We also thank Li Zhang from Sun Yat-sen University Cancer Center for helpful discussions. Computational and sequencing resources were provided by China National GeneBank (CNGB). This study made use of data generated by The Chinese University of Hong Kong (CUHK) Circulating Nucleic Acids Research Group, as reported by Jiang et al. in Cancer Discov and by the Sidney Kimmel Comprehensive Cancer Center, Johns Hopkins University School of Medicine, Baltimore, MD, USA, as reported by Mathios et al. in Nat Commun. We thank Hu Xi from The CUHK (Data for EGAS00001003409) and Jillian Phallen from Johns Hopkins University School of Medicine (Data for EGAS00001005340) for providing help with data access (https://ega-archive.org/studies/EGAS00001003409, Accession No. EGAS00001003409; and https://ega-archive.org/studies/EGAS00001005340, Accession No. EGAS00001005340). Finally, we thank H. Nikki March, PhD, from Liwen Bianji (Edanz) (https://www.liwenbianji.cn/) for editing the English text of a draft of this manuscript.
Funding: This work was supported by the National Key Research and Development Program of China Grant (No. 2016YFC0905501 to CW), the National Natural Science Foundation of China (No. 82173038 to DY), and the Guangdong Enterprise Key Laboratory of Human Disease Genomics (No. 2020B1212070028 to KW).
Footnote
Reporting Checklist: The authors have completed the TRIPOD reporting checklist. Available at https://tlcr.amegroups.com/article/view/10.21037/tlcr-22-647/rc
Data Sharing Statement: Available at https://tlcr.amegroups.com/article/view/10.21037/tlcr-22-647/dss
Conflicts of Interest: All authors have completed the ICMJE uniform disclosure form (available at https://tlcr.amegroups.com/article/view/10.21037/tlcr-22-647/coif). AR reports consultancy/advisory board role for Astra Zeneca, MSD, Pfizer, and Novartis. The other authors have no conflicts of interest to declare.
Ethical Statement: The authors are accountable for all aspects of the work in ensuring that questions related to the accuracy or integrity of any part of the work are appropriately investigated and resolved. The study was conducted in accordance with the Declaration of Helsinki (as revised in 2013). This study was approved by the ethical committee of Tianjin Medical University Cancer Institute and Hospital (approval Nos. bc2016014, bc2018009, and bc2019091), and all participants provided written informed consent.
Open Access Statement: This is an Open Access article distributed in accordance with the Creative Commons Attribution-NonCommercial-NoDerivs 4.0 International License (CC BY-NC-ND 4.0), which permits the non-commercial replication and distribution of the article with the strict proviso that no changes or edits are made and the original work is properly cited (including links to both the formal publication through the relevant DOI and the license). See: https://creativecommons.org/licenses/by-nc-nd/4.0/.
References
- Gould MK, Tang T, Liu IL, et al. Recent Trends in the Identification of Incidental Pulmonary Nodules. Am J Respir Crit Care Med 2015;192:1208-14. [Crossref] [PubMed]
- Mazzone PJ, Lam L. Evaluating the Patient With a Pulmonary Nodule: A Review. JAMA 2022;327:264-73. [Crossref] [PubMed]
- National Lung Screening Trial Research Team. Reduced lung-cancer mortality with low-dose computed tomographic screening. N Engl J Med 2011;365:395-409. [Crossref] [PubMed]
- Horeweg N, van Rosmalen J, Heuvelmans MA, et al. Lung cancer probability in patients with CT-detected pulmonary nodules: a prespecified analysis of data from the NELSON trial of low-dose CT screening. Lancet Oncol 2014;15:1332-41. [Crossref] [PubMed]
- Shieh Y, Bohnenkamp M, Low-Dose CT. Scan for Lung Cancer Screening: Clinical and Coding Considerations. Chest 2017;152:204-9. [Crossref] [PubMed]
- de Koning HJ, Meza R, Plevritis SK, et al. Benefits and harms of computed tomography lung cancer screening strategies: a comparative modeling study for the U.S. Preventive Services Task Force. Ann Intern Med 2014;160:311-20. [Crossref] [PubMed]
- Bach PB, Mirkin JN, Oliver TK, et al. Benefits and harms of CT screening for lung cancer: a systematic review. JAMA 2012;307:2418-29. [Crossref] [PubMed]
- Bueno J, Landeras L, Chung JH. Updated Fleischner Society Guidelines for Managing Incidental Pulmonary Nodules: Common Questions and Challenging Scenarios. Radiographics 2018;38:1337-50. [Crossref] [PubMed]
- Yankelevitz DF, Henschke CI. Overdiagnosis in lung cancer screening. Transl Lung Cancer Res 2021;10:1136-40. [Crossref] [PubMed]
- Lenaerts L, Vandenberghe P, Brison N, et al. Genomewide copy number alteration screening of circulating plasma DNA: potential for the detection of incipient tumors. Ann Oncol 2019;30:85-95. [Crossref] [PubMed]
- Lenaerts L, Brison N, Maggen C, et al. Comprehensive genome-wide analysis of routine non-invasive test data allows cancer prediction: A single-center retrospective analysis of over 85,000 pregnancies. EClinicalMedicine 2021;35:100856. [Crossref] [PubMed]
- Chaudhuri AA, Chabon JJ, Lovejoy AF, et al. Early Detection of Molecular Residual Disease in Localized Lung Cancer by Circulating Tumor DNA Profiling. Cancer Discov 2017;7:1394-403. [Crossref] [PubMed]
- Abbosh C, Birkbak NJ, Wilson GA, et al. Phylogenetic ctDNA analysis depicts early-stage lung cancer evolution. Nature 2017;545:446-51. [Crossref] [PubMed]
- Abbosh C, Birkbak NJ, Swanton C. Early stage NSCLC - challenges to implementing ctDNA-based screening and MRD detection. Nat Rev Clin Oncol 2018;15:577-86. [Crossref] [PubMed]
- Leighl NB, Page RD, Raymond VM, et al. Clinical Utility of Comprehensive Cell-free DNA Analysis to Identify Genomic Biomarkers in Patients with Newly Diagnosed Metastatic Non-small Cell Lung Cancer. Clin Cancer Res 2019;25:4691-700. [Crossref] [PubMed]
- García-Pardo M, Makarem M, Li JJN, et al. Integrating circulating-free DNA (cfDNA) analysis into clinical practice: opportunities and challenges. Br J Cancer 2022;127:592-602. [Crossref] [PubMed]
- Chabon JJ, Hamilton EG, Kurtz DM, et al. Integrating genomic features for non-invasive early lung cancer detection. Nature 2020;580:245-51. [Crossref] [PubMed]
- Rolfo C, Russo A. Liquid biopsy for early stage lung cancer moves ever closer. Nat Rev Clin Oncol 2020;17:523-4. [Crossref] [PubMed]
- Ptashkin RN, Mandelker DL, Coombs CC, et al. Prevalence of Clonal Hematopoiesis Mutations in Tumor-Only Clinical Genomic Profiling of Solid Tumors. JAMA Oncol 2018;4:1589-93. [Crossref] [PubMed]
- Newman AM, Bratman SV, To J, et al. An ultrasensitive method for quantitating circulating tumor DNA with broad patient coverage. Nat Med 2014;20:548-54. [Crossref] [PubMed]
- Hu Y, Ulrich BC, Supplee J, et al. False-Positive Plasma Genotyping Due to Clonal Hematopoiesis. Clin Cancer Res 2018;24:4437-43. [Crossref] [PubMed]
- Van der Linden M, Van Gaever B, Raman L, et al. Application of an Ultrasensitive NGS-Based Blood Test for the Diagnosis of Early-Stage Lung Cancer: Sensitivity, a Hurdle Still Difficult to Overcome. Cancers (Basel) 2022;14:2031. [Crossref] [PubMed]
- Leary RJ, Sausen M, Kinde I, et al. Detection of chromosomal alterations in the circulation of cancer patients with whole-genome sequencing. Sci Transl Med 2012;4:162ra154. [Crossref] [PubMed]
- Molparia B, Nichani E, Torkamani A. Assessment of circulating copy number variant detection for cancer screening. PLoS One 2017;12:e0180647. [Crossref] [PubMed]
- Tao K, Bian Z, Zhang Q, et al. Machine learning-based genome-wide interrogation of somatic copy number aberrations in circulating tumor DNA for early detection of hepatocellular carcinoma. EBioMedicine 2020;56:102811. [Crossref] [PubMed]
- Xia S, Huang CC, Le M, et al. Genomic variations in plasma cell free DNA differentiate early stage lung cancers from normal controls. Lung Cancer 2015;90:78-84. [Crossref] [PubMed]
- Raman L, Van der Linden M, Van der Eecken K, et al. Shallow whole-genome sequencing of plasma cell-free DNA accurately differentiates small from non-small cell lung carcinoma. Genome Med 2020;12:35. [Crossref] [PubMed]
- Sanchez C, Roch B, Mazard T, et al. Circulating nuclear DNA structural features, origins, and complete size profile revealed by fragmentomics. JCI Insight 2021;6:144561. [Crossref] [PubMed]
- Mathios D, Johansen JS, Cristiano S, et al. Detection and characterization of lung cancer using cell-free DNA fragmentomes. Nat Commun 2021;12:5060. [Crossref] [PubMed]
- Cristiano S, Leal A, Phallen J, et al. Genome-wide cell-free DNA fragmentation in patients with cancer. Nature 2019;570:385-9. [Crossref] [PubMed]
- Bianchi DW, Chudova D, Sehnert AJ, et al. Noninvasive Prenatal Testing and Incidental Detection of Occult Maternal Malignancies. JAMA 2015;314:162-9. [Crossref] [PubMed]
- Ji X, Li J, Huang Y, et al. Identifying occult maternal malignancies from 1.93 million pregnant women undergoing noninvasive prenatal screening tests. Genet Med 2019;21:2293-302. [Crossref] [PubMed]
- Liang W, Zhao Y, Huang W, et al. Non-invasive diagnosis of early-stage lung cancer using high-throughput targeted DNA methylation sequencing of circulating tumor DNA (ctDNA). Theranostics 2019;9:2056-70. [Crossref] [PubMed]
- Goldstraw P, Chansky K, Crowley J, et al. The IASLC Lung Cancer Staging Project: Proposals for Revision of the TNM Stage Groupings in the Forthcoming (Eighth) Edition of the TNM Classification for Lung Cancer. J Thorac Oncol 2016;11:39-51. [Crossref] [PubMed]
- Travis WD, Brambilla E, Nicholson AG, et al. The 2015 World Health Organization Classification of Lung Tumors: Impact of Genetic, Clinical and Radiologic Advances Since the 2004 Classification. J Thorac Oncol 2015;10:1243-60. [Crossref] [PubMed]
- Jiang P, Sun K, Peng W, et al. Plasma DNA End-Motif Profiling as a Fragmentomic Marker in Cancer, Pregnancy, and Transplantation. Cancer Discov 2020;10:664-73. [Crossref] [PubMed]
- Robin X, Turck N, Hainard A, et al. pROC: an open-source package for R and S+ to analyze and compare ROC curves. BMC Bioinformatics 2011;12:77. [Crossref] [PubMed]
- Adalsteinsson VA, Ha G, Freeman SS, et al. Scalable whole-exome sequencing of cell-free DNA reveals high concordance with metastatic tumors. Nat Commun 2017;8:1324. [Crossref] [PubMed]
- Li K, Luo H, Huang L, et al. Microsatellite instability: a review of what the oncologist should know. Cancer Cell Int 2020;20:16. [Crossref] [PubMed]
- Fujimoto A, Fujita M, Hasegawa T, et al. Comprehensive analysis of indels in whole-genome microsatellite regions and microsatellite instability across 21 cancer types. Genome Res 2020;30:334-46. [Crossref] [PubMed]
- Grogan EL, Weinstein JJ, Deppen SA, et al. Thoracic operations for pulmonary nodules are frequently not futile in patients with benign disease. J Thorac Oncol 2011;6:1720-5. [Crossref] [PubMed]
- Silva S, Danson S, Teare D, et al. Genome-Wide Analysis of Circulating Cell-Free DNA Copy Number Detects Active Melanoma and Predicts Survival. Clin Chem 2018;64:1338-46. [Crossref] [PubMed]
- Wei T, Zhang J, Li J, et al. Genome-wide profiling of circulating tumor DNA depicts landscape of copy number alterations in pancreatic cancer with liver metastasis. Mol Oncol 2020;14:1966-77. [Crossref] [PubMed]
- Paracchini L, Beltrame L, Grassi T, et al. Genome-wide Copy-number Alterations in Circulating Tumor DNA as a Novel Biomarker for Patients with High-grade Serous Ovarian Cancer. Clin Cancer Res 2021;27:2549-59. [Crossref] [PubMed]
- Shen SY, Singhania R, Fehringer G, et al. Sensitive tumour detection and classification using plasma cell-free DNA methylomes. Nature 2018;563:579-83. [Crossref] [PubMed]
- Luo H, Zhao Q, Wei W, et al. Circulating tumor DNA methylation profiles enable early diagnosis, prognosis prediction, and screening for colorectal cancer. Sci Transl Med 2020;12:eaax7533. [Crossref] [PubMed]
- Liu MC, Oxnard GR, Klein EA, et al. Sensitive and specific multi-cancer detection and localization using methylation signatures in cell-free DNA. Ann Oncol 2020;31:745-59. [Crossref] [PubMed]
- Liu B, Ricarte Filho J, Mallisetty A, et al. Detection of Promoter DNA Methylation in Urine and Plasma Aids the Detection of Non-Small Cell Lung Cancer. Clin Cancer Res 2020;26:4339-48. [Crossref] [PubMed]
- Liang N, Li B, Jia Z, et al. Ultrasensitive detection of circulating tumour DNA via deep methylation sequencing aided by machine learning. Nat Biomed Eng 2021;5:586-99. [Crossref] [PubMed]
- Zeng D, Wang C, Mu C, et al. Cell-free DNA from bronchoalveolar lavage fluid (BALF): a new liquid biopsy medium for identifying lung cancer. Ann Transl Med 2021;9:1080. [Crossref] [PubMed]
- Li L, Ye Z, Yang S, et al. Diagnosis of pulmonary nodules by DNA methylation analysis in bronchoalveolar lavage fluids. Clin Epigenetics 2021;13:185. [Crossref] [PubMed]
- Statello L, Guo CJ, Chen LL, et al. Gene regulation by long non-coding RNAs and its biological functions. Nat Rev Mol Cell Biol 2021;22:96-118. [Crossref] [PubMed]
- Slack FJ, Chinnaiyan AM. The Role of Non-coding RNAs in Oncology. Cell 2019;179:1033-55. [Crossref] [PubMed]
- Gould MK, Ananth L, Barnett PG, et al. A clinical model to estimate the pretest probability of lung cancer in patients with solitary pulmonary nodules. Chest 2007;131:383-8. [Crossref] [PubMed]
- Swensen SJ, Silverstein MD, Edell ES, et al. Solitary pulmonary nodules: clinical prediction model versus physicians. Mayo Clin Proc 1999;74:319-29. [Crossref] [PubMed]
- Gao Q, Wang C, Yang X, et al. A multi-cancer early detection model based on liquid biopsy of multi-omics biomarkers: A proof of concept study (PROMISE study). Ann Oncol 2022;33:S417-26. [Crossref]
(English Language Editor: A. Kassem)