Multi-omics consensus portfolio to refine the classification of lung adenocarcinoma with prognostic stratification, tumor microenvironment, and unique sensitivity to first-line therapies
Highlight box
Key findings
• Four lung adenocarcinoma subtypes were identified successfully through using a multi-omics approach.
• Four lung adenocarcinoma subtypes showed distinct molecular patterns in transcriptome expression, epigenetic methylation and somatic mutations.
• Seven genes (
What is known and what is new?
• It is known that classification of lung adenocarcinoma based on transcriptomic features has been extensively studied.
• In this study, we innovatively integrate multi-omics data to refine the classification of lung adenocarcinoma.
What is the implication, and what should change now?
• Application of multi-omics consistent approach will promote the development of precision medicine and clinical strategies.
• What we need to do is to further optimize multi-omics methods so they can be applied from bench to bedside.
Introduction
Lung cancer is currently the second most common cancer in the world in terms of new cases and the number one cause of cancer death. In 2020, approximately 2.2 million new cases of lung cancer are diagnosed worldwide (1). The prognosis of lung cancer is relatively poor, with 5-year survival rates ranging from 4% to 17%, depending on the stage of the disease at the time of diagnosis (2). Lung cancer is a heterogeneous disease (3,4), and includes several subtypes with pathological and clinical relevance. Lung cancer is generally classified into two major groups: small cell lung carcinoma (SCLC, 15% of cases) and non-small cell lung carcinoma (NSCLC, 85% of cases). Of these, lung adenocarcinoma (LUAD) is the most common histological type, accounting for nearly 60% of NSCLCs, and is characterized by genomic instability and a highly pathogenic mutational burden (5).
Molecular analysis helps classify LUAD into multiple molecular subtypes to guide prognosis prediction and treatment selection more precisely. The understanding of LUAD biology has been significantly improved by multiple molecular classifications of various gene expression profiles at the transcriptome level. The development of malignant transformation requires molecular alterations at many levels. Single-level histological approaches are attempting to unravel the mechanisms of cancer development by interrogating entire genomic libraries, epigenomes, transcripts, proteins, microbiomes, and metabolites through increasingly affordable high-throughput technologies (6). However, there is no single molecule level approach to fully explain the complexity of this problem, such as the cancer genome or pinpointing oncogenic driving mechanisms. Therefore, a multi-omics signature-based classification scheme for LUAD has been proposed, which could potentially reveal the heterogeneity of LUAD. Recently, a multicomponent classification and integration model of LUAD by integrative histology was reported (7), revealing crosstalk features between different molecules, but only KEAP1/NFE2L2/CUL3 alterations in LUAD compromised the normal function of the antioxidant signaling pathway, which hardly explains the heterogeneity of LUAD. A computational machine learning prediction model (PReceur) developed based on genomic molecular features, compared with the TNM system, can better predict LUAD recurrence risk and guide clinical treatment (8). This revealed the promising applications of molecular classifications in the LUAD.
The ability to explain molecular complexity and variation at multiple levels such as genome, epigenome, transcriptome, proteome and metabolome enables us to better understand the occurrence and development of diseases. With the development of sequencing technology, biology increasingly relies on data generated at these levels, which are collectively referred to as “multi-omics” data (9). The Cancer Genome Atlas (TCGA) database has multi-omics data of more than 400 LUAD simples. In this study we aimed to identify integration consistency in LUAD by generating consistent sets from classifications generated by multiple algorithms based on mRNA and lncRNA expression, epigenomic DNA methylation profiles and genomic mutations subtypes (IC) to better outline tumor heterogeneity and biological processes. We also constructed a prognostic model based on the marker genes of each subtype. We present the following article in accordance with the TRIPOD reporting checklist (available at https://tlcr.amegroups.com/article/view/10.21037/tlcr-22-775/rc).
Methods
Multi-omics datasets
The molecular profile of TCGA-NSCLC dataset of TCGA-LUAD cohort was obtained by downloading from TCGA database, including 437 LUAD cases with complete transcriptome expression, somatic mutation, copy number change(CNA), DNA methylation and clinical information. The R package “TCGAbiolinks” is used to process raw sequencing data (10) GENCODE27 mapping was used to transform Ensemble IDs into gene symbols. DNA methylation profile was downloaded from the XENA database (https://xenabrowser.net/). Copy number segment data were downloaded from FireBrowse (http://firebrowse.org/). Somatic mutations and clinical information were downloaded from cBioPortal (https://www.cbioportal.org/). The clinical information is provided in website: https://cdn.amegroups.cn/static/public/tlcr-22-775-01.xlsx.
External transcriptomic datasets
External validation was performed using a microarray dataset with transcriptomic expression profiles and overall clinical outcomes. Lung tumors other than LUAD were not included in this study (e.g., large cell lung cancer, squamous lung cancer, etc.). The microarray dataset (GSE68465, n=442) sequenced by Affymetrix GeneChip was collected from the Gene Expression Omnibus (GEO) database (11). Information on the platform, corresponding sample sizes and clinical characteristics of the dataset are described in detail in website: https://cdn.amegroups.cn/static/public/tlcr-22-775-02.xlsx. For the microarray data, median values were considered if multi-probe IDs were used to annotate gene symbols. The R package “sva” (12) in an empirical Bayesian framework eliminated potential crossover dataset batch effects and further investigated batch effects using principal component analysis.
Multi-omics integration and visualization
For integrated clustering, the TCGA-LUAD dataset was preprocessed to form four data matrices, where rows corresponded to features and columns corresponded to cases (n=437). We extracted probes located on the promoter CpG island and for genes with multiple probes mapping to their promoters, the beta median was applied to obtain the final genes. For the mutation information, the status of the mutant gene was defined as 1 while the wild-type gene was 0. For CNAs, we condensed the genomic fragments described in the literature (13). Features with flat values were removed to better fit the model and to improve clustering efficiency. Specifically, we selected the top 1,500 most variable mRNAs, lncRNAs, and methylated genes based on the absolute deviation from the median. In addition, genes with mutation rate >3% were selected for subtype analysis. Cluster partitioning of the multi-omics data was completed by the “movics” R package, which provides a unified interface to 10 state-of-the-art multi-omics clustering algorithms and normalizes the output of each (14). The 10 algorithms were cimlr, iclusterbayes, mocluster, coca, consensusclustering, intnmf, lracluster, Nemo, pinsplus, and SNF. Among many algorithms, these algorithms had been evaluated for performance in past researches (15). To find an optimal number of clusters, we calculated the clustering prediction index and gap statistics (14) with reference to the previous molecular subtype numbers of LUAD.
Bioinformatics analysis
We analyzed the mutations using the R package “maftools” and initially removed 100 markers (16). Mutation characteristics were also evaluated by the R package “deconstructSigs” (17). GISTIC2.0 detection and localization of recurrent focal somatic CNAs by genotype (https://www.genepattern.org/) with a copy number amplification/deletion threshold equal to ±0.3 (q-value <0.05) (18). Individual scores of copy number altered genomes (FGA) in the TCGA-LUAD samples were computed from copy number fragment data:
where FGA is the ratio of genomes with log2 (copy number) >0.3 to genomes analyzed for copy number Br denotes genomes with |log2R| >0.3 and B denotes the cardinality in all segments. Methylation analysis between tumor and normal tissue was performed using the R program package “ChAMP” (19); detection of hypermethylated promoters followed the following strict criteria: mean methylation β>0.5 in tumor samples with false discovery rate (FDR) <0.05 and mean methylation β<0.2 in adjacent normal samples. In addition to the microarray based predictive analysis (previously identified as TCGA-LUAD subtypes), the R package “consensesmibc” was performed for extensively identifying the individual consensus molecular subtypes (cms). The external cohort was used to verify the robustness of subtype division by nearest template prediction (NTP) (20).
Calculation of microenvironmental cell abundance and enrichment pathways
To create a summary of the list of genes associated with specific microenvironmental cells, two gene markers [CIBERSORT (21) and MCPcounter (22)] needed to be modified. Because CIBERSORT does not contain signals associated with fibroblasts and endothelial cells, 40 genes (32 endothelial cell genes and 8 fibroblast genes) were added to our compendium, of which 364 genes represented 24 microenvironmental cell types. We then analyzed these gene sets using gene set variation analysis (GSVA) and generated enrichment scores for each cell using the R package “GSVA”. The presence of infiltrating immune/stromal cells in the tumor tissue was estimated by the R package “Estimation” (23). In addition, DNA methylation scores of tumor-infiltrating lymphocytes (MeTIL) in the TCGA-LUAD cohort were calculated separately according to the scheme outlined in the literature (24). Ten oncogenic pathways were enriched using the method of GSVA (25). Replication stress signals were enriched via single-sample gene set enrichment analysis (ssGSEA) (26).
Analysis of chemotherapy sensitivity
Chemosensitivity of LUAD was predicted using the R package pRRophetic (https://www.cancerrxgene.org/) using 727 human cancer cell lines as a training group; IC50 was estimated using ridge regression for each sample treated with a specific chemotherapeutic agent (lower IC50 indicated increased sensitivity to treatment) (27).
Exploration of immunotherapeutic response and stemness features
Tumor Immune Dysfunction and Exclusion (TIDE) uses a set of gene expression markers to evaluate mechanisms of the dysfunction of tumor-infiltrating cytotoxic T lymphocytes (CTLs) and the rejection of CTLs by immunosuppressive factors (28). The higher the TIDE score, the less effective the immune checkpoint blockade (ICB) will be. Potential ICB response was predicted with the TIDE algorithm. Data from The Cancer Immunome Atlas (TCIA) database (https://tcia.at/home) were used to predict the response to CTLA-4 and PD-1 immunotherapy. The occurrence of cancer is usually accompanied by gradual loss of differentiation phenotypes and the acquisition of progenitor and stem cell-like characteristics (29). We used a regression machine learning (OCLR) algorithm to calculate the stemness score of each sample for assessing the degree of oncogenic dedifferentiation (29).
Construction of the prognosis model
Biomarkers represent the molecular characteristics of each subtype to a certain extent. We wanted to deeply study the relationship between prognosis and classification and identify biomarkers related to prognosis to explore relevant clinical applications. In order to increase the accuracy of statistical analysis, cases from TCGA with missing OS values or OS values <30 days, missing clinical data (age, gender, stage and TNM classification) were excluded. The top 50 biomarkers significantly upregulated and downregulated in each subtype were used to construct the prognosis model. First, univariate Cox proportional hazard regression analysis was used to seek genes closely related to prognosis. Next, Lasso regression with 1,000 cycles of 10-fold cross-validation was applied for building the model with this formula:
where coef (genek) is the short form of the coefficient of genes correlated with survival and expr (genek) is the expression of genes. We divided the samples into low and high groups based on the median risk score. Overall, 1,000 times of random stimulation in each cycle was performed to avoid overfitting. We established a nomogram that can predict the 1-, 2-, and 3-year survival of LUAD patients. A correction curve according to the Hosmer-Lemeshow test was used to evaluate the accuracy of the nomogram. Meanwhile, immunohistochemical (IHC) results from the HPA database (https://www.proteinatlas.org/) were used to validate the protein level of these gene signatures in normal and tumor tissues.
Statistical analysis
All statistical tests were performed via R software (version:4.0.2), including the two-sample Mann-Whitney test, Fisher’s exact test, log-rank test for Kaplan-Meier curves, and Cox proportional hazards regression for estimating hazard ratios (HRs) and 95% confidence intervals (CIs). The treatment effect of immune checkpoint inhibitors was measured by two non-proportional hazards statistics, namely restricted mean survival and long-term survival extrapolation, using the R packages “survRM2” and “ComparisonSurv” (30). Most of these analytical procedures were embedded in the R package “MOVICS”.
Ethical statement
The study was conducted in accordance with the Declaration of Helsinki (as revised in 2013).
Results
Multi-omics integration of molecular isoforms of LUAD
Based on the two clustering statistics and final calculation results, we identified four optimal clusters (Figure 1A,1B), named CS1 (n=77), CS2 (n=146), CS3 (n=125) and CS4 (n=89). They showed distinct molecular patterns (Figure 1C). These classifications significantly correlated with age, sex, pathological stage (Figure 1C). Notably, CS1 showed the worst OS among the four clusters (Figure 1D). The detailed demographics and clinical information are in the Table S1.
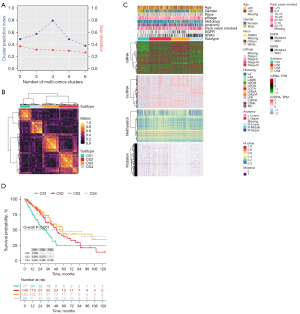
Identification of genetic characteristics of subtypes
We found the highest mutation rate of TP53 and CSMD3 in CS1 and CS2, and the highest mutation rate of KRAS in CS3 and CS4 (Figure 2A). In our further investigation of the genomic heterogeneity of LUAD, we found that CS1 and CS2 showed a higher tumor mutation burden (TMB; Figure 2B) compared with the other subtypes. We then investigated chromosomal instability by calculating FGA scores and found that CS3 had better chromosomal stability than other isoforms, with significantly lower copy number loss or gain (all P<0.001; Figure 2C).
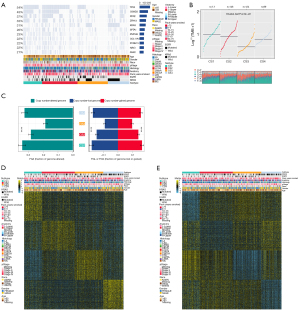
Prognostic value of subtype-specific signatures in the peripheral LUAD cohort
Given that transcriptome-level data are the most commonly used molecular profiles in cancer research, we identified 200 mRNAs with unique and significantly upregulated expression in the TCGA-LUAD cohort as classifiers for each subtype and generated them to individually predict LUAD subtypes identified in the external dataset. The upregulated biomarker heatmap and downregulated biomarker heatmap are shown in Figure 2D,2E. Each subtype of the validation set had many marker genes consistent with the TCGA cohort, which showed the robustness of the classification method (Figure 3A). NTP classified each sample in the external cohort as an already identified subtype (Figure 3B). Among all subtypes, CS1 had the worst prognosis (Figure 3C) and this was highly consistent with the results of the TCGA cohort.
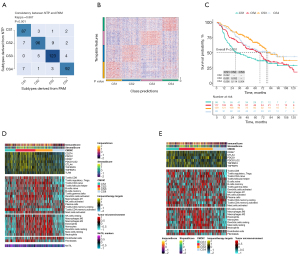
Immune differences among LUAD subtypes
Combined with the immune difference analysis of TCGA cohort and validation set, we found that immune checkpoint-related genes such as CTLA4 and PDCD1LG2 were highly expressed in group CS1 and CS2, which suggested that patients in these subgroups may be more sensitive to the corresponding immunotherapy. CS2 and CS3 had a high immune infiltration environment. Immune-related biological processes were more active in these subgroups. The enrichment degree of endothelial cells and fibroblasts showed the opposite trend (Figure 3D,3E), which indicated a close interaction between these cell types.
Cancer-related pathway analysis and chemosensitivity
Biological processes of DNA damage response detection and transcription-coupled nucleotide excision repair were overactivated in CS1 (Figure 4A), which may explain why CS1 had high FGA and fraction of genome gain/loss (FGG/FGL) values. Numerous immune-related processes, such as response to type I interferon, antigen processing and presentation and the Fcreceptor mediated stimulatory signaling pathway, were overactivated in CS2 (Figure 4A), which was consistent with the analysis of the immune environment. Cell cycle-related processes such as mitotic sister chromatid separation, chromosome segregation and regulation of chromosome separation were inhibited in CS3 (Figure 4B). Treatment targeting cell cycle disorders may be more effective in this subgroup. Many immune-related processes were inhibited in Group CS4, which was again consistent with the preceding results. We evaluated the sensitivity of the four subtypes to commonly used chemotherapeutic drugs and they showed differing sensitivities (Figure 4C). GSVA enrichment analysis showed that each of the four subtypes had characteristic hallmark pathways (Figure 4D).
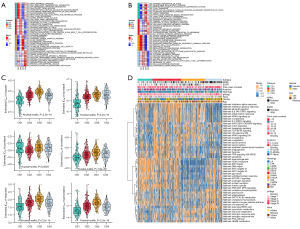
Immunotherapeutic response and stemness features
The TIDE scores of each subtype from high to low were CS2, CS3, CS4 and CS1 (Figure 5A). Patients in CS1 might gain long-lasting clinical benefit from ICB. For CS1, CTLA-4 immunotherapy may have better therapeutic effect (Figure 5B-5E), which was also consistent with the immune analysis results. Combination PD-1 and CTLA-4 immunotherapy might be more effective treatment for patients in CS2 (Figure 5D). Each subtype had distinct differences in stemness features (Figure 5F). CS3 had the highest stemness score, which suggested that these tumor cells could be more invasive, making metastasis to distant tissues easier. Anti-metastasis therapy should be considered for patients in CS3.
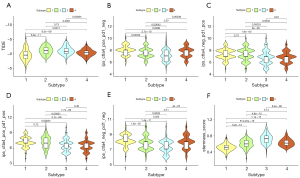
Construction of the prognosis model
We further explored the relationship between marker genes and disease prognosis. According to univariate Cox regression analysis, a total of 99 genes were associated with disease risk (Figure 6A). Among them, 7 (DKK1, TSPAN7, ID1, DLGAP5, HHIPL2, CD40 and SEMA3C) were most suitable for constructing a prognostic model when the first-rank value of log(λ) was the minimum likelihood of deviance (Figure 6B,6C). Principal component analysis showed that the model could clearly divide the samples into high- and low-risk groups (Figure 6D).
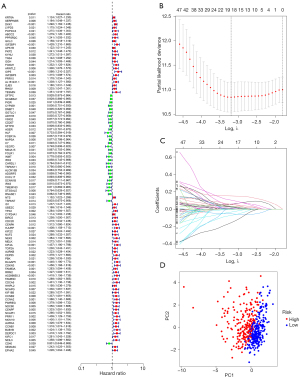
The risk score was calculated with this formula:
risk score = exp (DKK1) × 0.223536825351734+exp (TSPAN7) × (−0.238742797324981)+exp (ID1) × 0.215841381833051+exp (DLGAP5) × 0.182602422124916+exp (HHIPL2) × 0.116091089482409+exp (CD40) × (−0.366461553946006)+exp (SEMA3C) × 0.180363936710061. There were significant differences in survival time between the two groups in both the training set and test set (Figure 7A). The expressions of the 7 genes also showed great differences between the two groups (Figure 7B). Univariate Cox and multivariate Cox regression analyses showed that the risk score could be an independent prognostic factor (Figure 7C,7D). In this model, the 1-year area under the curve (AUC) of the risk score was 0.779, and the AUCs of age, gender and stage were 0.502, 0.589 and 0.655 respectively (Figure 8A). The 1-, 2- and 3-year AUCs of the risk score were 0.779, 0.742 and 0.678 (Figure 8B). The model had a stable effect on predicting the prognosis of patients with different clinical characteristics (Figure 8C-8H). This model also had good performance in the validation test set (Figure 8I,8J). From the IHC results, we found that the protein expressions of DLGAP5 and ID1 were higher in tumor tissues while CD40 was lower in tumor tissues than in normal tissues (Figure S1). This was consistent with the prognosis formula.
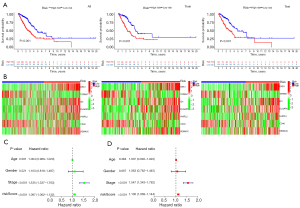
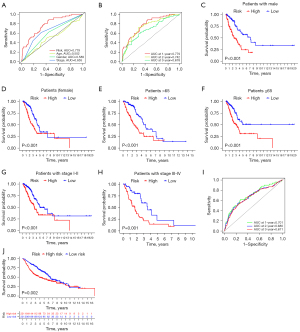
We built a nomogram to predict the 1-, 3- and 5-year survival probability for patients (Figure 9A). A calibration plot was then used to evaluate the accuracy of the nomogram (Figure 9B). The results showed that the nomogram could accurately predict. Additionally, KEGG signal pathways and immune microenvironment were different between the two groups (Figure 9C-9F). The information may help us carry out better personalized treatment against LUAD.
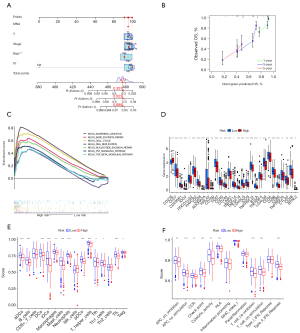
Discussion
To refine the classification of LUAD and clinically relevant biomarkers, we performed a comprehensive and consistent integrated analysis of LUAD in a multi-omics framework and defined four subtypes that provide new ideas for stratifying patients for first-line treatment. We developed and validated a single-sample 200-gene signature to refine the classification of LUAD, and notably, we further constructed a 7-gene base classifier capable of distinguishing patients with poor prognosis from those with traditional base class LUAD. Gene signatures were successfully validated in the external cohort. In general, our results suggested that a more detailed classification of patients based on multi-omics was helpful to further study the pathological mechanism and clinical treatment. Although the current subtypes have different molecular patterns and multi-omics perspectives, we suggest the application of transcriptome-based markers or classifiers in the clinic, because expression profiles can directly reflect the biological processes of tumors and possible therapeutic options.
We attempted to analyze the differences between subtypes. The mutation rate of TP53 and CSMD3 was high in CS1. TP53 acts as a tumor suppressor in many tumor types. The antiproliferative effect of p53 protein in coping with multiple stress and in physiological processes such as aging makes it one of the main inducements of cell carcinogenesis (31). Studies have found that TP53 is closely related to poor prognosis in many cancers, such as breast cancer (32). TP53 deficiency can strengthen the proliferation and invasive activity of LUAD cells and promote angiogenesis and CD8+ T cell failure in the tumor microenvironment through G55-dependent secreted proteins (33). LUAD with TP53 mutations has significantly higher levels of antitumor immune characteristics (34). Additionally, cancers with TP53 mutations are more likely to have higher TMB and tumor aneuploidy level (TAL) (34), which is consistent with our analysis. CSMD3 is involved in regulation of dendrite development. It is a common mutation gene that may be a potential therapeutic target in pulmonary carcinosarcomas (35). Metastasis of LUAD usually leads to poor prognosis. Compared with the primary LUAD, metastases usually show a significantly higher mutation burden and chromosomal instability (36). CS3 had better chromosomal stability and this may become one of the reasons for good prognosis.
Cancer associated fibroblasts (CAFs) are mesenchymal cells with heterogeneous phenotypes. They are the main sources of cytokines and extracellular matrix molecules, which can enhance the invasive activity of tumor cells, help build the vascular system and inhibit antitumor immunity (37). Studies have shown that co-implantation of CAFs and cancer cells can recruit endothelial progenitor cells through SDF-1, which accelerates tumor growth and enhances angiogenesis (38). CAFs also indirectly promote hypoxia in the tumor matrix, resulting in hypoxia-inducible factor (HIF)-1α and vascular endothelial growth factor (VEGF) production and promoting angiogenesis (39). Interestingly, we found that the infiltration abundances of endothelial cells and fibroblasts in the tumor microenvironment were opposite, which suggested a complex regulatory relationship between the two cell populations. Exploring the specific inhibitory mechanism between them may contribute to clinical treatment.
Patients in the high-risk group had lower immune infiltration than those in the low-risk group. High densities of tumor-infiltrating lymphocytes (TILs) are associated with good prognosis (40). Lower densities of TILs in our high-risk group confirmed this. Furthermore, we speculate that the prognosis of the two groups was related to cytokines in the tumor microenvironment. Cytokines play an important role in the pathological process of lung cancer (41). HIF participates in the VEGF pathway regulating angiogenesis (42). Tumor necrosis factor (TNF)-α can cooperate with natural killer cells and CD8+ T cells to activate the immune system against the tumor (43). In addition, TNF-α can activate different pathways to induce tumor cell apoptosis and inhibit tumor angiogenesis (44). Interferon gamma (IFN-γ), known as a cancer inhibitor, mediates signaling pathways such as JAK-STAT, PI3K-AKT, MAPK and NF-κB (45). Transforming growth factor β (TGFβ) is the main immunosuppressive factor secreted by tumor cells and it inhibits the production of interleukin (IL)-2, IL-12 and IFN-γ (46). Many interleukins, such as IL-6, IL-17, IL-8, IL-10, IL-22, IL-1β, and IL-18, are regarded as potential therapeutic targets for lung cancer (47). Inhibitor of DNA binding 1 (ID1) regulates a variety of cellular processes, including cellular growth, senescence, differentiation, apoptosis, angiogenesis, and neoplastic transformation. ID1, an adverse prognostic marker, is an effector of the p53-dependent DNA damage response pathway (48).
The 7 genes used to construct our model may be closely related to the prognosis of LUAD. Dickkopf-1 (DKK1) is an inhibitor of the Wnt/β-catenin signaling pathway. DKK1 may play a crucial role in the progression of NSCLC (49). Activation of DKK1 is associated with poor prognosis of esophageal cancer via the Dickkopf1-CKAP4 pathway and therefore, targeting CKAP4 may be an effective treatment (50). Tetraspanins are a transmembrane 4 superfamily (TM4SF) of proteins that participate in metastasis and invasion of tumor cells (51). TSPAN7 is a member of TM4SF and it has been found that TSPAN7 is a promising prognostic marker in clear-cell renal cell carcinoma (52). Overexpression of DLGAP5 has been found in many types of human cancers and silencing it could inhibit the proliferation of NSCLC cells (53). HHIPL2 is highly expressed in gastric cancer and its overexpression is associated with copy number gain (54). CD40 is essential for T cell-dependent immunoglobulin class switching, memory B cell development, and germinal center formation. CD40 agonist antibodies could enhance T cell infiltration and change the tumor microenvironment to improve the efficacy of chemotherapy and immunotherapy (55). Such therapy may be especially effective for patients in the high-risk group with low expression of CD40. Overexpression of SEMA3C correlates with an increase in cancer cell invasion and adhesion. In prostate cancer, it can active multiple growth factor receptor tyrosine kinase to drive cancer growth (56). A study also found that SEMA3C was associated with poor prognosis in cervical cancer because of the activation of the p-ERK pathway (57). Taking all these findings together, these 7 genes may be potential therapeutic targets for LUAD.
It was shown that immune infiltration played important role in the prognosis of LUAD so we attempted to explore the correlation between immune infiltration and these genes. The expression level of DKK1 was positively correlated with the infiltration level of myeloid derived suppressor cells (MDSCs) in 20 types of cancers and negatively correlated with CD8+ T cells in 4 of these 20 cancer types (58). Tumor vessels highly expressing TSPAN7 gene are associated with extensive infiltration of T cells and B cells and the occurrence of tertiary lymphoid structures (59). CD40 can authorize dendritic cells to promote anti-tumor T cell activation and reactive macrophages to destroy tumor matrix (55). Although no studies have reported the relationship between the remaining genes and immune infiltration, these genes may still affect tumor immune invasion through indirect biological processes.
We should also acknowledge that this analysis has some limitations. All results were based on bioinformatics analysis in this study. It was better to verify the constructed prognostic model by real world data. It takes a lot of time and research to transform the multi-omics technology in the laboratory into the common medical technology at the bedside. A major obstacle is the uneven maturity of the different histological approaches used for routine clinical applications (6). Therefore, in the future it will be necessary to combine these RNA-based findings with multiplex IHC to study the intrinsic alterations of tumor cells and their interaction with the tumor microenvironment that determines the therapeutic response. The accuracy of data classification depends on sample size, sample type, sample preparation, and omics data type. In addition, environmental parameters including laboratory conditions and the choice of experimental materials can also affect the results of multi-omics studies (15). In order to improve the translation of multi-omics, the parameters of the latent variables should be carefully determined and harmonized as much as possible.
Conclusions
In a word, through a combined multi-omics approach, we successfully classified LUAD into 4 subtypes, which are closely related to prognosis, tumor microenvironmental features, molecular characteristics and first-line therapies. Our findings may help us better understand and explore the heterogeneity of LUAD and the corresponding pathological mechanism. We hope that this innovative approach for LUAD classification will further aid precision medicine and inform the development of rational clinical strategies for both targeted and immune therapy.
Acknowledgments
We greatly appreciate the patients and investigators who participated in the corresponding medical project for providing data.
Funding: This project was supported by the Natural Science Foundation of Hubei Province (No. 2021CFB372, to Hua Xiong) and the National Natural Science Foundation of China (No. 82272902, to Yanmei Zou).
Footnote
Reporting Checklist: The authors have completed the TRIPOD reporting checklist. Available at https://tlcr.amegroups.com/article/view/10.21037/tlcr-22-775/rc
Conflicts of Interest: All authors have completed the ICMJE uniform disclosure form (available at https://tlcr.amegroups.com/article/view/10.21037/tlcr-22-775/coif). The authors have no conflicts of interest to declare.
Ethical Statement: The authors are accountable for all aspects of the work in ensuring that questions related to the accuracy or integrity of any part of the work are appropriately investigated and resolved. The study was conducted in accordance with the Declaration of Helsinki (as revised in 2013).
Open Access Statement: This is an Open Access article distributed in accordance with the Creative Commons Attribution-NonCommercial-NoDerivs 4.0 International License (CC BY-NC-ND 4.0), which permits the non-commercial replication and distribution of the article with the strict proviso that no changes or edits are made and the original work is properly cited (including links to both the formal publication through the relevant DOI and the license). See: https://creativecommons.org/licenses/by-nc-nd/4.0/.
References
- Sung H, Ferlay J, Siegel RL, et al. Global Cancer Statistics 2020: GLOBOCAN Estimates of Incidence and Mortality Worldwide for 36 Cancers in 185 Countries. CA Cancer J Clin 2021;71:209-49. [Crossref] [PubMed]
- Hirsch FR, Scagliotti GV, Mulshine JL, et al. Lung cancer: current therapies and new targeted treatments. Lancet 2017;389:299-311. [Crossref] [PubMed]
- Yang D, Liu Y, Bai C, et al. Epidemiology of lung cancer and lung cancer screening programs in China and the United States. Cancer Lett 2020;468:82-7. [Crossref] [PubMed]
- de Sousa VML, Carvalho L. Heterogeneity in Lung Cancer. Pathobiology 2018;85:96-107. [Crossref] [PubMed]
- Comprehensive molecular profiling of lung adenocarcinoma. Nature 2014;511:543-50. [Crossref] [PubMed]
- Menyhárt O, Győrffy B. Multi-omics approaches in cancer research with applications in tumor subtyping, prognosis, and diagnosis. Comput Struct Biotechnol J 2021;19:949-60. [Crossref] [PubMed]
- Jin X, Zheng Y, Chen Z, et al. Integrated analysis of patients with KEAP1/NFE2L2/CUL3 mutations in lung adenocarcinomas. Cancer Med 2021;10:8673-92. [Crossref] [PubMed]
- Jones GD, Brandt WS, Shen R, et al. A Genomic-Pathologic Annotated Risk Model to Predict Recurrence in Early-Stage Lung Adenocarcinoma. JAMA Surg 2021;156:e205601. [Crossref] [PubMed]
- Subramanian I, Verma S, Kumar S, et al. Multi-omics Data Integration, Interpretation, and Its Application. Bioinform Biol Insights 2020;14:1177932219899051. [Crossref] [PubMed]
- Colaprico A, Silva TC, Olsen C, et al. TCGAbiolinks: an R/Bioconductor package for integrative analysis of TCGA data. Nucleic Acids Res 2016;44:e71. [Crossref] [PubMed]
- Shedden K, Taylor JM, et al. Gene expression-based survival prediction in lung adenocarcinoma: a multi-site, blinded validation study. Nat Med 2008;14:822-7. [Crossref] [PubMed]
- Leek JT, Johnson WE, Parker HS, et al. The sva package for removing batch effects and other unwanted variation in high-throughput experiments. Bioinformatics 2012;28:882-3. [Crossref] [PubMed]
- Mo Q, Wang S, Seshan VE, et al. Pattern discovery and cancer gene identification in integrated cancer genomic data. Proc Natl Acad Sci U S A 2013;110:4245-50. [Crossref] [PubMed]
- Lu X, Meng J, Zhou Y, et al. MOVICS: an R package for multi-omics integration and visualization in cancer subtyping. Bioinformatics 2020;btaa1018. Epub ahead of print. [Crossref] [PubMed]
- Pierre-Jean M, Deleuze JF, Le Floch E, et al. Clustering and variable selection evaluation of 13 unsupervised methods for multi-omics data integration. Brief Bioinform 2020;21:2011-30. [Crossref] [PubMed]
- Mayakonda A, Lin DC, Assenov Y, et al. Maftools: efficient and comprehensive analysis of somatic variants in cancer. Genome Res 2018;28:1747-56. [Crossref] [PubMed]
- Rosenthal R, McGranahan N, Herrero J, et al. DeconstructSigs: delineating mutational processes in single tumors distinguishes DNA repair deficiencies and patterns of carcinoma evolution. Genome Biol 2016;17:31. [Crossref] [PubMed]
- Mermel CH, Schumacher SE, Hill B, et al. GISTIC2.0 facilitates sensitive and confident localization of the targets of focal somatic copy-number alteration in human cancers. Genome Biol 2011;12:R41. [Crossref] [PubMed]
- Tian Y, Morris TJ, Webster AP, et al. ChAMP: updated methylation analysis pipeline for Illumina BeadChips. Bioinformatics 2017;33:3982-4. [Crossref] [PubMed]
- Hoshida Y. Nearest template prediction: a single-sample-based flexible class prediction with confidence assessment. PLoS One 2010;5:e15543. [Crossref] [PubMed]
- Newman AM, Liu CL, Green MR, et al. Robust enumeration of cell subsets from tissue expression profiles. Nat Methods 2015;12:453-7. [Crossref] [PubMed]
- Becht E, Giraldo NA, Lacroix L, et al. Estimating the population abundance of tissue-infiltrating immune and stromal cell populations using gene expression. Genome Biol 2016;17:218. [Crossref] [PubMed]
- Yoshihara K, Shahmoradgoli M, Martínez E, et al. Inferring tumour purity and stromal and immune cell admixture from expression data. Nat Commun 2013;4:2612. [Crossref] [PubMed]
- Jeschke J, Bizet M, Desmedt C, et al. DNA methylation-based immune response signature improves patient diagnosis in multiple cancers. J Clin Invest 2017;127:3090-102. [Crossref] [PubMed]
- Sanchez-Vega F, Mina M, Armenia J, et al. Oncogenic Signaling Pathways in The Cancer Genome Atlas. Cell 2018;173:321-337.e10. [Crossref] [PubMed]
- Dreyer SB, Upstill-Goddard R, Paulus-Hock V, et al. Targeting DNA Damage Response and Replication Stress in Pancreatic Cancer. Gastroenterology 2021;160:362-377.e13. [Crossref] [PubMed]
- Geeleher P, Cox NJ, Huang RS. Clinical drug response can be predicted using baseline gene expression levels and in vitro drug sensitivity in cell lines. Genome Biol 2014;15:R47. [Crossref] [PubMed]
- Jiang P, Gu S, Pan D, et al. Signatures of T cell dysfunction and exclusion predict cancer immunotherapy response. Nat Med 2018;24:1550-8. [Crossref] [PubMed]
- Malta TM, Sokolov A, Gentles AJ, et al. Machine Learning Identifies Stemness Features Associated with Oncogenic Dedifferentiation. Cell 2018;173:338-354.e15. [Crossref] [PubMed]
- Liang F, Zhang S, Wang Q, et al. Treatment effects measured by restricted mean survival time in trials of immune checkpoint inhibitors for cancer. Ann Oncol 2018;29:1320-4. [Crossref] [PubMed]
- Levine AJ. p53, the cellular gatekeeper for growth and division. Cell 1997;88:323-31. [Crossref] [PubMed]
- Petitjean A, Achatz MI, Borresen-Dale AL, et al. TP53 mutations in human cancers: functional selection and impact on cancer prognosis and outcomes. Oncogene 2007;26:2157-65. [Crossref] [PubMed]
- Tan X, Shi L, Banerjee P, et al. A protumorigenic secretory pathway activated by p53 deficiency in lung adenocarcinoma. J Clin Invest 2021;131:137186. [Crossref] [PubMed]
- Li L, Li M, Wang X. Cancer type-dependent correlations between TP53 mutations and antitumor immunity. DNA Repair (Amst) 2020;88:102785. [Crossref] [PubMed]
- Li F, Hu S, Kong K, et al. Next-Generation Sequencing Analysis Identified Genomic Alterations in Pathological Morphologies of 3 Cases of Pulmonary Carcinosarcoma. Onco Targets Ther 2020;13:7963-72. [Crossref] [PubMed]
- Li D, Huang Y, Cai L, et al. Genomic landscape of metastatic lung adenocarcinomas from large-scale clinical sequencing. Neoplasia 2021;23:1204-12. [Crossref] [PubMed]
- Bartoschek M, Oskolkov N, Bocci M, et al. Spatially and functionally distinct subclasses of breast cancer-associated fibroblasts revealed by single cell RNA sequencing. Nat Commun 2018;9:5150. [Crossref] [PubMed]
- Orimo A, Gupta PB, Sgroi DC, et al. Stromal fibroblasts present in invasive human breast carcinomas promote tumor growth and angiogenesis through elevated SDF-1/CXCL12 secretion. Cell 2005;121:335-48. [Crossref] [PubMed]
- Brown EB, Boucher Y, Nasser S, et al. Measurement of macromolecular diffusion coefficients in human tumors. Microvasc Res 2004;67:231-6. [Crossref] [PubMed]
- Fridman WH, Pagès F, Sautès-Fridman C, et al. The immune contexture in human tumours: impact on clinical outcome. Nat Rev Cancer 2012;12:298-306. [Crossref] [PubMed]
- Abolfathi H, Sheikhpour M, Shahraeini SS, et al. Studies in lung cancer cytokine proteomics: a review. Expert Rev Proteomics 2021;18:49-64. [Crossref] [PubMed]
- Semenza GL. Targeting HIF-1 for cancer therapy. Nat Rev Cancer 2003;3:721-32. [Crossref] [PubMed]
- Prévost-Blondel A, Roth E, Rosenthal FM, et al. Crucial role of TNF-alpha in CD8 T cell-mediated elimination of 3LL-A9 Lewis lung carcinoma cells in vivo. J Immunol 2000;164:3645-51. [Crossref] [PubMed]
- Watanabe N, Niitsu Y, Umeno H, et al. Toxic effect of tumor necrosis factor on tumor vasculature in mice. Cancer Res 1988;48:2179-83. [PubMed]
- Zhang X, Zeng Y, Qu Q, et al. PD-L1 induced by IFN-γ from tumor-associated macrophages via the JAK/STAT3 and PI3K/AKT signaling pathways promoted progression of lung cancer. Int J Clin Oncol 2017;22:1026-33. [Crossref] [PubMed]
- McKarns SC, Schwartz RH, Kaminski NE. Smad3 is essential for TGF-beta 1 to suppress IL-2 production and TCR-induced proliferation, but not IL-2-induced proliferation. J Immunol 2004;172:4275-84. [Crossref] [PubMed]
- Lippitz BE. Cytokine patterns in patients with cancer: a systematic review. Lancet Oncol 2013;14:e218-28. [Crossref] [PubMed]
- Qian Y, Chen X. ID1, inhibitor of differentiation/DNA binding, is an effector of the p53-dependent DNA damage response pathway. J Biol Chem 2008;283:22410-6. [Crossref] [PubMed]
- Li S, Qin X, Guo X, et al. Dickkopf-1 is oncogenic and involved in invasive growth in non small cell lung cancer. PLoS One 2013;8:e84944. [Crossref] [PubMed]
- Shinno N, Kimura H, Sada R, et al. Activation of the Dickkopf1-CKAP4 pathway is associated with poor prognosis of esophageal cancer and anti-CKAP4 antibody may be a new therapeutic drug. Oncogene 2018;37:3471-84. [Crossref] [PubMed]
- Yáñez-Mó M, Barreiro O, Gordon-Alonso M, et al. Tetraspanin-enriched microdomains: a functional unit in cell plasma membranes. Trends Cell Biol 2009;19:434-46. [Crossref] [PubMed]
- Wuttig D, Zastrow S, Füssel S, et al. CD31, EDNRB and TSPAN7 are promising prognostic markers in clear-cell renal cell carcinoma revealed by genome-wide expression analyses of primary tumors and metastases. Int J Cancer 2012;131:E693-704. [Crossref] [PubMed]
- Wang Q, Chen Y, Feng H, et al. Prognostic and predictive value of HURP in nonsmall cell lung cancer. Oncol Rep 2018;39:1682-92. [PubMed]
- Junnila S, Kokkola A, Karjalainen-Lindsberg ML, et al. Genome-wide gene copy number and expression analysis of primary gastric tumors and gastric cancer cell lines. BMC Cancer 2010;10:73. [Crossref] [PubMed]
- Vonderheide RH. CD40 Agonist Antibodies in Cancer Immunotherapy. Annu Rev Med 2020;71:47-58. [Crossref] [PubMed]
- Peacock JW, Takeuchi A, Hayashi N, et al. SEMA3C drives cancer growth by transactivating multiple receptor tyrosine kinases via Plexin B1. EMBO Mol Med 2018;10:219-38. [Crossref] [PubMed]
- Liu R, Shuai Y, Luo J, et al. SEMA3C Promotes Cervical Cancer Growth and Is Associated With Poor Prognosis. Front Oncol 2019;9:1035. [Crossref] [PubMed]
- Chu HY, Chen Z, Wang L, et al. Dickkopf-1: A Promising Target for Cancer Immunotherapy. Front Immunol 2021;12:658097. [Crossref] [PubMed]
- Sawada J, Hiraoka N, Qi R, et al. Molecular Signature of Tumor-Associated High Endothelial Venules That Can Predict Breast Cancer Survival. Cancer Immunol Res 2022;10:468-81. [Crossref] [PubMed]