Landscape and clinical impact of metabolic alterations in non-squamous non-small cell lung cancer
Introduction
Cancer-associated metabolic reprogramming, a hallmark of tumorigenesis, refers to the biochemical processes that allow cells to retain energy, balance oxidation-reduction, and synthesize the extra building blocks required for cancer cell development, proliferation, and survival (1). In non-small cell lung cancer (NSCLC), several metabolic alterations have been shown to support cancer progression. Driver mutations such as EGFR, ALK, and KRAS have been found in 30–40% of patients with NSCLC (2,3). Mutations in EGFR can promote metabolic remodeling in NSCLC by increasing glycolysis and alterating pyrimidine biosynthesis (4). ALK rearrangements lead to upregulation of glucose metabolism, which is usually associated with more aggressive cancer phenotypes (5). Mutations in KRAS have been associated with upregulation of glucose uptake and the Warburg effect (6). Metabolic reprogramming is also a therapeutic target in non-squamous NSCLC. The clinical utility of antimetabolites such as pemetrexed and fluoropyrimidines is partly attributable to their ability to interfere with the increased metabolic demands of cancer cells for nucleotide biosynthesis and DNA replication (7-9).
Several groups have explored metabolic reprogramming role in non-squamous NSCLC by using mass spectrometry (10-12) and produced comprehensive maps of altered metabolites for different disease stages, demonstrating clear differences between malignant and benign tissues. Wikoff et al. (10), analyzed cellular metabolites from tissue samples of patients with stages IA-B lung adenocarcinoma (LUAD) using gas chromatography-time-of flight mass spectrometry (GC-TOF-MS) and Moreno et al. (11) analyzed cellular metabolites from tissue samples of patients with stages I-III LUAD using ultra performance liquid chromatography tandem mass spectrometry (UPLC/MS/MS) with gas chromatography mass spectrometry (GC-MS). Results from both studies showed broad metabolic alterations between malignant and non-malignant LUAD tissue, which affected 40% of metabolic functions that were investigated.
However, one key question remains: Do all metabolic alterations associated with LUAD equally impact clinical outcome or are some merely bystander events resulting from rapid cell growth? To answer this question, we applied a systems biology approach to (I) define consensus altered metabolic functions in non-squamous NSCLC, (II) identify the pool of genes directly controlling those metabolic functions using Kyoto Encyclopedia of Genes and Genomes (KEGG), and (III) interrogate The Cancer Genome Atlas (TCGA) to identify the metabolic alterations associated with overall survival (OS) in LUAD. Among consensus metabolic alterations, we identified 42 metabolic genes of clinical significance. The majority of them clustered within three metabolic superpathways (carbohydrates, amino acids, and nucleotides) and were associated with shorter OS. We present the following article in accordance with the STARD reporting checklist (available at https://tlcr.amegroups.com/article/view/10.21037/tlcr-22-377/rc).
Methods
Ethical statement
The study was conducted in accordance with the Declaration of Helsinki (as revised in 2013).
Metabolic data normalization and comparison
Quantitative data from two independent retrospective studies by Wikoff et al. (10) and Moreno et al. (11), that compared metabolic differences between malignant and benign NSCLC tissues, were compared. The Wikoff study surveyed 39 stages IA-B LUAD tumors and identified 183 altered metabolites; the Moreno study surveyed 33 stages IA-IIIB LUAD tumors and identified 851 altered metabolites (Table S1). To compare metabolic alterations between these two studies, we compiled the reported data, normalized the cellular metabolite nomenclature across studies using the KEGG ENZYME database (13), and calculated (or compiled when such data was available) log2 fold changes between malignant and benign NSCLC tissues. Cellular metabolites with log2 fold-change ranging from −1.52 to +3.20 and a false discovery rate (FDR)-adjusted P value <0.05 in at least one of the studies were considered significantly altered in non-squamous NSCLC.
Metabolic pathways annotation and gene ontology
To group cellular metabolites into metabolic pathways and superpathways, based on the biosynthesis or metabolism of related compounds, we used the KEGG PATHWAY database (RRID: SCR_012773) (14). We used the KEGG ENZYME database to identify each catabolic and anabolic enzyme associated with those metabolic pathways and the genes coding them (13), thereby converting the altered metabolic pathways and superpathways into gene signatures.
Whole exome sequencing (WES) data were used to identify function-altering mutations of metabolic enzyme coding genes. Transcriptomic (RNAseq) data were used to confirm and quantify metabolic gene expression. WES and RNAseq data from non-squamous NSCLC (LUAD cohort) were obtained from TCGA v21.0 (RRID: SCR_003193) (https://portal.gdc.cancer.gov/). Function-altering mutations were defined as non-synonymous mutations with an allele frequency >10%, a SIFT score <1, and a PolyPhen score >0. Included in the list of non-synonymous mutations were mutations modifying upstream or downstream genes, as well as splice region, frameshifts, mutations in introns, and 3' and 5' untranslated regions. Metabolic gene transcript abundance estimates calculated by the Cancer Genome Analysis group at the Broad Institute using RNA-Seq by Expectation Maximization (RSEM) (15) were compiled from GDAC Firehose (gdac_rnaseqv2_genes_RSEM_normalized_Level_3, 2016-02-18). Relative expression of metabolic genes are reported as number of transcripts per million mapped reads.
Clinical annotation of metabolomic and genomic non-squamous NSCLC cohorts
For each metabolomic cohort, we compiled demographic and clinical data from the 39 patients surveyed in the Wikoff study and 33 in the Moreno study (10,11). For the genomic cohort, demographic, clinical, and treatment information were compiled using GDAC Firehose (http://gdac.broadinstitute.org/), a TCGA data analysis infrastructure developed by the Broad Institute. The TCGA LUAD cohort contained 585 patients; however, clinical information was missing for 63 patients and thus were removed from the analyses; 522 TCGA LUAD patients were included in the final analyses (Table S1). All patients from both metabolomic cohorts and TCGA were treatment naïve.
Statistical analysis
Descriptive statistical analysis was performed with Graphpad Prism 9. ANOVA and pair-wise comparison analysis was performed using JMP15 software (RRID: SCR_014242) (SAS Institute, Cary, NC, USA). One-way ANOVA was used to test the frequency of function-altering mutations between metabolic pathways, and pair-wise comparison was used to compare differences between each metabolic pathway. Pair-wise comparison was also used to compare differences in metabolic genes expression between mutated vs. non-mutated genes. Fisher’s exact test was applied to determine whether demographic or clinical factors affect the frequency of mutations in genes of clinical significance in OS. Differences were considered significant at P<0.05.
Cox proportional hazards model was used to identify genes associated with survival in LUAD patients. The Benjamini-Hochberg procedure was used to control the FDR. Genes associated with survival (raw P value <0.1) were selected and grouped into their respective biochemical pathways.
For each patient, if a mutation was detected in at least one gene on the pathway, the patient was considered to have a mutation in this pathway. Cox proportional hazards model was then used to study the association between mutation on each pathway and survival time. Age, gender, smoking status, tumor stage, and therapy (chemo- or radiation therapy) were adjusted in the model. Hazard ratios and confidence intervals were calculated. Pathways with FDR-P<0.05 were considered statistically significant. To determine whether the effect of pathway mutations on OS varies by cancer stages or treatment type, pathway × stage interaction and treatment × stage interaction effect were also included in the initial Cox regression model. Interaction terms with P>0.2 were removed from the final model.
Results
Conserved patterns of metabolic alterations in non-squamous NSCLC across metabolomics studies
We compared quantitative analyses data from two retrospective studies by Wikoff et al. and Moreno et al. (10,11), comparing metabolic differences between malignant and benign NSCLC tissues. To account for methodological differences, we restricted our analyses to metabolites quantified in both studies. To compare data between these two studies, we normalized nomenclature of metabolites of reported data, compiled (or calculated) log2 fold changes and FDR-adjusted P values for each cellular metabolite detected in both studies. Thirty one of 183 tested (16.9%) in the Wikoff study and 49 of 581 tested (8.4%) in the Moreno study (Figure 1, FDR-P<0.05) were detected at significantly different levels between normal tissue and LUAD tissue, while 27 significantly altered metabolites were shared by both studies.
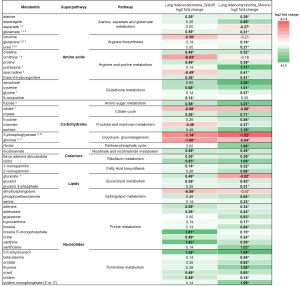
In total, we found that 53 cellular metabolites were detected at different levels between malignant and benign LUAD tissues in at least one of the studies (Figure 1, FDR-P<0.05). Across both data sets, 43 of those cellular metabolites (81.2%) were at higher concentrations in LUAD tumor tissue than in normal tissue, and 4 (7.5%) were at lower concentrations; 6 (11.3%) had discrepant results between studies. The magnitude of metabolic alterations was also very similar between the two studies (median log2 fold-change: 0.26, range −1.14 to +1.81 vs. 0.46, range −1.52 to + 3.20; P=0.912). Using the KEGG PATHWAY database, we categorized the 53 metabolites associated with LUAD into their respective 16 metabolic pathways, which clustered into 5 superpathways: amino acid, carbohydrate, cofactor, lipid, and nucleotide metabolism (Figure S1).
Evenly distributed cellular metabolism alterations in non-squamous NSCLC across metabolic superpathways
Given the similarities in metabolic alterations between the two LUAD cohorts, we hypothesized that gene mutations in those 16 metabolic pathways may be responsible for the alterations in the corresponding metabolites. Using KEGG ENZYME database, we catalogued 736 genes coding for the 490 enzymes that control the 16 metabolic pathways (Table 1). One hundred seventy six of 736 genes (23.9%) coded for enzymes directly controlling anabolism or catabolism of the 53 cellular metabolites altered in non-squamous NSCLC, the remaining 560 genes more broadly controlling the 16 metabolic pathways of interest. The number of genes that control each metabolic pathway varied between 8 for riboflavin metabolism and 133 for purine metabolism, with a median of 42.5 genes per pathway (Figure S1).
Table 1
Metabolic superpathway | Metabolic pathway | Number of enzymes | Number of enzyme-encoding genes |
---|---|---|---|
Amino acids | Alanine, aspartate & glutamate metabolism | 35 | 37 |
Glutathione metabolism | 31 | 57 | |
Arginine biosynthesis | 13 | 22 | |
Arginine and proline metabolism | 43 | 51 | |
Carbohydrates | Amino sugar | 44 | 48 |
Citrate cycle | 20 | 30 | |
Fructose and mannose metabolism | 23 | 33 | |
Glycolysis, gluconeogenesis | 43 | 67 | |
Pentose metabolism | 25 | 30 | |
Cofactors | Nicotinate and nicotinamide metabolism | 24 | 35 |
Riboflavin metabolism | 9 | 8 | |
Lipids | Fatty acid biosynthesis | 15 | 18 |
Glycerolipid metabolism | 33 | 61 | |
Sphingolipid metabolism | 32 | 49 | |
Nucleotides | Purine metabolism | 66 | 133 |
Pyrimidine metabolism | 34 | 57 |
We analyzed the WES data of the 522 patients represented in TCGA with LUAD for function-altering mutations in those 736 genes controlling cellular metabolism, and we identified 4,608 unique variants. The incidence of metabolic gene mutations was low (6.29%±0.25%), and the frequency of function-altering mutations was evenly distributed across superpathways (Figure 2A). However, pair-wise comparisons of pathway mutation frequencies did show some significant differences. The glutathione metabolism pathway (GSH), part of the amino acids superpathway, had a lowest mutation frequency than other pathways of amino acids superpathway (0.74% vs. 1.66% or 1.83%, P=0.0034 or P=0.0302 for alanine, aspartate and glutamate metabolism or arginine and proline metabolism, respectively). Mutation frequency of GSH was significantly lower than metabolic pathways of carbohydrates (0.74% vs. 1.05%; 1.30%; 1.13% and 1.26%; P=0.0305, P=0.0143, P=0.0131, P=0.0015 for amino sugar metabolism; citrate cycle, fructose and mannose metabolism or glycolysis, respectively), lower than fatty acid synthesis and glycerolipid metabolism (0.74% vs. 1.61% and 1.46%, P=0.0003 and P=0.0015 for fatty acid synthesis and glycerolipid metabolism, respectively). The frequency of mutations in GSH was also lower than the purine metabolism pathway (0.74% vs. 1.45%, P=0.0015) (Figure 2B). Only one gene was found mutated in more than 10% of patients—the gene encoding CPS1, part of the alanine, aspartate and glutamate metabolic pathway (amino acids superpathway). CPS1 is a mitochondrial enzyme catalyzing synthesis of carbamoyl phosphate from ammonia and bicarbonate.
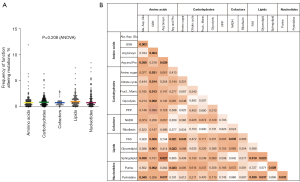
Metabolic gene mutations associated with OS in non-squamous NSCLC
We estimated the median follow up time to be 23.93 months for the 522 patients using the reverse Kaplan-Meier approach (16) (Table S1). Using clinical outcome data for these patients available through TCGA, we performed univariable analyses to determine whether any function-altering mutations in the 736 metabolic genes were associated with OS from TCGA LUAD cohort. Each gene was analyzed using a separate Cox regression model. Mutations in 42 genes were associated with OS (raw P<0.1) (Figure S2); 40 were associated with shorter OS (average HR =9.46, median HR =3.74, min–max 1.80–141.83), and two genes were associated with longer OS (PDE10A: HR =0.37, P=0.0866; GAD2: HR =0.30, P=0.0901). The mutation frequency for genes associated with OS was not significantly different from that of other metabolic genes (P=0.71; Figure S3).
We tested whether the 42 genes of clinical significance were expressed among tumors containing function altering mutations. All but one gene (GAD2) was expressed in non-squamous NSCLC tumors. Three metabolic genes (7.1%) were differentially expressed, each of them had higher transcript abundance estimate (i.e., transcripts per million estimated reads) in tumors containing function altering mutations compared with tumors with non-mutated metabolic genes: GBA (2,440±289 vs. 1,849±34); DGKG (150±25 vs. 97.1±4.1); GSS (1,653±204 vs. 1,215±20), all P<0.05 (Table S2).
To validate our data in a biological context, we further investigated whether the 42 clinically significant metabolic genes identified using our genomic TCGA cohort (Figure 3), were associated with metabolic alterations detected by mass spectrometry (MS) in the metabolomic studies (Figure 1). Using the KEGG ENZYME database we found that 34 of the 42 metabolic genes of clinical significance (81%) controlled non-squamous NSCLC metabolic alteration that was identified by MS (Figure 3, Figure S4). While 23 metabolic genes (54.8%) were associated with metabolic alterations detected in both metabolomic studies (10,11), there were eleven genes (26.2%) with metabolic alterations detected only in the Moreno study (11). The remaining 8 metabolic genes (19%), coding for 6 metabolic enzymes, did not match metabolomic studies findings (Figure 3). Lastly, among the 53 non-squamous NSCLC metabolic alterations detected by MS, 11 showed no association with metabolic genes of clinical significance (Figure 1, Figure S4).
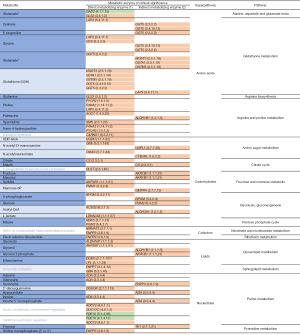
Ninety-three percent of the clinically significant metabolic genes in LUAD (38 of 42) were concentrated across three superpathways: amino acids, carbohydrates and nucleotides. Within the amino acids superpathway, non-silent mutations of genes controlling glutathione catabolism or proline levels (Figure S4B,S4D) were associated with shorter OS. Similarly, alterations to glucose, fructose and mannose metabolism (carbohydrates superpathway, Figure S4G-S4H) and uric acid biosynthesis (nucleotides superpathway, Figure S4O) were also associated with shorter survival. Two metabolic alterations were associated with longer OS: glutamate/glutamine biosynthesis (glutamate decarboxylase 2, GAD2; Figure S4A) and cAMP/cGMP hydrolysis (phosphodiesterase 10A, PDE10A, Figure S4O). The fourteen metabolites that were not associated with clinical significance were evenly distributed between the five superpathways (Figure S4) (11).
Independent association of metabolic alterations in non-squamous NSCLC with shorter OS
To measure the relative association of each metabolic superpathway with OS, we grouped the 40 metabolic genes associated with shorter survival (Figure S2) as follows: amino acids (14 genes), carbohydrates (15 genes), cofactors (two genes), lipids (6 genes) and nucleotides (7 genes). Four genes were shared across more than one superpathway (Figure 3).
We first tested the effect of gender (male vs. female), smoking status (non-smoking vs. smoking) and disease stage (early vs. late stage) on the frequency of mutated metabolic genes in the genomic TCGA LUAD cohort, grouping clinically significant genes by superpathways. We found that smoking status significantly impacted mutation frequency of clinically relevant genes controlling amino acid and nucleotide metabolic superpathway (Table S3). In both superpathways, smokers had almost double the mutation frequency of non-smokers: [amino acid 19.2% vs. 10.8% (P=0.027)] and [nucleotide 20.9% vs. 10.0% (P=0.004)]. We then tested the impact of the aforementioned demographic and clinical variables on clinical outcome and found that patients presenting with later stage disease had a worse overall clinical outcome (P<0.0001). No differences were observed among the other variables. On the basis of these results, we adjusted our model for gender, age, smoking status, disease stage, and therapy modality (chemotherapy and radiation therapy). Interaction terms were not considered in this analysis. After controlling for confounding factors, we found that carbohydrate metabolism had the biggest effect on OS (HR =5.21; 95% CI: 3.27 to 8.29; FDR-P<0.0001) followed by lipids (HR =4.05; 95% CI: 2.55 to 6.69; FDR-P<0.0001), cofactors (HR =4.00; 95% CI: 1.90 to 8.45; FDR-P=0.0003), amino acids (HR =3.35; 95% CI: 2.13 to 5.26; FDR-P<0.0001), and nucleotides (HR =2.58; 95% CI: 1.60 to 4.16; FDR-P=0.0001; Table 2).
Table 2
Metabolic superpathway | HR | 95% CI | P value | FDR-P value |
---|---|---|---|---|
Amino acids | 3.346 | 2.129–5.258 | <0.0001 | <0.0001 |
Carbohydrates | 5.208 | 3.272–8.291 | <0.0001 | <0.0001 |
Cofactors | 4.003 | 1.896–8.449 | 0.0003 | 0.0003 |
Lipids | 4.054 | 2.547–6.690 | <0.0001 | <0.0001 |
Nucleotides | 2.578 | 1.598–4.159 | 0.0001 | 0.0001 |
Forty genes that were associated with lower OS were grouped into five metabolic superpathways. The HR +/− 95% CI, P value and FDR corrected P value (FDR-P value) for each clinically relevant metabolic superpathway in lung adenocarcinoma cohort from the TCGA database. HR, hazard ratio; CI, confidence interval; FDR, false discovery rate; OS, overall survival; TCGA, The Cancer Genome Atlas.
Compared with the two metabolomic cohorts, the genomic TCGA LUAD cohort is comprised of a heterogeneous NSCLC patient population (Table S1). Most patients (77%) had early-stage disease (I–II). Fifty-four percent received chemotherapy only and 31% patients received radiation therapy only. For both treatment groups, patients with early-stage disease had more favorable clinical outcomes than those with later stage disease. For patients treated with chemotherapy only, the median survival time for early stage disease was 103.9 months, and for later stage disease was 41.5 months. For patients treated with radiation therapy only, the median survival time for early stage disease was 76.0 months, and for later stage disease was 15.0 months.
To examine whether metabolic superpathway dysfunction may have differentially impacted clinical outcomes by disease stage, we added the pathway × stage interaction effect to the multivariable Cox regression model. We conducted three separate analyses by patients groups: all patients, patients who received chemotherapy only, and patients who received radiation therapy only). The pathway × stage interaction effect was not significant for any of the superpathways in these analyses; P values ranged from 0.23 to 0.99. Similarly, pathway × treatment (chemotherapy or radiation therapy) interaction effect was also not significant, with P values ranging from 0.61 to 0.92. Confidence intervals for hazard ratios overlaped between early stages (I–II) and late stages (III–IV), and between different treatment types (Figure S5). Taken together, dysfunction of individual metabolic superpathways (amino acids, carbohydrates, nucleotides, lipids or cofactors) was associated with OS independently of disease stage and treatment modality.
Discussion
In this study, we sought to assess whether metabolic alterations in non-squamous NSCLC are a byproduct of accelerating cell growth or whether they may be a driving factor of tumor biology. Building on consensus findings from MS-based metabolomics studies, we developed a post-genomics research strategy to assess the clinical impact of metabolic alterations in non-squamous NSCLC. We specifically focused on five metabolic superpathways (amino acids, carbohydrates, cofactors, lipids, and nucleotides), reproducibly found altered in LUAD across metabolomic studies (10,11). Using KEGG PATHWAY and KEGG ENZYME databases to identify metabolic genes controlling those metabolic superpathways, we queried the TCGA LUAD genomic cohort for function-altering mutations affecting those genes (KEGG-TCGA analysis), as a surrogate marker for metabolic alterations. While this approach allowed us to probe the association between clinical outcomes and specific metabolic functions in large and clinically annotated cohorts, it is not without limitations. It only provides an indirect assessment of metabolic functions in lung cancer and is restricted to metabolic genes presenting with function-altering mutations. As a result, the overall frequency of metabolic gene mutations may introduce a selection bias and diminished statistical power. Nonetheless, the metabolic genes we identified as clinically relevant did not present with a higher frequency of mutations or mutation severity score (SIFT and PolyPhen) in studied cohort.
The TCGA LUAD genomic cohort data has several important features that we were able to leverage in our study. The data represents a large sample size, extensive clinical annotation with almost 2 years of clinical follow-up and includes comprehensive genomic data. While it provided us with a valuable hypothesis-generating dataset, we also acknowledge that it bears some limitations as it lacks extensive proteomic and metabolomic data and a matching validation cohort of comparable size, annotations, and length of clinical follow-up. In the context of metabolic studies, these limitations are compounded by the fact that a complex network of endogenous and exogenous factors regulates cellular metabolomic activity (17). We demonstrated that 41 of 42 metabolic genes of clinical significance were indeed expressed in non-squamous NSCLC tumors. However, our genomic data do not to account for post-translational modification and metabolic activity regulation, for example, protein folding or stability, protein phosphorylation/acetylation/ubiquitination, substrate and cofactor availability, enzymatic activity overlap between metabolic enzyme isoforms or interplay between anabolic and catabolic enzymes. To overcome such limitations, we employed a biological validation approach in which we paired genomic data from the TCGA LUAD cohort with metabolomic data derived from two independent non-squamous NSCLS cohorts (8,9). We showed that 81% of clinically relevant genes (34 of 42) controlled anabolism or catabolism of altered metabolites in non-squamous NSCLC (converging of KEGG-TCGA and MS finding). In the context of our analytical input, where only 176 out of 736 (23.9%) metabolic genes tested directly controlled non-squamous NSCLC metabolic alterations, such high level of overlap (81%) between genomic and metabolomic findings suggests a non-stochastic distribution of metabolic genes of clinical significance. Eleven non-squamous NSCLC metabolic alterations were unrelated to metabolic functions of clinical significance (MS findings only) and could be a byproduct of accelerating cell growth.
We found that clusters of genes controlling key metabolic functions of the carbohydrates metabolic superpathway had the most profound impact on OS. For instance, alterations in genes controlling glucose and fructose catabolism, two carbohydrates and key components of the Warburg effect, were associated with shorter OS. Metabolomic studies and clinical PET-CT examinations have previously shown that lung tumors exhibit hyperactive glucose metabolism (18,19). Lung tumors are dependent on glucose metabolism, and increased expression of glycolytic enzymes correlates with poor prognosis (20). In an unselected cohort of 342 patients with newly diagnosed NSCLC, patients with high levels of glucose in blood had shorter OS than those with normal glucose levels, independently of other prognostic factors (21). However, while hyperglycemia decreases the antiproliferative effect of chemotherapy in preclinical models, glycemic control interventions have shown inconsistent results in clinical trials (20). Beyond the Warburg effect, we found that alterations in mannose metabolism, a key carbohydrate in glycosylation (22), were also associated with shorter OS. Consistent with our results, high mannose-type glycans are prevalent in LUAD tissues (23) and are associated with disease progression (24).
Alterations to three additional gene clusters that control glutathione catabolism, proline synthesis (amino acids superpathway), and uric acid anabolism (nucleotides superpathway) were also associated with worse OS. The frequency of metabolic genes mutations controlling both amino acid and nucleotide superpathways was higher among smokers than non-smokers. Smoking is a known risk factor in NSCLC and is associated with poor clinical outcomes (25). In our model that was adjusted for variables such as smoking status, alterations in amino acid and nucleotide superpathways were independently associated with clinical outcome. In a hydrophilic environment, both glutathione and uric acid are antioxidants, acting as scavengers of free radicals produced by reactive oxygen species. Higher glutathione levels have been associated with resistance to both chemotherapy and radiation therapy (26,27). However, conflicting data in the literature supports the role of uric acid in cancer. Results of a study involving 354,110 participants showed that serum uric acid was cancer-protective and reduced risk cancer mortality (28), while data from another study involving 83,693 patients showed that high levels of serum uric acid were associated with a higher risk of all cancer mortality (29). In our study, alterations to genes controlling uric acid precursors anabolism was associated with shorter OS, suggesting that uric acid may indeed be harmful in non-squamous NSCLC. Similarly, our data showed that alterations to the biosynthesis of proline (i.e., PYCR2, P4HA2, and LAP3), a non-essential amino acid involved in cancer cell redox homeostasis (30,31) was associated with shorter OS. Overexpression of PYCR2 and P4HA2 has been described in many malignancies, including lung cancer (32,33). Conflicting with our findings, preclinical data attributed a pro-tumorigenic role to both genes in epithelial cancers (34,35).
While most of the metabolic genes that we identified as clinically relevant were associated with shorter OS, alterations to two genes were associated with improved OS: GAD2 and PDE10. Both metabolic genes are typically expressed in the brain and central nervous system but their ectopic expression in tumor types such as lung tumors has been described (36,37). Only PDE10 ectopic expression in non-squamous NSCLC tumors was confirmed in our study, GAD2 transcripts were not detected. GAD exists as two isoforms, GAD1 and GAD2, that shares enormous sequence homology at the protein level, but have different affinity for the cofactor pyridoxal 5’phosphate and distinct intracellular localization. Evidence suggest the presence of alternative splicing of GAD1 and a functional truncated version of GAD2 (38). From analyses of the LUAD TCGA data, we observed that GAD1 mRNA expression was a 5-fold increase in GAD1 mRNA expression within GAD2 mutated tumor compared with non-mutated (313.2±47.4 vs. 63.6±5, P<0.0001). GAD1/GAD2 catalyzes decarboxylation of glutamate to γ-aminobutyric acid (GABA), and PDE10A regulates various neurotransmitter receptors, including dopamine D1 and D2 (39,40). While emerging data suggest that cancer cells take advantage of the neurotransmitters-initiated signaling pathway to activate proliferation and tumor progression (41), both GABA and dopamine mostly activate cancer growth (42,43). In addition to regulating transmitter receptors, PDE10 also hydrolyzes cGMP, a strong antineoplastic agent across several cancer types (44). While our data cannot resolve those contradictory roles for GAD1-2/PDE10 in tumor biology, it appeared that function-altering mutations of those genes were associated with improved clinical outcomes for the cohort of patients that we studied. As such, synthetic inhibitors of those two metabolic enzymes, some of which are currently in development (45,46), may warrant further investigation for the treatment of non-squamous NSCLC.
This study presents a novel post-genomic research strategy for identifying metabolic alterations associated with clinical outcomes in non-squamous NSCLC. We identified a strong consensus between cancer metabolism data obtained by MS methods and clinically annotated genomics data. By providing a detailed map of the landscape of metabolic alterations in non-squamous NSCLC, our findings may offer valuable insights into the biology of the disease and enable the identification of new therapeutic targets to overcome resistance to chemotherapy and radiation therapy. Additional clinical and functional studies will be warranted to validate those finding and determine the actionability of those metabolic alterations.
Acknowledgments
Scientific writing and editing support were provided by Hazel F O’Connor, PhD, on behalf of Levine Cancer Institute. The results shown here are in part based upon data generated by the TCGA Research Network: https://www.cancer.gov/tcga.
Funding: This work was supported by the North Carolina Lung Cancer Initiative Research Fellow Grant to AV Ivanina Foureau.
Footnote
Reporting Checklist: The authors have completed the STARD reporting checklist. Available at https://tlcr.amegroups.com/article/view/10.21037/tlcr-22-377/rc
Conflicts of Interest: All authors have completed the ICMJE uniform disclosure form (available at https://tlcr.amegroups.com/article/view/10.21037/tlcr-22-377/coif). AVIF reports that this work was supported by North Carolina Lung Cancer Initiative Research Fellow grant. The other authors have no conflicts of interest to declare.
Ethical Statement: The authors are accountable for all aspects of the work in ensuring that questions related to the accuracy or integrity of any part of the work are appropriately investigated and resolved. The study was conducted in accordance with the Declaration of Helsinki (as revised in 2013).
Open Access Statement: This is an Open Access article distributed in accordance with the Creative Commons Attribution-NonCommercial-NoDerivs 4.0 International License (CC BY-NC-ND 4.0), which permits the non-commercial replication and distribution of the article with the strict proviso that no changes or edits are made and the original work is properly cited (including links to both the formal publication through the relevant DOI and the license). See: https://creativecommons.org/licenses/by-nc-nd/4.0/.
References
- Pavlova NN, Zhu J, Thompson CB. The hallmarks of cancer metabolism: Still emerging. Cell Metab 2022;34:355-77. [Crossref] [PubMed]
- Zito Marino F, Ronchi A, Accardo M, et al. Concomitant ALK/KRAS and ALK/EGFR mutations in non small cell lung cancer: different profile of response to target therapies. Transl Cancer Res 2017;6:S457-S460. [Crossref]
- Wang M, Herbst RS, Boshoff C. Toward personalized treatment approaches for non-small-cell lung cancer. Nat Med 2021;27:1345-56. [Crossref] [PubMed]
- Jin N, Bi A, Lan X, et al. Identification of metabolic vulnerabilities of receptor tyrosine kinases-driven cancer. Nat Commun 2019;10:2701. [Crossref] [PubMed]
- Majem B, Nadal E, Muñoz-Pinedo C. Exploiting metabolic vulnerabilities of Non small cell lung carcinoma. Semin Cell Dev Biol 2020;98:54-62. [Crossref] [PubMed]
- Mendes C, Serpa J. Metabolic Remodelling: An Accomplice for New Therapeutic Strategies to Fight Lung Cancer. Antioxidants (Basel) 2019;8:603. [Crossref] [PubMed]
- Luengo A, Gui DY, Vander Heiden MG. Targeting Metabolism for Cancer Therapy. Cell Chem Biol 2017;24:1161-80. [Crossref] [PubMed]
- Li X, Song H, Kong F, et al. Pemetrexed exerts anticancer effects by inducing G(0)/G(1)-phase cell cycle arrest and activating the NOXA/Mcl-1 axis in human esophageal squamous cell carcinoma cells. Oncol Lett 2019;17:1851-8. [PubMed]
- Wu HL, Gong Y, Ji P, et al. Targeting nucleotide metabolism: a promising approach to enhance cancer immunotherapy. J Hematol Oncol 2022;15:45. [Crossref] [PubMed]
- Wikoff WR, Grapov D, Fahrmann JF, et al. Metabolomic markers of altered nucleotide metabolism in early stage adenocarcinoma. Cancer Prev Res (Phila) 2015;8:410-8. [Crossref] [PubMed]
- Moreno P, Jiménez-Jiménez C, Garrido-Rodríguez M, et al. Metabolomic profiling of human lung tumor tissues - nucleotide metabolism as a candidate for therapeutic interventions and biomarkers. Mol Oncol 2018;12:1778-96. [Crossref] [PubMed]
- Rocha CM, Barros AS, Goodfellow BJ, et al. NMR metabolomics of human lung tumours reveals distinct metabolic signatures for adenocarcinoma and squamous cell carcinoma. Carcinogenesis 2015;36:68-75. [Crossref] [PubMed]
- Kanehisa M. Enzyme Annotation and Metabolic Reconstruction Using KEGG. Methods Mol Biol 2017;1611:135-45. [Crossref] [PubMed]
- Kanehisa M, Goto S. KEGG: kyoto encyclopedia of genes and genomes. Nucleic Acids Res 2000;28:27-30. [Crossref] [PubMed]
- Li B, Dewey CN. RSEM: accurate transcript quantification from RNA-Seq data with or without a reference genome. BMC Bioinformatics 2011;12:323. [Crossref] [PubMed]
- Schemper M, Smith TL. A note on quantifying follow-up in studies of failure time. Control Clin Trials 1996;17:343-6. [Crossref] [PubMed]
- Zapalska-Sozoniuk M, Chrobak L, Kowalczyk K, et al. Is it useful to use several "omics" for obtaining valuable results? Mol Biol Rep 2019;46:3597-606. [Crossref] [PubMed]
- Feinberg T, Alkoby-Meshulam L, Herbig J, et al. Cancerous glucose metabolism in lung cancer-evidence from exhaled breath analysis. J Breath Res 2016;10:026012. [Crossref] [PubMed]
- Tan H, Ma M, Huang J, et al. Preoperative Body Composition Combined with Tumor Metabolism Analysis by PET/CT Is Associated with Disease-Free Survival in Patients with NSCLC. Contrast Media Mol Imaging 2022;2022:7429319. [Crossref] [PubMed]
- Gerards MC, van der Velden DL, Baars JW, et al. Impact of hyperglycemia on the efficacy of chemotherapy-A systematic review of preclinical studies. Crit Rev Oncol Hematol 2017;113:235-41. [Crossref] [PubMed]
- Luo J, Chen YJ, Chang LJ. Fasting blood glucose level and prognosis in non-small cell lung cancer (NSCLC) patients. Lung Cancer 2012;76:242-7. [Crossref] [PubMed]
- Nan F, Sun Y, Liang H, et al. Mannose: A Sweet Option in the Treatment of Cancer and Inflammation. Front Pharmacol 2022;13:877543. [Crossref] [PubMed]
- Ruhaak LR, Taylor SL, Stroble C, et al. Differential N-Glycosylation Patterns in Lung Adenocarcinoma Tissue. J Proteome Res 2015;14:4538-49. [Crossref] [PubMed]
- Lattová E, Skřičková J, Hausnerová J, et al. N-Glycan profiling of lung adenocarcinoma in patients at different stages of disease. Mod Pathol 2020;33:1146-56. [Crossref] [PubMed]
- Bade BC, Dela Cruz CS. Lung Cancer 2020: Epidemiology, Etiology, and Prevention. Clin Chest Med 2020;41:1-24. [Crossref] [PubMed]
- Estrela JM, Ortega A, Obrador E. Glutathione in cancer biology and therapy. Crit Rev Clin Lab Sci 2006;43:143-81. [Crossref] [PubMed]
- Kennedy L, Sandhu JK, Harper ME, et al. Role of Glutathione in Cancer: From Mechanisms to Therapies. Biomolecules 2020.
- Kuo CF, See LC, Yu KH, et al. Significance of serum uric acid levels on the risk of all-cause and cardiovascular mortality. Rheumatology (Oxford) 2013;52:127-34. [Crossref] [PubMed]
- Strasak AM, Rapp K, Hilbe W, et al. Serum uric acid and risk of cancer mortality in a large prospective male cohort. Cancer Causes Control 2007;18:1021-9. [Crossref] [PubMed]
- Hollinshead KER, Munford H, Eales KL, et al. Oncogenic IDH1 Mutations Promote Enhanced Proline Synthesis through PYCR1 to Support the Maintenance of Mitochondrial Redox Homeostasis. Cell Rep 2018;22:3107-14. [Crossref] [PubMed]
- Schwörer S, Berisa M, Violante S, et al. Proline biosynthesis is a vent for TGFβ-induced mitochondrial redox stress. EMBO J 2020;39:e103334. [Crossref] [PubMed]
- Nilsson R, Jain M, Madhusudhan N, et al. Metabolic enzyme expression highlights a key role for MTHFD2 and the mitochondrial folate pathway in cancer. Nat Commun 2014;5:3128. [Crossref] [PubMed]
- Hu D, Ansari D, Zhou Q, et al. Low P4HA2 and high PRTN3 expression predicts poor survival in patients with pancreatic cancer. Scand J Gastroenterol 2019;54:246-51. [Crossref] [PubMed]
- Ou R, Zhang X, Cai J, et al. Downregulation of pyrroline-5-carboxylate reductase-2 induces the autophagy of melanoma cells via AMPK/mTOR pathway. Tumour Biol 2016;37:6485-91. [Crossref] [PubMed]
- Ding Z, Ericksen RE, Escande-Beillard N, et al. Metabolic pathway analyses identify proline biosynthesis pathway as a promoter of liver tumorigenesis. J Hepatol 2020;72:725-35. [Crossref] [PubMed]
- Dade M, Berzero G, Izquierdo C, et al. Neurological Syndromes Associated with Anti-GAD Antibodies. Int J Mol Sci 2020;21:3701. [Crossref] [PubMed]
- Zhu B, Lindsey A, Li N, et al. Phosphodiesterase 10A is overexpressed in lung tumor cells and inhibitors selectively suppress growth by blocking β-catenin and MAPK signaling. Oncotarget 2017;8:69264-80. [Crossref] [PubMed]
- Trifonov S, Yamashita Y, Kase M, et al. Glutamic acid decarboxylase 1 alternative splicing isoforms: characterization, expression and quantification in the mouse brain. BMC Neurosci 2014;15:114. [Crossref] [PubMed]
- Al-Wadei HA, Al-Wadei MH, Schuller HM. Cooperative regulation of non-small cell lung carcinoma by nicotinic and beta-adrenergic receptors: a novel target for intervention. PLoS One 2012;7:e29915. [Crossref] [PubMed]
- Niccolini F, Haider S, Reis Marques T, et al. Altered PDE10A expression detectable early before symptomatic onset in Huntington's disease. Brain 2015;138:3016-29. [Crossref] [PubMed]
- Jiang SH, Hu LP, Wang X, et al. Neurotransmitters: emerging targets in cancer. Oncogene 2020;39:503-15. [Crossref] [PubMed]
- Liang Y, Li H, Gan Y, et al. Shedding Light on the Role of Neurotransmitters in the Microenvironment of Pancreatic Cancer. Front Cell Dev Biol 2021;9:688953. [Crossref] [PubMed]
- Qian X, Zhang D, Cao Z, et al. Dopamine Pathway Mediated by DRD5 Facilitates Tumor Growth via Enhancing Warburg Effect in Esophageal Cancer. Front Oncol 2021;11:655861. [Crossref] [PubMed]
- Tuttle TR, Mierzwa ML, Wells SI, et al. The cyclic GMP/protein kinase G pathway as a therapeutic target in head and neck squamous cell carcinoma. Cancer Lett 2016;370:279-85. [Crossref] [PubMed]
- Choi YK, Park KG. Targeting Glutamine Metabolism for Cancer Treatment. Biomol Ther (Seoul) 2018;26:19-28. [Crossref] [PubMed]
- Amin HS, Parikh PK, Ghate MD. Medicinal chemistry strategies for the development of phosphodiesterase 10A (PDE10A) inhibitors - An update of recent progress. Eur J Med Chem 2021;214:113155. [Crossref] [PubMed]