Impact of image filtering and assessment of volume-confounding effects on CT radiomic features and derived survival models in non-small cell lung cancer
Introduction
In the last two decades, the increasing availability of digitalized medical imaging has fostered the use of multiple imaging modalities in Radiation Oncology (1-3). This has translated into a variety of applications, ranging from more accurate delineation of the target lesion(s) to the verification of intra- and inter-fractional movements (4). More recently, functional information from positron emission tomography (PET) and magnetic resonance imaging (MRI) has been used to complement standard morphologic imaging for the identification of metabolically-active areas within the volume of interest (5-7) or for a more accurate assessment of treatment response during and after treatment (8). Other than qualitative and semi-quantitative parameters (e.g., lesion dimension, standardized uptake value, SUV, diffusion-weighted MRI), there has been a growing interest in the integration of quantitative parameters into predictive and prognostic models (9,10). As a part of this scenario, radiomics, i.e., the extraction of quantitative data from routinely-acquired medical imaging, holds the promise to provide a bridge between imaging and biological information (11). Although the concept of computerized quantitative analysis is far from new (12), advances in computer sciences and increased computational capabilities have contributed to more reliable results, and brought these concepts closer to clinical implementation (13).
Similar to other big data-based approaches (e.g., genomics, proteomics, metabolomics), radiomics could be used to refine outcome modeling, to assist auto-segmentation tasks and to identify novel predictors of treatment response (14,15). The radiomic workflow is structured into a well-defined pipeline, which generally includes the following steps: image segmentation, preprocessing, features extraction and selection, model construction and validation (11). Although radiomic analysis is quite straightforward, several caveats and limitations are preventing its implementation in the clinics. Firstly, most of the published evidence relies on limited retrospective series, which require management of the disproportionally large number of features compared to the number of patients (16,17). Other than this “curse of dimensionality”, radiomic features also suffer from scarce repeatability and poor reproducibility: multiple extractions from the same subject often differ significantly, and the features values across different equipment, imaging acquisition modalities and software often vary greatly (18-20). More specifically, features show high dependency on the scanner of choice, acquisition parameters (e.g., slice thickness, bin width), intra- and inter-observer variability in segmentation, and from several image-related parameters, including noise (21,22). Convolutional operations and various algorithms for image reconstruction affect feature stability, as confirmed by several studies (18,23-26). However, the role of preprocessing techniques on the features’ reproducibility has received little attention (27).
In this study, we considered CT-based radiomics with non-small cell lung cancer (NSCLC) as the disease model of choice due to its high incidence and disease-related mortality (28). Moreover, several studies have suggested the potentials of radiomics in this clinical setting, with preliminary evidence associating features with tumor heterogeneity (29,30), gene expression (31,32) and clinical outcomes, also in response to radiotherapy (33). Moreover, the incorporation of radiomic signatures into prognostic and predictive models has yielded better performances compared to models built with clinical parameters only (34).
Based on these premises, there is a strong unmet need for a more solid methodological background to facilitate the implementation of radiomics into the clinics. To this aim, it is critical to discriminate whether the quantitative features extracted from medical images hold an independent value, or if any relevant associations exist with other parameters bringing a well-known prognostic/predictive value (e.g., volume). As radiomics lacks strict ground truth and guidelines, our intent is to assess the impact image filters has on volume dependency and survival modelling for different lesion sizes and to identify the most reproducible and informative features among the thousand possible features/preprocessing permutations. As tumor volume is one of the major prognosticators in oncology, the selection of robust volume-independent features would arguably reduce dimensionality without the risk of losing potentially meaningful information. Thus, using a publicly-available repository of NSCLC computed tomography (CT) (35,36), the aims of our work were to:
- Quantify the feature/volume dependency across multiple preprocessing methodologies and volume groups (high vs. low);
- Assess whether these variations have an impact on survival model performance;
- Serve as a hypothesis-generating work for further efforts in the field (e.g., other disease sites or image modalities).
We present the following article in accordance with the MDAR reporting checklist (available at https://tlcr.amegroups.com/article/view/10.21037/tlcr-22-248/rc).
Methods
Clinical dataset
The clinical dataset was retrieved from the publicly available repository curated by The Cancer Imaging Archive (TCIA) (https://www.cancerimagingarchive.net). The following variables were included in the analysis: age at diagnosis (numeric), overall stage (categorical), histology (categorical), overall survival time (numeric) and survival status at last follow-up (binary). The latter was encoded as 0 in case no event had occurred (right-censored or lost to follow-up) and as 1 if the patient was dead at last follow-up. Missing values were imputed with a k-nearest neighbors imputer with k=5 weighted by distance. The study was conducted in accordance with the Declaration of Helsinki (as revised in 2013).
Imaging data acquisition and region of interest segmentation
Four hundred twenty-two chest CTs and as many DICOM radiotherapy structure sets (RTSSs) were downloaded from the dataset source (https://wiki.cancerimagingarchive.net/display/Public/NSCLC-Radiomics). The complete imaging dataset was imported on 3D Slicer version 4.10.2, an open-source platform for imaging visualization, processing, three-dimensional visualization, and quantitative analysis (37). A single Radiation Oncologist (SV) revised each segmentation and edited the gross tumor volume (GTV) contour for the primary tumor, as needed. Moreover, nodal areas encompassed in the original GTV, if any, were removed to overcome possible sources of variability from radiomic features inhomogeneities between primary and nodal volumes.
Feature extraction
All radiomic features were extracted with Pyradiomics v3.0.1 in python 3.7.10 (Numpy 1.19, SimpleITK 2.0, and PyWavelet 1.1). All features and all image types (i.e., image preprocessing filters) were enabled. The default image types available in Pyradiomics are the following (please see the Pyradiomics docs for in-depth descriptions): original, wavelet, Laplacian of Gaussian (LoG), square, square root, logarithm, exponential, gradient, local binary pattern in 2D (lbp-2D), and local binary pattern in 3D (lbp-3D). Specifically, the wavelet image type has nine subcategories: all possible permutations of a high- (H) and low- (L) pass filter in XYZ-directions (e.g., LHL). The lbp-3D image type has an additional three subcategories where one is the kurtosis map (LBP-3D-k) and the other two are calculated with different levels of spherical harmonics (1 and 2 by default, namely, lbp-3D-m1 and lbp-3D-m2, respectively). For the LoG image type, features were calculated from three different sigma values: 1, 2, and 5 times the in-plane image spacing of 0.98 mm, respectively. The bin width was set to 25, which was the default value, and all remaining parameters were also set to their default values. No additional intensity normalization was performed due to the homogeneity of the CT intensities and no image resampling was carried out since the maximum deviation from the median spacing was 0.16 mm. Radiomic features with zero variance and higher than 0.9 Spearman correlation with volume were discarded.
Volume-feature dependence analysis
To quantify the relationship between radiomic features and tumor volume, the Spearman rank correlation coefficient between the volume and all other features was calculated at three different levels:
- For each image preprocessing method (aggregated over all features within the given method);
- For each feature (aggregated over its values for each different preprocessing method);
- For each feature category (aggregated over all its sub-features and preprocessing methods).
The pairwise Spearman correlation was also calculated between the original features and their corresponding values after applying each image preprocessing filter. This served as a proxy for the added value of each image filter, given that a 1.0 correlation with the original features adds no additional information.
Survival models
All experiments were carried out with two different survival models. First, we performed conventional Cox proportional hazards (PH) regression with least absolute shrinkage and selection operator (LASSO) regularization (equivalent to elastic net Cox PH with pure l1 regularization). In this model, the hazard function is assumed a linear function of some baseline hazards. The Cox PH model is often favored for its simplicity, ease of use and rather straightforward interpretation. Second, we trained gradient boosted decision tree ensembles, which often outperform Cox models in practice, but require more parameter tuning and computational overhead. Both models were implemented in Python 3.7; specifically, the Cox PH models were implemented with scikit-survival 0.16 (38) and the gradient boosted (GB) models were implemented with CatBoost 2.10 (39).
Both survival models were evaluated in terms of the concordance index (C-index). While Cox PH models were trained with maximum likelihood estimation, the CatBoost models were trained to regress the survival times directly. For the Cox PH model, the two categorical features were handled as follows: overall stage was ordinally encoded, separating stage IIIA and IIIB into distinct categories, while histology was one-hot encoded. The CatBoost model instead inherently handles categorical features with an embedding technique.
Model training and parameter tuning
To evaluate the impact of volume on survival prediction, two models were trained. The former encompassed clinical parameters, volume and radiomic features; the latter included all previous variables but volume. Our training and validation scheme for the CatBoost model can be divided into four parts as follows:
- Parameter search: an initial 128-step parameter search was performed where each parameter setting was evaluated by the average c-index over 16 repeated shuffled 5-fold cross validation splits. Apart from the regular model parameters, we also included a variable selection step in this search, where the number of clusters, n, in a variable clustering procedure was treated as a separate parameter. This clustering procedure (which was executed only on the radiomic features) can be summarized as follows:
- Fit a k-means clustering with k=n on the variables’ Spearman rank correlation absolute values.
- From each cluster, select the feature with the highest association (as measured by the c-index) with the outcome based on a univariate Cox proportional hazards model.
In addition to the number of clusters, this parameter search explored the following parameter space (see CatBoost documentation for detailed description of the parameters):- n_estimators: 1–256,
- max_depth: 1–6,
- l2_leaf_reg: 0.001–10 (log-uniform prior),
- random_strength: 0.1–3 (log-uniform prior).
- Intermediate model training: the best parameter setting was further evaluated with another shuffled 5-fold cross validation that was instead repeated 64 times. Then, only the variables above 1% importance were selected for the remainder of the training pipeline (CatBoost uses a prediction-value-change definition of importance by default, which shows how much on average the prediction changes if the feature value changes).
- Parameter search two: with the most important variables from the previous step, another parameter search was performed such that the parameters were specifically optimized for the selected features. This was done with another 128-step search of 16 repeated shuffled 5-fold cross validations.
- Final model training: the best parameter setting was finally evaluated with another 64 repeated shuffled 5-fold cross validation.
Because the LASSO Cox PH model implicitly selects the most important features, it was only trained with steps 1 and 4. The Cox PH model also only has one internal parameter that needs to be tuned, namely the l1 regularization term.
Models’ performance when trained with and without volume for each preprocessing method were compared using Wilcoxon signed-ranked test for paired samples, both for Cox PH and CatBoost.
In addition to using the above pipeline to compare models trained with and without volume, we also included a baseline model that was trained only on clinical variables (age, overall stage, histology) and tumor volume. Then, to further explore the variability across different preprocessing filters, the above analysis was repeated independently for each image type (original, wavelet, LoG, square, square root, logarithm, exponential, gradient, lbp-2D, and lbp-3D). A final evaluation was also made on all features considered together.
We also compared the models’ performance when trained separately on low-volume [median volume 9,873 mm3; interquartile range (IQR), 3,897–17,432 mm3] and high-volume (median volume 92,339 mm3; IQR, 52,590–150,240 mm3) patients (as separated by the median value; clinical, volume and radiomic features included) by performing Mann-Whitney U tests. This can elucidate the role of volume when calculating the radiomic features, as well as quantify the strength of its impact.
Finally, we assessed whether the variation in model performances across the three groups could be affected by underlying clinical differences (i.e., sample size, patients’ and tumor characteristics and event rate) rather than radiomic features alone. Wilcoxon signed-rank test was applied on clinical + volume + radiomics vs clinical + volume models (both Cox PH and CatBoost).
Results
Five patients were removed from the dataset due to metadata inconsistencies or corrupt labels (e.g., clashes between the CT image and segmentation file), leaving 417 patients for analysis. In this subset, age was missing for 22 patients, histology for 41 patients, and stage for one patient. The number of censored and tied events was 44 in both cases. Overall, 1,620 features were extracted: 242 were removed due to correlation with volume higher than 0.9, while no features were removed for zero variance.
Considering available clinical parameters, median age at diagnosis was 68.4 years (IQR, 61.2–75.9 years), the most prevalent stage was IIIB (n=175/417, followed by stage IIIA: n=109/417); early stages were the least common, with 92/417 patients belonging to stage I category and 40/417 to stage II. Median GTV for the whole cohort was 30.3 cm3. Squamous and large-cell histotypes were almost equally frequent and constituted 70% of the population altogether. Adenocarcinomas and not otherwise specified were diagnosed in 51 and 62 cases, respectively. Median survival time was 17.9 months (IQR, 9.4–45.5 months).
Volume-feature dependence analysis
Spearman correlation indexes between tumor volume and the different image preprocessing filters are shown in Figure 1A. The median correlation ranged from 0.82 for the lbp-3D-m1 filter to 0.18 for the exponential image.
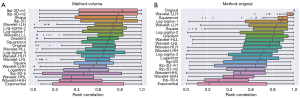
Pairwise Spearman correlation indexes between original features and their corresponding values after the application of preprocessing filter is displayed in Figure 1B. The median correlation ranged from 0.97 for the wavelet-LLL filter to 0.21 for the exponential image.
Spearman correlation indexes between tumor volume and feature categories are displayed in Figure S1. Spearman correlation indexes between tumor volume and the different radiomic features (aggregated over the different image preprocessing filters) are shown in Figure S2. Many of the different shape features (e.g., surface area and axis length) have a high degree of collinearity with volume.
Survival models
The performance of the different models is shown in Table 1. Overall, model performances were not substantially affected by the imaging preprocessing filters, with an overall C-index ranging between 0.539–0.590 for the Cox PH model and between 0.589–0.612 for CatBoost. Cox PH and CatBoost models trained on clinical features with the addition of volume resulted in a C-index of 0.586 and 0.582, respectively. Considering Cox PH models, the median C-index across all used filters was 0.586 (IQR, 0.586–0.587) when all variables including volume were considered, and of 0.585 (IQR, 0.573–0.589) when volume was omitted. Regarding the CatBoost model, the resulting median C-index was 0.597 (IQR, 0.596–0.607) and 0.589 (IQR, 0.596–0.608), with and without volume respectively.
Table 1
Filtering method | Cox PH | CatBoost | |||||||||
---|---|---|---|---|---|---|---|---|---|---|---|
With volume | Without volume | With volume | Without volume | ||||||||
Mean | std | Mean | std | Mean | std | Mean | std | ||||
Wavelet | 0.590* | 0.031 | 0.589* | 0.030 | 0.610* | 0.034 | 0.610* | 0.032 | |||
Original | 0.586 | 0.031 | 0.584 | 0.032 | 0.600 | 0.032 | 0.600 | 0.031 | |||
Log | 0.588* | 0.030 | 0.587 | 0.030 | 0.591 | 0.032 | 0.594 | 0.031 | |||
Square | 0.586 | 0.031 | 0.571 | 0.033 | 0.597 | 0.030 | 0.597 | 0.031 | |||
Squareroot | 0.586 | 0.031 | 0.590* | 0.033 | 0.597 | 0.032 | 0.596 | 0.032 | |||
Logarithm | 0.586 | 0.031 | 0.585 | 0.032 | 0.596 | 0.034 | 0.597 | 0.032 | |||
Exponential | 0.539 | 0.022 | 0.539 | 0.022 | 0.610* | 0.030 | 0.612* | 0.029 | |||
Gradient | 0.567 | 0.033 | 0.567 | 0.033 | 0.593 | 0.028 | 0.589 | 0.033 | |||
Lbp | 0.586 | 0.031 | 0.577 | 0.032 | 0.597 | 0.029 | 0.597 | 0.030 | |||
All | 0.590* | 0.031 | 0.589* | 0.033 | 0.612* | 0.030 | 0.612* | 0.031 |
*, the three best-performing models within each column. PH, proportional hazards; Lbp, local binary pattern; std, standard deviation.
The best performances of the Cox PH models (Table 1, bold values) resulted from the wavelet and square root filters, while the best CatBoost models came from the wavelet and exponential filters. For both survival models, considering all filters together resulted in similar (in the Cox PH case) and slightly better (in the CatBoost case) c-indexes compared to any single filter alone. Moreover, the Wilcoxon signed-rank test showed that the inclusion of volume significantly improved the performance of the Cox PH when LoG, square, square root and lbp filters were applied (Figure 2A). Conversely, for CatBoost, Log, exponential, square root and gradient were associated with significant differences in performances, which were improved following the inclusion of volume in the last two cases (Figure 2B).
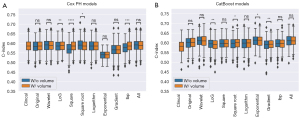
The average total feature importance of the volume variable in all different image preprocessing methods can be seen in Figure S3.
The analysis that considered high and low volume patients separately revealed that, for the Cox PH model, in six out of the ten cases, including all patients had a significantly better performance than only including high volume patients (Figure S4A). In two cases (exponential and all filters), the performance was significantly better with only high-volume patients, and in two cases (square root and logarithm) the difference was insignificant. Conversely, regarding the CatBoost model (Figure S4B), the performance with only high-volume patients was significantly better in all the applied preprocessing methods except for three, in which case the performance difference was insignificant. Moreover, all low volume models had significantly worse performance than the high-volume ones, with P<0.0001 in both models.
Finally, despite some differences in clinical variables exist across the three subgroups, we still could identify an improvement in model performances following the incorporation of radiomic features, as shown in Figure 3. Specifically, the relative gain in model performance was more relevant in the high-volume group as compared to the small volume one (+10.0% vs. +5.4% for Cox PH, and +10.2% vs. 8.7% for CatBoost, P values shown in figure caption).
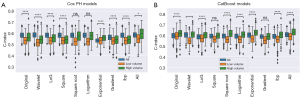
Discussion
Our results show that the use of different preprocessing methods has a potentially relevant impact on feature/volume correlation. On this dataset, we could demonstrate that features/volume dependency varies according to the selected preprocessing technique. Moreover, the features’ value was found to be affected by preprocessing method. This is consistent with the use of preprocessing as a strategy to enhance specific image properties and to potentially unveil hidden information. In our dataset, the lowest degree of variability across filters—and therefore an overlapping informative content as compared to the original image—was observed for the wavelet LLL, square root, and LoG-sigma-1 methods. Considering the specific role of volume in model construction and performance, we observed that its exclusion did not hamper performance when lesions of all sizes were considered, with the sole exception of the square and lpb filters (Cox PH model). This may be explained by the fact that the radiomic features used for model construction may have retained volume-related information. Indeed, results may be affected by the high correlation threshold we have chosen for features selection, which led to a rather modest exclusion of the ones having a high collinearity with volume. More interestingly, we could demonstrate that models trained on high-volume lesions consistently showed significantly better performances as compared to models built on low-volume tumors only (P<0.0001). The same observation was confirmed when high-volume models were tested against those including all lesions, with more consistent improvements for CatBoost models. While such observation is not completely unexpected (33), the entity of these findings is quite relevant, and worth to be considered when designing a new study, since patients’ selection based on lesion volume might impact overall model performance. Overall, this study represents an ideal continuation of the explorative work on the effect of preprocessing published by Fave et al. (27). In their 2016 work, the authors investigated whether different image preprocessing filters could impact the volume dependency of CT-based radiomic features and their prognostic potential. Reported results showed that preprocessing can strongly affect the feature/volume dependency and the prognostic significance of each feature at univariate analysis. This suggests that the volumetric effect should be considered to balance the risks of feature-volume collinearity and the loss of potentially informative content. Unfortunately—to the best of our knowledge—while the final proposed solution of the work is the creation of “standardized features”, such claim has found little to no response in subsequent literature. Admittedly, the main pitfalls of the above-discussed study consist both in the relatively small sample size (n=107), and in the rather limited set of applied filters (namely, 8-bit depth resampling, Butterworth smoothing, and a combination of both).
In contrast with the methodology proposed by Fave et al. (27), we chose to exclude features with zero variance and high correlation with volume (Spearman correlation coefficient ≥0.9) instead of applying normalization strategies. While our decision reflects the aim of isolating the impact of volume on model performance, we still adopted a quite conservative threshold not to discard potentially relevant information. Overall, the confounding effects of volume in radiomic studies warrant further investigations, as the current lack of standardization may strongly impair reproducibility and comparison among studies. This issue has been debated in a recent work by Traverso et al. (40), which has shown that nearly 30% of the 841 extracted features showed a correlation with volume greater than 0.75. Of note, the elimination of redundant features with different correlation coefficients (thresholds ranging from 0 to 1, with 0.05 increments) worsened the stratification of patients per their risk of death. While their results may derive by the fact that radiomic features themselves are not particularly informative in the analyzed dataset, the general recommendation of the authors is to evaluate pairwise correlations between volume and features and in order to maintain only non-redundant ones.
Comparatively, volume dependency across different feature classes- namely, first order, shape texture, and wavelet- was similar in our study as compared to the one by Traverso et al. (40), with texture features being generally more correlated with volume than features belonging to the first order category. Their results highlight how volume represents a confounder for radiomic features, suggesting that appropriate safeguards should be integrated in the radiomic workflow to mitigate these effects. In this regard, it should be noted that, although the Lung1 dataset was used in both works, the segmentations used in our study include the primary tumor only, without encompassing nodal volumes. While this may suggest the generalizability of this finding, further confirmation is needed to fully assess class/volume dependencies in NSCLC. However, despite this similarity, the two works significantly differ in their results, as Traverso and Colleagues did not find any effect of filtering on the volume effect (40). Admittedly, the authors applied only high and low-pass filters, and we could not exclude that the results may have been different if more preprocessing methods had been used. However, the use of all available built-in filters in this study should not be considered as an example for future investigations in the field, but rather a methodological assessment on how preprocessing may affect radiomic analyses. In this regard, we fully share the caveats made by Traverso et al. regarding the cautious use of image filtering, in order to avoid unjustified increases in data dimensionality (40).
Considering model selection, we performed a comparison between a conventional Cox PH regression with LASSO regularization, and gradient boosted decision tree ensembles. The former is the most common approach to identify prognostic factors in oncology, thanks to ease of use, fast computation times, and most importantly, meaningful and straightforwardly interpretable outcomes (41,42). Lately, several machine learning (ML) algorithms have been developed to overcome the shortcomings of statistical models, such as high dimensionality and non-linearities (43-45). Of these, gradient boosted algorithms are used in several works, often in combination with various feature selection techniques, with satisfactory performances (43,46-50). Considering specific applications for NSCLC, a systematic review and metanalysis by Kothari et al. has recently provided a state-of-art representation of radiomics for this subset of patients (33). While 40% of the 40 included studies published between 2013 and 2019 used Cox PH models, other work investigated the use of different ML algorithms, often in combination with various feature selection techniques. As an example, Sun et al. could demonstrate that gradient boosting linear models based on Cox PH’s partial likelihood with the C-index feature selection method outperformed Cox PH, which is true also for the present series, and in line with broader literature data (43,51,52). Another relevant highlight from the work by Kothari et al. (33) is that, overall, model performances benefited from the inclusion of imaging features to conventional clinical data (27,53,54). Our findings are in line with this observation, with percentage improvements of 0.6% for the best performing Cox PH (wavelet filter), and of 5% for the best CatBoosts (all filters combined, exponential and wavelet). Notably, even after correcting for clinical variables we still could observe that radiomics adds potentially more informative content, especially when the high-volume subgroup was considered alone. Despite the above-mentioned strategies (i.e., integration of radiomic features into clinical models, modeling per distinct volumes subgroups, filtering), our overall performances, with C-indexes ranging from 0.539 to 0.612, were only partially satisfactory, yet in line with published literature. As a matter of fact, Kothari et al. reported a random effect estimate for C-index of 0.57 (95% CI: 0.53–0.62), which emphasizes the need of a general refinement of radiomics studies in the field of lung cancer. However, we must acknowledge the limitations of our study. Firstly, the TCIA NSCLC dataset does not provide several well-known clinical prognosticators such as comorbidities and mutational status; and the only outcome available is overall survival, with no information on cancer-specific survival. Additionally, these models currently lack validation on external datasets, which would help to achieve higher robustness. Finally, the quality of the tumor segmentations may have been partially affected by the absence of intravenous contrast agent, especially for the delineation of centrally located lesions; in addition, the editing of the publicly available contours from the TCIA dataset may slightly impair the reproducibility of our work in other centers. On the other hand, this study has several strengths. To start with, a single Radiation Oncologist has segmented the whole dataset, so no inter-observer bias exists. Secondly, the dataset is large, and well beyond the median number of enrolled patients in this type of studies, i.e., 100 (IQR, 50–154), according to Kothari et al. (33). Additionally, radiomic features extraction was compliant with the Image Biomarker Standardization Initiative (IBSI) recommendations, and followed an easily--reproducible methodology, also thanks to the use of open-source tools, such as 3DSlicer and Pyradiomics. Finally, while the inclusion of different disease stages is usually considered as a limitation, in this study it has represented the opportunity of investigating the informative potential of radiomic features extracted across multiple volumes, and to develop high- and low-volume models, and to compare their relative performances.
Conclusions
Our study clearly indicates that radiomic features complement well-known prognostic factors such as volume. However, the features dependence on tumor volume is a critical issue that should be adequately managed in order to limit hindrances such as collinearity and overfitting. In addition, the performance of our survival models suggests that the value of radiomics may be diminished in small-volume lesions, which supports the prior findings that radiomics on small-volume ROIs may be detrimental or uninformative, raising concerns about the clinical applicability of radiomics in these scenarios. However, the precise volume at which these effects start to become critical remains uncertain. Regarding different image filters, the differences between performance outcomes are not strong enough to warrant favoring some filters over others. Therefore, we recommend performing radiomic analysis on multiple filters simultaneously. The performances of our gradient boosted models support findings indicating that modern ML frameworks such as XGBoost, LightGBM, and CatBoost may outperform traditional statistical models, and should therefore be highly regarded in high-stakes scenarios such as healthcare when high dimensional data are involved. More informative datasets, exploration of further modeling techniques, and external validation of the results are strongly encouraged to validate the findings of this study.
Acknowledgments
Funding: The study was fully funded by the University of Milan with APC funds. IEO (European Institute of Oncology) received an institutional research grant from Accuray Inc. and was also partially supported by the Italian Ministry of Health with Ricerca Corrente and 5×1000 funds. The sponsors did not play any role in the study design, collection, analysis and interpretation of data, nor in the writing of the manuscript, nor in the decision to submit the manuscript for publication.
Footnote
Reporting Checklist: The authors have completed the MDAR reporting checklist. Available at https://tlcr.amegroups.com/article/view/10.21037/tlcr-22-248/rc
Peer Review File: Available at https://tlcr.amegroups.com/article/view/10.21037/tlcr-22-248/prf
Conflicts of Interest: All authors have completed the ICMJE uniform disclosure form (available at https://tlcr.amegroups.com/article/view/10.21037/tlcr-22-248/coif). BAJF reports grants and personal fees from Accuray, grants from Fondazione IEO-CCM (Istituto Europeo di Oncologia-Centro Cardiologico Monzino) & FUV (Fondazione Umberto veronesi), grants from AIRC (Italian Association for Cancer Research), personal fees from IBA, personal fees from Elekta, personal fees from Ferring, personal fees from Astra Zeneca, personal fees from Astellas, personal fees from Ipsen, personal fees from Carl Zeiss, personal fees from Janssen, personal fees from Bayer, personal fees from Roche, outside the submitted work. MGV reports grants from AIRC IG-22159, outside the submitted work. MZ reports grants from AIRC-IG 22159, outside the submitted work. SV reports grants from Accuray, outside the submitted work. The other authors have no conflicts of interest to declare.
Ethical Statement: The authors are accountable for all aspects of the work in ensuring that questions related to the accuracy or integrity of any part of the work are appropriately investigated and resolved. The study was conducted in accordance with the Declaration of Helsinki (as revised in 2013).
Open Access Statement: This is an Open Access article distributed in accordance with the Creative Commons Attribution-NonCommercial-NoDerivs 4.0 International License (CC BY-NC-ND 4.0), which permits the non-commercial replication and distribution of the article with the strict proviso that no changes or edits are made and the original work is properly cited (including links to both the formal publication through the relevant DOI and the license). See: https://creativecommons.org/licenses/by-nc-nd/4.0/.
References
- Oderinde OM, Shirvani SM, Olcott PD, et al. The technical design and concept of a PET/CT linac for biology-guided radiotherapy. Clin Transl Radiat Oncol 2021;29:106-12. [Crossref] [PubMed]
- Kesner A, Laforest R, Otazo R, et al. Medical imaging data in the digital innovation age. Med Phys 2018;45:e40-52. [Crossref] [PubMed]
- Ireland RH, Tahir BA, Wild JM, et al. Functional Image-guided Radiotherapy Planning for Normal Lung Avoidance. Clin Oncol (R Coll Radiol) 2016;28:695-707. [Crossref] [PubMed]
- Stieb S, McDonald B, Gronberg M, et al. Imaging for Target Delineation and Treatment Planning in Radiation Oncology: Current and Emerging Techniques. Hematol Oncol Clin North Am 2019;33:963-75. [Crossref] [PubMed]
- van Houdt PJ, Saeed H, Thorwarth D, et al. Integration of quantitative imaging biomarkers in clinical trials for MR-guided radiotherapy: Conceptual guidance for multicentre studies from the MR-Linac Consortium Imaging Biomarker Working Group. Eur J Cancer 2021;153:64-71. [Crossref] [PubMed]
- Ahangari S, Hansen NL, Olin AB, et al. Toward PET/MRI as one-stop shop for radiotherapy planning in cervical cancer patients. Acta Oncol 2021;60:1045-53. [Crossref] [PubMed]
- van Houdt PJ, Yang Y, van der Heide UA. Quantitative Magnetic Resonance Imaging for Biological Image-Guided Adaptive Radiotherapy. Front Oncol 2021;10:615643. [Crossref] [PubMed]
- Cremonesi M, Gilardi L, Ferrari ME, et al. Role of interim 18F-FDG-PET/CT for the early prediction of clinical outcomes of Non-Small Cell Lung Cancer (NSCLC) during radiotherapy or chemo-radiotherapy. A systematic review. Eur J Nucl Med Mol Imaging 2017;44:1915-27. [Crossref] [PubMed]
- Gurney-Champion OJ, Mahmood F, van Schie M, et al. Quantitative imaging for radiotherapy purposes. Radiother Oncol 2020;146:66-75. [Crossref] [PubMed]
- Press RH, Shu HG, Shim H, et al. The Use of Quantitative Imaging in Radiation Oncology: A Quantitative Imaging Network (QIN) Perspective. Int J Radiat Oncol Biol Phys 2018;102:1219-35. [Crossref] [PubMed]
- Lambin P, Leijenaar RTH, Deist TM, et al. Radiomics: the bridge between medical imaging and personalized medicine. Nat Rev Clin Oncol 2017;14:749-62. [Crossref] [PubMed]
- Meyers PH, Nice CM Jr. Automated computer analysis of radiographic images. Arch Environ Health 1964;8:774-5. [Crossref] [PubMed]
- Rogers W, Thulasi Seetha S, Refaee TAG, et al. Radiomics: from qualitative to quantitative imaging. Br J Radiol 2020;93:20190948. [Crossref] [PubMed]
- Coates JTT, Pirovano G, El Naqa I. Radiomic and radiogenomic modeling for radiotherapy: strategies, pitfalls, and challenges. J Med Imaging (Bellingham) 2021;8:031902. [Crossref] [PubMed]
- Volpe S, Pepa M, Zaffaroni M, Bellerba F, et al. Machine Learning for Head and Neck Cancer: A Safe Bet?-A Clinically Oriented Systematic Review for the Radiation Oncologist. Front Oncol 2021;11:772663. [Crossref] [PubMed]
- Clarke R, Ressom HW, Wang A, et al. The properties of high-dimensional data spaces: implications for exploring gene and protein expression data. Nat Rev Cancer 2008;8:37-49. [Crossref] [PubMed]
- Park JE, Park SY, Kim HJ, et al. Reproducibility and Generalizability in Radiomics Modeling: Possible Strategies in Radiologic and Statistical Perspectives. Korean J Radiol 2019;20:1124-37. [Crossref] [PubMed]
- Denzler S, Vuong D, Bogowicz M, et al. Impact of CT convolution kernel on robustness of radiomic features for different lung diseases and tissue types. Br J Radiol 2021;94:20200947. [Crossref] [PubMed]
- Jha AK, Mithun S, Jaiswar V, et al. Repeatability and reproducibility study of radiomic features on a phantom and human cohort. Sci Rep 2021;11:2055. [Crossref] [PubMed]
- Traverso A, Wee L, Dekker A, et al. Repeatability and Reproducibility of Radiomic Features: A Systematic Review. Int J Radiat Oncol Biol Phys 2018;102:1143-58. [Crossref] [PubMed]
- Larue RT, Defraene G, De Ruysscher D, et al. Quantitative radiomics studies for tissue characterization: a review of technology and methodological procedures. Br J Radiol 2017;90:20160665. [Crossref] [PubMed]
- Midya A, Chakraborty J, Gönen M, et al. Influence of CT acquisition and reconstruction parameters on radiomic feature reproducibility. J Med Imaging (Bellingham) 2018;5:011020. [Crossref] [PubMed]
- Erdal BS, Demirer M, Little KJ, et al. Are quantitative features of lung nodules reproducible at different CT acquisition and reconstruction parameters? PLoS One 2020;15:e0240184. [Crossref] [PubMed]
- Mackin D, Ger R, Gay S, et al. Matching and Homogenizing Convolution Kernels for Quantitative Studies in Computed Tomography. Invest Radiol 2019;54:288-95. [Crossref] [PubMed]
- Kim H, Park CM, Lee M, et al. Impact of Reconstruction Algorithms on CT Radiomic Features of Pulmonary Tumors: Analysis of Intra- and Inter-Reader Variability and Inter-Reconstruction Algorithm Variability. PLoS One 2016;11:e0164924. [Crossref] [PubMed]
- Rinaldi L, De Angelis SP, Raimondi S, et al. Reproducibility of radiomic features in CT images of NSCLC patients: an integrative analysis on the impact of acquisition and reconstruction parameters. Eur Radiol Exp 2022;6:2. [Crossref] [PubMed]
- Fave X, Zhang L, Yang J, et al. Impact of image preprocessing on the volume dependence and prognostic potential of radiomics features in non-small cell lung cancer. Transl Cancer Res 2016;5:349-63. [Crossref]
- Ferlay J, Ervik M, Lam F, et al. Global Cancer Observatory (GLOBOCAN): Cancer Today, 2020. 2020 [cited 2021 Jan 27]. Available online: https://gco.iarc.fr/today
- Cucchiara F, Del Re M, Valleggi S, et al. Integrating Liquid Biopsy and Radiomics to Monitor Clonal Heterogeneity of EGFR-Positive Non-Small Cell Lung Cancer. Front Oncol 2020;10:593831. [Crossref] [PubMed]
- Ferreira JR Junior, Koenigkam-Santos M, de Vita Graves C, et al. Quantifying intratumor heterogeneity of lung neoplasms with radiomics. Clin Imaging 2021;74:27-30. [Crossref] [PubMed]
- Kirienko M, Sollini M, Corbetta M, et al. Radiomics and gene expression profile to characterise the disease and predict outcome in patients with lung cancer. Eur J Nucl Med Mol Imaging 2021;48:3643-55. [Crossref] [PubMed]
- Zhao W, Wu Y, Xu Y, et al. The Potential of Radiomics Nomogram in Non-invasively Prediction of Epidermal Growth Factor Receptor Mutation Status and Subtypes in Lung Adenocarcinoma. Front Oncol 2020;9:1485. [Crossref] [PubMed]
- Kothari G, Korte J, Lehrer EJ, et al. A systematic review and meta-analysis of the prognostic value of radiomics based models in non-small cell lung cancer treated with curative radiotherapy. Radiother Oncol 2021;155:188-203. [Crossref] [PubMed]
- Chetan MR, Gleeson FV. Radiomics in predicting treatment response in non-small-cell lung cancer: current status, challenges and future perspectives. Eur Radiol 2021;31:1049-58. [Crossref] [PubMed]
- Aerts HJWL, Wee L, Rios Velazquez E, Leijenaar RTH, Parmar C, Grossmann P, et al. Data From NSCLC-Radiomics. The Cancer Imaging Archive; 2019 [cited 2021 Jun 30]. Available online: https://wiki.cancerimagingarchive.net/x/FgL1
- The Cancer Imaging Archive (TCIA). NSCLC-Radiomics. [cited 2021 Jun 3]. Available online: https://wiki.cancerimagingarchive.net/display/Public/NSCLC-Radiomics
- Fedorov A, Beichel R, Kalpathy-Cramer J, et al. 3D Slicer as an image computing platform for the Quantitative Imaging Network. Magn Reson Imaging 2012;30:1323-41. [Crossref] [PubMed]
- Pölsterl S. Scikit-survival: A Library for Time-to-Event Analysis Built on Top of scikit-learn. J Mach Learn Res 2020;21:1-6.
-
.Prokhorenkova L Gusev G Vorobev A Dorogush AV Gulin A CatBoost: un-biased boosting with categorical features. arXiv:170609516. Available from: https://arxiv.org/abs/1706.09516 - Traverso A, Kazmierski M, Zhovannik I, Welch M, Wee L, Jaffray D, Dekker A, Hope A. Machine learning helps identifying volume-confounding effects in radiomics. Phys Med. 2020;71:24-30. [Crossref] [PubMed]
- Cox DR. Regression Models and Life-Tables. J R Stat Soc Ser B Methodol 1972;34:187-202. [Crossref]
- Mallett S, Royston P, Waters R, et al. Reporting performance of prognostic models in cancer: a review. BMC Med 2010;8:21. [Crossref] [PubMed]
- Moncada-Torres A, van Maaren MC, Hendriks MP, et al. Explainable machine learning can outperform Cox regression predictions and provide insights in breast cancer survival. Sci Rep 2021;11:6968. [Crossref] [PubMed]
- Kim DW, Lee S, Kwon S, et al. Deep learning-based survival prediction of oral cancer patients. Sci Rep 2019;9:6994. [Crossref] [PubMed]
- Ishwaran H, Kogalur UB, Blackstone EH, et al. Random survival forests. Ann Appl Stat 2008;2:841-60. [Crossref]
- Yang L, Pelckmans K. Machine Learning Approaches to Survival Analysis: Case Studies in Microarray for Breast Cancer. Int J Mach Learn Comput 2014;4:483-90. [Crossref]
- Wang Y, Su J, Zhao X. Penalized semiparametric Cox regression model on XGBoost and random survival forests. Commun Stat - Simul Comput 2021;1-12. [Crossref]
- Wang X, You X, Zhang L, et al. A radiomics model combined with XGBoost may improve the accuracy of distinguishing between mediastinal cysts and tumors: a multicenter validation analysis. Ann Transl Med 2021;9:1737. [Crossref] [PubMed]
- Nazari M, Shiri I, Zaidi H. Radiomics-based machine learning model to predict risk of death within 5-years in clear cell renal cell carcinoma patients. Comput Biol Med 2021;129:104135. [Crossref] [PubMed]
- Corso F, Tini G, Lo Presti G, et al. The Challenge of Choosing the Best Classification Method in Radiomic Analyses: Recommendations and Applications to Lung Cancer CT Images. Cancers (Basel) 2021;13:3088. [Crossref] [PubMed]
- Sun W, Jiang M, Dang J, et al. Effect of machine learning methods on predicting NSCLC overall survival time based on Radiomics analysis. Radiat Oncol 2018;13:197. [Crossref] [PubMed]
- Liu P, Fu B, Yang SX, et al. Optimizing Survival Analysis of XGBoost for Ties to Predict Disease Progression of Breast Cancer. IEEE Trans Biomed Eng 2021;68:148-60. [Crossref] [PubMed]
- Ohri N, Duan F, Snyder BS, et al. Pretreatment 18F-FDG PET Textural Features in Locally Advanced Non-Small Cell Lung Cancer: Secondary Analysis of ACRIN 6668/RTOG 0235. J Nucl Med 2016;57:842-8. [Crossref] [PubMed]
- Fried DV, Mawlawi O, Zhang L, et al. Stage III Non-Small Cell Lung Cancer: Prognostic Value of FDG PET Quantitative Imaging Features Combined with Clinical Prognostic Factors. Radiology 2016;278:214-22. [Crossref] [PubMed]